SWADESH: A Comprehensive Platform for Multimodal Data and Analytics for Advanced Research in Alzheimer’s Disease and Other Brain Disorders
Alzheimer’s disease (AD), the most common neurodegenerative disorder, affects millions of people worldwide annually. The scientific community has been using neuroimaging data extensively for understanding its cause, progression, and therapeutic development. AD-specific open-source databases are primarily imaging-based and lack neurochemical, genetic, and epigenetic information. To identify early diagnostic biomarkers of AD, it is crucial to use holistic approaches that assess neurochemical as well as structural alterations of the brain. Neuroimaging is a powerful technique to study structural, functional, and pharmacological impact of the nervous system. In 1971, computed tomography (CT) was clinically introduced to examine the internal structure of the brain. Later, in the early 1980s, magnetic resonance imaging (MRI) was developed for investigating noninvasively various anatomical characteristics of the human brain. Subsequently, in the 1990s, functional MRI (fMRI) came to be a dominant brain mapping technique for measuring brain activity associated with changes in the blood flow [1]. Various MRI techniques, such as diffusion-weighted MRI, quantitative susceptibility mapping (QSM), multinuclear MR spectroscopy (MRS), and arterial spin-labeled MRI, are also generating complementary functional, neurochemical, and metabolic information. Positron emission tomography (PET) is another modality that holds a unique potential in characterizing cerebral metabolism and neurotransmitter action through nuclear imaging technology. Moreover, the advent of various brain computer interfaces and other modalities, such as electroencephalography (EEG), magnetoencephalography (MEG), and functional near infrared spectroscopy, has substantially accelerated neuroscience research globally [2]. These imaging modalities provide critical features for the understanding of the early disease process.
Neuroimaging-based AD research is primarily conducted including healthy old, mild cognitive impaired, and AD-affected individuals, but is not often compared with other disorders, such as schizophrenia, bipolar disorder, and epilepsy. To conduct an in-depth analysis using data-driven methods, the range of data, including that obtained from patients with neurodegenerative, neuropsychiatric, and neurodevelopmental disorders and healthy controls, should be expanded. It will give substantial insights into AD-specific biomarkers. Having enough multimodal neuroimaging data from AD cases and other conditions (Fig. 1) will allow researchers to strengthen existing hypotheses and differentiate neuropsychiatric and neurodevelopmental diseases. In this editorial, we introduce project SWADESH, a novel brain research initiative that covers a comprehensive database and data analytics platforms. Project SWADESH aims to build India’s brain initiative focusing on neuroimaging, neurochemical, neuropsychological data, and analytics for AD and various brain disorders. This project is unique as it contains certified data where researchers can access neuroimaging data and comparative analyses of various disorders. This approach is elaborated sequentially.
Fig. 1
Framework for SWADESH: a comprehensive platform for multimodal neuroimaging data, quality control, and data analytics. The major components are: (a) data acquisition, (b) big data management and analytics framework, and (c) visualization and applications.
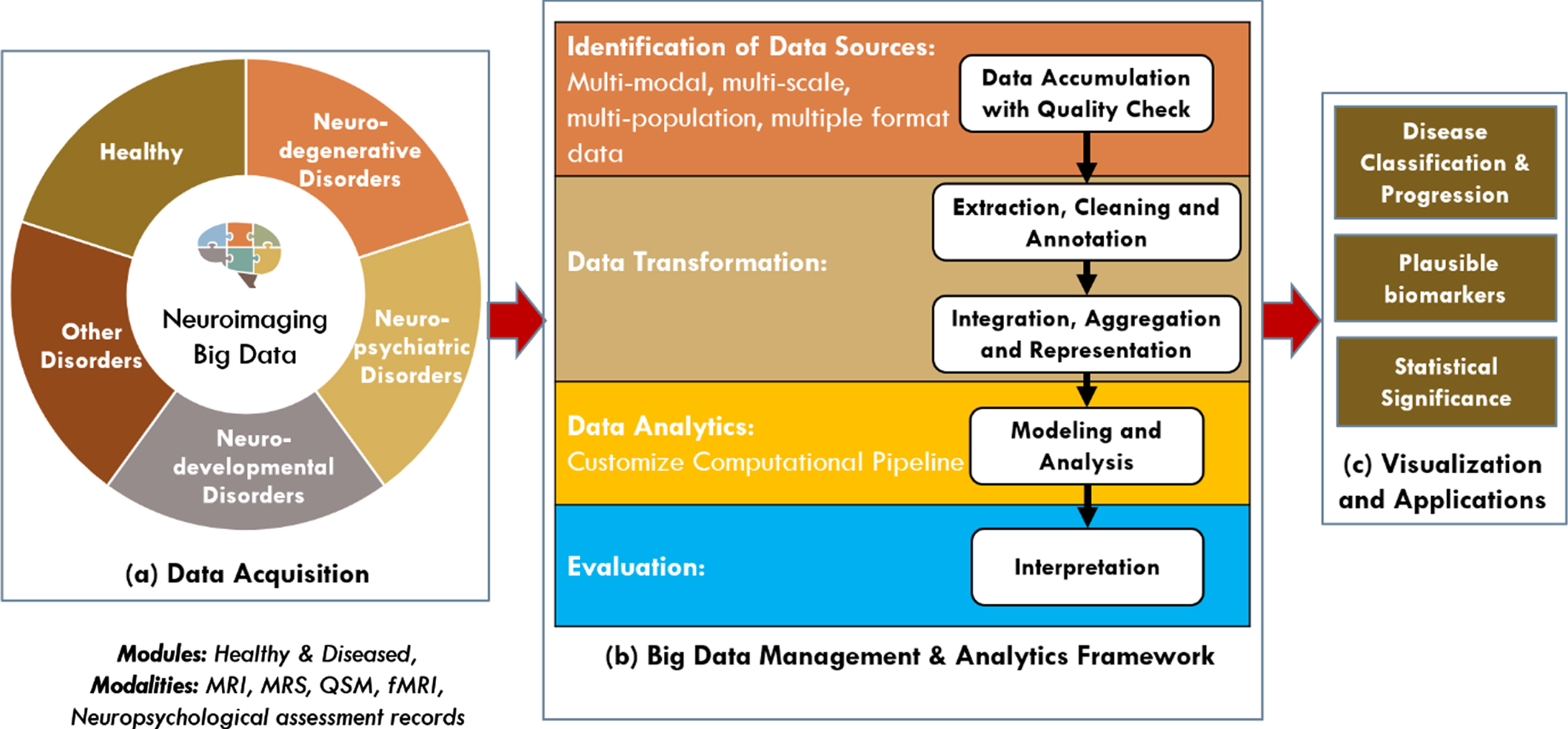
The first AD-specific database was reported by the National Alzheimer’s Coordinating Center, which included MRI, genetic, and behavioral data of AD-affected and healthy old individuals [3]. Due to an increase in the number of experiments, heterogeneous datasets were filled in, which necessitated standardization and distribution. This led to the development of the Image and Data Archive (IDA) initiative by the US Laboratory for Neuroimaging (LONI) [4]. This IDA–LONI platform houses hundreds of disease-related datasets and continues to add new datasets with user-friendly functionalities. Alzheimer’s Disease Neuroimaging Initiative (ADNI) [5] and Human Connectome [6] are also linked with IDA–LONI. Subsequently, the Longitudinal Online Research Imaging System (LORIS) [7] was developed by McGill University that hosts behavioral, clinical, neuroimaging, and genetic data. It is a web-based platform that provides a framework for storing and processing data. The accessibility of worldwide datasets is expanding, and databases are getting enriched in terms of the quantity, quality, and usage of neuroimaging modalities. The Mind Research Institute, USA, developed Collaborative Informatics and Neuroimaging Suite comprising nearly 300 studies with 19,000 MRI, MEG, and EEG assessments [8]. Recently, the latest version of Open Access Series of Imaging Study (OASIS)-3 [9] included longitudinal neuroimaging technique based on MRI and PET as well as clinical and cognitive assessments of normal aging and AD. Another project ANSH developed at National Brain Research Centre (NBRC), India, included glutathione, gamma aminobutyric acid levels, and other neurochemicals using MRS for the first time along with MRI-based structural information for healthy old, mild cognitive impaired, and AD-affected individuals [10].
A huge number of databases are available for specific neuropsychiatric and neurodevelopmental disorders; e.g., SchizConnect [11] is an open database containing structural, diffusion, and fMRI-based data of patients with schizophrenia and bipolar disorders along with healthy controls. The Bipolar Disorder Neuroimaging Database [12] contains meta-analyses of studies utilizing MRI and CT to study brain structure in patients with bipolar disorders in comparison to a control group. The Autism Brain Imaging Data Exchange [13] is a large collection of multisite, multimodal, and resting state data collected from participants with autism spectrum disorder and healthy children. IEEG portal [14] contains a repository for high-quality intracranial EEG data from patients with epilepsy. This cloud-based platform enables data sharing and offers neural data analysis to users. Similarly, several databases are available for Parkinson’s disease (PD), namely Parkinson Progression Marker Initiative [15] and Quebec Parkinson Network [16], that contain multimodal neuroimaging data. The list of databases for various diseases is huge and expanding. These heterogeneous neuroimaging datasets can be managed individually, but as a group, they are too complex to be processed by a single processing system. Open-sharing platforms allow easy accessibility of various multimodal data. However, data organization and analysis are crucial for analyzing a particular disease because of heterogeneities at various levels from different datasets. These concerns require intelligent organization and analysis of neuroimaging big data for healthy and diseased states and the prevention of redundant data collection. This calls for an association for standardization, sharing, management, and analysis of multimodal neuroimaging big data for conclusive understanding of various brain disorders.
The majority of large-scale accessible neuroimaging platforms are MRI-based, e.g., OpenNeuro [17] has established an open archive of neuroimaging data consisting of nearly 500 human datasets with majority MRI-based data obtained from various healthy and diseased brains. It also includes data from other modalities, such as EEG, MEG, and PET. On the other hand, the Neuro-Imaging Tools and Resource Collaboratory [18] hosts various neuroimaging software repositories, toolboxes, and raw data under one roof. The resources of this platform have expanded over time to cover various domains, including MR, PET, CT, optical imaging, MEG, EEG, genetic imaging, clinical neuroinformatics, and computational neuroscience. It hosts thousands of public datasets of normal and diseased subjects, including those for dementia, attention deficit hyperactivity disorder, PD, schizophrenia, and autism. Various country-specific brain projects, huge-scale data collection initiatives, and international alliances have been working for sharing neuroimaging data to understand the complexities of the brain. The US Brain Research through Advancing Innovative Neurotechnologies (BRAIN) initiative was launched in 2013 with the aim of developing new tools for discoveries related to neural circuit functioning in health and disease [19]. In the same year, the European Union launched the Human Brain Project for focusing on building large-scale computational models and neuroinformatic standards for neuroscience databases. Subsequently, in 2014, Japan initiated the brain mapping by integrated neuro-technologies for disease-specific studies. This initiative focuses on genetic of primate models for understanding brain mechanisms and modeling brain disorders. The Brain Canada project has a vision of collaborative research for understanding the brain in health and diseases. Similarly, national projects were launched by Australia, Korea, Israel, and China with different emphases [19].
The Indian subcontinent, which is a home to >1.4 billion people with vast human diversity, has a population-specific brain morphology. It was found that there were variations in the size, volume, and location of certain brain regions in several Indian population groups compared to those observed in populations of other countries. An Indian brain template BRAHMA was developed at NBRC [20]. Therefore, morphological features specific to a population are important when dealing with structural MRI datasets. ANSH database provides a dynamic comprehensive database system for managing heterogeneous neuroimaging datasets made for the Indian population, particularly MRI, multinuclear MRS, and neuropsychological test scores for healthy senior citizens and MCI and AD categories [10]. This simple dynamic distributed platform is supported by JAVA-based workflow environment and Python. It also provides quality control, data analysis reports, and backups of data, supported by using a dedicated storage system. Its development will facilitate the integration of multi-site data and collaborative research around the globe [10].
The subsequent project launched by NBRC was BHARAT, an integrated big-data analytic model for early diagnostic biomarkers of AD [21]. The design included a Hadoop-based big-data framework integrating MRI, MRS, and neuropsychological test scores. Efforts have been made to expand these projects and include rich multimodal neuroimaging datasets for healthy and diseased cases.
Project SWADESH proposed a big-data architecture for managing five modules as follows: (a) neurodegenerative (AD, PD, etc.), (b) neuropsychiatric (schizophrenia, bipolar disorder, etc.), (c) neurodevelopmental (autism, epilepsy, etc.), (d) other disorders, and (e) healthy subjects (Fig. 1). In the initial phase, project SWADESH aims to integrate neuroimaging data generated through MRI, MRS, QSM, fMRI, and neuropsychological assessment at NBRC and its collaborating institutes. The project focuses on integrating a quality-control system using GANGOTRI pipeline for the raw data of each modality prior to placing the data into the integrated system. To the best of our knowledge, this is the first large-scale multimodal neuroimaging database initiative designed specifically for the Indian population with big-data architecture and data analytics for various disease categories under one platform. This is a sincere attempt towards building and enriching a multidimensional neuroimaging platform for identification of the causal molecular processes of various brain disorders. Identification of the molecular cause is urgently required for AD and other disorders that will lead to effective clinical trials for therapeutic development [22]. SWADESH project is designed in this focused approach to identify specific characteristic features directly linked to the disease. Artificial intelligence and machine learning algorithms [23] are the backbone to SWADESH project.
ACKNOWLEDGMENTS
Prof. Pravat K. Mandal thanks Dr. Yashika Arora (Scientist, NINS Lab) for helping to construct critical information. Thanks to Mr. Kuldeep Singh (R&D engineer, NINS Lab) and Ms. Komal Jindal (Senior R&D engineer, NINS lab) for building critical infrastructure of SWADESH project. Financial support from the Department of Biotechnology (Tata Innovation Fellowship to PKM, and Indo Australian strategic funding from Department of Biotechnology, Ministry of Science and Technology, Govt. of India to PKM) is highly acknowledged. Partial financial support from NBRC flagship program entitled “Comparative mapping of common mental disorder over lifespan” (Role: Program Coordinator) (Department of Biotechnology, Govt. of India).
George Perry is the Editor-in-Chief of the Journal of Alzheimer’s Disease, but was not involved in the peer-review process nor had access to any information regarding this article’s peer review.
Authors’ disclosures available online (https://www.j-alz.com/manuscript-disclosures/21-5354r1).
REFERENCES
[1] | Leeds NE , Kieffer SA ((2000) ) Evolution of diagnostic neuroradiology from 1904 to 1999. Radiology 217: , 309–318. |
[2] | Gui X , Chuansheng C , Zhong-Lin L , Qi D ((2010) ) Brain imaging techniques and their applications in decision-making research. Xin li xue bao Acta Psychol Sin 42: , 120. |
[3] | Beekly DL , Ramos EM , van Belle G , Deitrich W , Clark AD , Jacka ME , Kukull WA ((2004) ) The national Alzheimer’s coordinating center (NACC) database: An Alzheimer disease database. Alzheimer Dis Assoc Disord 18: , 270–277. |
[4] | Crawford KL , Neu SC , Toga AW ((2016) ) The image and data archive at the laboratory of neuro imaging. Neuroimage 124: , 1080–1083. |
[5] | Petersen RC , Aisen P , Beckett LA , Donohue M , Gamst A , Harvey DJ , Jack C , Jagust W , Shaw L , Toga A ((2010) ) Alzheimer’s disease neuroimaging initiative (ADNI): Clinical characterization. Neurology 74: , 201–209. |
[6] | Rosen B , Wedeen VJ , Horn J , Fischl B , Buckner RL , Wald L , Hamalainen M , Stufflebeam S , Roffman J , Shattuck DW ((2010) ) The human connectome project. Proceedings Organization for Human Brain Mapping 16th Annual Meeting, Barcelona. |
[7] | Das S , Zijdenbos AP , Vins D , Harlap J , Evans AC ((2012) ) LORIS: A web-based data management system for multi-center studies. Front Neuroinform 5: , 37. |
[8] | Scott A , Courtney W , Wood D , De la Garza R , Lane S , Wang R , King M , Roberts J , Turner JA , Calhoun VD ((2011) ) COINS: An innovative informatics and neuroimaging tool suite built for large heterogeneous datasets. Front Neuroinform 5: , 33. |
[9] | LaMontagne PJ , Benzinger TL , Morris JC , Keefe S , Hornbeck R , Xiong C , Grant E , Hassenstab J , Moulder K , Vlassenko A ((2019) ) OASIS-3:Longitudinal neuroimaging, clinical, and cognitive dataset fornormal aging and Alzheimer disease. MedRxiv 2019.12.13.19014902. |
[10] | Mandal PK , Sandal K , Shukla D , Tripathi M , Singh K , Roy S ((2020) ) ANSH: Multimodal neuroimaging database including MR spectroscopic data from each continent to advance Alzheimer’s disease research. Front Neuroinform 14: , 43. |
[11] | Wang L , Alpert KI , Calhoun VD , Cobia DJ , Keator DB , King MD , Kogan A , Landis D , Tallis M , Turner MD ((2016) ) SchizConnect: Mediating neuroimaging databases on schizophrenia and related disorders for large-scale integration. Neuroimage 124: , 1155–1167. |
[12] | Kempton MJ , Geddes JR , Ettinger U , Williams SC , Grasby PM ((2008) ) Meta-analysis, database, and meta-regression of 98 structural imaging studies in bipolar disorder. Arch Gen Psychiatry 65: , 1017–1032. |
[13] | Di Martino A , Yan C-G , Li Q , Denio E , Castellanos FX , Alaerts K , Anderson JS , Assaf M , Bookheimer SY , Dapretto M ((2014) ) The Autism Brain Imaging Data Exchange: Towards a large-scale evaluation of the intrinsic brain architecture in autism. Mol Psychiatry 19: , 659–667. |
[14] | Wagenaar JB , Brinkmann BH , Ives Z , Worrell GA , Litt B ((2013) ) A multimodal platform for cloud-based collaborative research. 2013 6th international IEEE/EMBS conference on neural engineering (NER) IEEE, pp. 1386–1389. |
[15] | Marek K , Jennings D , Lasch S , Siderowf A , Tanner C , Simuni T , Coffey C , Kieburtz K , Flagg E , Chowdhury S ((2011) ) The Parkinson progression marker initiative (PPMI). Prog Neurobiol 95: , 629–635. |
[16] | Gan-Or Z , Rao T , Leveille E , Degroot C , Chouinard S , Cicchetti F , Dagher A , Das S , Desautels A , Drouin-Ouellet J ((2020) ) The Quebec Parkinson network: A researcher-patient matching platform and multimodal biorepository. J Parkinsons Dis 10: , 301–313. |
[17] | Gorgolewski K , Esteban O , Schaefer G , Wandell B , Poldrack R ((2017) ) OpenNeuro—a free online platform for sharing and analysis of neuroimaging data. Organization for human brain mapping Vancouver, Canada, 1677. |
[18] | Kennedy DN , Haselgrove C , Riehl J , Preuss N , Buccigrossi R ((2016) ) The NITRC image repository. Neuroimage 124: , 1069–1073. |
[19] | Yuste R , Bargmann C ((2017) ) Toward a global BRAIN initiative. Cell 168: , 956–959. |
[20] | Pai PP , Mandal PK , Punjabi K , Shukla D , Goel A , Joon S , Roy S , Sandal K , Mishra R , Lahoti R ((2020) ) BRAHMA: Population specific t1, t2, and FLAIR weighted brain templates and their impact in structural and functional imaging studies. Magn Reson Imaging 70: , 5–21. |
[21] | Sharma A , Shukla D , Goel T , Mandal PK ((2019) ) BHARAT: An integrated big data analytic model for early diagnostic biomarker of Alzheimer’s disease. Front Neurol 10: , 9. |
[22] | Mandal PK , Samkaria A , Maroon JC ((2021) ) AD hypotheses and suggested clinical trials. ACS Chem Neurosci 12: , 3968–3971. |
[23] | SharmaS, MandalPK ((2021) ) A Comprehensive Report on Machine Learning-based Detection of AD using Multimodal Neuroimaging Data. ACM Computing Survey, doi: 10.1145/3492865. |