Accelerometer-Measured Sedentary Behavior Patterns, Brain Structure, and Cognitive Function in Dementia-Free Older Adults: A Population-Based Study
Abstract
Background:
Sedentary behavior is associated with cognitive impairment, but the neuropathological mechanisms underlying their associations are poorly understood.
Objective:
To investigate the associations of accelerometer-measured sedentary behavior patterns with brain structure and cognition, and further to explore the potential mechanisms.
Methods:
This community-based study included 2,019 older adults (age≥60 years, 59% women) without dementia derived from participants in the baseline examination of MIND-China (2018–2020). We assessed sedentary parameters using an accelerometer and cognitive function using a neuropsychological test battery. Structural brain markers were assessed on the structural brain MRI scans in a subsample (n = 1,009). Data were analyzed using the general linear, isotemporal substitution, and mediation models.
Results:
In the total sample (n = 2,019), adjusting for multiple covariates and moderate-to-vigorous-intensity physical activity, longer mean sedentary bout duration was linearly related with lower z-scores of global cognition, verbal fluency, and memory (ptrend < 0.05), whereas greater total sedentary time was linearly associated with lower z-scores of global cognition, verbal fluency, and memory only among individuals with long sedentary time (>10 h/day) (ptrend < 0.05); Breaking up sedentary time with same amount of light-intensity physical activity was significantly associated with higher verbal fluency and memory z-scores (p < 0.05). In the MRI subsample (n = 1,009), separately entering structural brain MRI markers into the mediation models substantially attenuated the associations of mean sedentary bout duration with global cognition, verbal fluency, and memory z-scores.
Conclusion:
Prolonged uninterrupted sedentary time is associated with poor global cognition, memory, and verbal fluency among rural older adults, and structural brain markers could partially mediate the association.
INTRODUCTION
Sedentary behavior is defined as any waking behavior characterized by an energy expenditure≤1.5 metabolic equivalents in a sitting, reclining, or lying posture [1]. Sedentary behavior has been associated with various adverse health outcomes such as obesity, diabetes, cardiovascular disease, and mortality [2, 3]. Systematic reviews supported the association of sedentary behavior with cognitive impairment and dementia [4, 5]. However, most of the previous studies had relied on self-reported sedentary behavior, in which the findings might be affected by recall bias [6, 7].
Previously, accelerometer-measured total sedentary time has been associated with poor cognitive function in some studies [8–10], but not in others [11, 12]. Apart from total sedentary time, patterns of sedentary behavior, such as average duration of prolonged sedentary time (sedentary bouts) and frequency of interrupted sedentary time (sedentary breaks), appear to be related to cognitive outcomes. The randomized controlled trials showed that replacing prolonged sitting with short bouts of light-to-moderate intensity activity could promote postprandial glucose metabolism [13, 14], suggesting that both the total sedentary time and the manner in which it is accumulated are associated with health outcomes. However, population-based studies have rarely explored the association between accruing sitting time in prolonged, uninterrupted bouts and cognitive function, especially among rural-dwelling older adults, who usually have lower education, poorer socioeconomic status, and worse lifestyles compared with urban populations.
The potential mechanisms linking sedentary behavior with cognitive function are poorly understood. Previous studies have suggested that less sedentary time (or more physical activity) is associated with fewer brain lesions such as brain atrophy and white matter hyperintensities (WMHs) [15, 16]. In addition, the extent of brain atrophy and WMHs has been associated with accelerated cognitive impairment and dementia in middle-aged and older adults [17, 18]. Therefore, a conceivable hypothesis could be that structural brain properties may mediate, at least partly, the link of sedentary behavior with cognitive phenotypes.
In this community-based study of Chinese rural older adults, we sought to examine the dose-response associations of objectively measured total sedentary time and mean sedentary bout duration with global and domain-specific cognitive function, and further to explore the extent to which their associations with cognition are mediated by structural brain markers. We hypothesize that sedentary parameters are associated with poor cognitive outcomes, and that structural brain markers might partially mediate these associations.
METHODS
Participants
This population-based cross-sectional study used data from the baseline survey of the randomized controlled Multimodal INterventions to delay Dementia and disability in rural China (MIND-China), as previously described [19]. In August 2018-December 2020, a cluster (village)-based subsample (n = 2382) who were free of dementia and major mental health problems derived from the participants of MIND-China took part in the ActiGraph substudy [20]. Of these, 363 persons were excluded due to < 3 valid days of wearing the accelerometer (n = 281) and missing cognitive data (n = 82), leaving 2019 persons for the analysis. Of these, structural brain MRI data were available in 1009 participants. Supplementary Figure 1 shows the flowchart of study participants.
The Ethics Committee of Shandong Provincial Hospital approved the MIND-China protocol. Written informed consent was obtained from all participants. MIND-China was registered in the Chinese Clinical Trial Registry (registration no.: ChiCTR1800017758).
Data collection and definitions
Data on demographics, lifestyles, medical history (e.g., coronary heart disease, diabetes, and stroke), and use of medications were collected through the face-to-face interview, neuropsychological testing, clinical examination, and laboratory tests [19]. Alcohol drinking and smoking status were categorized as never, former, and current drinking or smoking. Body mass index (BMI) and arterial blood pressure were measured as previously described [19]. Hypertension, diabetes, and dyslipidemia was defined as previously described [21]. The APOE genotype was measured using multiple-polymerase chain reaction amplification and was divided into any ɛ4 allele versus no ɛ4 allele [22].
ActiGraph data collection and processing
Participants wore a triaxial accelerometer ActiGraph wGT3X-BT (ActiGraph, LLC, Pensacola, FL) on their hip, to affix to an elastic belt during all waking hours for 7 consecutive days, and only to remove it for swimming or bathing [20]. Wear season, accelerometer parameters, and non-wear time were defined as previously described [20]. We used data from participants who wore the device for≥10 h/day for≥3 days [23]. We defined sedentary behavior, light-intensity physical activity (LPA), and moderate-to-vigorous-intensity physical activity (MVPA) as counts per minute of 0–99, 100–1951, and≥1952, respectively [24].
Definitions of sedentary behavior parameters
All sedentary accumulated metrics were assessed based on the most frequently used measure of sedentary time, defined as having < 100 counts per minute recorded on the vertical axis [20]. Total sedentary time was defined as the average time (minutes) per day spent in sedentary bout for≥1 min. A sedentary bout was defined as consecutive time (minutes) recorded on the accelerometer in which counts per minute were < 100. Mean sedentary bout duration was calculated using data from all valid days by dividing total sedentary time (minutes) by the total number of sedentary bouts. Lower bout durations indicate interrupted patterns, whereas higher bout durations indicate more accumulation patterns. We also assessed the prolonged sedentary time (time spent in sedentary bouts≥30 min) and the number of breaks per sedentary hour (a break was defined as≥1 min of non-sedentary bout after a sedentary bout).
Neuropsychological test
The neuropsychological test battery was used to evaluate memory, attention, verbal fluency, and executive function [19, 25]. Specifically, memory was assessed with the Auditory Verbal Learning Test that included learning, delayed recall, and delayed recognition. Attention was assessed with the Trail Making Test-A and the sum of the Digit Span Forward. Verbal fluency was measured with the Verbal Fluency Test, including fruit, animal, and vegetable categories. Executive function was assessed with the Trail Making Test-B and Digit Span Backwards. The composite z-score for a specific cognitive domain was calculated by averaging the z-scores of relevant neuropsychological tests within the domain [26]. The composite z-score for global cognitive function was estimated by averaging the composite z-scores across the four cognitive domains.
MRI acquisition and data processing
All eligible participants were scanned on either the Philips Archiva 3.0T MR System in the Liaocheng People’s Hospital (n = 95) or the Philips Ingenia 3.0T MR System in the Southwestern Lu Hospital (n = 914) [19].
For image processing, 3D T1-weighted images was used to quantify the brain volumes. We segmented brain volumes using the Computational Anatomy Toolbox in Matlab (http://dbm.neuro.uni-jena.de/cat12/) [27]. The Computational Anatomy Toolbox used a standard brain template of Montreal Neurological Institute to automatically normalize the skull-stripping brain, estimate the total intracranial volumes (TIV), and segment brain tissues into gray matter and white matter based on the Adaptive Maximum A Posteriori technique [28, 29]. Then, we extracted the bilateral hippocampal gray matter volumes using the Hammers atlas [30]. In addition, the T1 and T2-FLAIR images were processed in AccuBrain® (BrainNow Medical Technology Ltd., Shenzhen, Guangdong, China) to acquire the volume of ventricles and WMH, respectively, as previously reported [30, 31]. In brief, AccuBrain® automatically estimated the TIV using the normalized T1-weighted images. Subsequently, AccuBrain® used the pre-defined normalized brain mask to segment brain tissue on T1-weighted image and then automatically estimated the ventricular volumes. Besides, AccuBrain® used T2-FLAIR images to calculate WMH as previously described [32]. All segmentations were visually checked for accuracy, and segmentation failures were excluded from analysis or corrected if possible. WMH volume was log transformed owing to skewed distribution [32].
Statistical analysis
General linear models were used to analyze the associations of sedentary parameters with cognitive z-scores. We reported the main results from three models: Model 1 was adjusted for demographics, APOE ɛ4 allele, MRI scanner, and accelerometer wear-season; Model 2 was additionally adjusted for lifestyle, cardiometabolic factors, coronary heart disease, and stroke; and MVPA was added in Model 3. The mediation role was assessed using the bootstrapping regression models adjusting for demographics and TIV. To account for correlation between total sedentary time and accelerometer wear-time, we standardized total sedentary time to 16 h of wear time per day using the residuals methods [33, 34].
The dose-response associations of cognitive z-scores with total sedentary time and mean sedentary bout duration were examined using restricted cubic spline functions [35]. We reported the results from models using 3 knots (10th, 50th, and 90th percentiles) for total sedentary time and 4 knots (5th, 35th, 65th, and 95th percentiles) for mean sedentary bout duration. The spline models were used to describe the nonlinear associations, and when the association was linear, the general linear model was used.
We used compositional isotemporal substitution model to estimate the associations with cognitive z-scores of replacing sedentary behavior variable with physical activity variable [36]. This model was performed using the R packages “Compositions”, “robCompositions”, and “lmtest”. Briefly, the compositional averages of daily sedentary behavior, LPA, and MVPA across the recording period were normalized to the proportion of 960 min (16 h). We examined how replacing 1 to 60 min of total sedentary time with other types of behavior was associated with cognitive z-scores. We estimated replacements up to 60 min to align with previous studies of mental health that used the compositional methods [37]. All the compositional isotemporal substitution models were adjusted for age, sex, education, APOE ɛ4 allele, accelerometer wear-season, smoking, alcohol consumption, BMI, stroke, coronary heart disease, diabetes, hypertension, and dyslipidemia.
Stata Statistical Software: Release 15 (StataCorp LLC., College Station, TX, USA) or R (R Foundation for Statistical Computing; Vienna, Austria) or IBM SPSS version 26.0 for Windows (IBM SPSS Inc., Chicago, IL, USA) was used for all analyses.
RESULTS
3.1Characteristics of study participants
Of the 2019 participants, the mean age was 69.9 (SD = 4.7) years, 58.9% were women, and 36.9% had no formal schooling (Table 1). Overall, participants spent 58.4% of daily waking time in sedentary behavior, 40.9% in LPA, and only 1.5% in MVPA.
Table 1
Characteristic of study participants
Characteristics | Total sample |
(n = 2,019) | |
Age (y) | 69.9 (4.7) |
Sex (female), n (%) | 1190 (58.9) |
Education, n (%) | |
No formal schooling | 746 (36.9) |
Primary school | 881 (43.6) |
Middle school or above | 392 (19.4) |
Current smoking, n (%) | 390 (19.3) |
Alcohol assumption, n (%) | |
Never | 1,233 (61.1) |
Former | 160 (7.9) |
Current | 626 (31.0) |
Body mass index (kg/m2) | 25.1 (3.7) |
Hypertension, n (%) | 1,375 (68.1) |
Diabetes, n (%) | 305 (15.1) |
Dyslipidemia, n (%) | 464 (23.0) |
Stroke, n (%) | 274 (13.6) |
Coronary heart disease, n (%) | 434 (21.5) |
APOE ɛ4 allele, n (%) | 294 (14.6) |
Daily wear time, min | 846.9 (80.8) |
% in Sedentary behavior | 58.4 (13.4) |
% in LPA | 40.2 (12.5) |
% in MVPA | 1.5 (1.9) |
Mean sedentary bout duration, minutes/bout | 11.9 (6.5) |
Data are mean (standard deviation), unless otherwise specified. SD, standard deviation; MRI, magnetic resonance imaging; APOE, apolipoprotein E gene; LPA, light-intensity physical activity; MVPA, moderate-to-vigorous-intensity physical activity.
Sedentary behavior and cognitive function
In the ActiGraph sample (n = 2,019), adjusting for demographics, APOE ɛ4, and accelerometer wear-reason, greater total sedentary time was significantly associated with lower z-scores of memory and verbal fluency, but not with global cognition, attention, and executive function. The associations remained significant after adjusting for additional covariates and MVPA (Table 2). Restricted cubic spline regression analysis suggested non-linear relationships of total sedentary time with global cognition, memory, and verbal fluency z-scores (all p-nonlinear < 0.05, Fig. 1A). The restricted cubic splines showed a linear association of total sedentary time with the cognitive z-scores after certain thresholds of total sedentary time. In addition, longer mean sedentary bout duration was linearly associated with lower z-scores of global cognition, memory, and verbal fluency (all p-nonlinear > 0.05, Fig. 1B).
Table 2
Association of sedentary parameters with cognitive function (n = 2019)
Cognitive function (outcomes) | β coefficient (95% confidence interval), cognitive z-score | ||
Model 1b | Model 2b | Model 3b | |
Global cognition | |||
Total sedentary timea | –0.011 (–0.023, 0.001) | –0.008 (–0.021, 0.004) | –0.008 (–0.022, 0.006) |
Mean sedentary bout duration | –0.007 (–0.011, –0.003)‡ | –0.006 (–0.010, –0.002)† | –0.006 (–0.010, –0.002)† |
Memory | |||
Total sedentary timea | –0.034 (–0.053, –0.016)‡ | –0.029 (–0.047, –0.010)† | –0.029 (–0.050, –0.007)† |
Mean sedentary bout duration | –0.012 (–0.018, –0.006)‡ | –0.009 (–0.016, –0.003)† | –0.009 (–0.015, –0.003)† |
Verbal fluency | |||
Total sedentary timea | –0.022 (–0.038, –0.005)† | –0.018 (–0.035, –0.002)* | –0.019 (–0.038, –0.000)* |
Mean sedentary bout duration | –0.011 (–0.016, –0.005)‡ | –0.009 (–0.015, –0.004)‡ | –0.009 (–0.015, –0.004)† |
Attention | |||
Total sedentary timea | 0.008 (–0.008, 0.025) | 0.010 (–0.006, 0.027) | 0.010 (–0.009, 0.029) |
Mean sedentary bout duration | –0.004 (–0.009, 0.001) | –0.003 (–0.008, 0.003) | –0.003 (–0.009, 0.002) |
Executive function | |||
Total sedentary timea | 0.004 (–0.013, 0.020) | 0.003 (–0.013, 0.020) | 0.007 (–0.012, 0.026) |
Mean sedentary bout duration | –0.003 (–0.008, 0.002) | –0.002 (–0.008, 0.003) | –0.002 (–0.007, 0.004) |
aTotal sedentary time was corrected for accelerometer wear-time and expressed as the estimated sedentary time (hours) per day given a standardized 16 hours of wearing accelerometer. bModel 1 was adjusted for age, sex, education, APOE ɛ4 allele, and accelerometer wear-season; Model 2 was additionally adjusted for smoking, alcohol consumption, body mass index, stroke, coronary heart disease, diabetes, hypertension, and dyslipidemia; and in Model 3 moderate-to-vigorous physical activity was added to model 2. *p < 0.05, †p < 0.01, ‡p < 0.001.
Fig. 1
Dose-response associations of total sedentary time (A) and mean sedentary bout duration (B) with cognition. Data on the associations of total sedentary time and mean sedentary bout duration with global cognition, memory, and verbal fluency z-scores were fitted by restricted cubic spline models. The solid lines and shaded areas represent the β coefficients and 95% confidence intervals, respectively. Models were adjusted for age, sex, education, APOE ɛ4 allele, accelerometer wear-season, smoking, alcohol consumption, body mass index, stroke, coronary heart disease, diabetes, hypertension, dyslipidemia, and moderate-to-vigorous physical activity. Total sedentary time was corrected for accelerometer wear-time and expressed as the estimated sedentary time (hours) per day given a standardized 16 hours of wearing accelerometer.
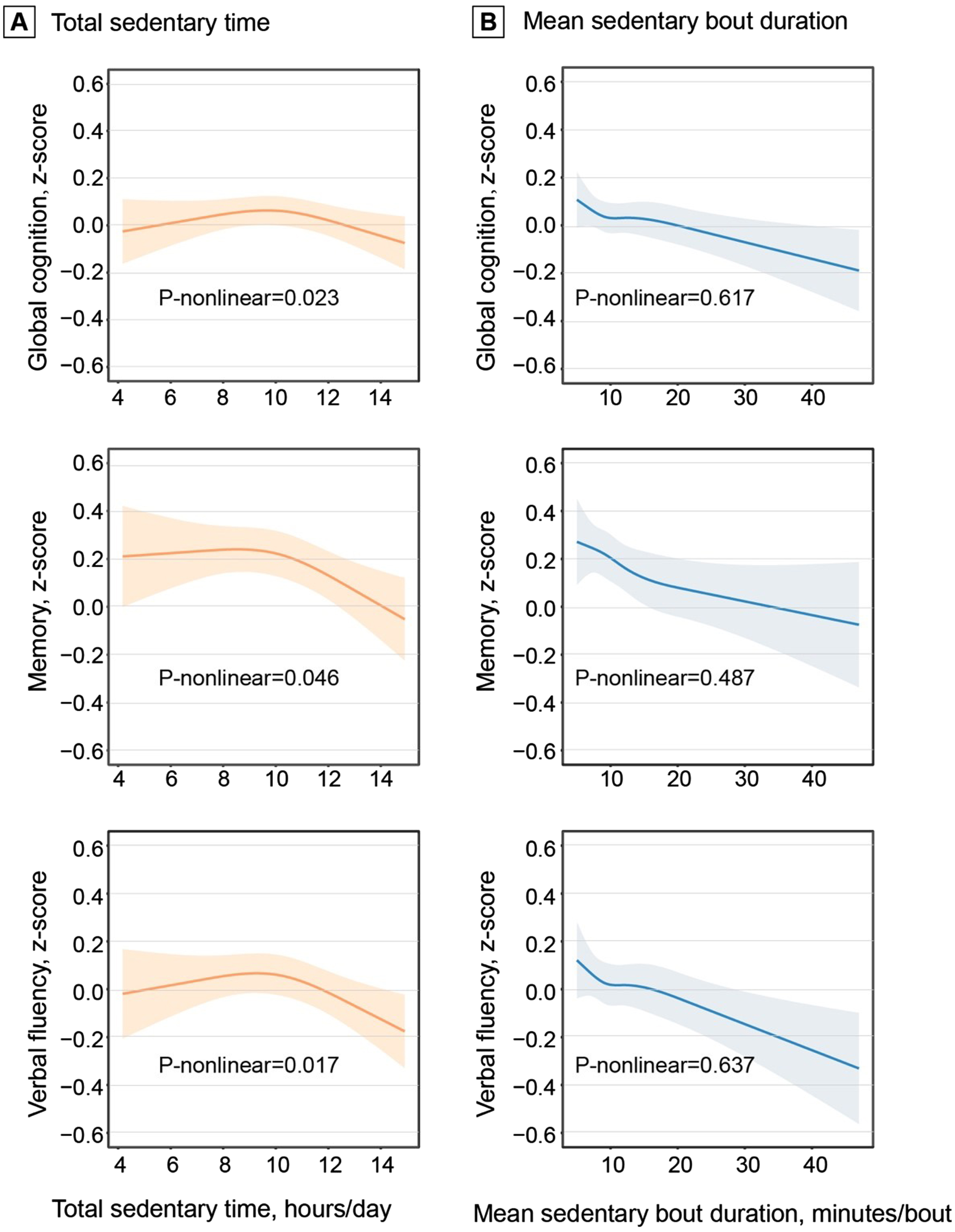
In addition, we examined the potential effect on the association with cognitive z-score of replacing 1-60 min of sedentary behavior with an equal amount of LPA and MVPA in the total sample (Fig. 2). Replacing 60 min of sedentary behavior with 60 min of LPA in a 16-h period was associated with higher z-scores of memory (β= 0.0283; 95% CI: 0.0091–0.0476) and verbal fluency (0.0242; 0.0070-0.0413). However, replacing sedentary time with MVPA was not significantly associated with memory and verbal fluency z-scores.
Fig. 2
Associations of replacing daily sedentary time with light-intensity physical activity (A) and moderate-to-vagarous-intensity physical activity (B) with cognition (n = 2,019). The predicted β-coefficients and 95% confidence intervals of global cognition, memory, and verbal fluency z-scores when reallocating a given amount of time between daily sedentary time and physical activity while keeping the remaining components constant as compositional means in 16 hours (we omitted 8 hours of sleep time per day). The results were controlled for age, sex, education, APOE ɛ4 allele, accelerometer wear-season, smoking, alcohol consumption, body mass index, stroke, coronary heart disease, diabetes, hypertension, and dyslipidemia. LPA, light-intensity physical activity; MVPA, moderate-to-vigorous-intensity physical activity.
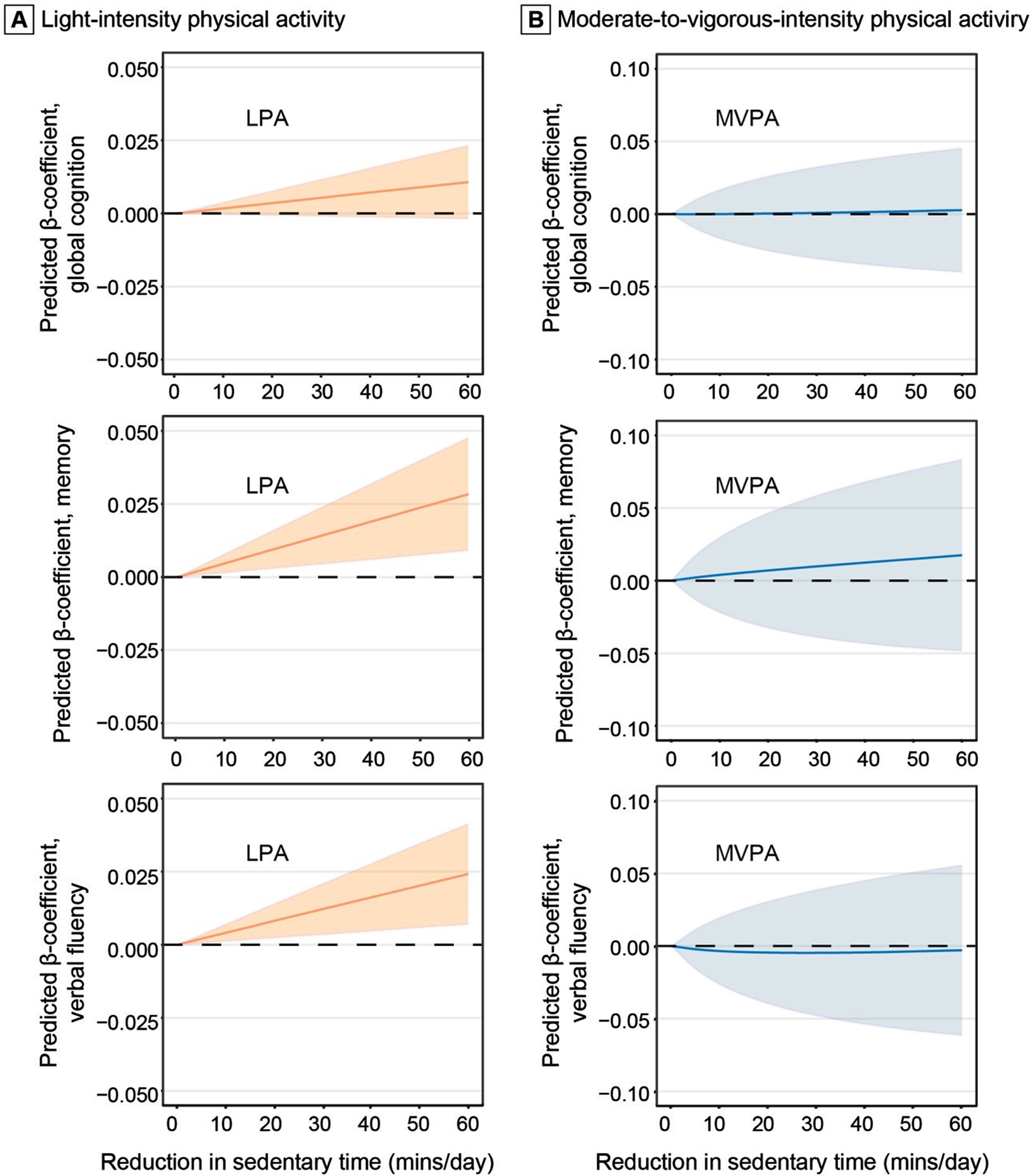
Mediating effect of brain markers on associations of sedentary behavior with cognition
In the MRI sample (n = 1,009), controlling for demographics and TIV, mean sedentary bout duration was significantly associated with a lower global cognitive z-score and was marginally associated with a lower verbal fluency z-score (p = 0.074), but not with the memory z-score (Table 3). When volumes of WMH, total white matter, ventricles, and hippocampus were entered into the mediation model separately, the associations of mean sedentary bout duration with global cognition, memory, and verbal fluency z-scores were significantly attenuated, and the mediation in the associations with global cognition and fluency z-scores was statistically significant (Table 3). The volumes of WMH, ventricles, and hippocampus, but not total white matter, significantly mediated the associations of mean sedentary bout duration with memory z-score. When entering the four structural brain MRI markers into the mediation models separately, the direct association of mean bout duration with global cognition and fluency z-scores became statistically nonsignificant (Table 3).
Table 3
Mediating effects of structural brain markers on the associations of mean sedentary bout duration with cognitive function (n = 1009)
Mean sedentary bout durationb | β coefficient (95% confidence interval)a | ||
and mediators | Global cognition, z-score | Memory, z-score | Verbal fluency, z-score |
Total effect of sedentary behavior | –0.0068 (–0.0134, –0.0002) | –0.0078 (–0.0177, 0.0020) | –0.0081 (–0.0169, 0.0008) |
Mediator, WMH volume | |||
Direct effect of sedentary behavior | –0.0046 (–0.0112, 0.0020) | –0.0048 (–0.0147, 0.0050) | –0.0058 (–0.0147, 0.0031) |
Mediating effect | –0.0022 (–0.0040, –0.0011) | –0.0030 (–0.0054, –0.0014) | –0.0022 (–0.0043, –0.0009) |
Percent mediation | 32.35% | 38.46% | 27.16% |
Mediator, total white matter volume | |||
Direct effect of sedentary behavior | –0.0053 (–0.0120, 0.0013) | –0.0069 (–0.0168, 0.0031) | –0.0057 (–0.0147, 0.0032) |
Mediating effect | –0.0015 (–0.0027, –0.0005) | –0.0010 (–0.0027, 0.0005) | –0.0023 (–0.0040, –0.0009) |
Percent mediation | 22.06% | 12.82% | 28.40% |
Mediator, ventricular volume | |||
Direct effect of sedentary behavior | –0.0047 (–0.0114, 0.0020) | –0.0058 (–0.0158, 0.0042) | –0.0062 (–0.0151, 0.0028) |
Mediating effect | –0.0021 (–0.0035, –0.0009) | –0.0020 (–0.0041, –0.0003) | –0.0019 (–0.0037, –0.0005) |
Percent mediation | 30.88% | 25.64% | 23.46% |
Mediator, hippocampal volume | |||
Direct effect of sedentary behavior | –0.0052 (–0.0118, 0.0014) | –0.0058 (–0.0156, 0.0041) | –0.0063 (–0.0152, 0.0026) |
Mediating effect | –0.0016 (–0.0031, –0.0004) | –0.0020 (–0.0041, –0.0005) | –0.0017 (–0.0036, –0.0004) |
Percent mediation | 23.53% | 25.64% | 20.99% |
WMH, white matter hyperintensity. a β coefficient (95% confidence interval) was derived from the mediation models, controlling for age, sex, and education. bMean sedentary bout duration was calculated as total sedentary time divided by total number of sedentary bouts.
DISCUSSION
This population-based study engaged older adults who were living in rural communities in China. The main findings from this study were summarized as follows. First, there was a dose-response association of longer mean sedentary bout duration (or less frequent breaks in sedentary time) with lower z-scores of global cognition, verbal fluency, and memory independent of MVPA, whereas the linear association of greater sedentary time with poorer global cognitive function, verbal fluency, and memory existed only among older people with long sedentary time (> 10 h/day); Second, breaking up sedentary time with LPA was associated with better verbal fluency and memory function; Finally, the associations of mean bout duration with global cognition, memory, and verbal fluency were largely mediated by WMH, white matter, ventricular, and hippocampal volumes.
The pooling data of five population-based cohorts showed neither cross-sectional nor longitudinal association between self-reported total undifferentiated sedentary time and global cognition [11]. The cross-sectional data from the UK Biobank showed associations of more time spent watching TV with poor cognitive function [38]. However, studies based solely on self-reported sedentary time are prone to recall bias towards underreporting the sedentary time [6, 7], which may underestimate its association with health outcomes. Our study provided solid evidence supporting an association between accelerometer-assessed sedentary time and poor cognitive function in multiple domains among rural-dwelling Chinese older adults.
Notably, our analysis revealed a dose-response relationship between daily prolonged sedentary time and poor cognitive function, especially among individuals with long sedentary time (> 10 h/day). Furthermore, we found that replacing sedentary time with equal amount of LPA might help maintain memory and fluency function in older adults, which is consistent with the findings from the cross-sectional Arakawa 85 + study in Japan [39]. Collectively, these findings imply that movement per se (e.g., sitting less and more frequently taking breaks during sedentary time), rather than intensity, is crucial for cognitive health in older adults. From the public health perspective, this has significant implications for promoting brain health in old age.
Another key contribution of our study was that apart from total sedentary time, the patterns of sedentary behavior were important for brain health and cognition. Previous studies have repeatedly linked prolonged sedentary time (a metric that is highly correlated with longer sedentary bout duration and fewer breaks in sedentary behavior) with cardiometabolic risk factors, cardiovascular disease, and all-cause mortality [33, 40, 41]. These findings highlight the relevance of “prolonger” versus “breaker” hypothesis, i.e., both the amount of sedentary time and the manner in which it is accumulated are important for brain and cognitive health. Our study revealed that prolonged uninterrupted sedentary time was associated with poor memory and verbal fluency function, while more frequent breaks in sedentary behavior with LPA were associated with better memory and verbal fluency.
Clarifying the mediations of structural brain markers in the associations of sedentary behavior with cognition represents the third important contribution of our study. The association between structural brain measures and cognition has been well established [17, 18]. In addition, previous studies have frequently linked sedentary time with structural brain markers in older adults. For example, the cross-sectional data from the Icelandic AGES-Reykjavik Study showed that self-reported physical activity was associated with greater gray matter and white matter volumes [42]. We further revealed that the association of prolonged uninterrupted sedentary behavior with global and domain-specific cognition was largely mediated by MRI markers of WMH and brain atrophy. The community-based studies of older adults in the USA and Sweden showed that larger WMH volume was associated with reduced volumes of cortical gray matter and the hippocampus [43–45]. Our study supported the chain mediating role of both WMH and hippocampal volumes in the association between long sedentary bout duration (or less frequent breaks in sedentary behavior or prolonged sedentary time) and poor cognition. Taken together, these studies support the view that prolonged, uninterrupted sedentary time could be linked with poor cognitive function through mixed pathologies of cerebral microvascular lesions and neurodegeneration.
Several pathophysiological pathways may link sedentary time and patterns with structural brain alterations and poor cognition in older adults. First, prolonged uninterrupted sedentary behavior could affect brain glucose metabolism via elevating postprandial hyperglycemia, proportional hyperinsulinemia, and subsequent insulin resistance [46, 47], thus affecting cognitive function. Furthermore, prolonged sedentary time was associated with reduced shear stress in lower limb blood vessels [48], resulting in viscous blood flow in the lower limb and lower cerebral blood flow [49], which in turn may damage brain structure and lead to cognitive dysfunction [50].
The major strength of our study refers to the community-based design that engaged the largely ignored demographic group of rural-dwelling older adults in China by the research community, in which the objectively measured data on sedentary parameters were integrated with comprehensive cognitive and structural brain MRI data. Thus, we were able to explore not only the relationship of sedentary parameters with cognitive function but also the potential neuropathological pathways underlying the relationships. However, some limitations of our study deserve mentioning. First, the cross-sectional nature of the study design prevented us from making causal inferences or interpretations for any of the observed associations and mediations, and the cross-sectional association was subject to selective survival bias that usually led to an underestimation of the true associations. Furthermore, there was a time gap between assessments of cognitive function (March-September 2018) and collection of ActiGraph and brain MRI data (August 2018-December 2020) that should be considered when interpreting the results. Third, the ActiGraph accelerometer cannot distinguish different postures (e.g., sitting and standing), thus sedentary time may be overestimated by including time of certain standing positions with no movement. Finally, the study sample was derived from only one rural area in western Shandong Province and may not be representative of older population in rural China, which should be kept in mind when generalizing our study findings to other rural populations.
In conclusion, this population-based study of rural-dwelling older adults clearly revealed that accumulation patterns of prolonged sedentary time were linearly associated with poor global cognition, memory, and verbal fluency function, in which the association was largely mediated by structural brain lesions. Furthermore, breaking up sedentary time with LPA was associated with better memory and verbal fluency function. Future longitudinal studies will help further increase our understanding of the causal relationship and potential mechanisms linking sedentary behavior with structural brain aging and cognitive dysfunction in older people. This is highly relevant for the development of interventions to achieve healthy brain aging in rural populations.
ACKNOWLEDGMENTS
We would like to thank all the participants in the MIND-China study as well as the MIND-China Research Group for their collaboration in data collection and management.
FUNDING
This work was supported in part by grants from the National Natural Science Foundation of China (grants no.: 82171175, 81861138008, and 81772448), the Alzheimer’s Association Grant (grant no.: AACSFD-22-922844), the National Key R&D Program of China Ministry of Sciences and Technology (grant no.: 2017YFC1310100), the Natural Science Foundation of Shandong Province (grant no.: ZR2021MH005), the Academic Promotion Program of Shandong First Medical University (2019QL020), the Integrated Traditional Chinese and Western Medicine Program in Shandong Province (YXH2019ZXY008), Shandong Provincial Key Research and Development Program (2021LCZX03), and the Brain Science and Brain-like Intelligence Technology Research Projects of China (2021ZD0201801 and 2021ZD0201808). CQ received grants from the Swedish Research Council (grants no.: 2017-05819 and 2020-01574), the Swedish Foundation for International Cooperation in Research and Higher Education (grant no.: CH2019-8320), and Karolinska Institutet, Stockholm, Sweden. LJL is supported by the Intramural Research Program, National Institute on Aging, Maryland, USA. The funding agency had no role in the study design, data collection and analysis, the writing of this manuscript, and in the decision to submit the work for publication.
CONFLICT OF INTEREST
The authors have no conflict of interest to report.
DATA AVAILABILITY
The datasets used and/or analyzed during the current study are available from the corresponding author upon reasonable request.
SUPPLEMENTARY MATERIAL
[1] The supplementary material is available in the electronic version of this article: https://dx.doi.org/10.3233/JAD-230575.
REFERENCES
[1] | Network. SBR ((2012) ) Letter to the editor: Standardized use of the terms “sedentary” and “sedentary behaviours”. Appl Physiol Nutr Metab 37: , 540–542. |
[2] | Wilmot EG , Edwardson CL , Achana FA , Davies MJ , Gorely T , Gray LJ , Khunti K , Yates T , Biddle SJ ((2012) ) Sedentary time in adults and the association with diabetes, cardiovascular disease and death: Systematic review and meta-analysis. Diabetologia 55: , 2895–2905. |
[3] | Ekelund U , Tarp J , Steene-Johannessen J , Hansen BH , Jefferis B , Fagerland MW , Whincup P , Diaz KM , Hooker SP , Chernofsky A , Larson MG , Spartano N , Vasan RS , Dohrn IM , Hagstromer M , Edwardson C , Yates T , Shiroma E , Anderssen SA , Lee IM ((2019) ) Dose-response associations between accelerometry measured physical activity and sedentary time and all cause mortality: Systematic review and harmonised meta-analysis. BMJ 366: , l4570. |
[4] | Raichlen DA , Klimentidis YC , Sayre MK , Bharadwaj PK , Lai MHC , Wilcox RR , Alexander GE ((2022) ) Leisure-time sedentary behaviors are differentially associated with all-cause dementia regardless of engagement in physical activity. Proc Natl Acad Sci U S A 119: , e2206931119. |
[5] | Dillon K , Morava A , Prapavessis H , Grigsby-Duffy L , Novic A , Gardiner PA ((2022) ) Total sedentary time and cognitive function in middle-aged and older adults: A systematic review and meta-analysis. Sports Med Open 8: , 127. |
[6] | Prince SA , Cardilli L , Reed JL , Saunders TJ , Kite C , Douillette K , Fournier K , Buckley JP ((2020) ) A comparison of self-reported and device measured sedentary behaviour in adults: A systematic review and meta-analysis. Int J Behav Nutr Phys Act 17: , 31. |
[7] | Wanner M , Richard A , Martin B , Faeh D , Rohrmann S ((2017) ) Associations between self-reported and objectively measured physical activity, sedentary behavior and overweight/obesity in NHANES 2003-2006. Int J Obes (Lond) 41: , 186–193. |
[8] | Amagasa S , Inoue S , Murayama H , Fujiwara T , Kikuchi H , Fukushima N , Machida M , Chastin S , Owen N , Shobugawa Y ((2020) ) Associations of sedentary and physically-active behaviors with cognitive-function decline in community-dwelling older adults: Compositional data analysis from the NEIGE Study. J Epidemiol 30: , 503–508 . |
[9] | Wu ZJ , Wang ZY , Hu BQ , Zhang XH , Zhang F , Wang HL , Li FH ((2020) ) Relationships of accelerometer-based measured objective physical activity and sedentary behaviour with cognitive function: A comparative cross-sectional study of China’s elderly population. BMC Geriatr 20: , 149. |
[10] | Rojer AGM , Ramsey KA , Amaral Gomes ES , D’Andrea L , Chen C , Szoeke C , Meskers CGM , Reijnierse EM , Maier AB ((2021) ) Objectively assessed physical activity and sedentary behavior and global cognitive function in older adults: A systematic review. Mech Ageing Dev 198: , 111524. |
[11] | Maasakkers CM , Claassen J , Gardiner PA , Olde Rikkert MGM , Lipnicki DM , Scarmeas N , Dardiotis E , Yannakoulia M , Anstey KJ , Cherbuin N , Haan MN , Kumagai S , Narazaki K , Chen T , Ng TP , Gao Q , Nyunt MSZ , Crawford JD , Kochan NA , Makkar SR , Sachdev PS , Collaborators C , Thijssen DHJ , Melis RJF ((2020) ) The association of sedentary behaviour and cognitive function in people without dementia: A coordinated analysis across five cohort studies from COSMIC. Sports Med 50: , 403–413 . |
[12] | Maasakkers CM , Weijs RWJ , Dekkers C , Gardiner PA , Ottens R , Olde Rikkert MGM , Melis RJF , Thijssen DHJ , Claassen JAHR ((2022) ) Sedentary behaviour and brain health in middle-aged and older adults: A systematic review. Neurosci Biobehav Rev 140: , 104802. |
[13] | Peddie MC , Bone JL , Rehrer NJ , Skeaff CM , Gray AR , Perry TL ((2013) ) Breaking prolonged sitting reduces postprandial glycemia in healthy, normal-weight adults: A randomized crossover trial. Am J Clin Nutr 98: , 358–366. |
[14] | Dunstan DW , Kingwell BA , Larsen R , Healy GN , Cerin E , Hamilton MT , Shaw JE , Bertovic DA , Zimmet PZ , Salmon J , Owen N ((2012) ) Breaking up prolonged sitting reduces postprandial glucose and insulin responses. Diabetes Care 35: , 976–983. |
[15] | Fox FAU , Diers K , Lee H , Mayr A , Reuter M , Breteler MMB , Aziz NA ((2022) ) Association between accelerometer-derived physical activity measurements and brain structure: A population-based cohort study. Neurology 99: , e1202–e1215. |
[16] | Raja D , Ravichandran S , Chandrasekaran B , Kadavigere R , Babu MGR , Almeshari M , Alyahyawi AR , Alzamil Y , Abanomy A , Sukumar S ((2022) ) Association between physical activity levels and brain volumes in adults visiting radio-imaging center of tertiary care hospital. Int J Environ Res Public Health 19: , 17079. |
[17] | Kantarci K , Weigand SD , Przybelski SA , Preboske GM , Pankratz VS , Vemuri P , Senjem ML , Murphy MC , Gunter JL , Machulda MM , Ivnik RJ , Roberts RO , Boeve BF , Rocca WA , Knopman DS , Petersen RC , Jack CR , Jr ((2013) ) MRI and MRS predictors of mild cognitive impairment in a population-based sample. Neurology 81: , 126–133. |
[18] | Boyle PA , Yu L , Fleischman DA , Leurgans S , Yang J , Wilson RS , Schneider JA , Arvanitakis Z , Arfanakis K , Bennett DA ((2016) ) White matter hyperintensities, incident mild cognitive impairment, and cognitive decline in old age. Ann Clin Transl Neurol 3: , 791–800. |
[19] | Wang Y , Han X , Zhang X , Zhang Z , Cong L , Tang S , Hou T , Liu C , Han X , Zhang Q , Feng J , Yin L , Song L , Dong Y , Liu R , Li Y , Ngandu T , Kivipelto M , Snyder H , Carrillo M , Persson J , Fratiglioni L , Launer LJ , Jia J , Du Y , Qiu C ((2022) ) Health status and risk profiles for brain aging of rural-dwelling older adults: Data from the interdisciplinary baseline assessments in MIND-China. Alzheimers Dement (N Y) 8: , e12254. |
[20] | Han X , Wang X , Wang C , Wang P , Han X , Zhao M , Han Q , Jiang Z , Mao M , Chen S , Welmer AK , Launer LJ , Wang Y , Du Y , Qiu C ((2022) ) Accelerometer-assessed sedentary behaviour among Chinese rural older adults: Patterns and associations with physical function. J Sports Sci 40: , 1940–1949. |
[21] | Han X , Jiang Z , Li Y , Wang Y , Liang Y , Dong Y , Tang S , Du Y , Qiu C ((2021) ) Sex disparities in cardiovascular health metrics among rural-dwelling older adults in China: A population-based study. BMC Geriatr 21: , 158. |
[22] | Liang X , Liu C , Liu K , Cong L , Wang Y , Liu R , Fa W , Tian N , Cheng Y , Wang N , Hou T , Du Y , Qiu C ((2022) ) Association and interaction of TOMM40 and PVRL2 with plasma amyloid-β and Alzheimer’s disease among Chinese older adults: A population-based study. Neurobiol Aging 113: , 143–151. |
[23] | Tudor-Locke C , Camhi SM , Troiano RP ((2012) ) A catalog of rules, variables, and definitions applied to accelerometer data in the National Health and Nutrition Examination Survey, 2003-2006. Prev Chronic Dis 9: , E113. |
[24] | Freedson PS ME , Sirard JR ((1998) ) Calibration of the computer science and applications, Inc. accelerometer. Med Sci Sports Exerc 30: , 777–781. |
[25] | Cong L , Ren Y , Wang Y , Hou T , Dong Y , Han X , Yin L , Zhang Q , Feng J , Wang L , Tang S , Grande G , Laukka EJ , Du Y , Qiu C ((2023) ) Mild cognitive impairment among rural-dwelling older adults in China: A community-based study. Alzheimers Dement 19: , 56–66. |
[26] | Qiu C , Sigurdsson S , Zhang Q , Jonsdottir MK , Kjartansson O , Eiriksdottir G , Garcia ME , Harris TB , van Buchem MA , Gudnason V , Launer LJ ((2014) ) Diabetes, markers of brain pathology and cognitive function: The Age, Gene/Environment Susceptibility-Reykjavik Study. Ann Neurol 75: , 138–146. |
[27] | Li Y , Cong L , Hou T , Chang L , Zhang C , Tang S , Han X , Wang Y , Wang X , Kalpouzos G , Du Y , Qiu C ((2021) ) Characterizing global and regional brain structures in amnestic mild cognitive impairment among rural residents: A population-based study. J Alzheimers Dis 80: , 1429–1438. |
[28] | Ashburner J ((2007) ) A fast diffeomorphic image registration algorithm. Neuroimage 38: , 95–113. |
[29] | Rajapakse JC , Giedd JN , Rapoport JL ((1997) ) Statistical approach to segmentation of single-channel cerebral MR images. IEEE Trans Med Imaging 16: , 176–186. |
[30] | Wang M , Li Y , Cong L , Hou T , Luo Y , Shi L , Chang L , Zhang C , Wang Y , Wang X , Du Y , Qiu C ((2021) ) High-density lipoprotein cholesterol and brain aging amongst rural-dwelling older adults: A population-based magnetic resonance imaging study. Eur J Neurol 28: , 2882–2892. |
[31] | Dong Y , Li Y , Liu K , Han X , Liu R , Ren Y , Cong L , Zhang Q , Hou T , Song L , Tang S , Shi L , Luo Y , Kalpouzos G , Laukka EJ , Winblad B , Wang Y , Du Y , Qiu C ((2023) ) Anosmia, mild cognitive impairment, and biomarkers of brain aging in older adults. Alzheimers Dement 19: , 589–601. |
[32] | Wang R , Fratiglioni L , Kalpouzos G , Lovden M , Laukka EJ , Bronge L , Wahlund LO , Backman L , Qiu C ((2017) ) Mixed brain lesions mediate the association between cardiovascular risk burden and cognitive decline in old age: A population-based study. Alzheimers Dement 13: , 247–256. |
[33] | Bellettiere J , LaMonte MJ , Evenson KR , Rillamas-Sun E , Kerr J , Lee IM , Di C , Rosenberg DE , Stefanick M , Buchner DM , Hovell MF , LaCroix AZ ((2019) ) Sedentary behavior and cardiovascular disease in older women: The Objective Physical Activity and Cardiovascular Health (OPACH) Study. Circulation 139: , 1036–1046. |
[34] | Gilchrist SC , Howard VJ , Akinyemiju T , Judd SE , Cushman M , Hooker SP , Diaz KM ((2020) ) Association of sedentary behavior with cancer mortality in middle-aged and older US adults. JAMA Oncol 6: , 1210–1217. |
[35] | Desquilbet L , Mariotti F ((2010) ) Dose-response analyses using restricted cubic spline functions in public health research. Stat Med 29: , 1037–57. |
[36] | Dumuid D , Pedisic Z , Stanford TE , Martin-Fernandez JA , Hron K , Maher CA , Lewis LK , Olds T ((2019) ) The compositional isotemporal substitution model: A method for estimating changes in a health outcome for reallocation of time between sleep, physical activity and sedentary behaviour. Stat Methods Med Res 28: , 846–857. |
[37] | Kandola AA , Del Pozo Cruz B , Osborn DPJ , Stubbs B , Choi KW , Hayes JF ((2021) ) Impact of replacing sedentary behaviour with other movement behaviours on depression and anxiety symptoms: A prospective cohort study in the UK Biobank. BMC Med 19: , 133. |
[38] | Bakrania KEC , Khunti K , Bandelow S , Davies MJ , Yates T ((2018) ) Associations between sedentary behaviors and cognitive function: Cross-sectional and prospective findings from the UK Biobank. Am J Epidemiol 187: , 441–454. |
[39] | Suzuki K , Niimura H , Kida H , Eguchi Y , Kitashima C , Takayama M , Mimura M ((2020) ) Increasing light physical activity helps to maintain cognitive function among the community-dwelling oldest old population: A cross-sectional study using actigraph from the Arakawa 85+study. Geriatr Gerontol Int 20: , 773–778. |
[40] | Healy GN , Matthews CE , Dunstan DW , Winkler EA , Owen N ((2011) ) Sedentary time and cardio-metabolic biomarkers in US adults: NHANES 2003-06. Eur Heart J 32: , 590–597. |
[41] | Diaz KM , Howard VJ , Hutto B , Colabianchi N , Vena JE , Safford MM , Blair SN , Hooker SP ((2017) ) Patterns of sedentary behavior and mortality in U.S. middle-aged and older adults: A national cohort study. Ann Intern Med 167: , 465–475. |
[42] | Arnardottir NY , Koster A , Domelen DRV , Brychta RJ , Caserotti P , Eiriksdottir G , Sverrisdottir JE , Sigurdsson S , Johannsson E , Chen KY , Gudnason V , Harris TB , Launer LJ , Sveinsson T ((2016) ) Association of change in brain structure to objectively measured physical activity and sedentary behavior in older adults: Age, Gene/Environment Susceptibility-Reykjavik Study. Behav Brain Res 296: , 118–124. |
[43] | Van Etten EJ , Bharadwaj PK , Hishaw GA , Huentelman MJ , Trouard TP , Grilli MD , Alexander GE ((2021) ) Influence of regional white matter hyperintensity volume and apolipoprotein E epsilon4 status on hippocampal volume in healthy older adults. Hippocampus 31: , 469–480. |
[44] | Wang R , Fratiglioni L , Laveskog A , Kalpouzos G , Ehrenkrona CH , Zhang Y , Bronge L , Wahlund LO , Backman L , Qiu C ((2014) ) Do cardiovascular risk factors explain the link between white matter hyperintensities and brain volumes in old age? A population-based study. Eur J Neurol 21: , 1076–1082. |
[45] | Li Y , Kalpouzos G , Laukka EJ , Dekhtyar S , Bäckman L , Fratiglioni L , Qiu C ((2022) ) Progression of neuroimaging markers of cerebral small vessel disease in older adults: A 6-year follow-up study. Neurobiol Aging 112: , 204–211. |
[46] | Wheeler MJ , Dempsey PC , Grace MS , Ellis KA , Gardiner PA , Green DJ , Dunstan DW ((2017) ) Sedentary behavior as a risk factor for cognitive decline? A focus on the influence of glycemic control in brain health. Alzheimers Dement (N Y) 3: , 291–300. |
[47] | Dempsey PC , Larsen RN , Winkler EAH , Owen N , Kingwell BA , Dunstan DW ((2018) ) Prolonged uninterrupted sitting elevates postprandial hyperglycaemia proportional to degree of insulin resistance. Diabetes Obes Metab 20: , 1526–1530. |
[48] | Kruse NT , Hughes WE , Benzo RM , Carr LJ , Casey DP ((2018) ) Workplace strategies to prevent sitting-induced endothelial dysfunction. Med Sci Sports Exerc 50: , 801–808. |
[49] | Hachiya T , Walsh ML , Saito M , Blaber AP ((2010) ) Delayed vasoconstrictor response to venous pooling in the calf is associated with high orthostatic tolerance to LBNP. J Appl Physiol (1985) 109: , 996–1001. |
[50] | Hakim AM ((2021) ) A proposed hypothesis on dementia: Inflammation, small vessel disease, and hypoperfusion is the sequence that links all harmful lifestyles to cognitive impairment. Front Aging Neurosci 13: , 679837. |