Risk Factors for Longer-Term Mortality in Discharged Patients with Dementia and SARS-CoV-2 Infection: A Matched Case-Control Study
Abstract
Background:
Persisting symptoms and increased mortality after SARS–CoV–2 infection has been described in COVID-19 survivors.
Objective:
We examined longer-term mortality in patients with dementia and SARS-CoV-2 infection.
Methods:
A retrospective matched case-control study of 165 patients with dementia who survived an acute hospital admission with COVID-19 infection, and 1325 patients with dementia who survived a hospital admission but without SARS-CoV-2 infection. Potential risk factors investigated included socio-demographic factors, clinical features, and results of investigations. Data were fitted using a Cox proportional hazard model.
Results:
Compared to patients with dementia but without SARS-CoV-2 infection, people with dementia and SARS-CoV-2 infection had a 4.4-fold risk of death (adjusted hazard ratio [aHR] = 4.44, 95% confidence interval [CI] 3.13–6.30) even beyond the acute phase of infection. This excess mortality could be seen up to 125 days after initial recovery but was not elevated beyond this time. Risk factors for COVID-19-associated mortality included prescription of antipsychotics (aHR = 3.06, 95% CI 1.40–6.69) and benzodiazepines (aHR = 3.00, 95% CI 1.28–7.03). Abnormalities on investigation associated with increased mortality included high white cell count (aHR = 1.21, 95% CI 1.04–1.39), higher absolute neutrophil count (aHR = 1.28, 95% CI 1.12–1.46), higher C-reactive protein (aHR = 1.01, 95% CI 1.00–1.02), higher serum sodium (aHR = 1.09, 95% CI 1.01–1.19), and higher ionized calcium (aHR = 1.03, 95% CI 1.00–1.06). The post-acute COVID mortality could be modeled for the first 120 days after recovery with a balanced accuracy of 87.2%.
Conclusion:
We found an increased mortality in patients with dementia beyond the acute phase of illness. We identified several investigation results associated with increased mortality, and increased mortality in patients prescribed antipsychotics or benzodiazepines.
INTRODUCTION
Physical, neuropsychiatric, and cognitive symptoms frequently persist in COVID-19 survivors, and symptom persistence is associated with initial disease severity [1–18]. Cognitive symptoms can persist for a longer than other symptoms [1, 9, 19], and people with dementia may suffer disproportionally from persisting symptoms [20–22].
Infection with SARS-CoV-2 does not only increase the risk of death during the period of acute infection. Increased risk of death has been reported for 12 months after initial infection [23, 24]. The reasons for this persistently increased risk are not clear but may relate to ongoing inflammatory or pro-thrombotic effects of infection. Dementia, independent of age, is a well-described risk factor for mortality during acute infection with SARS-CoV-2 [25–30]. To date, the longer-term mortality for patients with dementia who survive the acute phase of infection, and any risk factors for mortality in this context, have not been described. Accurate identification of risk factors may have clinical application as it would allow for enhanced care for those most at risk.
We used routinely collected UK National Health Service (NHS) data, examining a cohort of patients with dementia, with or without SARS-CoV-2 infection, to address three questions. Firstly, is there increased longer-term mortality among people with dementia after SARS-CoV-2 infection, beyond the acute phase? If so, for how long does this period of higher mortality risk last? Thirdly, what baseline demographic, clinical, and biomarker factors are associated with that additional risk, and can these be used to model mortality?
METHODS
Study design and participants
We performed a retrospective matched case-control study using structured electronic health record (EHR) data collated in the EpiCov database, which was extracted from the Cambridge University Hospitals (CUH) National Health Service (NHS) Foundation Trust, a large UK teaching hospital Trust that has 1268 beds and provides local, regional, and national services [31]. CUH’s electronic clinical records contain patient information recorded during routine treatment, including socio-demographic information, diagnoses, prescription data, laboratory results, and death status. Clinicians enter aspects of this information in a systematic and structured way [32]. We extracted demographic, clinical, and laboratory data relating to people with coded diagnoses of dementias, including associated COVID-19 test data, to compare survival trajectories in people with dementia with or without SARS-CoV-2 (COVID-19) infection who were admitted to hospital. Data were de-identified before researchers were given access and were analyzed under approvals from the East of England Cambridge East NHS Research Ethics Committee (reference 20/EE/0270).
Data were collected from 23 March 2020 to 10 April 2022. Eligible patients were those with a coded diagnosis of dementia using World Health Organization (WHO) International Statistical Classification of Diseases (ICD-10) codes F00 and G30 (Alzheimer’s disease, AD), F01 (vascular dementia, VD), F02 (dementia in other diseases), F03 (unspecified dementia), and G31.8 (dementia with Lewy bodies, DLB). We only included those who required admission for COVID-19 (likely reflecting moderate/severe disease). We excluded those who had been diagnosed with dementia subsequent to SARS-CoV-2 infection, and people with more than one infection with SARS-CoV-2. The “exposure” cohort were those people with dementia and laboratory-confirmed SARS-CoV-2. The control group were matched (at a maximum ratio of 10:1) from admitted patients with dementia but no confirmed SARS-CoV-2 infection, matched on age (±1 year), sex, and dementia diagnosis date (±1 year). Laboratory confirmation of SARS-CoV-2 was based on diagnostic reverse transcription polymerase chain reaction (RT-PCR) tests, as recommended by the WHO. The procedure is summarized in Fig. 1.
Fig. 1
STROBE diagram showing construction of the cohorts.
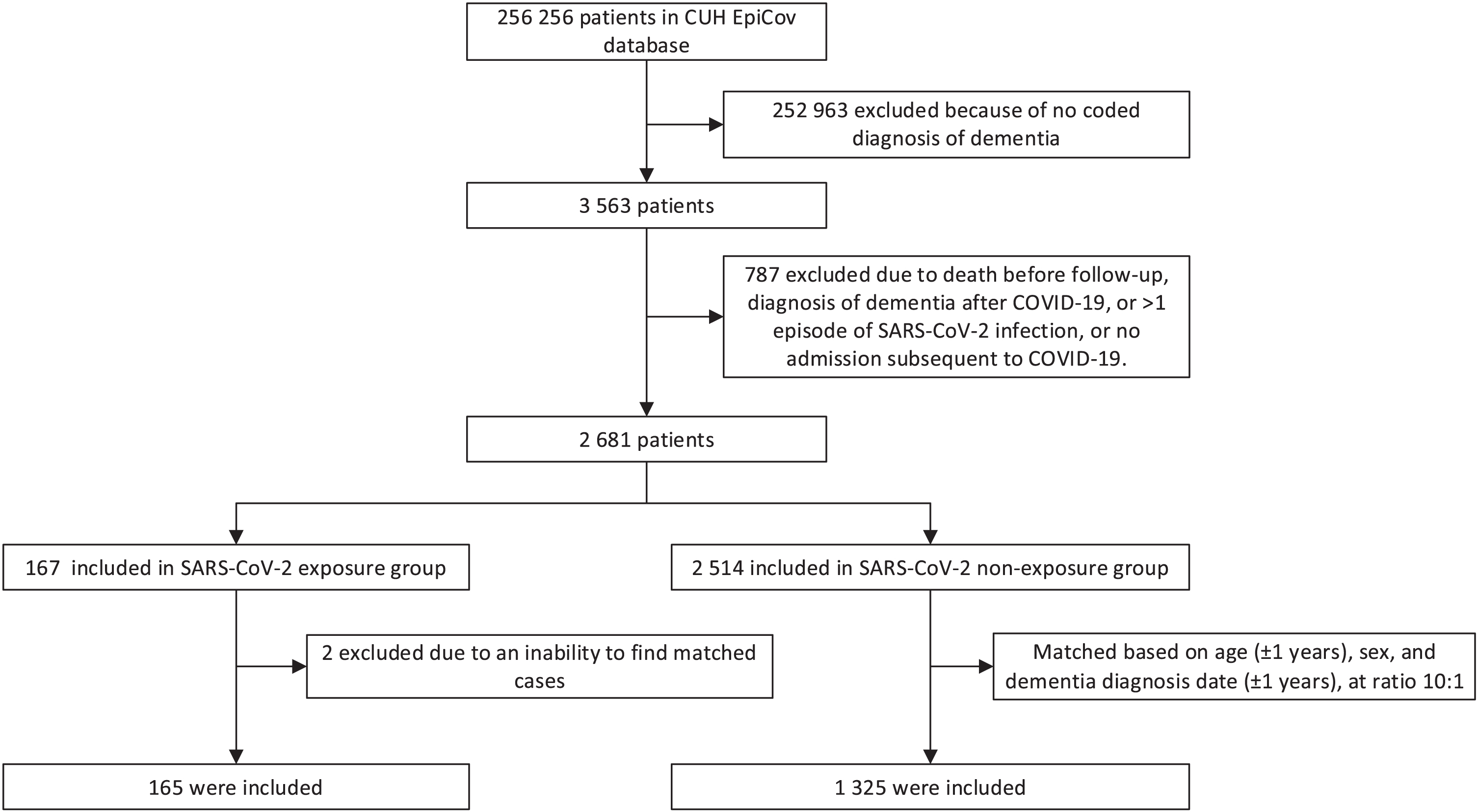
The period of follow-up was selected to exclude the early/acute phase of COVID-19 infection and thus examine subsequent trajectories. Therefore, the index date was the date of discharge. Follow-up was until the date of their death, or the study end date, whichever occurred first.
Data collection
Death status was ascertained by regular linkage to national NHS Spine mortality data for all patients known to CUH. The regular linkage ensured that death was well recorded in the CUH even though it happened after discharge.
We examined the following socio-demographic variables: age at baseline (in years), sex (male versus female), marital status (married, cohabiting, or civil partnership versus single, divorced, or widowed), ethnicity (white, other ethnicities, and not known).
We included the following clinical features in the analysis: physical co-morbidity (measured by the Charlson Comorbidity Index [CCI]) and medicine utilization at baseline. The CCI contains 19 categories of comorbidity (covering most comorbidities that were plausible risk factors for a worse prognosis in people infected with COVID-19 [33, 34]) and can be used to predict 10-year mortality for patients who have a range of comorbid conditions [35]. The formula and ICD-10 codes used for identifying comorbidities can be found elsewhere [36, 37]. Medicines examined included those used to treat dementia or its behavioral and psychological sequelae (Supplementary Table 1), including antipsychotics, antidepressants, antiepileptic drugs, benzodiazepines, opioids, acetylcholinesterase inhibitors (AChEIs), and memantine. Medicines were selected according to UK National Institute for Health and Care Excellence (NICE) guidelines and extracted from structured prescription records. Patients were defined as having been using medicines if these were corresponding prescriptions within three months before the index date.
We also investigated laboratory blood results at baseline, including inflammatory markers (white blood cell count [WBC], procalcitonin, and C-reactive protein [CRP]), renal function markers (estimated glomerular filtration rate [eGFR], serum sodium, serum potassium, serum urea), liver function markers (albumin, alkaline phosphatase, alanine transaminase [ALT], total bilirubin), respiratory markers (venous pO2 and pCO2), and other hematology and biochemistry markers. Biomarker values were extracted from the laboratory tests closest to but before the index date (date of discharge).
Statistical analysis
To describe the baseline characteristics of this cohort, categorical variables were reported as number (percentage), and continuous variables were reported as mean (standard deviation, SD). Baseline differences between groups were assessed via two-tailed t tests (for continuous variables) and chi-square tests (for categorical variables).
A Cox proportional hazard model was used to estimate the association between SARS-CoV-2 infection and mortality, controlling for socio-demographic variables, physical comorbidity, and use of antipsychotics, antidepressants, antiepileptic drugs, benzodiazepines, AChEIs, and memantine, with matching group identity as a cluster variable. The preliminary results (Supplementary Table 2, showing the Schoenfeld test for the proportional hazards assumption, and Supplementary Figure 1, showing the hazard ratio with 95% confidence intervals [CI] against follow-up time) indicate that the influence of SARS-CoV-2 infection on death violated the proportional hazard assumption of Cox regression, and suggested that the effect of SARS-CoV-2 infection had a point of inflexion at about 125 days. We therefore estimated coefficients by splitting the follow-up time into two intervals and including this as a stratification variable. To ensure the consistency of our results, the Cox regression was conducted in a hierarchical approach.
Two sensitivity analyses were conducted by including only exposure cases and their matched control cases with index dates between 1 October 2020 and 1 June 2021, and (separately) between 1 August 2021 and 1 April 2022. These two sensitivity analyses aimed to check the consistency of our results under possible changes in treatment efficacy (treatments were improving across our study period) and the lethality of SARS-CoV-2 (virus strains were evolving likewise).
We explored the risk factors for the additional risk of death caused by the SARS-CoV-2 infection during the first 125 days afterwards the acute phase of COVID-19 identified in the above cox model. Interactions between potential risk factors or biomarkers and COVID-19 status (yes or no) were included in the above Cox model but limit the follow-up time to the first 125 days.
We built eight prediction models covering linear algorithms (including linear discriminant analysis and logistic regression), non-liner algorithms (including decision tree, k-nearest neighbors algorithm, neural network, and naive Bayes), and advanced algorithms (including support vector machines and random forest), to predict the death within 1 week, 3 weeks, 1 month, 2 months, 3 months, and 4 months, after the acute period of COVID-19, based on the baseline data. All models fitted for each time window separately. The cohort data was randomly split into a “training” dataset (accounting for 80% of the cohort data) and a “test” dataset (comprising the remaining 20%). Given the relatively small number of deaths, training dataset was balanced by synthetic minority oversampling technique (SMOTE), an oversampling technique where the synthetic samples are generated for the minority class [38]. All of the eight models were trained and tuned in the training dataset exclusively using a 5-fold cross-validation. Because a detailed description of the methodology of the above prediction models is beyond the scope of this applied study, all parameters were set to default. We include our source code in the supplementary data to provide detailed information about our pre-processing methods. Briefly, these models were performed via the ‘caret’ package (version 6.0–92) in R, with method “lda” for linear discriminant analysis, method “glm” and family “binomial” for logistic regression, method “rpart” for decision tree, method “knn” for k-nearest neighbors algorithm, method “nnet” for neural network, method “naive_bayes” for naive Bayes, method “svmRadial” for support vector machines (SVM), and method “rf” for random forest. The outcome was death status (yes or no) during the prediction window, and predictors included all the variables (listed in Table 1) and their interactions with SARS-CoV-2 infection (yes or no). Model performance was reported exclusively on the test dataset, with the index of accuracy.
Table 1
Patient characteristics at baseline. Baseline means the date of discharge from hospital. Data are shown as mean (standard deviation [SD]) or number (percentage). p values for continuous variables were obtained by ANOVA, and for categorical variables via Pearson’s chi-square test. U, units
SARS-CoV-2 unexposed group (N = 1,325) | SARS-CoV-2 exposed group (N = 165) | p | |
DEMOGRAPHICS | |||
Age (y) | 85.2 (7.1) | 85.6 (7.4) | 0.5948 |
Sex (=male) | 532 (40.2%) | 68 (41.2%) | 0.8588 |
Marital status (=married, cohabiting, or civil partnership) | 620 (46.8%) | 71 (43%) | 0.4059 |
Ethnicity | |||
White | 1,067 (80.5%) | 123 (74.5%) | 0.0648 |
Others | 16 (1.2%) | 5 (3.0%) | |
Not Known | 242 (18.3%) | 37 (22.4%) | |
Death (=yes) | 342 (25.8%) | 71 (43.0%) | <0.0001 |
Follow-up duration (days) | 363.8 (234.0) | 239.4 (237.0) | <0.0001 |
CLINICAL FEATURES | |||
Physical comorbidity (CCI score) | 5.3 (1.8) | 5.5 (2.0) | 0.1718 |
Antidepressant use (=yes) | 460 (34.7%) | 74 (44.8%) | 0.0134 |
Antipsychotic use (=yes) | 228 (17.2%) | 55 (33.3%) | <0.0001 |
Antiepileptic use (=yes) | 212 (16%) | 46 (27.9%) | 0.0002 |
Benzodiazepine use (=yes) | 460 (34.7%) | 90 (54.5%) | <0.0001 |
Opioid use (=yes) | 691 (52.2%) | 108 (65.5%) | 0.0016 |
AChEI use (=yes) | 302 (22.8%) | 50 (30.3%) | 0.0409 |
Memantine use (=yes) | 260 (19.6%) | 31 (18.8%) | 0.8800 |
BIOMARKERS | |||
Inflammatory markers | |||
White blood cell count (×109/L) | 8.707 (3.631) | 7.563 (2.61) | <0.0001 |
Absolute neutrophil count (×109/L) | 6.199 (3.04) | 5.53 (2.373) | 0.0013 |
Absolute lymphocyte count (×109/L) | 1.386 (1.683) | 1.257 (0.717) | 0.0836 |
Absolute monocyte count (×109/L) | 0.529 (0.231) | 0.475 (0.2) | 0.0015 |
Absolute eosinophil count (×109/L) | 0.172 (0.162) | 0.128 (0.116) | <0.0001 |
Absolute basophil count (×109/L) | 0.04 (0.023) | 0.034 (0.019) | 0.0007 |
Procalcitonin (μg/L) | 0.221 (0.072) | 0.25 (0.089) | 0.0001 |
C-reactive protein (mg/L) | 37.871 (48.114) | 46.199 (45.273) | 0.0303 |
Renal function/electrolytes | |||
eGFR (mL min–1 1.73 m–2) | 62.017 (18.348) | 61.158 (17.757) | 0.6845 |
Serum sodium (Na+) (mM) | 138.233 (3.909) | 139.696 (4.503) | 0.0001 |
Serum potassium (K+) (mM) | 4.161 (0.43) | 4.086 (0.433) | 0.0394 |
Serum urea (mM) | 8.261 (4.004) | 8.352 (3.643) | 0.7699 |
Liver function tests | |||
Albumin (g/L) | 32.48 (5.138) | 28.567 (4.744) | <0.0001 |
Alkaline phosphatase (U/L) | 98.092 (65.65) | 109.47 (88.649) | 0.1147 |
Alanine transaminase (alanine aminotransferase) (U/L) | 24.263 (32.74) | 30.711 (40.046) | 0.0524 |
Total bilirubin (μM) | 11.368 (8.706) | 13.229 (38.852) | 0.5431 |
Respiratory markers | |||
Venous PO2 (kPa) | 4.775 (3.281) | 4.375 (1.618) | 0.0157 |
Venous PCO2 (kPa) | 6.042 (0.854) | 5.986 (0.929) | 0.4731 |
OTHER BIOMARKERS | |||
Hematology | |||
Hematocrit (L/L) | 0.381 (0.052) | 0.378 (0.049) | 0.4595 |
MCHC [g/L] | 30.404 (2.251) | 29.752 (1.835) | <0.0001 |
Mean cell volume (fl) | 91.585 (5.867) | 91.389 (4.889) | 0.6392 |
Red blood cell count (×1012/L) | 4.125 (0.571) | 4.132 (0.555) | 0.8781 |
Platelet count (×109/L) | 253.149 (85.697) | 286.767 (108.84) | 0.0002 |
Hemoglobin (g/L) | 122.083 (17.191) | 121.942 (15.6) | 0.9149 |
Red blood cell distribution width (%) | 14.531 (1.491) | 14.497 (1.527) | 0.7868 |
Platelet distribution width (%) | 51.932 (6.776) | 52.283 (6.632) | 0.5276 |
Mean platelet volume (fl) | 8.816 (1.032) | 8.822 (0.879) | 0.9353 |
Point-of-care biochemistry: | |||
(Point-of-care test) ionized calcium (mM) | 1.184 (0.062) | 1.167 (0.062) | 0.0014 |
(Point-of-care test) FiO2 | 22.435 (5.646) | 23.028 (5.63) | 0.2178 |
(Point-of-care test) Temperature (°C) | 36.957 (0.481) | 37.093 (0.459) | 0.0054 |
(Point-of-care test) Hydrogen ion concentration (H+) (μM) | 41.944 (3.847) | 41.341 (4.336) | 0.1758 |
(Point-of-care test) Lactate (mM) | 1.894 (0.88) | 1.94 (0.782) | 0.5847 |
(Point-of-care test) Base excess (mM) | 0.978 (2.901) | 0.919 (2.71) | 0.8011 |
(Point-of-care test) Venous oxygen saturation (SpO2) [% ] | 57.392 (20.065) | 55.521 (19.45) | 0.3571 |
Other biochemistry | |||
Glucose, venous (mM) | 7.241 (2.397) | 7.139 (2.481) | 0.6262 |
Bicarbonate (HCO3–) (mM) | 26.175 (2.955) | 26.136 (2.991) | 0.8786 |
Calcium (Ca2 +) (mM) [corrected] | 2.374 (0.134) | 2.331 (0.133) | 0.0003 |
Phosphate (PO42–) (mM) | 1.092 (0.191) | 1.03 (0.189) | 0.0003 |
Thyroid-stimulating hormone (mU/L) | 2.885 (5.688) | 2.845 (4.316) | 0.9258 |
CCI, Charlson Comorbidity Index; AChEI, acetylcholinesterase inhibitors; eGFR, estimated glomerular filtration rate; MCHC, Mean corpuscular hemoglobin concentration; FiO2, fraction of inspired oxygen.
We used R (version 3.6.0) for all analyses and defined statistical significance as p < 0.05. Results are reported following the STROBE checklist for cohort studies.
RESULTS
Between 23 March 2020 and 10 April 2022, a total of 165 discharged patients with dementia who went through their acute phase of COVID-19 were included in the exposure cohort, and 1,325 dementia patients without SARS-CoV-2 infection were included in the control cohort (Fig. 1). The cohorts were followed up for a mean of 239.4 and 363.8 days, respectively (Table 1). There were some differences in the baseline (date of discharge from hospital) characteristics of patients between the two cohorts (Table 1). Patients in the SARS-CoV-2 exposure cohort were more likely to have been prescribed antidepressants (p = 0.0134), antipsychotics (p < 0.0001), antiepileptic drugs (p = 0.0002), benzodiazepines (p < 0.0001), opioids (p = 0.0016), and AChEIs (p = 0.0409). No significant difference between the two groups was observed for demographics characteristics, including age (p = 0.5948), sex (p = 0.8588), marital status (p = 0.4057), and ethnicity (p = 0.0648), or other clinical features including physical comorbidity (p = 0.1718) and memantine use (p = 0.88). Comparison of the biomarkers is also shown in Table 1.
Figure 2 presents survival curves. In unadjusted analyses, mortality rates were higher after people with dementia had survived their acute phase of COVID-19 infection, compared to those who were not exposed to SARS-CoV-2 (p < 0.0001). Figure 2 also shows a violation of the proportional hazards assumption of Cox regression, as also indicated by the results shown in Supplementary Table 2 and Supplementary Figure 1.
Fig. 2
Kaplan-Meier survival curves of mortality in people with dementia, by COVID-19 status. p values are calculated from the log-rank test.
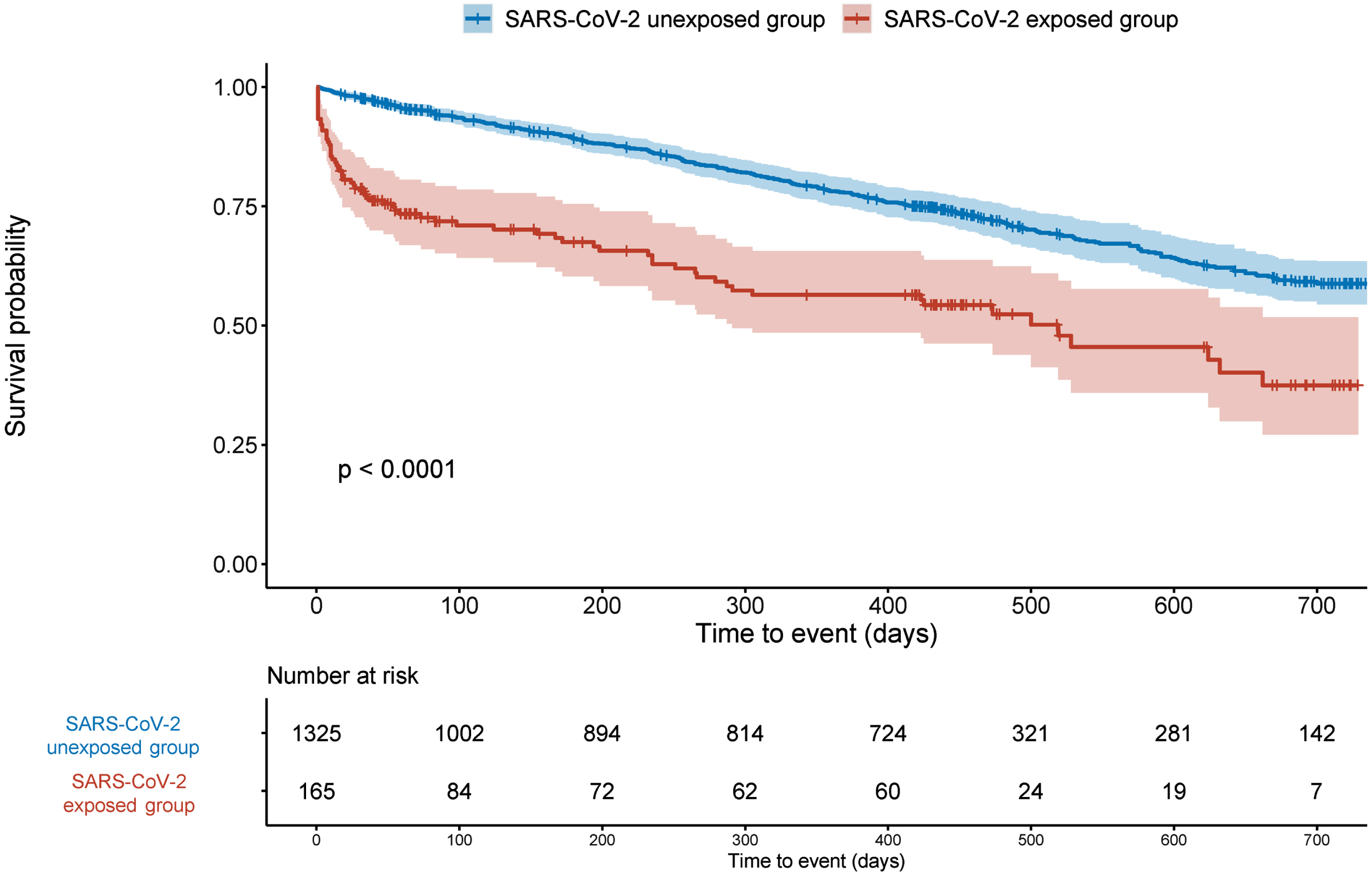
After controlling for confounding by sociodemographic factors (age, sex, marital status, ethnicity) and clinical features (physical comorbidity, antidepressant use, antipsychotic use, antiepileptic drug use, benzodiazepine use, opioid use, AChEI use, and memantine use), compared with patients with dementia but without SARS-CoV-2 infection, people with dementia and SARS-CoV-2 infection had a 4.4-fold risk of death (adjusted hazard ratio [HR] 4.44, 95% confidence interval [CI] 3.13–6.30) during the first 125 follow-up days after they survived their acute phase of COVID-19. This additional mortality risk decreased significantly after 125 follow-up days (HR 0.26, CI 0.15–0.44) and were similar to the general level among people with dementia but without SARS-CoV-2 infection (Supplementary Figure 1). The hierarchical analysis (shown in models 1 model 2 in Table 2) confirmed the consistency of these results. The sensitivity analyses examining different time periods, to account for potential changes in treatment efficacy and SARS-CoV-2 lethality (Supplementary Figures 2 and 3; Supplementary Tables 3 and 4) also confirmed the consistency of the results.
Table 2
Association between SARS-CoV-2 infection and mortality during different follow-up days
Model 1 | Model 2 | Model 3 | |
COVID-19 status | |||
No COVID-19 infection | Reference | Reference | Reference |
COVID-19 infection | 5.10 (3.60–7.17)*** | 5.00 (3.53–7.10)*** | 4.44 (3.13–6.30)*** |
COVID-19 infection×follow-up >125 days (=yes) | 0.25 (0.15–0.43)*** | 0.24 (0.14–0.40)*** | 0.26 (0.15–0.44)*** |
Data are shown as hazard ratios (HRs) and their 95% confidence intervals. Follow-up time was split into two segments as the risk changed over time (see Methods). Model 1 estimated the unadjusted HR. Model 2 is adjusted for age, sex, marital status, and ethnicity. Model 3 is adjusted for age, sex, marital status, ethnicity, physical comorbid, antidepressant use, antipsychotic use, antiepileptic drug use, benzodiazepines use, opioid use, acetylcholinesterase inhibitor use, and memantine use. *p < 0.5, **p < 0.01, ***p < 0.001.
Table 3 shows the risk factors that predicted additional mortality risk in people with dementia after admission with SARS-CoV-2 infection, compared to people with dementia after admission without SARS-CoV-2 infection. The risk factors included unknown ethnicity (adjusted HR 3.06, CI 1.04–8.94), taking an antipsychotic (adjusted HR 3.06, CI 1.40–6.69), taking a benzodiazepine (adjusted HR 3.00, CI 1.28–7.03), and having a higher WBC (adjusted HR 1.21, CI 1.04–1.39), a higher absolute neutrophil count (adjusted HR 1.28, CI 1.12–1.46), higher C-reactive protein (adjusted HR 1.01, CI 1.00–1.02), higher serum sodium (adjusted HR 1.09, CI 1.01–1.19), and higher ionized calcium (adjusted HR 1.03, CI 1.00–1.06), while the factors associated with decreased mortality were a higher absolute lymphocyte count (adjusted HR 0.35, CI 0.13–0.95), higher absolute basophil count (adjusted HR 0.00, CI 0.00–0.28), higher albumin (adjusted HR 0.90, CI 0.84–0.98), and higher bicarbonate (adjusted HR 0.87, CI 0.76–0.99).
Table 3
Risk factors and biomarkers for additional risk of death among people with dementia, compared to those without COVID-19. Data are shown as adjusted hazard ratios (HRs) and their 95% confidence intervals (CIs). Results (both for demographic/clinical variables and biomarkers) were extracted from interactions (between the term shown and COVID-19 status) in a Cox model, controlling for age, sex, marital status, ethnicity, physical comorbidity, antidepressant use, antipsychotic use, antiepileptic drug use, benzodiazepine use, opioid use, AChEI use, and memantine use. A single regression, with interactions, was fitted for clinical/demographic variables, using a Cox model. For biomarkers, each biomarker was fitted one by one in consideration of the high correlation between biomarkers. *p < 0.5, **p < 0.01, ***p < 0.001
variable | Hazard ratio (95% CI) |
DEMOGRAPHICS | |
Age (y) | 0.96 (0.91–1.02) |
Sex (=male) | 1.49 (0.73–3.06) |
Marital status (=married, cohabiting or civil partnership) | 0.74 (0.35–1.58) |
Ethnicity | |
White | |
Others | 0.45 (0.03–5.99) |
Not Known | 3.06 (1.04–8.94)* |
CLINICAL FEATURES | |
Physical comorbidity (CCI score) | 0.91 (0.76–1.08) |
Antidepressant use (=yes) | 1.16 (0.54–2.51) |
Antipsychotic use (=yes) | 3.06 (1.40–6.69) ** |
Antiepileptic use (=yes) | 1.49 (0.62–3.60) |
Benzodiazepine use (=yes) | 3.00 (1.28–7.03)* |
Opioid use (=yes) | 2.10 (0.88–4.95) |
AChEI use (=yes) | 0.92 (0.36–2.36) |
Memantine use (=yes) | 1.84 (0.75–4.53) |
BIOMARKERS | |
Inflammatory markers | |
White blood cell count (×109/L) | 1.21 (1.04–1.39)* |
Absolute neutrophil count (×109/L) | 1.28 (1.12–1.46) *** |
Absolute lymphocyte count (×109/L) | 0.35 (0.13–0.95)* |
Absolute monocyte count (×109/L) | 1.32 (0.19–9.03) |
Absolute eosinophil count (×109/L) | 0.06 (0.00–5.37) |
Absolute basophil count (×109/L) | 0.00 (0.00–0.28)* |
Procalcitonin (μg/L) | 2.53 (0.70–9.21) |
C-reactive protein (mg/L) | 1.01 (1.00–1.02) ** |
Renal function/electrolytes | |
eGFR (mL min–1 1.73 m–2) | 1.01 (0.98–1.03) |
Serum sodium (Na+) (mM) | 1.09 (1.01–1.19)* |
Serum potassium (K+) (mM) | 0.97 (0.32–3.00) |
Serum urea (mM) | 1.11 (0.99–1.23) |
Liver function tests | |
Albumin (g/L) | 0.90 (0.84–0.98) ** |
Alkaline phosphatase (U/L) | 1.00 (1.00–1.00) |
Alanine transaminase (alanine aminotransferase) (U/L) | 1.01 (1.00–1.01) |
Total bilirubin (μM) | 1.02 (0.99–1.05) |
Respiratory markers | |
Venous PO2 (kPa) | 0.97 (0.78–1.21) |
Venous PCO2 (kPa) | 0.68 (0.43–1.06) |
OTHER BIOMARKERS | |
Hematology | |
Hematocrit (L/L) | 1.01 (0.98–1.03) |
MCHC [g/L] | 0.99 (0.83–1.19) |
Mean cell volume (fl) | 1.01 (0.95–1.08) |
Red blood cell count (×1012/L) | 1.13 (0.49–2.61) |
Platelet count (×109/L) | 1.00 (1.00–1.00) |
Hemoglobin (g/L) | 1.01 (0.98–1.03) |
Red blood cell distribution width (%) | 0.98 (0.80–1.19) |
Platelet distribution width (%) | 1.03 (0.98–1.09) |
Mean platelet volume (fl) | 1.01 (0.68–1.51) |
Point-of-care biochemistry: | |
(Point-of-care test) ionized calcium (mM) | 1.03 (1.00–1.06)* |
(Point-of-care test) FiO2 | 1.03 (0.96–1.09) |
(Point-of-care test) Temperature (°C) | 1.23 (0.64–2.34) |
(Point-of-care test) Hydrogen ion concentration (H+) (μM) | 1.02 (0.87–1.20) |
(Point-of-care test) Lactate (mM) | 1.03 (0.61–1.77) |
(Point-of-care test) Base excess (mM) | 0.88 (0.76–1.01) |
(Point-of-care test) Venous oxygen saturation (SpO2) [% ] | 1.01 (0.99–1.04) |
Other biochemistry | |
Glucose, venous (mM) | 0.91 (0.78–1.08) |
Bicarbonate (HCO3–) (mM) | 0.87 (0.76–0.99)* |
Calcium (Ca2 +) (mM) [corrected] | 1.12 (0.80–1.54) |
Phosphate (PO42–) (mM) | 0.28 (0.03–3.00) |
Thyroid-stimulating hormone (mU/L) | 1.01 (0.95–1.07) |
CI, Charlson Comorbidity Index; AChEI, acetylcholinesterase inhibitors; eGFR, estimated glomerular filtration rate; MCHC, Mean corpuscular hemoglobin concentration; FiO2, fraction of inspired oxygen.
Figure 3 presents the accuracy with different prediction windows. These indicate that using baseline data, post-acute COVID-associated death after discharge from hospital could be predicted, taking the results from the random forest model as an example, within 7 days with a balanced accuracy of 94% and within 120 days with a balanced accuracy of 87.2%.
Fig. 3
Accuracy with different prediction windows. All models fitted for each time window separately.
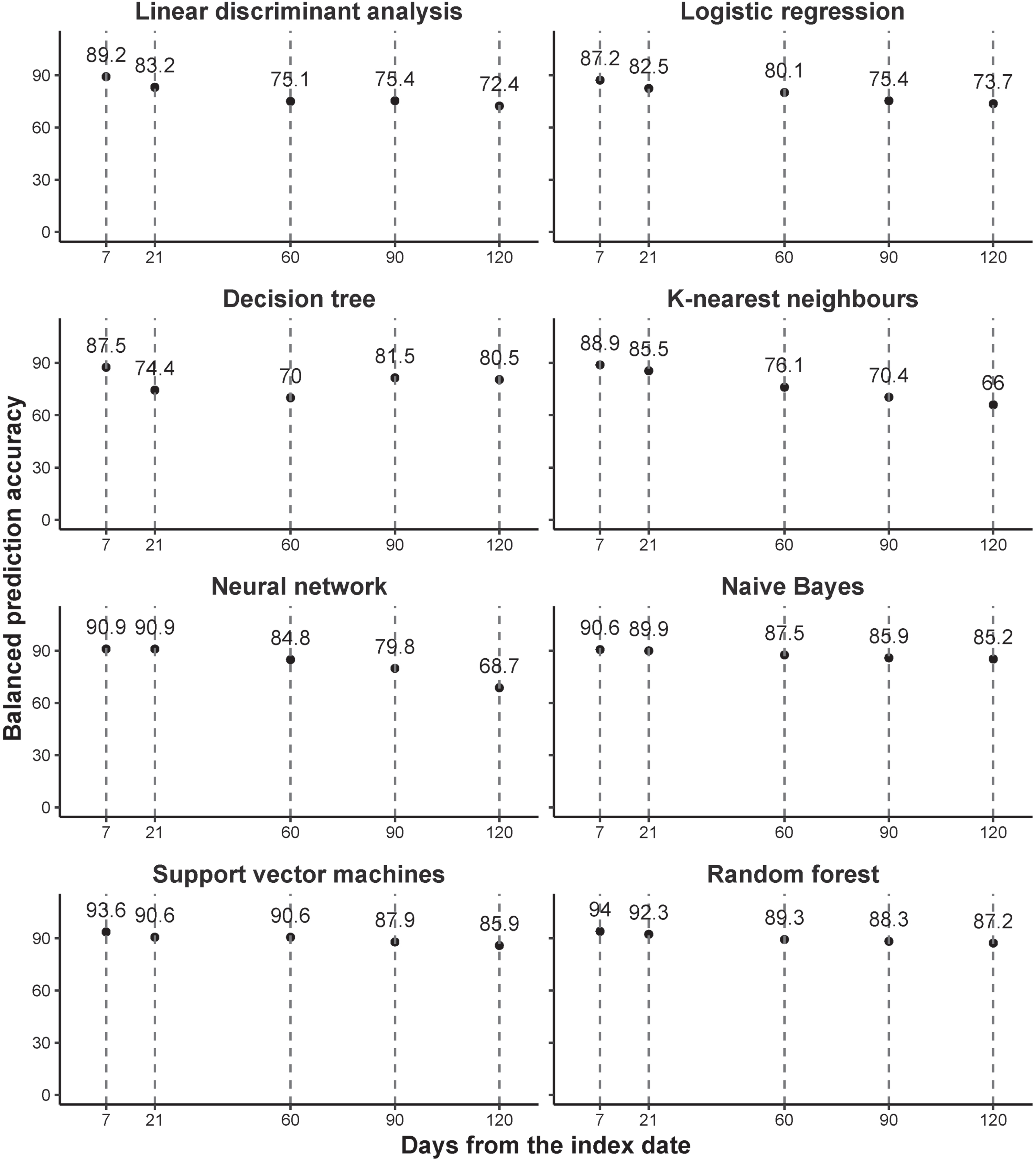
DISCUSSION
Using a retrospective matched case-control study based on a large and comprehensive clinical record database, and after controlling for known risk factors for mortality including socio-demographic factors, medications, physical and psychiatric morbidities, we found that SARS-CoV-2 infection was associated with a nearly 4-fold higher risk of death among people with dementia after recovery from acute infection and discharge from hospital. This excess mortality persisted for 125 days. Risk factors (demographic, clinical features, and biomarkers) for these additional risks were identified. We were able to use the risk factors identified to predict mortality during the period of increased risk with reasonable accuracy.
Our findings confirmed our hypothesis that the mortality following SARS-CoV-2 infection among people with dementia remains raised beyond the acute phase of the illness. The longer-term excess mortality identified in our study is consistent with emerging evidence identifying persistence of symptoms [1–18], especially cognitive symptoms [1, 9, 19] following recovery from the acute phase of illness. The additional mortality risk identified in our study is also in keeping with another UK study including 24,673 discharged COVID-19 patients, which indicated that all-cause post-discharge mortality was higher in the COVID-19 group than in the general population [24]. Here we show that post-acute-COVID mortality is clearly raised among people with dementia and that this might be a particularly vulnerable group.
Our findings suggest that the excess post-acute COVID-associated mortality only persists for 125 days after initial recovery (discharge from hospital). The 125-day cut-off identified in our study was partly consistent with a 1-year follow-up study from Spain including 3,210 COVID-19 patients with severe symptoms, which found that the median time between hospital admission and mortality in those patients who died while not in the hospital was 145 days [39], another 1-year follow-up study from Spain including 56 hemodialysis patients with COVID-19, which indicated that increased risk of death was predominantly within the first three months after COVID-19 diagnosis [40], and a further study from the UK covering 24,673 discharged COVID-19 patients, which found that excess death risk in discharged COVID-19 patients (versus the general population) was more pronounced in the earlier follow-up period after discharge but decreased over time. Our findings suggest that patients with dementia who have been infected with SARS-CoV-2 may benefit from enhanced follow-up for the first four months after the acute infection.
We identified a number of clinical factors and investigation results for people with dementia and COVID-19 associated with increased mortality. Some of the significant factors, such as acute inflammation, hypernatremia, and liver dysfunction, have been previously described, suggesting that these general risk factors for mortality in patients infected with SARS-CoV-2 [40–43]. We also identified that lower albumin was associated with a higher mortality risk, which is consistent with previous studies [44, 45]. Low albumin may have indicated pre-existing malnutrition and that being a risk factor, but we should also note that a decrease in albumin is part of the acute phase response to SARS-CoV-2 infection, so more severe inflammation is a cause of lower albumin and it is the more severe inflammation itself that may be a cause of death [46].
Perhaps our most striking finding was the two-fold increased risk of death in patients prescribed antipsychotic and benzodiazepine drugs. The medications recorded on our used dataset would include medicines being taken on admission and medicines prescribed subsequent to admission. Antipsychotics and benzodiazepine are known to be associated with increased mortality in the context of dementia [47, 48] and may aggravate neuropsychiatric symptoms in the context of SARS-CoV-2 infection [49], but it is possible those with superimposed SARS-CoV-2 infection might be even more vulnerable. Alternatively, it may be that antipsychotics and benzodiazepine are more likely to be prescribed late in the dementia disease course when patients might be at higher risk of death following infection. Therefore, the increased mortality seen with antipsychotics may be a direct result of the medication, but also may be because these drugs are prescribed to treat symptoms associated with delirium and therefore be correlated with more severe infection or alternatively be prescribed to manage the neuropsychiatric symptoms of more advanced dementia. Any or all of these could explain the association with increased mortality. However, our data should serve as a reminder to be cautious about prescribing these drugs in this patient group and suggests further investigation of the nature of this risk is appropriate.
Importantly, we found that baseline data could be used to predict post-acute-COVID mortality with acceptable accuracy. This raises the possibility of targeted follow-up or intervention for those identified at highest risk.
Strengths of our study included the use of an anonymized electronic records database derived from routinely collected clinical records, reducing problems such as sample selection bias, attrition, and recall bias relative to other data sources. In addition, the large longitudinal and population-based data from an electronic record, containing relatively complete information on patients and disease characteristics, enabled us to examine the risk factors and build a model to predict the excess post-acute COVID-associated mortality identified.
Our work has several limitations. Firstly, neither the vaccination status nor SARS-CoV-2 variant details were available in our dataset. However, we matched control cases for each infected person over the same time period, and vaccination in the UK was prioritized in the elderly with very high rates of coverage, so within each matched case-control group, the vaccine status was likely to be similar. The sensitivity analysis covering different sub-periods, to account for potential changes in treatment efficacy and the lethality of SARS-CoV-2 strains over time (Supplementary Figures 3 and 4, Supplementary Table 3 and 4), also confirmed the consistency of our results. Secondly, the sample size is relatively small. This prevented us from performing subgroup analyses for different types of dementia, and different dementia subtypes may be impacted differently by concomitant infection [50]. Thirdly, we cannot be sure that the control cohort did not include patients with undiagnosed COVID-19 infection, though during the period covered by our study, the clinical presentation of COVID-19 was well understood, and widespread PCR testing was available. Fourthly, the reason for admission is not available. Although requiring admission likely reflects moderate to severe COVID-19 in the exposure group, we cannot completely exclude the possible that the SARS-CoV-2 exposure cases were admitted for other reasons with SARS-CoV-2 infection being incidental or contracted during admission. Reasons for admission in the control group will of course vary widely. Fifthly, the naturalistic study prevents us from making causal inferences. For instance, although we identified that use of antipsychotics/benzodiazepines was associated with a higher mortality risk after discharge (following admission associated with COVID-19) among people with dementia, this could be because of a direct drug effect, or because patients admitted with COVID-19 are more likely to be given these drugs to treat agitated delirium. Sixthly, we controlled the marital status, but it does not describe the context of the patient’s life that may affect the risk of mortality (e.g., the presence of a caregiver, or institutionalized patient). In addition, socioeconomic indicators (e.g., living in urban or rural areas, income) were not available in our database. Both unavailable factors have associations with comorbidity and medication utilization [51, 52]. Considering these factors in further studies could be necessary for precise information on which subgroups of people with dementia infected with SARS-CoV-2 are vulnerable to comorbidity and prescriptions. Seventhly, a considerable proportion of included patients were recorded as “unknown ethnicity”. “Unknown ethnicity” status might include the patient not being asked their ethnicity, the patient declining to provide details, or the patient being unable to provide an answer (e.g., being too ill at initial registration, without an informant to provide these details). The first two situations are perhaps unlikely to be associated with mortality amongst relatively well patients, and an ethnicity bias amongst the “unknown” group is less likely because no other ethnicity differences in mortality were observed compared with White (Table 3), but the possibility that illness severity is related to ethnicity non-recording remains plausible.
In summary, we confirm excess mortality for those with dementia infected with SARS-CoV-2 that persists beyond the acute phase of illness and after discharge from hospital. This increased risk persisted up to, but not beyond, 125 days. We confirmed features previously associated with increased mortality in patients without dementia experiencing SARS-CoV-2 infection and found that these are also relevant in those suffering from dementia. In addition, we identified antipsychotic and benzodiazepine use as being associated with increased risk of death in this patient group, and verified that baseline data could be used to predict the risk of mortality with reasonable accuracy. These findings highlight the possibility of targeting care to patients with dementia who are at high risk of death after they have been discharged from hospital, with a view to decreasing mortality. Our findings also highlight the importance of including patients with dementia in trials designed to decrease longer-term mortality after acute SARS-CoV-2 infection, such as HEAL-COVID [53].
ACKNOWLEDGMENTS
The results reported in this publication are in part or entirely based on the analysis of electronic health record (EHR) data collated in the EpiCov database. The EpiCov database has been established by Cambridge University Hospitals in partnership with the Research Computing Services (RCS) at the University of Cambridge and other stakeholders to support COVID-19 relevant strategic monitoring and research analysis of EHR data with the aim to improve the care of individuals infected with SARS-CoV-2 at Cambridge University Hospitals and in other health and social care settings in the UK and beyond. Members of the clinical informatics team at CUH have been instrumental for preparing and pseudonymisation of EHR data before transfer to the EpiCov database.
We thank Mark R. Toshner (Department of Medicine, University of Cambridge) for conveying the existence of the EpiCov Database used in this study.
The EpiCov Database core group
Cambridge University Hospitals NHS Foundation Trust (CUH): Vince Taylor, Helen Street, Adam Loveday, Habeebat Ibraheem, Jacob Letowski, Peter Driscoll, Afzal Chaudhry; Research Computing Service (RCS), University of Cambridge: Mark Sharpley, Guilherme Balzana, Wojciech J Turek, Stuart J Rankin, Paul Calleja; NIHR Biomedical Research Center (BRC), CUH and University of Cambridge: Nicholas S Gleadall, Connor Rochford, John R Bradley, Willem H Ouwehand, Stefan Gräf.
FUNDING
SC and JOB were supported by the UK Alzheimer’s Society (grant AS-PG-16-006 to JOB) (https://www.alzheimers.org.uk/). RNC was supported by the Medical Research Council (grants MC_PC_17213, MR/W014386/1) (https://cambridgebrc.nihr.ac.uk/). BRU is partly funded by a donation from Gnodde Goldman Sachs Giving to the University of Cambridge. This research was supported in part by the UK National Institute for Health and Care Research (NIHR) Cambridge Biomedical Research Centre (BRC-1215-20014) (https://cambridgebrc.nihr.ac.uk/).
The funder of the study had no role in study design, data collection, data analysis, data interpretation, or writing of the article. The views expressed are those of the authors and not necessarily those of the NHS, the NIHR, or the Department of Health and Social Care.
CONFLICT OF INTEREST
SC declares no conflict of interest with this work.
RNC consults for Campden Instruments Ltd and receives royalties from Cambridge University Press, Cambridge Enterprise, and Routledge.
J. O’Brien has acted as a consultant for TauRx, Eisai, Novo Nordisk, Biogen and GE Healthcare and received grant support from Avid/ Lilly, Merck and Alliance Medical.
BRU is clinical director for older people’s and adult community services at CPFT. He is clinical director of the Windsor Unit at Fulbourn Hospital (CPFT), which delivers clinical trials in dementia/mild cognitive impairment for academic and commercial organisations without personal benefit, and is the clinical lead for dementia for the NIHR Clinical Research Network (CRN) in the East of England. His salary is part-funded by the NIHR CRN. His lectureship is funded by Gnodde Goldman-Sachs Giving. He has been principal investigator on trials for Axovant, Lilly, and EIP Pharma; his institution has benefited from payment for research carried out but he has not personally received any money. His wife is the lead for mental health for Suffolk Clinical Commissioning Group.
DATA AVAILABILITY
Patient-level data is not publicly available but can be accessed by researchers who meet the criteria for access to de-identified sensitive data under NHS Research Ethics terms. Full details of how to request access to CUH COVID-19-related data for research can be found at: https://www.hra.nhs.uk/planning-and-improving-research/application-summaries/research-summaries/epicov-version10/ or email for further information.
SUPPLEMENTARY MATERIAL
[1] The supplementary material is available in the electronic version of this article: https://dx.doi.org/10.3233/JAD-221093.
REFERENCES
[1] | Tortajada C , Navarro A , Andreu-Ballester JC , Mayor A , Añón S , Flores J ((2022) ) Prevalence and duration of symptoms among moderate and severe COVID-19 patients 12 months after discharge. Intern Emerg Med 17: , 929–934. |
[2] | Sibila O , Perea L , Albacar N , Moisés J , Cruz T , Mendoza N , Solarat B , Lledó G , Espinosa G , Barberà JA , Badia JR , Agustí A , Sellarés J , Faner R ((2022) ) Elevated plasma levelsof epithelial and endothelial cell markers in COVID-19 survivorswith reduced lung diffusing capacity six months after hospitaldischarge. Respir Res 23: , 37. |
[3] | Rivera-Izquierdo M , Láinez-Ramos-Bossini AJ , de Alba IG , Ortiz-González-Serna R , Serrano-Ortiz Á , Fernández-Martínez NF , Ruiz-Montero R , Cervilla JA ((2022) ) Long COVID 12 months after discharge: Persistent symptoms inpatients hospitalised due to COVID-19 and patients hospitalised dueto other causes-a multicentre cohort study. BMC Med 20: , 92. |
[4] | Ortelli P , Ferrazzoli D , Sebastianelli L , Maestri R , Dezi S , Spampinato D , Saltuari L , Alibardi A , Engl M , Kofler M , Quartarone A , Koch G , Oliviero A , Versace V ((2022) ) Altered motor cortex physiology and dysexecutive syndrome in patients with fatigue and cognitive difficulties after mild COVID-19. Eur J Neurol 29: , 1652–1662. |
[5] | Nersesjan V , Fonsmark L , Christensen RHB , Amiri M , Merie C , Lebech AM , Katzenstein T , Bang LE , Kjærgaard J , Kondziella D , Benros ME ((2022) ) Neuropsychiatric and cognitive outcomes in patients 6 monthsafter COVID-19 requiring hospitalization compared with matchedcontrol patients hospitalized for non-COVID-19 illness. JAMAPsychiatry 79: , 486–497. |
[6] | Mirfazeli FS , Sarabi-Jamab A , Pereira-Sanchez V , Kordi A , Shariati B , Shariat SV , Bahrami S , Nohesara S , Almasi-Dooghaee M , Faiz SHR ((2022) ) Chronic fatigue syndrome and cognitive deficit are associated with acute-phase neuropsychiatric manifestations of COVID-19: A 9-month follow-up study. Neurol Sci 43: , 2231–2239. |
[7] | Liu YH , Chen Y , Wang QH , Wang LR , Jiang L , Yang Y , Chen X , Li Y , Cen Y , Xu C , Zhu J , Li W , Wang YR , Zhang LL , Liu J , Xu ZQ , Wang YJ ((2022) ) One-year trajectory of cognitive changes in older survivors of COVID-19 in Wuhan, China: A longitudinal cohort study. JAMA Neurol 79: , 509–517. |
[8] | Kingery JR , Safford MM , Martin P , Lau JD , Rajan M , Wehmeyer GT , Li HA , Alshak MN , Jabri A , Kofman A , Babu CS , Benitez EK , Palacardo F , Das IG , Kaylor K , Woo KM , Roberts NL , Rahiel S , Gali V , Han L , Lee J , Roszkowska N , Kim YE , Bakshi S , Hogan C , McNairy M , Pinheiro LC , Goyal P ((2022) ) Health status, persistent symptoms, and effort intolerance one year after acute COVID-19 infection. J Gen Intern Med 37: , 1218–1225. |
[9] | Kim Y , Bitna H , Kim SW , Chang HH , Kwon KT , Bae S , Hwang S ((2022) ) Post-acute COVID-19 syndrome in patients after 12 months from COVID-19 infection in Korea. BMC Infect Dis 22: , 93. |
[10] | Ikonomidis I , Lambadiari V , Mitrakou A , Kountouri A , Katogiannis K , Thymis J , Korakas E , Pavlidis G , Kazakou P , Panagopoulos G , Andreadou I , Chania C , Raptis A , Bamias A , Thomas K , Kazakou P , Grigoropoulou S , Kavatha D , Antoniadou A , Dimopoulos MA , Filippatos G ((2022) ) Myocardial work and vascular dysfunction are partially improved at 12 months after COVID-19 infection. Eur J Heart Fail 24: , 727–729. |
[11] | Heesakkers H , van der Hoeven JG , Corsten S , Janssen I , Ewalds E , Simons KS , Westerhof B , Rettig TCD , Jacobs C , van Santen S , Slooter AJC , van der Woude MCE , van den Boogaard M , Zegers M ((2022) ) Clinical outcomes among patients with 1-year survival following intensive care unit treatment for COVID-19. JAMA 327: , 559–565. |
[12] | Fumagalli C , Zocchi C , Tassetti L , Silverii MV , Amato C , Livi L , Giovannoni L , Verrillo F , Bartoloni A , Marcucci R , Lavorini F , Fumagalli S , Ungar A , Olivotto I , Rasero L , Fattirolli F , Marchionni N ((2022) ) Factors associated with persistence of symptoms 1 year after COVID-19: A longitudinal, prospective phone-based interview follow-up cohort study. Eur J Intern Med 97: , 36–41. |
[13] | Frontera JA , Yang D , Medicherla C , Baskharoun S , Bauman K , Bell L , Bhagat D , Bondi S , Chervinsky A , Dygert L , Fuchs B , Gratch D , Hasanaj L , Horng J , Huang J , Jauregui R , Ji Y , Kahn DE , Koch E , Lin J , Liu S , Olivera A , Rosenthal J , Snyder T , Stainman R , Talmasov D , Thomas B , Valdes E , Zhou T , Zhu Y , Lewis A , Lord AS , Melmed K , Meropol SB , Thawani S , Troxel AB , Yaghi S , Balcer LJ , Wisniewski T , Galetta S ((2022) ) Trajectories of neurologic recovery 12 months after hospitalization for COVID-19: A prospective longitudinal study. Neurology 99: , e33–e45. |
[14] | Fischer D , Snider SB , Barra ME , Sanders WR , Rapalino O , Schaefer P , Foulkes AS , Bodien YG , Edlow BL ((2022) ) Disorders of consciousness associated with COVID-19: A prospective multimodal study of recovery and brain connectivity. Neurology 98: , e315–e325. |
[15] | Faverio P , Luppi F , Rebora P , D’Andrea G , Stainer A , Busnelli S , Catalano M , Modafferi G , Franco G , Monzani A , Galimberti S , Scarpazza P , Oggionni E , Betti M , Oggionni T , De Giacomi F , Bini F , Bodini BD , Parati M , Bilucaglia L , Ceruti P , Modina D , Harari S , Caminati A , Intotero M , Sergio P , Monzillo G , Leati G , Borghesi A , Zompatori M , Corso R , Valsecchi MG , Bellani G , Foti G , Pesci A ((2022) ) One-year pulmonary impairment after severe COVID-19: A prospective, multicenter follow-up study. Respir Res 23: , 65. |
[16] | Wu X , Liu X , Zhou Y , Yu H , Li R , Zhan Q , Ni F , Fang S , Lu Y , Ding X , Liu H , Ewing RM , Jones MG , Hu Y , Nie H , Wang Y ((2021) ) 3-month, 6-month, 9-month, and 12-month respiratory outcomes in patients following COVID-19-related hospitalisation: A prospective study. Lancet Respir Med 9: , 747–754. |
[17] | Writing Committee for the CSG, Morin L , Savale L , Pham T , Colle R , Figueiredo S , Harrois A , Gasnier M , Lecoq AL , Meyrignac O , Noel N , Baudry E , Bellin MF , Beurnier A , Choucha W , Corruble E , Dortet L , Hardy-Leger I , Radiguer F , Sportouch S , Verny C , Wyplosz B , Zaidan M , Becquemont L , Montani D , Monnet X ((2021) ) Four-month clinical status of a cohort of patients after hospitalization for COVID-19. JAMA 325: , 1525–1534. |
[18] | Huang C , Huang L , Wang Y , Li X , Ren L , Gu X , Kang L , Guo L , Liu M , Zhou X , Luo J , Huang Z , Tu S , Zhao Y , Chen L , Xu D , Li Y , Li C , Peng L , Li Y , Xie W , Cui D , Shang L , Fan G , Xu J , Wang G , Wang Y , Zhong J , Wang C , Wang J , Zhang D , Cao B ((2021) ) 6-month consequences of COVID-19 in patients discharged from hospital: A cohort study. Lancet 397: , 220–232. |
[19] | Ferrucci R , Dini M , Rosci C , Capozza A , Groppo E , Reitano MR , Allocco E , Poletti B , Brugnera A , Bai F , Monti A , Ticozzi N , Silani V , Centanni S , D’Arminio Monforte A , Tagliabue L , Priori A ((2022) ) One-year cognitive follow-up of COVID-19 hospitalized patients. Eur J Neurol 29: , 2006–2014. |
[20] | Douaud G , Lee S , Alfaro-Almagro F , Arthofer C , Wang C , McCarthy P , Lange F , Andersson JLR , Griffanti L , Duff E , Jbabdi S , Taschler B , Keating P , Winkler AM , Collins R , Matthews PM , Allen N , Miller KL , Nichols TE , Smith SM ((2022) ) SARS-CoV-2 is associated with changes in brain structure in UK Biobank. Nature 604: , 697–707. |
[21] | Lyra ESNM , Barros-Aragão FGQ , De Felice FG , Ferreira ST ((2022) ) Inflammation at the crossroads of COVID-19, cognitive deficits and depression. Neuropharmacology 209: , 109023. |
[22] | Lozada-Martínez ID , Díaz-Castillo OJ , Pearson-Arrieta AC , Galeano-Buelvas A , Moscote-Salazar LR ((2022) ) Post-COVID 19 neurological syndrome: A new risk factor that modifies the prognosis of patients with dementia. Alzheimers Dement 18: , 542–543. |
[23] | Mainous AG 3rd, Rooks BJ , Wu V , Orlando FA ((2021) ) COVID-19 post-acute sequelae among adults: 12 month mortality risk. Front Med (Lausanne) 8: , 778434. |
[24] | Bhaskaran K , Rentsch CT , Hickman G , Hulme WJ , Schultze A , Curtis HJ , Wing K , Warren-Gash C , Tomlinson L , Bates CJ , Mathur R , MacKenna B , Mahalingasivam V , Wong A , Walker AJ , Morton CE , Grint D , Mehrkar A , Eggo RM , Inglesby P , Douglas IJ , McDonald HI , Cockburn J , Williamson EJ , Evans D , Parry J , Hester F , Harper S , Evans SJ , Bacon S , Smeeth L , Goldacre B ((2022) ) Overall and cause-specific hospitalisation and death after COVID-19 hospitalisation in England: A cohort study using linked primary care, secondary care, and death registration data in the OpenSAFELY platform. PLoS Med 19: , e1003871. |
[25] | Williamson EJ , Walker AJ , Bhaskaran K , Bacon S , Bates C , Morton CE , Curtis HJ , Mehrkar A , Evans D , Inglesby P , Cockburn J , McDonald HI , MacKenna B , Tomlinson L , Douglas IJ , Rentsch CT , Mathur R , Wong AYS , Grieve R , Harrison D , Forbes H , Schultze A , Croker R , Parry J , Hester F , Harper S , Perera R , Evans SJW , Smeeth L , Goldacre B ((2020) ) Factors associated with COVID-19-related death using OpenSAFELY. Nature 584: , 430–436. |
[26] | Kim J , Blaum C , Ferris R , Arcila-Mesa M , Do H , Pulgarin C , Dolle J , Scherer J , Kalyanaraman Marcello R , Zhong J ((2022) ) Factors associated with hospital admission and severe outcomes for older patients with COVID-19. J Am Geriatr Soc 70: , 1906–1917. |
[27] | Wang Q , Davis PB , Gurney ME , Xu R ((2021) ) COVID-19 and dementia: Analyses of risk, disparity, and outcomes from electronic health records in the US. Alzheimers Dement 17: , 1297–1306. |
[28] | Vrillon A , Mhanna E , Aveneau C , Lebozec M , Grosset L , Nankam D , Albuquerque F , Razou Feroldi R , Maakaroun B , Pissareva I , Cherni Gherissi D , Azuar J , François V , Hourrègue C , Dumurgier J , Volpe-Gillot L , Paquet C ((2021) ) COVID-19 in adults with dementia: Clinical features and risk factors of mortality-a clinical cohort study on 125 patients. Alzheimers Res Ther 13: , 77. |
[29] | Tahira AC , Verjovski-Almeida S , Ferreira ST ((2021) ) Dementia is an age-independent risk factor for severity and death in COVID-19 inpatients. Alzheimers Dement 17: , 1818–1831. |
[30] | Chen S , Jones PB , Underwood BR , Fernandez-Egea E , Qin P , Lewis JR , Cardinal RN ((2021) ) Risk factors for excess deaths during lockdown among older users of secondary care mental health services without confirmed COVID-19: A retrospective cohort study. International Journal of Geriatric Psychiatry 36: , 1899–1907. |
[31] | Cambridge University Hospitals NHS Foundation Trust (2021) Annual Report and Accounts 2020/21. Cambridge. https://buckupcuh-production.s3.amazonaws.com/documents/Annual_Report_and_Accounts_2020-21_FULL_VERSION.pdf |
[32] | Gouliouris T , Warne B , Cartwright EJP , Bedford L , Weerasuriya CK , Raven KE , Brown NM , Torok ME , Limmathurotsakul D , Peacock SJ ((2018) ) Duration of exposure to multiple antibiotics is associated with increased risk of VRE bacteraemia: A nested case-control study. J Antimicrob Chemother 73: , 1692–1699. |
[33] | Nemani K , Li C , Olfson M , Blessing EM , Razavian N , Chen J , Petkova E , Goff DC ((2021) ) Association of psychiatric disorders with mortality among patients with COVID-19. JAMA Psychiatry 78: , 380–386. |
[34] | Jeon HL , Kwon JS , Park SH , Shin JY ((2021) ) Association of mental disorders with SARS-CoV-2 infection and severe health outcomes: Nationwide cohort study. Br J Psychiatry 218: , 344–351. |
[35] | Price A , Farooq R , Yuan JM , Menon VB , Cardinal RN , O’Brien JT ((2017) ) Mortality in dementia with Lewy bodies compared with Alzheimer’s dementia: A retrospective naturalistic cohort study. BMJ Open 7: , e017504. |
[36] | Charlson ME , Pompei P , Ales KL , MacKenzie CR ((1987) ) A new method of classifying prognostic comorbidity in longitudinal studies: Development and validation. J Chronic Dis 40: , 373–383. |
[37] | Quan H , Sundararajan V , Halfon P , Fong A , Burnand B , Luthi JC , Saunders LD , Beck CA , Feasby TE , Ghali WA ((2005) ) Coding algorithms for defining comorbidities in ICD-9-CM and ICD-10 administrative data. Med Care 43: , 1130–1139. |
[38] | Chawla NV , Bowyer KW , Hall LO , Kegelmeyer WP ((2002) ) SMOTE: Synthetic minority over-sampling technique. J Artif Intell Res 16: , 321–357. |
[39] | Ceccato A , Pérez-Arnal R , Motos A , Barbé F , Torres A ((2022) ) One-year mortality after ICU admission due to COVID-19 infection. Intensive Care Med 48: , 366–368. |
[40] | Carriazo S , Mas-Fontao S , Seghers C , Cano J , Goma E , Avello A , Ortiz A , Gonzalez-Parra E ((2022) ) Increased 1-year mortality in haemodialysis patients with COVID-19: A prospective, observational study. Clin Kidney J 15: , 432–441. |
[41] | Berni A , Malandrino D , Corona G , Maggi M , Parenti G , Fibbi B , Poggesi L , Bartoloni A , Lavorini F , Fanelli A , Scocchera G , Nozzoli C , Peris A , Pieralli F , Pini R , Ungar A , Peri A ((2021) ) Serum sodium alterations in SARS CoV-2 (COVID-19) infection: Impact on patient outcome. Eur J Endocrinol 185: , 137–144. |
[42] | García-Grimshaw M , Chirino-Pérez A , Flores-Silva FD , Valdés-Ferrer SI , Vargas-Martínez M , Jiménez-ÁvilaAI , Chávez-Martínez OA , Ramos-Galicia EM , Marché-Fernández OA , Ramírez-Carrillo MF , Grajeda-González SL , Ramírez-Jiménez ME , Chávez-Manzanera EA , Tusié-Luna MT , Ochoa-Guzmán A , Cantú-Brito C , Fernandez-Ruiz J , Chiquete E ((2022) ) Critical roleof acute hypoxemia on the cognitive impairment after severe COVID-19pneumonia: A multivariate causality model analysis. NeurolSci 43: , 2217–2229. |
[43] | Wang SM , Park SH , Kim NY , Kang DW , Na HR , Um YH , Han S , Park SS , Lim HK ((2021) ) Association between dementia and clinical outcome after COVID-19: A nationwide cohort study with propensity score matched control in South Korea. Psychiatry Investig 18: , 523–529. |
[44] | Cornejo-Pareja I , Vegas-Aguilar IM , Garcia-Almeida JM , Bellido-Guerrero D , Talluri A , Lukaski H , Tinahones FJ ((2022) ) Phase angle and standardized phase angle from bioelectrical impedance measurements as a prognostic factor for mortality at 90 days in patients with COVID-19: A longitudinal cohort study. Clin Nutr 41: , 3106–3114. |
[45] | Weber GM , Hong C , Xia Z , Palmer NP , Avillach P , L’Yi S , Keller MS , Murphy SN , Gutiérrez-Sacristán A , Bonzel CL , Serret-Larmande A , Neuraz A , Omenn GS , Visweswaran S , Klann JG , South AM , Loh NHW , Cannataro M , Beaulieu-Jones BK , Bellazzi R , Agapito G , Alessiani M , Aronow BJ , Bell DS , Benoit V , Bourgeois FT , Chiovato L , Cho K , Dagliati A , DuVall SL , Barrio NG , Hanauer DA , Ho YL , Holmes JH , Issitt RW , Liu M , Luo Y , Lynch KE , Maidlow SE , Malovini A , Mandl KD , Mao C , Matheny ME , Moore JH , Morris JS , Morris M , Mowery DL , Ngiam KY , Patel LP , Pedrera-Jimenez M , Ramoni RB , Schriver ER , Schubert P , Balazote PS , Spiridou A , Tan ALM , Tan BWL , Tibollo V , Torti C , Trecarichi EM , Wang X ; Consortium for ClinicalCharacterization of COVID-19 by EHR (4CE); Kohane IS , Cai T , Brat GA ((2022) ) International comparisons of laboratory values from the 4CEcollaborative to predict COVID-19 mortality.. NPJ Digit Med 5: , 74. |
[46] | Gruys E , Toussaint MJ , Niewold TA , Koopmans SJ ((2005) ) Acute phase reaction and acute phase proteins. J Zhejiang Univ Sci B 6: , 1045–1056. |
[47] | Phiri P , Engelthaler T , Carr H , Delanerolle G , Holmes C , Rathod S ((2022) ) Associated mortality risk of atypical antipsychotic medication in individuals with dementia. World J Psychiatry 12: , 298–307. |
[48] | Norgaard A , Jensen-Dahm C , Gasse C , Wimberley T , Hansen ES , Waldemar G ((2020) ) Association of benzodiazepines and antidepressants with 180-day mortality among patients with dementia receiving antipsychotic pharmacotherapy: A nationwide registry-based study. J Clin Psychiatry 81: , 19m12828. |
[49] | Freudenberg-Hua Y , Makhnevich A , Li W , Liu Y , Qiu M , Marziliano A , Carney M , Greenwald B , Kane JM , Diefenbach M , Burns E , Koppel J , Sinvani L ((2022) ) Psychotropic medication use is associated with greater 1-year incidence of dementia after COVID-19 hospitalization. Front Med (Lausanne) 9: , 841326. |
[50] | van de Beek M , van Steenoven I , Ramakers I , Aalten P , Koek HL , Olde Rikkert MGM , Mannien J , Papma JM , de Jong FJ , Lemstra AW , van der Flier WM ((2019) ) Trajectories and determinants of quality of life in dementia with Lewy bodies and Alzheimer’s disease. J Alzheimers Dis 70: , 389–397. |
[51] | Sigglekow F , Wilson N , Blakely T ((2023) ) Income and economic productivity loss associated with comorbidity: Longitudinal analysis of linked individual-level data for a whole country. J Epidemiol Community Health 77: , 97–100. |
[52] | Pedrosa-Naudin MA , Gutierrez-Abejon E , Herrera-Gomez F , Fernandez-Lazaro D , Alvarez FJ ((2022) ) Non-adherence to antidepressant treatment and related factors in a region of Spain: A population-based registry study. Pharmaceutics 14: , 2696. |
[53] | Summers C , Helping alleviate the longerterm consequences of COVID-19, https://www.isrctn.com/ISRCTN15851697, Accessed May 20, 2022. |