Aggregate Trends of Apolipoprotein E on Cognition in Transgenic Alzheimer’s Disease Mice
Abstract
Background:
Apolipoprotein E (APOE) genotypes typically increase risk of amyloid-β deposition and onset of clinical Alzheimer’s disease (AD). However, cognitive assessments in APOE transgenic AD mice have resulted in discord.
Objective:
Analysis of 31 peer-reviewed AD APOE mouse publications (n = 3,045 mice) uncovered aggregate trends between age, APOE genotype, gender, modulatory treatments, and cognition.
Methods:
T-tests with Bonferroni correction (significance = p < 0.002) compared age-normalized Morris water maze (MWM) escape latencies in wild type (WT), APOE2 knock-in (KI2), APOE3 knock-in (KI3), APOE4 knock-in (KI4), and APOE knock-out (KO) mice. Positive treatments (t+) to favorably modulate APOE to improve cognition, negative treatments (t–) to perturb etiology and diminish cognition, and untreated (t0) mice were compared. Machine learning with random forest modeling predicted MWM escape latency performance based on 12 features: mouse genotype (WT, KI2, KI3, KI4, KO), modulatory treatment (t+, t–, t0), mouse age, and mouse gender (male = g_m; female = g_f, mixed gender = g_mi).
Results:
KI3 mice performed significantly better in MWM, but KI4 and KO performed significantly worse than WT. KI2 performed similarly to WT. KI4 performed significantly worse compared to every other genotype. Positive treatments significantly improved cognition in WT, KI4, and KO compared to untreated. Interestingly, negative treatments in KI4 also significantly improved mean MWM escape latency. Random forest modeling resulted in the following feature importance for predicting superior MWM performance: [KI3, age, g_m, KI4, t0, t+, KO, WT, g_mi, t–, g_f, KI2] = [0.270, 0.094, 0.092, 0.088, 0.077, 0.074, 0.069, 0.061, 0.058, 0.054, 0.038, 0.023].
Conclusion:
APOE3, age, and male gender was most important for predicting superior mouse cognitive performance.
INTRODUCTION
Alzheimer’s disease and the Apolipoprotein E gene
Alzheimer’s disease (AD) is a neurodegenerative disorder that gradually impairs cognition in patients suffering from the condition [1] and is considered to be a polygenic disease [2]. The Apolipoprotein E (APOE) gene synthesizes various isoforms of apoE, a biomarker strongly hypothesized to be implicated in AD, as initially observed by researchers at the Duke Alzheimer’s Disease Research Center in 1993 [3–5]. The biochemical exhibits three common isoforms in humans: apoE2, apoE3, and apoE4, of which apoE2 [6] and apoE3 [7] have been shown to confer protective effects, while apoE4 has been documented to increase cognitive impairment [7–10]. The protein apoE primarily functions in brain lipid transport, glucose metabolism, and neuronal signaling, among other roles [11]. Its biochemical pathway involves binding to surface apoE receptors on cells throughout the body to initiate further metabolism, transport, and signaling, but the single amino acid differences between apoE2, E3, and E4 result in a diversity of protein structures that, therefore, affect receptor binding in different ways respective to each isoform [12]. For instance, apoE3 and E4 display 50-fold greater binding affinity when compared to apoE2 [12].
Moreover, through yet-uncovered mechanisms, APOE isoforms are also believed to accentuate or attenuate pathways involving other well-established AD biomarkers, such as amyloid-β (Aβ) [13] and p-tau [14]. The amyloid cascade hypothesis posits that the deposition of Aβ plaques from improper cleavage of amyloid-β protein precursor is the most important molecule in AD pathogenesis, but heterogeneity of results in the literature has challenged this notion [15, 16]. For example, Foley et al. showed that mice with increased Aβ levels do not perform significantly worse on cognitive assessments than mice with lower levels of Aβ, suggesting that the plaques could instead be a side effect of AD pathogenesis [13]. The interaction between APOE isoforms and amyloid metabolism was examined to show that APOE4 was associated with elevated Aβ secretion and defective Aβ uptake compared to isogenic APOE3 human brain cells [8]. This interplay of APOE isoforms with the amyloid cascade further strengthens the literature trend that APOE4 confers negative effects on cognition since conversion of apoE4 to E3 repeatedly mitigated various pathologies related to AD [8]. Lastly, meta-analysis work revealed that p-tauopathy is a greater predictor of cognitive decline than Aβ in mouse models [14].
AD APOE mouse model
Endogenous mouse apoE is structurally different from human isoforms, so the most prominent APOE mouse models use human isoforms for study [13, 17]. The selection of appropriate promoters and other regulatory elements is important to note since APOE4 expressed in neurons have demonstrated more detrimental effects compared to its expression in astrocytes [18]. Furthermore, mouse APOE4 knock-in (KI) groups display neural plasticity defects that appear to be absent in APOE3 KI groups [19–21].
Genotype effect on AD
Extensive research has been conducted on transgenic mouse models to investigate the effects of various features, such as genotype, treatment, age, and gender on AD pathogenesis. Because of the high-dimensional nature of AD diagnosis, there is heterogeneity within the literature about the importance of such features [13]. For example, Shi et al. demonstrated that APOE4 KI mice exert a gain of toxic function while APOE knock-out (KO) mice instead displayed protective effects [22]. However, others have found conflicting results among the same genotypic groups. Zerbi et al. found that both APOE4 KI and APOE KO mice demonstrate a comparable magnitude of brain functional connectivity deficiencies in progressing from adulthood (12 months) to old age (18 months) [23]. Thus, uncovering aggregate trends is pivotal to AD APOE research. But genotype is not the only feature whose effect on AD is still under debate.
Treatment effect on AD
The effects of various forms of treatment have shown similarly unclear findings. While prior studies have tested treatment regimens that may be promising avenues for remediating AD progression in transgenic mouse models [24], the overall efficacy of treatment is still undetermined. For instance, pharmacological targeting of the apoE/Aβ interaction pathway significantly reduced aggregation of both apoE and Aβ in APOE2 and APOE4 KI mice, preventing memory decline in both groups [24]. However, Cramer et al. demonstrated that oral administration of bexarotene, a retinoid X receptor agonist, reversed various cognitive deficiencies observed in WT mice but without significant effect on APOE KO groups [25]. The complicated interplay between APOE and other AD biomarkers makes it difficult to discern whether APOE modulatory therapy could successfully lessen cognitive decline in AD. Furthermore, treatments that have been investigated in transgenic AD mice have not translated well to human trials. Such difficulty in translating APOE therapies from mice to humans is likely due to inherent differences in APOE between species. Humans express three major APOE isoforms, while only one is naturally observed in wild type mice [11].
Gender and age effect on AD
Female gender and old age are frequently cited in human studies as probable AD risk factors. However, it is difficult to assess whether gender has a significant effect on cognition due to confounding variables such as life expectancy, innate differences in performance on cognitive tasks, and difference in hormone levels [26]. Although many studies have shown that AD may occur more often and more severely in women [27–29], the underlying mechanism by which women are more predisposed than men remains undiscovered [26, 30–33]. Meanwhile, incidence of AD increasing with age is perhaps the most substantiated pattern documented in the literature [34–37]. However, even this trend is still under scrutiny due to a lack of understanding of how the effects of natural aging can be compounded by other non-age-related features to result in increased AD risk for certain populations [37].
Scope of present study
A complex and difficult to interpret relationship exists between age, gender, treatment, and genotype on cognition in AD, making it challenging to develop broadly effective treatments through diet, vitamin supplementation, exercise, or other specific apoE modulatory drugs. Though age has most clearly and consistently been shown to be the biggest risk factor for developing AD [37], the relationships between both age and cognition [34–37] as well as gender and cognition [26, 30–33] are less well known. The effects of various APOE treatments are also similarly disputed [24, 25, 38]. Likewise, categorizing genotypes by the effect they have on cognition has yielded inconsistent results [19–23]. Therefore, there is a need for a deeper understanding of the relationship between various features that contribute to AD pathogenesis across a large, aggregate set of data from multiple published APOE transgenic mouse experimental studies. The objective of the present study was to ascertain aggregate trends in AD APOE mouse literature by performing statistical analysis machine learning feature importance modeling to ascertain the extent that cognition in AD APOE mice is affected by APOE genotype, APOE modulatory treatment, mouse gender, and mouse age.
METHODS
The goal of this work was to perform an aggregate analysis of cognition in APOE transgenic AD mice to determine the importance specific features, namely mouse genotype, type of APOE modulatory treatment, mouse age, and mouse gender, in predicting cognition as measured via Morris water maze (MWM) escape latency. PubMed database (http://www.pubmed.gov) searches identified peer-reviewed publications that were manually reviewed to determine if their data met criteria for inclusion into the present study. All quantifiable experimental data from tables and figures were transcribed into a relational database with resultant transcription accuracy in excess of 98.8%under consistent oversight by meticulous quality control using a published protocol [39].
Inclusion criteria
PubMed was queried using the key search terms “Alzheimer’s Disease”, “APOE”, and “Morris Water Maze” in addition to various synonymous combinations and abbreviations. To be included in the present study, each publication must have included MWM escape latency results at baseline and after 4 or 5 days of training; inclusion of a control and treatment group; wild type genotype, APOE KI genotype (namely APOE2, APOE3, or APOE4 knock-in), or APOE KO genotype; demarcation of mouse gender; demarcation of mouse age at time of MWM testing. A total of 31 peer-reviewed papers met all requirements for inclusion. Figure 1 displays a PRISMA Flow Diagram for the present study.
Fig. 1
PRISMA Flow Diagram for the systematic review of PubMed articles related to AD APOE. The PRISMA flow diagram represents the systematic review of PubMed articles and the compilation and curation of journal article data into a manually constructed relational database. The final set of included studies comprised 31 journal articles and 3,045 mice. Features assessed include mouse genotype (wild type, APOE2 knock-in, APOE3 knock-in, APOE4 knock-in, APOE knock-out); type of external APOE modulatory treatment and its intended impact on the underlying etiology and corresponding cognition (positive treatment, negative treatment, untreated); mouse age (in days); and mouse gender (male, female, or mixed/unknown).
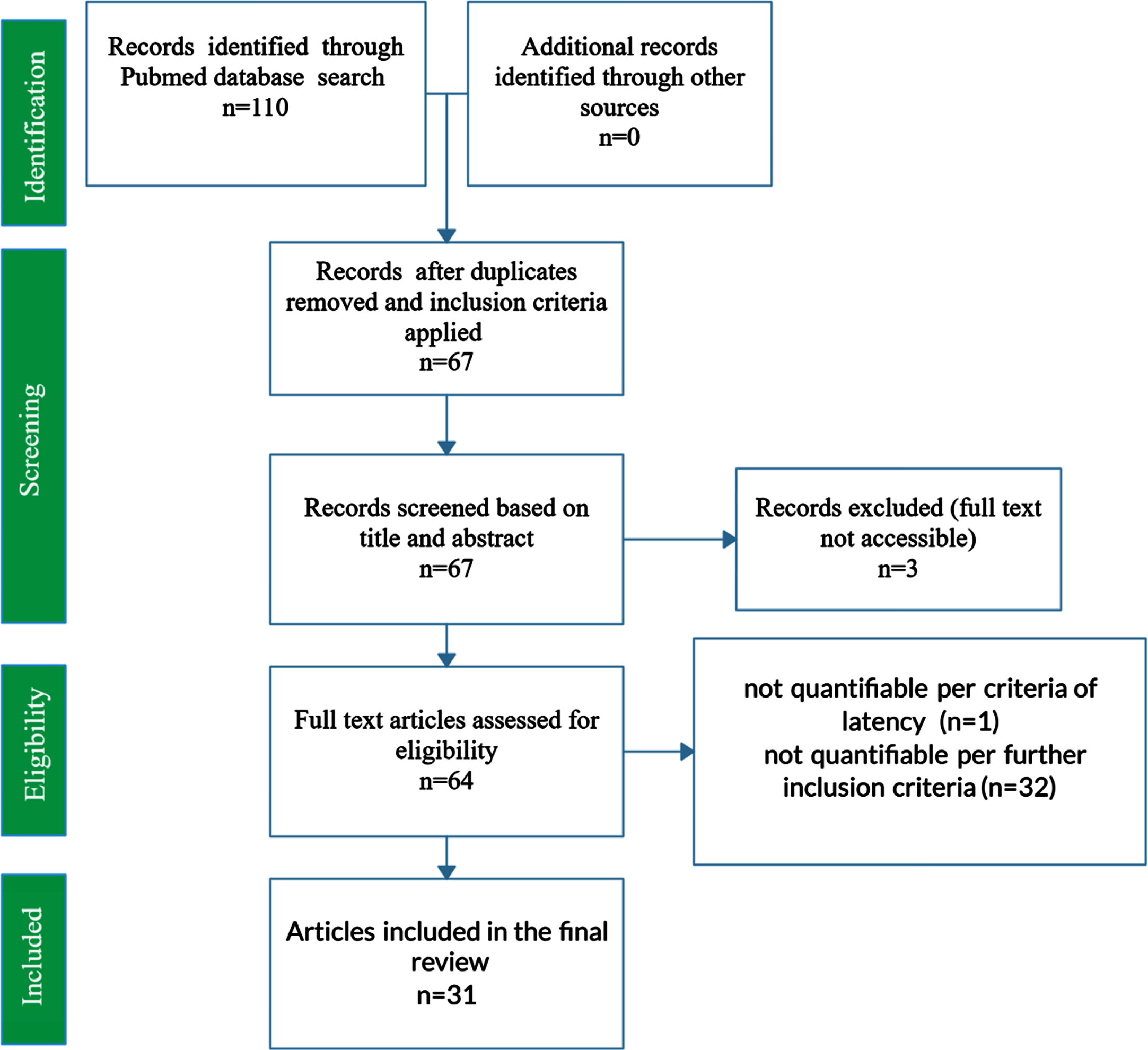
Morris water maze
The MWM is used to test spatial memory as a measure of cognitive functioning and was first developed by Richard Morris in the early 1980s [40]. MWM is a standard metric of cognitive performance in mouse models, and due to its widespread use in pre-clinical AD studies [40, 41], MWM escape latency was chosen as the outcome metric for mouse cognition in the present study. In the beginning of the MWM, a mouse is placed into a pool of cold water (13°C) that contains a visibly discernible platform [42, 43]. On the first day of training, the mouse learns to find the visible platform and, thus, escape the cold-water bath. Subsequently, the mouse is reintroduced to the maze with the platform hidden under the water, instead. As a result, the mouse must rely on visible cues outside of the maze to locate the platform. Training with the hidden platform usually takes place approximately four times daily over the course of four to seven days. The amount of time it takes for the mouse to find the hidden platform is measured as escape latency [41]. To prevent skewed results from over-training in the MWM, only the first 5 days of training trials were included for analysis. Overtraining due to either too many days of training or too may trials per day can cause all of the mice in the experiment, whether wild type or transgenic, to display similar escape latency values that inhibit quantitative assessment of learning differences [44]. Trials on the first day of training are used as a control group between mice to account for individual swimming performance [42, 43]. Supplementary Table 1 includes the specific description of the MWM used in each data source included in the present study.
Data sources
The C57BL/6 mouse model is the most widely utilized genetic background of altered mice for study of human pathology due to its ability to maximally express most mutations [45]. In addition to being relatively easy to breed, the mouse model is highly sensitive to cold temperatures, making it particularly useful for examining cognition through the MWM [46]. The C57BL/6 mouse model subline was originally isolated at the Bussey Institute and most extensively distributed by the Jackson Laboratory; however, as a result of its ubiquity in research, many groups have bred colonies in isolation from one another for many generations, potentially exacerbating the effects of genetic drift, the random change in allele frequency in a population over subsequent generations [47]. The studies included in the database range from years 1997 to 2020, with the majority from 2013 to 2020. Table 1 details collected data segregated into the subpopulations of interest used in the present aggregate analysis. Supplementary Table 1 displays all included studies, their respective publication years, available source information for mouse backcrossing, and detailed treatment descriptions.
Table 1
Mean normalized escape latency and standard deviation is the age-normalized Morris water maze escape latency in seconds. Mice n is the sample size of the group, whereas study n is the number of studies utilized for the group. Bracketed numbers in the sources column correspond to full data source references. There were 1,430 female mice, 1,181 male mice, and 258 mixed/unknown gender mice
Group | Mean Norm. Latency (s) | Std. Dev. Norm. Latency (s) | Mice (n) | Data Sources (n) | Data Source References |
KI3_t– | 37.6 | – | 8 | 1 | [48] |
KI4_t– | 30.9 | 11.0 | 66 | 3 | [48–50] |
KO_t– | 47.7 | 6.2 | 62 | 4 | [49, 51–53] |
WT_t– | 32.8 | 9.5 | 203 | 5 | [49–53] |
all_t– | 35.3 | 11.0 | 339 | 6 | [48–53] |
KI2_t0 | 38.2 | 5.4 | 354 | 3 | [34, 54, 55] |
KI3_t0 | 30.6 | 9.0 | 677 | 16 | [34, 48, 54–67] |
KI4_t0 | 48.6 | 15.3 | 738 | 19 | [34, 49, 54–57, 59–71] |
KO_t0 | 40.9 | 15.3 | 337 | 10 | [34, 49, 53, 58, 63, 72–76] |
WT_t0 | 35.8 | 17.2 | 326 | 11 | [49, 50, 53, 63, 64, 68–70, 72, 74, 75] |
all_t0 | 39.3 | 14.9 | 2432 | 28 | [34, 48–50, 53–76] |
KI3_t+ | 29.2 | 5.2 | 61 | 5 | [56, 61, 64–66] |
KI4_t+ | 33.5 | 8.9 | 115 | 9 | [56, 61, 64–66, 68, 70, 71, 77] |
KO_t+ | 28.3 | 6.0 | 72 | 3 | [58, 74, 75] |
WT_t+ | 25.8 | 1.6 | 26 | 2 | [68, 74] |
all_t+ | 30.4 | 7.9 | 274 | 12 | [56, 58, 61, 64–66, 68, 70, 71, 74, 75, 77] |
Assessed features
Twelve possible categorical or continuous features of each study were curated in the present work as follows: transgenic APOE knockout (KO), transgenic APOE2 knock-in (KI2), transgenic APOE3 knock-in (KI3), transgenic APOE4 knock-in (KI4), wild type (WT), treatments meant to improve cognition or improve the AD etiology (t+), treatments meant to decrease cognition or worsen the AD etiology (t–), untreated mice (t0), female gender (g_f), male gender (g_m), mixed or unstated gender (g_mi), mouse age in days (age), and MWM escape latency in seconds (latency). Notably, mouse age was normalized and resampled such that treated and untreated groups had the same mean age within 0.05%. Unless stated otherwise, reported MWM escape latency is the normalized MWM escape latency in seconds.
Varied treatment methods were employed to directly or indirectly change the underlying neurodegenerative etiology and corresponding mouse cognition. To appropriately aggregate treatment effects, treatments were categorized based on their intended effect on cognition (positive or negative) using descriptions of the Methods in the original published works. Examples of positive treatments include bexarotene administration [56], medical ganglionic eminence transplantation [64], and acetyl-L-carnitine and dexlipotam dietary supplementation [70]. Examples of negative treatments include repetitive traumatic brain injury [50], intraperitoneal injection of EtOH and AcH [53], and a high-fat diet [49]. Detailed treatment descriptions and treatment category labels for each included data source are listed in Supplementary Table 1.
Statistical analysis
Age-normalized mean MWM escape latency and standard deviation was used to conduct all statistical analysis in the present study as noted under Assessed Features section above.
The reported aggregate mean and standard deviation for each group in Table 1 was calculated using the frequency distribution, which weights the average and standard deviation for each group based on the sample size associated with each observation within the group. As such, the contributions of individual original data sources to the reported aggregate mean and standard deviation are a function of the original source’s reported mouse sample size for each observed group. Equal weighting of all studies, which would disregard mouse sample size for each reported study when calculating the aggregate group mean and standard deviation, was not possible due to lack of statistical power.
Data distributions were assessed for normality using Shapiro-Wilk test and found to exhibit a sufficiently Gaussian distribution for assessment via ANOVA and t-tests. An ANOVA was performed to assess which groups should be further examined using post-hoc testing to identify pairwise significant differences. To explore pairwise differences in cognitive performance as measured by mean normalized MWM escape latency, two-tailed t-tests were performed at an overall alpha of 0.05. Bonferroni correction was utilized to correct the p-value threshold for significance for multiple comparisons, resulting in a p-value threshold for significance of p < 0.002. All statistical analysis was performed in Microsoft Excel.
Random forest modeling
The MWM escape latency data in the present study was used to produce a supervised random forest machine learning model in Python version 3.8.3 (with Pandas version 1.0.4, NumPy version 1.18.0, and Scikit-learn version 0.23.2 python packages) to predict the importance of twelve binary features: transgenic KO, KI2, KI3, KI4, WT, treatments meant to improve cognition or improve the AD etiology (t+), treatments meant to decrease cognition or worsen the AD etiology (t–), untreated mice (t0), female gender (g_f), male gender (g_m), mixed or unstated gender (g_mi), and mouse age (age). The model was set to classify either superior or inferior normalized MWM escape latency performance using a threshold obtained via data standardization. Curated data was standardized and converted into binomial features (feature present = 1, feature not present = 0). The continuous features of normalized escape latency and age were also standardized using a z-score. For example, normalized escape latency is standardized using the following procedure: [(normalized escape latency for each mouse –mean normalized escape latency for all mice)/ standard deviation of normalized mean escape latency for all mice]. Standardized age was calculated using the same z-score method. A standardized normalized escape latency less than or equal to the mean was binomially classified as “superior” performance, whereas inferior performance was binomially classified as “inferior” performance. Likewise, standardized mouse age less than the mean was binomially classified as “young”, whereas standardized mouse age greater than the mean was classified as “old”.
Random forest models have been widely used in AD literature to assess the high dimensional nature of diagnosis [78]. A random forest is composed of a set of decision trees that each consist of split nodes and leaf nodes. The decision tree is fed a predictor, or target variable, as well as corresponding features used to predict the target variable [79]. MWM escape latency was used as the labelled predictor in this study. Each sample passed to the model is assessed by the split node before being passed to its left or right child, depending on the sample’s features. The decision trees are fed random samples (with replacement) from a subset of the training data or testing data. Beginning with the top split node, or root node, each subsequent node in the tree is trained to continuously split until it achieves stop criteria such as maximal tree length or minimal number of samples. The mean and variance of the target group in the data subset of each decision tree is stored in leaf nodes for future forecasting [79].
While in the training stage, the random forest model can see the labels on the target variable so that it can recognize when a mistake is made by incorrectly predicting the target variable based on the corresponding features for a particular observation. The process of training is how the model learns. During the independent testing stage, the model is exposed to data that was not seen during the training phase, so it does not know the true value of the target variable [79].
RESULTS
This systematic review and meta-analysis with adjunctive machine learning compares cognitive function as empirically measured through escape latency from the MWM test in various WT, APOE KI, and APOE KO mice. A total of 3,045 mice from 31 peer-reviewed scientific papers [34, 48–77] were included in the analysis. The included peer-reviewed studies consisted of MWM escape latency results at baseline and after 4 or 5 days of training; inclusion of a control and treatment group; WT or APOE transgenic mouse type; mouse age in days; and mouse gender (female, male, mixed/unknown). There were 1,430 female mice, 1,181 male mice, and 258 mixed/unknown mice.
Table 1 displays the study citation references for each aggregated data pool utilized for standard statistical analysis to compare the normalized escape latency between groups. Groups are categorized by transgenic mouse type and treatment with an underscore “_” in between. Mouse genotypes include: KO, KI2, KI3, KI4, WT, and all mice in the study (all). Treatment types include those meant to directly or indirectly improve the AD etiology and thus improve cognition (t+), treatments meant to perturb or assess the worsening of AD etiology and thus diminish cognition (t–), or untreated mice (t0) for which no additional treatments or procedures were performed. For standard statistical analysis, the age was normalized such that there was less than a 0.05%difference between groups (see Methods). To maintain statistical power, gender was not considered in the statistical analysis comparing normalized escape latency between groups, but gender was considered in the supervised machine learning model. Unless stated otherwise, reported MWM escape latency is the normalized MWM escape latency in seconds.
Comparison of cognition in untreated mice
Standard statistical analysis with two-tailed t-tests and overall alpha of 0.05 was utilized to compare the impact of mouse genotype on cognition as measured via the normalized MWM escape latency. To prevent skew of data results from over-training in the MWM, only the first 5 days of training trials were included for analysis. The threshold for significance was adjusted using a Bonferonni correction for multiple comparisons (with p < 0.002 required for significance), which greatly reduced the likelihood of a false positive.
First, mean normalized MWM escape latencies were examined for all included mouse genotypes without any additional modulatory treatments. Figure 2 illustrates the mean escape latency in seconds for each untreated group (t0) with the error bars denoting the corresponding positive standard deviation. Untreated transgenic APOE3 KI mice (KI3_t0) was the only group to have a significantly lower normalized mean escape latency compared to WT. Untreated KI2 mean normalized MWM escape latency was not significantly different than WT. However, KI4 and KO had significantly longer MWM escape latencies compared to WT. Moreover, untreated KI4 mice had the highest normalized mean escape latency, meaning they had significantly worse cognitive performance compared to the other untreated groups. The colored * above each bar in Fig. 2 represents a significant pairwise comparison between the group corresponding to the labeled bar and the group corresponding to the color of the asterisk. For example, untreated transgenic APOE3 KI (KI3_t0) had a significantly different normalized mean escape latency than every other untreated group (KI2, KI4, KO, WT, and all).
Fig. 2
Comparison of mean normalized Morris Water Maze (MWM) mean escape latency (in seconds) between untreated (t0) wild type and transgenic APOE genotypes. MWM escape latency is normalized for age but not gender. Mean normalized escape latency comprises MWM escape latency measurements for baseline through day 4 or 5 of training. The error bar corresponds to the positive standard deviation for the corresponding group. Groups are as follows: transgenic APOE knockout (KO), transgenic APOE2 knock-in (KI2), transgenic APOE3 knock-in (KI3), transgenic APOE4 knock-in (KI4), wild type (WT), all mice in the study (all). The color-coded asterisk (*) indicates Bonferonni-corrected pairwise statistical significance between groups (p < 0.002).
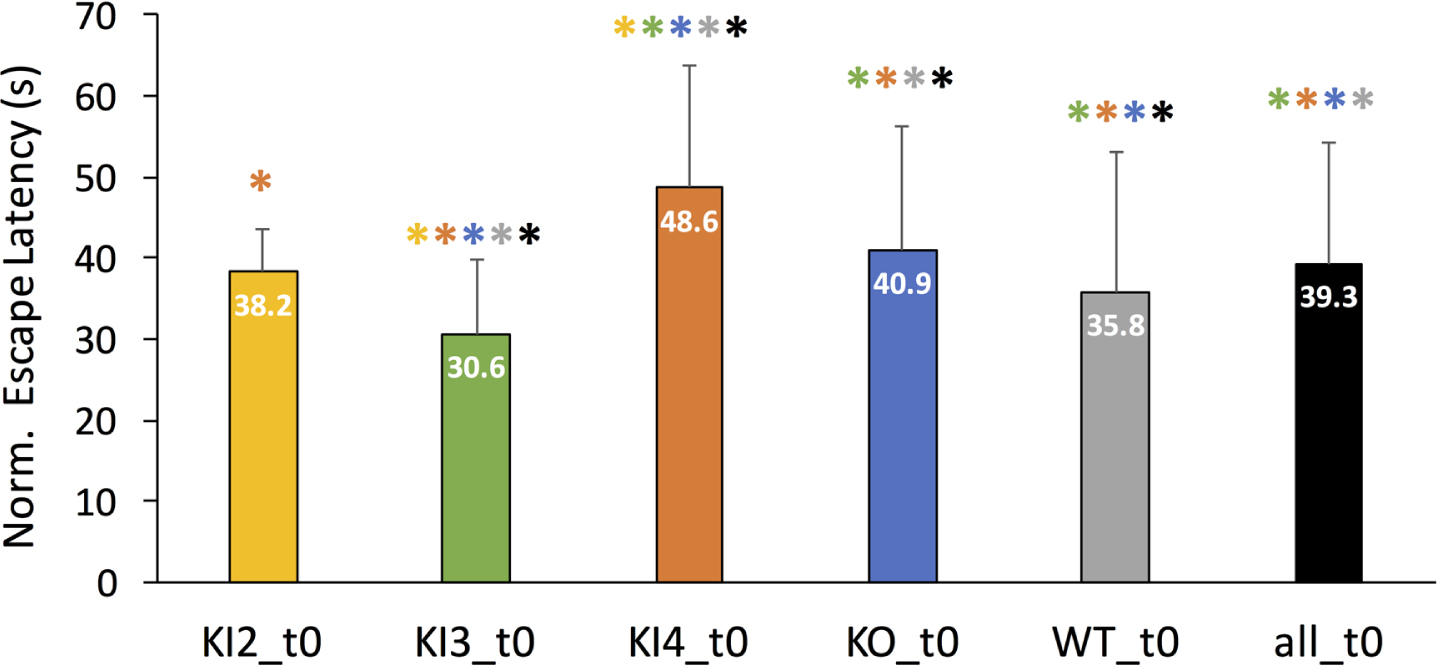
Treatment effect on cognition in APOE mice
The normalized mean MWM escape latency was analyzed for mice that were treated with APOE modulatory treatments. Such treatments could include either direct or indirect modulation of underling etiology beyond the original APOE genotype modifications listed in Table 1. Treatments were separated based on their intended effect. Positive treatments (t+) were meant to lessen the AD etiology and thus improve cognition, whereas negative treatments (t–) were meant to perturb or worsen the AD etiology, and thus, further impair cognition. A full description of treatments for each study is given in Supplementary Table 1. The untreated group (t0) for each mouse genotype is shown in each panel of Fig. 3 for visual comparison. Again, to maintain statistical power, the statistical analysis was not segregated by mouse gender. However, all groups were normalized for age to better isolate the impact of the treatment and mouse genotype.
Fig. 3
Comparison of modulatory treatments on normalized Morris Water Maze (MWM) escape latency across wild type (WT) or APOE mouse genotypes. Data is normalized for age but not gender. Mean normalized escape latency comprises MWM escape latency measurements for baseline through day 4 or 5 of training. The error bar corresponds to the positive standard deviation for the corresponding group. Positive treatments (t+) were meant to lessen the AD etiology and/or improve cognition; negative treatments (t–) were meant to worsen the AD etiology and/or worsen cognition; untreated mice (t0) of the same genotype are shown for comparison. A full description of the treatments for each study is given in Supplementary Table 1. The asterisk (*) indicates Bonferonni-corrected pairwise statistical significance between groups (p < 0.002). a.) All mice genotypes; b.) Wild type mice; c.) Transgenic APOE2 knock-in (KI2) mice; d.) Transgenic APOE3 knock-in (KI3) mice; e.) Transgenic APOE4 knock-in (KI4) mice; f.) Transgenic APOE knockout (KO) mice.
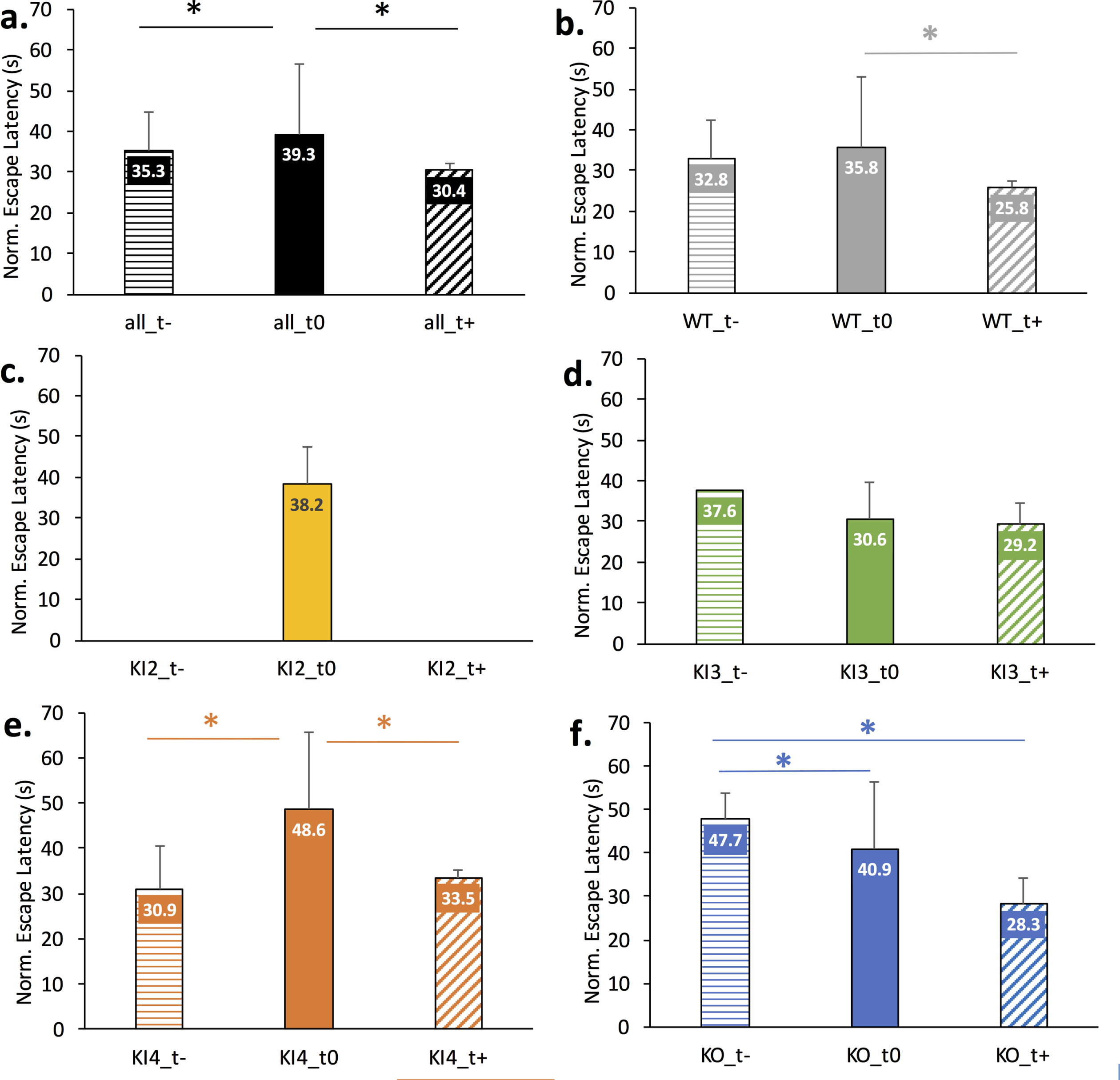
Figure 3 illustrates the impact of positive treatment (t+), negative treatment (t–), or no treatment (t0) for each mouse genotype. A couple of groups had extremely limited data. Namely, only untreated data was available for KI2 and only one study was available for negatively treated transgenic APOE3 KI (KI3_t-). For the remaining groups, the mean normalized escape latency is shown with the error bar visually denoting standard deviation. Pairwise significance is denoted by the * and corresponds to significance less than the Bonferonni-corrected threshold of p < 0.002. Figure 3a shows a significant pairwise difference between the normalized escape latencies of all untreated mice (all_t0) and all negatively treated mice (all_t–) as well as between all untreated mice (all_t0) and all positively treated mice (all_t+). Figure 3b illustrates a significant difference in normalized escape latency between untreated WT (WT_t0) and positively treated WT (WT_t+) mice. Figure 3c illustrates the normalized escape latency of untreated transgenic APOE2 KI (KI2_t0) given treatment data was absent for this genotype. Figure 3d illustrates no significant difference in normalized escape latency between treated and untreated transgenic APOE3 KI mice. Figure 3e illustrates significant pairwise differences in normalized escape latency between negatively treated transgenic APOE4 KI (KI4_t–) and untreated (KI4_t0) as well as between untreated and positively treated APOE4 KI (KI4_t+). Figure 3f illustrates a significant pairwise difference in normalized escape latency between negatively treated transgenic APOE KO (KO_t–) and untreated (KO_t0) and between KO_t–and positively treated (KO_t+).
Evaluation of feature importance with random forest modeling
A supervised machine learning technique, random forest modeling, was performed to assess the impact of twelve specific binary features on the prediction of normalized MWM escape latency, including gender (g_m = male; g_f = female, g_mi = mixed/unknown), which was not analyzed in the pairwise statistical analysis presented above. Data from the curated relational database was randomly partitioned into a training set (80%of data) and an independent testing set (20%of data). A supervised random forest model was performed using a binomial standardized normalized MWM escape latency as the predictor where latency was classified as either “superior” or “inferior” (see Methods). Model accuracy on independent test data was 82%. Random forest modeling yielded the following feature importance for the prediction of superior cognition as measured via standardized and normalized MWM escape latency: [KI3, age, g_m, KI4, t0, t+, KO, WT, g_mi, t–, g_f, KI2] = [0.270, 0.094, 0.092, 0.088, 0.077, 0.074, 0.069, 0.061, 0.058, 0.054, 0.038, 0.023] (Fig. 4). These results indicate KI3 is most important for predicting superior cognition. Mouse age, male gender, and KI4 comprise the next group of closely ranked features that was second-most important for predicting superior cognition. Untreated (t0), positively treated (t+), and KO comprise the third tier of features for predicting superior cognition. WT, mixed gender (g_mi), and negative treatment (t–) comprise the fourth tier of features for predicting superior cognition. Female gender (g_f) and KI2 comprise the fifth and final tier of features for predicting superior cognition; as such, this final tier is least important for predicting superior cognition as measured by standardized and normalized MWM escape latency.
Fig. 4
Importance of binomial features on normalized and standardized Morris Water Maze (MWM) escape latency as predicted by supervised random forest modeling. Data was randomly partitioned into a training set (80%) and an independent test set (20%). Overall model accuracy during independent testing was 82%. Twelve binary features were utilized to predict escape latency; thus, each feature was marked for each mouse as being either present or absent. In descending order, the importance of the following binary features was predicted for classifying “superior” cognition: [KI3, age, g_m, KI4, t0, t+, KO, WT, g_mi, t–, g_f, KI2] = [0.270, 0.094, 0.092, 0.088, 0.077, 0.074, 0.069, 0.061, 0.058, 0.054, 0.038, 0.023]. Superior cognition was defined as a standardized and normalized MWM escape latency less than or equal to the overall sample mean (see Methods). Feature legend is as follows: transgenic APOE3 knock-in (KI3), male gender (g_m), transgenic APOE4 knock-in (KI4), untreated (t0), positive treatment meant to enhance cognition (t+), transgenic APOE knockout (KO), wild type (WT), mixed or unknown gender (g_mi), negative treatment (t–), female gender (g_f), and transgenic APOE2 knock-in (KI2). The overall sample size for the supervised random forest model was n = 3,045 mice. There were 1,430 female mice, 1,181 male mice, and 258 mixed/unknown gender mice. The breakdown of mouse genotype sample sizes is given in Table 1.
![Importance of binomial features on normalized and standardized Morris Water Maze (MWM) escape latency as predicted by supervised random forest modeling. Data was randomly partitioned into a training set (80%) and an independent test set (20%). Overall model accuracy during independent testing was 82%. Twelve binary features were utilized to predict escape latency; thus, each feature was marked for each mouse as being either present or absent. In descending order, the importance of the following binary features was predicted for classifying “superior” cognition: [KI3, age, g_m, KI4, t0, t+, KO, WT, g_mi, t–, g_f, KI2] = [0.270, 0.094, 0.092, 0.088, 0.077, 0.074, 0.069, 0.061, 0.058, 0.054, 0.038, 0.023]. Superior cognition was defined as a standardized and normalized MWM escape latency less than or equal to the overall sample mean (see Methods). Feature legend is as follows: transgenic APOE3 knock-in (KI3), male gender (g_m), transgenic APOE4 knock-in (KI4), untreated (t0), positive treatment meant to enhance cognition (t+), transgenic APOE knockout (KO), wild type (WT), mixed or unknown gender (g_mi), negative treatment (t–), female gender (g_f), and transgenic APOE2 knock-in (KI2). The overall sample size for the supervised random forest model was n = 3,045 mice. There were 1,430 female mice, 1,181 male mice, and 258 mixed/unknown gender mice. The breakdown of mouse genotype sample sizes is given in Table 1.](https://content.iospress.com:443/media/jad/2021/83-1/jad-83-1-jad210492/jad-83-jad210492-g004.jpg)
DISCUSSION
The statistical meta-analysis and machine learning prediction of MWM escape latencies in transgenic mice performed in the current study provided aggregate insight into the APOE etiology and therapeutic modulation of APOE to improve cognition in AD transgenic mice. Further perspective on the impact of APOE or AD modulatory treatment, mouse age, mouse gender, and mouse genotype on cognitive performance as measured by normalized MWM escape latency. The results are explored in the context of prior and common literature examining the role of APOE in experimental animal models and in clinical AD in humans.
Impact of APOE genotype on cognitive performance
The APOE gene is primarily involved in the synthesis of apolipoprotein E, and its expression has been documented in both the central and peripheral nervous system. However, the role of apoE in the body is potentially broader than just lipid metabolism, as its namesake implies. This may include maintaining healthy brain functioning, particularly as apoE acts as a carrier for various cholesterols involved in neuronal activity and repair. As such, normal apoE is important for clearing of plaques and other wastes from dying cells [80], as well as in the initiation of regenerating neurons [81]. However, pathological apoE, due to alteration of copy number or the presence of an APOE mutation, contributes to degeneration resulting in decreased cognition [13, 82].
Among the three human isoforms of APOE (E2, E3, and E4), APOE4 is commonly known to be the greatest genetic risk factor for onset of human AD, while APOE2 has been demonstrated to decrease the risk of onset in humans [6]. The APOE4 allele is carried by 3–41%of the global human population [83]. Moreover, there is increasing evidence that the APOE4 isoform increases risk of AD in humans via pathways involved in loss of function or gain of toxic function. These include well-studied biomarkers of AD, such as tau pathology, tau-mediated neurodegeneration, and an expanding list of Aβ-dependent and Aβ-independent pathways that are conditionally affected by different apoE isoforms [13, 84]. In the case of human Aβ pathways, APOE4 expression has been shown to catalyze the folding of Aβ peptides into higher order sheets and other conformations, which contributes to AD pathogenesis by facilitating plaque accumulation in the brain [85].
In the present study’s aggregate data analysis, statistical comparison of mean normalized MWM escape latency data in untreated transgenic APOE AD mice indicated that untreated knock-in APOE3 (KI3_t0, Fig. 2) mice out-performed untreated wild type mice (WT_t0, Fig. 2). Like results seen in human AD risk studies on the basis of APOE isoform, untreated APOE2 knock-in (KI2_t0) transgenic AD mice had the closest normalized escape latency to untreated wild type mice (Fig. 2). However, untreated APOE4 knock-in (KI4_t0, Fig. 2) and APOE knock-out (KO_t0,) transgenic AD mice performed significantly worse than wild type (Fig. 2). These results illustrate that either loss of apoE function or gain of toxic apoE function can exacerbate AD pathology and corresponding decreased cognition in transgenic mice, which is consistent with human AD observational studies.
The strong association of APOE4 with MWM escape latency in both the pairwise statistical analysis (Figs. 2 and 3) and the machine learning feature importance (Fig. 4) underscored its known prominence in the AD etiology. The fact that APOE2 knock-in appeared less protective in the present study compared to observations noted in human studies could be explained by experimental differences in APOE in transgenic mice. Mice have fundamental differences in APOE compared to humans; even APOE knock-in mice that utilize human APOE isoforms have drastic quantitative differences in apoE content compared to WT mice [86]. While the presence of altered APOE genotype or copy number often garners the most attention in human studies, lack of APOE can also cause neurodegenerative etiology. As noted in the present study, APOE knock-out mice performed worse than WT (Figs. 2 and 3), a finding which is also supported by prior work [58].
The present study examined the role of APOE in preclinical data through transgenic mouse models. As such, it is important to note a few marked differences between APOE expression and functioning in mice when compared to humans. For instance, mice are shown to naturally express only one isoform of APOE [11], whereas humans exhibit three. Furthermore, mice and humans differ in their relative lipoprotein levels, which may alter respective clearance mechanisms. Moreover, mice utilize HDL as a cholesterol shuttler as opposed to the primary use of LDL by humans. In particular, APOE KO mice have much higher plasma lipid levels, the effects of which could potentially contribute to synaptic dysfunction and resulting neurodegeneration via independent mechanisms. Overall, the immediate phenotype of deficient APOE levels may differ considerably in mice and humans [87].
Impact of age on cognition in the Morris water maze
Age has been widely cited as the single greatest risk factor for AD development in humans [37]. The process of aging is highly intricate and results from the aggregate contribution of various body systems being influenced at the cellular level. Many physiological pathways have been identified in the aging process, and those that most directly affect brain functioning and have been most strongly associated with AD risk include mitochondrial dysfunction, inflammatory reactions in the innate immune system, insufficient glucose metabolism, disruption of lipid homeostasis, worsening Aβ processing, and decreased regenerative ability [35, 36]. This age-linked neurodegeneration in transgenic AD mice has been demonstrated through downregulation of an NMDAR signaling pathway resulting in a loss of function [34].
Notably, the present aggregate study took extensive care to control for confounds introduced by differences in mouse age or differences in neurodegeneration at the time of MWM escape latency testing. In the present study, the mean escape latency of trials from baseline through day 4 or 5 MWM training was utilized to prevent skewed escape latencies, and mice ages are normalized and resampled to ensure a minimal standard deviation (see Methods). These procedures reduced experimental confounds over the timeline of MWM testing [72, 88, 89] that would have otherwise clouded study results. Because of the normalization and resampling, assessment of the impact of mouse age on cognition with traditional statistics was not appropriate. However, the random forest machine learning model provided a means to assess the impact of age alongside other features, including APOE mouse genotypes, treatment types, and gender. The random forest feature importance (Fig. 4) illustrated that mouse age (in days) was the second-most important individual feature for predicting cognitive performance as measured by MWM escape latency. Specifically, mice with ages younger than the sample mean are more likely to have superior performance in the MWM.
APOE-targeted treatments have a significant impact on cognition independent of age
Multiple potential treatments have been studied to alter levels of APOE expression to improve cognitive performance [38]. Most studies focus on “positive” treatments meant to indirectly or directly reduce the APOE-related pathology, and correspondingly, improve cognition. However, some studies apply “negative” treatment in an attempt to perturb the neurodegenerative process to allow further etiological assessment; such negative treatments worsen the cognition of the mice compared to their baseline or untreated genotype. For this reason, the present study divided modulatory treatments as positive (t+), negative (t–), or untreated (t0), to better elucidate the external modulation of APOE in WT or transgenic mice. A detailed description of modulatory treatments for each study is given in Supplementary Table 1.
It is the positive treatments that are most important for translational development of future human AD treatments. Statistical analysis of modulatory treatments in the present study illustrated that positive treatments were able to significantly to improve cognition in wild type (WT_t+, Fig. 3b), APOE4 knock-in (KI4_t+, Fig. 3e), and APOE knock-out (KO_t+, Fig. 3f). Positively treated APOE3 knock-in (KI3_t+, Fig. 3d) has a small, albeit insignificant improvement in MWM escape latency. No prior positively treated APOE2 mouse model study data met criteria for inclusion in the present study; therefore, the impact of positive treatment on APOE2 knock-in (KI2, Fig. 3c) was unable to be assessed.
Many AD treatment routes attempt to improve cognitive performance through the use of drug agonists for defined receptors in the brain. A commonly used agonist is bexarotene, which has been suggested to provide neuroprotection and synaptic plasticity. Another important connection is the inclusion of ABCA1-mediated lipidation in agonist treatments. ABCA1 typically causes better cognitive performance in both KO and WT. Looking into the subcategory of diet-related treatments, lower caloric intake was seen to have a positive impact on cognitive performance. However, a key issue with many treatments intended to positively modulate APOE is that they may be effective in WT mice but not in transgenic APOE KI or KO mice.
Negative treatments are less meaningful for human clinical treatment development, but still provide interesting perspective on the underlying AD etiology in transgenic AD mice experiments. In the present study, only negative treatments for APOE4 knock-in (KI4_t–, Fig. 3e) and APOE knock-out (KO_t–, Fig. 3f) had significantly different normalized MWM escape latencies compared to untreated mice of the same genotype. However, the negatively treated APOE4 knock-in (KI4_t–, Fig. 3e) ironically had improved normalized MWM escape latency compared to untreated APOE4 knock-in (KI4_t0, Fig. 3e). The aforementioned finding with APOE4 illustrates its heterogeneity and overall complexity in the AD etiology.
Challenges in translation of pre-clinical APOE treatments to human Alzheimer’s disease
Many treatments that show promise in transgenic mice do not translate to human trials. This is likely due to the vast differences of APOE in mice, who naturally present one isoform, versus humans, which present three isoforms [90]. Another challenge is that APOE pathology is not isolated in human AD, as apoE interacts with multiple factors that contribute to neurodegeneration and corresponding cognitive decline [91]. Nonetheless, parenteral administration of citicoline, an intermediate in the anabolism of structural membrane phospholipids, has been one of the more promising treatments in humans with APOE4 genetic risk who have early signs of cognitive decline [92]. Moreover, although the study was limited due to a relatively small sample size and uncontrolled design, supplementation of a drink containing antioxidants, omega-3 fatty acids, and resveratrol appeared to elicit positive immune and cognitive effects in APOE E3/E3 patients [93].
In a double-blind, placebo-controlled human clinical trial of bexarotene, an agonist of the retinoid X receptor, the overall results were negative. Bexarotene was shown to increase serum Aβ in APOE4 noncarriers; as such, it increased associated risk factor for contracting cardiovascular disease without conferring any positive effects on cognition [94]. Similarly, in phase III trials of bapineuzumab, a humanized anti-Aβ antibody, results were negative in that no significant difference between the treatment and placebo groups was observed [95]. In summary, ongoing research is still required to successfully translate APOE modulatory treatments for human AD.
Impact of gender on cognition in AD
In the present aggregate data analysis, traditional pairwise statistical analysis could not separately address the impact of gender on each transgenic AD mouse model’s mean normalized cognitive performance in the MWM due to a lack of statistical power. However, the supervised random forest machine learning model was able to examine the importance of gender in context with the other features that predict normalized MWM escape latency, including mouse genotype, mouse age, and APOE modulatory treatments. The random forest model calculated the feature importance for 12 binary features used to predict “superior” MWM performance, which was defined as a normalized MWM escape latency faster than the sample mean. The plot of random forest feature importance (Fig. 4) illustrated that the male gender (g_m) is the third-most important individual feature for predicting superior cognitive performance in the MWM. The male gender fell into the second of five tiers that grouped features of similar importance in predicting normalized MWM escape latency. Mixed gender (g_mi) and female gender (g_f) were not prominent features for predicting superior MWM escape latency. In fact, mixed gender ranked eighth (of twelve) as an individual feature and fell into the fourth (of five) tiers of grouped features to predict superior MWM escape latency. Likewise, female gender ranked eleventh (of twelve) as an individual feature and fell in the fifth or least important tier for predicting superior cognitive performance via MWM escape latency. Therefore, female gender was not a common feature present among mice that performed superiorly in the MWM.
The findings of gender in the present aggregate study do align with what has been seen with human AD patients in the clinic, as females tend to be at greater risk for AD. Regarding the role of gender, Mielke et al. propose that several possibilities could explain the discrepancy in AD incidence between men and women, including risk factors with equal frequency in each gender but are stronger in one group, risk factors with the same effect but different frequency in each gender, risk factors that vary in effect and frequency in each gender, and risk factors limited to one gender [26]. An example of risk factor with the same effect but different frequency is smoking, of which rates are higher in men, and an example of a risk factor that varies in effect and frequency is that women appear to be more sensitive to head trauma despite the fact that men suffer from such injuries at higher rates [26].
Additionally, several studies have indicated that women have a higher risk for AD than men if they are APOE4 carriers, but the reasoning behind this discrepancy is still disputed [27]. Moreover, prior studies have suggested that decreasing levels of estrogen as women age may lead to higher rates of cognitive decline due to the effects of menopause on mitochondrial pathways associated with AD risk [28]. Interestingly, previous work found that estrogen appears to upregulate the APOE gene [29], yet other studies did not arrive at the same conclusion, mainly due to the complications associated with isolating the effects of estrogen in multiple brain pathways [27].
Females have been documented as more susceptible to development of AD across epidemiological studies, as well, which have similarly attributed the phenomenon to declines in estrogen levels [26, 30–33]. Estrogen has a demonstrated involvement in Aβ pathways associated with neurodegeneration. Mitochondria from young females confers protection against Aβ toxicity that is eventually lost as females reach old age [31]. Additionally, multiple studies have shown that female transgenic AD mice express higher plaque load in comparison to male mice [30].
In an effort to improve outcomes in women, human estrogen replacement therapy trials have attempted to remediate the hormone-linked components of AD pathogenesis. However, there are mixed results in the literature. The most notable negative outcomes are increased risk of cancer and cardiovascular disease upon estradiol administration [31]. Unfortunately, there remains a deficiency of findings addressing the efficacy of pharmacotherapies aimed at preventing and treating AD in at-risk women [32].
ACKNOWLEDGMENTS
This research was funded by the National Science Foundation CAREER award 1944247 to C.M., Alzheimer’s Association research grant AARG-2018-59104 to C.M., Emory Alzheimer’s Disease Research Center pilot (P50 AG025688) and research education center grant award (P30 AG066511) to C.M., and Georgia Institute of Technology President’s Undergraduate Research Awards to Y.W., B.N., J.H.K.
Authors’ disclosures available online (https://www.j-alz.com/manuscript-disclosures/21-0492r1).
SUPPLEMENTARY MATERIAL
[1] The supplementary material is available in the electronic version of this article: https://dx.doi.org/10.3233/JAD-210492.
REFERENCES
[1] | Ballard C , Gauthier S , Corbett A , Brayne C , Aarsland D , Jones E ((2011) ) Alzheimer’s disease. Lancet 377: , 1019–1031. |
[2] | Leonenko G , Sims R , Shoai M , Frizzati A , Bossu P , Spalletta G , Fox NC , Williams J , consortium G , Hardy J , Escott-Price V ((2019) ) Polygenic risk and hazard scores for Alzheimer’s disease prediction. Ann Clin Transl Neurol 6: , 456–465. |
[3] | Saunders AM , Strittmatter WJ , Schmechel D , George-Hyslop PH , Pericak-Vance MA , Joo SH , Rosi BL , Gusella JF , Crapper-MacLachlan DR , Alberts MJ , et al. ((1993) ) Association of apolipoprotein E allele epsilon 4 with late-onset familial and sporadic Alzheimer’s disease. Neurology 43: , 1467–1472. |
[4] | Roses AD ((1996) ) Apolipoprotein E alleles as risk factors in Alzheimer’s disease. Annu Rev Med 47: , 387–400. |
[5] | Roses AD ((2006) ) On the discovery of the genetic association of Apolipoprotein E genotypes and common late-onset Alzheimer disease. J Alzheimers Dis 9: , 361–366. |
[6] | Li Z , Shue F , Zhao N , Shinohara M , Bu G ((2020) ) APOE2: Protective mechanism and therapeutic implications for Alzheimer’s disease. Mol Neurodegener 15: , 63. |
[7] | Huang YA , Zhou B , Nabet AM , Wernig M , Sudhof TC ((2019) ) Differential signaling mediated by ApoE2, ApoE3, and ApoE4 in human neurons parallels Alzheimer’s disease risk. J Neurosci 39: , 7408–7427. |
[8] | Lin YT , Seo J , Gao F , Feldman HM , Wen HL , Penney J , Cam HP , Gjoneska E , Raja WK , Cheng J , Rueda R , Kritskiy O , Abdurrob F , Peng Z , Milo B , Yu CJ , Elmsaouri S , Dey D , Ko T , Yankner BA , Tsai LH ((2018) ) APOE4 causes widespread molecular and cellular alterations associated with Alzheimer’s disease phenotypes in human iPSC-derived brain cell types. Neuron 98: , 1141–1154e1147. |
[9] | Theendakara V , Peters-Libeu CA , Bredesen DE , Rao RV ((2018) ) Transcriptional effects of ApoE4: Relevance to Alzheimer’s disease. Mol Neurobiol 55: , 5243–5254. |
[10] | Safieh M , Korczyn AD , Michaelson DM ((2019) ) ApoE4: An emerging therapeutic target for Alzheimer’s disease. BMC Med 17: , 64. |
[11] | Balu D , Karstens AJ , Loukenas E , Maldonado Weng J , York JM , Valencia-Olvera AC , LaDu MJ ((2019) ) The role of APOE in transgenic mouse models of AD. Neurosci Lett 707: , 134285. |
[12] | Williams T , Borchelt DR , Chakrabarty P ((2020) ) Therapeutic approaches targeting Apolipoprotein E function in Alzheimer’s disease. Mol Neurodegener 15: , 8. |
[13] | Foley AM , Ammar ZM , Lee RH , Mitchell CS ((2015) ) Systematic review of the relationship between amyloid-beta levels and measures of transgenic mouse cognitive deficit in Alzheimer’s disease. J Alzheimers Dis 44: , 787–795. |
[14] | Huber CM , Yee C , May T , Dhanala A , Mitchell CS ((2018) ) Cognitive decline in preclinical Alzheimer’s disease: Amyloid-beta versus tauopathy. J Alzheimers Dis 61: , 265–281. |
[15] | Ricciarelli R , Fedele E ((2017) ) The amyloid cascade hypothesis in Alzheimer’s disease: It’s time to change our mind. Curr Neuropharmacol 15: , 926–935. |
[16] | Selkoe DJ , Hardy J ((2016) ) The amyloid hypothesis of Alzheimer’s disease at 25 years. EMBO Mol Med 8: , 595–608. |
[17] | Hall AM , Roberson ED ((2012) ) Mouse models of Alzheimer’s disease. Brain Res Bull 88: , 3–12. |
[18] | Buttini M , Masliah E , Yu GQ , Palop JJ , Chang S , Bernardo A , Lin C , Wyss-Coray T , Huang Y , Mucke L ((2010) ) Cellular source of apolipoprotein E4 determines neuronal susceptibility to excitotoxic injury in transgenic mice. Am J Pathol 177: , 563–569. |
[19] | Bour A , Grootendorst J , Vogel E , Kelche C , Dodart JC , Bales K , Moreau PH , Sullivan PM , Mathis C ((2008) ) Middle-aged human apoE4 targeted-replacement mice show retention deficits on a wide range of spatial memory tasks. Behav Brain Res 193: , 174–182. |
[20] | Grootendorst J , Bour A , Vogel E , Kelche C , Sullivan PM , Dodart JC , Bales K , Mathis C ((2005) ) Human apoE targeted replacement mouse lines: h-apoE4 and h-apoE3 mice differ on spatial memory performance and avoidance behavior. Behav Brain Res 159: , 1–14. |
[21] | Trommer BL , Shah C , Yun SH , Gamkrelidze G , Pasternak ES , Ye GL , Sotak M , Sullivan PM , Pasternak JF , LaDu MJ ((2004) ) ApoE isoform affects LTP in human targeted replacement mice. Neuroreport 15: , 2655–2658. |
[22] | Shi Y , Yamada K , Liddelow SA , Smith ST , Zhao L , Luo W , Tsai RM , Spina S , Grinberg LT , Rojas JC , Gallardo G , Wang K , Roh J , Robinson G , Finn MB , Jiang H , Sullivan PM , Baufeld C , Wood MW , Sutphen C , McCue L , Xiong C , Del-Aguila JL , Morris JC , Cruchaga C , Alzheimer’s Disease Neuroimaging I, Fagan AM , Miller BL , Boxer AL , Seeley WW , Butovsky O , Barres BA , Paul SM , Holtzman DM ((2017) ) ApoE4 markedly exacerbates tau-mediated neurodegeneration in a mouse model of tauopathy. Nature 549: , 523–527. |
[23] | Zerbi V , Wiesmann M , Emmerzaal TL , Jansen D , Van Beek M , Mutsaers MP , Beckmann CF , Heerschap A , Kiliaan AJ ((2014) ) Resting-state functional connectivity changes in aging apoE4 and apoE-KO mice. J Neurosci 34: , 13963–13975. |
[24] | Wisniewski T , Drummond E ((2020) ) APOE-amyloid interaction: Therapeutic targets. Neurobiol Dis 138: , 104784. |
[25] | Cramer PE , Cirrito JR , Wesson DW , Lee CY , Karlo JC , Zinn AE , Casali BT , Restivo JL , Goebel WD , James MJ , Brunden KR , Wilson DA , Landreth GE ((2012) ) ApoE-directed therapeutics rapidly clear beta-amyloid and reverse deficits in AD mouse models. Science 335: , 1503–1506. |
[26] | Mielke MM ((2018) ) Sex and gender differences in Alzheimer’s disease dementia. Psychiatr Times 35: , 14–17. |
[27] | Ungar L , Altmann A , Greicius MD ((2014) ) Apolipoprotein E, gender, and Alzheimer’s disease: An overlooked, but potent and promising interaction. Brain Imaging Behav 8: , 262–273. |
[28] | Cholerton B , Gleason CE , Baker LD , Asthana S ((2002) ) Estrogen and Alzheimer’s disease: The story so far. Drugs Aging 19: , 405–427. |
[29] | Ratnakumar A , Zimmerman SE , Jordan BA , Mar JC ((2019) ) Estrogen activates Alzheimer’s disease genes. Alzheimers Dement (N Y) 5: , 906–917. |
[30] | Schafer S , Wirths O , Multhaup G , Bayer TA ((2007) ) Gender dependent APP processing in a transgenic mouse model of Alzheimer’s disease. J Neural Transm (Vienna) 114: , 387–394. |
[31] | Vina J , Lloret A ((2010) ) Why women have more Alzheimer’s disease than men: Gender and mitochondrial toxicity of amyloid-beta peptide. J Alzheimers Dis 20: (Suppl 2), S527–533. |
[32] | Podcasy JL , Epperson CN ((2016) ) Considering sex and gender in Alzheimer disease and other dementias. Dialogues Clin Neurosci 18: , 437–446. |
[33] | Schuessel K , Leutner S , Cairns NJ , Muller WE , Eckert A ((2004) ) Impact of gender on upregulation of antioxidant defence mechanisms in Alzheimer’s disease brain. J Neural Transm (Vienna) 111: , 1167–1182. |
[34] | Liu DS , Pan XD , Zhang J , Shen H , Collins NC , Cole AM , Koster KP , Ben Aissa M , Dai XM , Zhou M , Tai LM , Zhu YG , LaDu M , Chen XC ((2015) ) APOE4 enhances age-dependent decline in cognitive function by down-regulating an NMDA receptor pathway in EFAD-Tg mice. Mol Neurodegener 10: , 7. |
[35] | Zhao N , Ren Y , Yamazaki Y , Qiao W , Li F , Felton LM , Mahmoudiandehkordi S , Kueider-Paisley A , Sonoustoun B , Arnold M , Shue F , Zheng J , Attrebi ON , Martens YA , Li Z , Bastea L , Meneses AD , Chen K , Thompson JW , St John-Williams L , Tachibana M , Aikawa T , Oue H , Job L , Yamazaki A , Liu CC , Storz P , Asmann YW , Ertekin-Taner N , Kanekiyo T , Kaddurah-Daouk R , Bu G ((2020) ) Alzheimer’s risk factors age, APOE genotype, and sex drive distinct molecular pathways. Neuron 106: , 727–742 e726. |
[36] | Riedel BC , Thompson PM , Brinton RD ((2016) ) Age, APOE and sex: Triad of risk of Alzheimer’s disease. J Steroid Biochem Mol Biol 160: , 134–147. |
[37] | Guerreiro R , Bras J ((2015) ) The age factor in Alzheimer’s disease. Genome Med 7: , 106. |
[38] | Lewandowski CT , Maldonado Weng J , LaDu MJ ((2020) ) Alzheimer’s disease pathology in APOE transgenic mouse models: The who, what, when, where, why, and how. Neurobiol Dis 139: , 104811. |
[39] | Mitchell CS , Cates A , Kim RB , Hollinger SK ((2015) ) Undergraduate biocuration: Developing tomorrow’s researchers while mining today’s data. J Undergrad Neurosci Educ 14: , A56–65. |
[40] | Brandeis R , Brandys Y , Yehuda S ((1989) ) The use of the Morris Water Maze in the study of memory and learning. Int J Neurosci 48: , 29–69. |
[41] | D’Hooge R , De Deyn PP ((2001) ) Applications of the Morris water maze in the study of learning and memory. Brain Res Brain Res Rev 36: , 60–90. |
[42] | Morris R ((1984) ) Developments of a water-maze procedure for studying spatial learning in the rat. J Neurosci Methods 11: , 47–60. |
[43] | Vorhees CV , Williams MT ((2006) ) Morris water maze: Procedures for assessing spatial and related forms of learning and memory. Nat Protoc 1: , 848–858. |
[44] | Sandi C , Loscertales M , Guaza C ((1997) ) Experience-dependent facilitating effect of corticosterone on spatial memory formation in the water maze. Eur J Neurosci 9: , 637–642. |
[45] | Song HK , Hwang DY ((2017) ) Use of C57BL/6N mice on the variety of immunological researches. Lab Anim Res 33: , 119–123. |
[46] | Van Dam D , Lenders G , De Deyn PP ((2006) ) Effect of Morris water maze diameter on visual-spatial learning in different mouse strains. Neurobiol Learn Mem 85: , 164–172. |
[47] | Zurita E , Chagoyen M , Cantero M , Alonso R , Gonzalez-Neira A , Lopez-Jimenez A , Lopez-Moreno JA , Landel CP , Benitez J , Pazos F , Montoliu L ((2011) ) Genetic polymorphisms among C57BL/6 mouse inbred strains. Transgenic Res 20: , 481–489. |
[48] | Castranio EL , Mounier A , Wolfe CM , Nam KN , Fitz NF , Letronne F , Schug J , Koldamova R , Lefterov I ((2017) ) Gene co-expression networks identify Trem2 and Tyrobp as major hubs in human APOE expressing mice following traumatic brain injury. Neurobiol Dis 105: , 1–14. |
[49] | Janssen CI , Jansen D , Mutsaers MP , Dederen PJ , Geenen B , Mulder MT , Kiliaan AJ ((2016) ) The effect of a high-fat diet on brain plasticity, inflammation and cognition in female ApoE4-knockin and ApoE-knockout mice. PLoS One 11: , e0155307. |
[50] | Mannix R , Meehan WP , Mandeville J , Grant PE , Gray T , Berglass J , Zhang J , Bryant J , Rezaie S , Chung JY , Peters NV , Lee C , Tien LW , Kaplan DL , Feany M , Whalen M ((2013) ) Clinical correlates in an experimental model of repetitive mild brain injury. Ann Neurol 74: , 65–75. |
[51] | Higuchi Y , Nelson GA , Vazquez M , Laskowitz DT , Slater JM , Pearlstein RD ((2002) ) Apolipoprotein E expression and behavioral toxicity of high charge, high energy (HZE) particle radiation.S. J Radiat Res 43: (Suppl), 219–224. |
[52] | Huang ZJ , Cao F , Wu Y , Peng JH , Zhong JJ , Jiang Y , Yin C , Guo ZD , Sun XC , Jiang L , Cheng CJ ((2020) ) Apolipoprotein E promotes white matter remodeling via the Dab1-dependent pathway after traumatic brain injury. CNS Neurosci Ther 26: , 698–710. |
[53] | Jamal M , Ameno K , Miki T , Tanaka N , Ono J , Shirakami G , Sultana R , Yu N , Kinoshita H ((2012) ) High ethanol and acetaldehyde impair spatial memory in mouse models: Opposite effects of aldehyde dehydrogenase 2 and apolipoprotein E on memory. Pharmacol Biochem Behav 101: , 443–449. |
[54] | Johnson LA , Olsen RH , Merkens LS , DeBarber A , Steiner RD , Sullivan PM , Maeda N , Raber J ((2014) ) Apolipoprotein E-low density lipoprotein receptor interaction affects spatial memory retention and brain ApoE levels in an isoform-dependent manner. Neurobiol Dis 64: , 150–162. |
[55] | Reverte I , Klein AB , Domingo JL , Colomina MT ((2013) ) Long term effects of murine postnatal exposure to decabromodiphenyl ether (BDE-209) on learning and memory are dependent upon APOE polymorphism and age. Neurotoxicol Teratol 40: , 17–27. |
[56] | Boehm-Cagan A , Michaelson DM ((2014) ) Reversal of apoE4-driven brain pathology and behavioral deficits by bexarotene. J Neurosci 34: , 7293–7301. |
[57] | Knoferle J , Yoon SY , Walker D , Leung L , Gillespie AK , Tong LM , Bien-Ly N , Huang Y ((2014) ) Apolipoprotein E4 produced in GABAergic interneurons causes learning and memory deficits in mice. J Neurosci 34: , 14069–14078. |
[58] | Lane-Donovan C , Wong WM , Durakoglugil MS , Wasser CR , Jiang S , Xian X , Herz J ((2016) ) Genetic restoration of plasma ApoE improves cognition and partially restores synaptic defects in ApoE-deficient mice. J Neurosci 36: , 10141–10150. |
[59] | Leung L , Andrews-Zwilling Y , Yoon SY , Jain S , Ring K , Dai J , Wang MM , Tong L , Walker D , Huang Y ((2012) ) Apolipoprotein E4 causes age- and sex-dependent impairments of hilar GABAergic interneurons and learning and memory deficits in mice. PLoS One 7: , e53569. |
[60] | Liraz O , Boehm-Cagan A , Michaelson DM ((2013) ) ApoE4 induces Abeta42, tau, and neuronal pathology in the hippocampus of young targeted replacement apoE4 mice. Mol Neurodegener 8: , 16. |
[61] | Loizzo S , Rimondini R , Travaglione S , Fabbri A , Guidotti M , Ferri A , Campana G , Fiorentini C ((2013) ) CNF1 increases brain energy level, counteracts neuroinflammatory markers and rescues cognitive deficits in a murine model of Alzheimer’s disease. PLoS One 8: , e65898. |
[62] | Moreau PH , Bott JB , Zerbinatti C , Renger JJ , Kelche C , Cassel JC , Mathis C ((2013) ) ApoE4 confers better spatial memory than apoE3 in young adult hAPP-Yac/apoE-TR mice. Behav Brain Res 243: , 1–5. |
[63] | Raber J , Wong D , Buttini M , Orth M , Bellosta S , Pitas RE , Mahley RW , Mucke L ((1998) ) Isoform-specific effects of human apolipoprotein E on brain function revealed in ApoE knockout mice: Increased susceptibility of females. Proc Natl Acad Sci U S A 95: , 10914–10919. |
[64] | Tong LM , Djukic B , Arnold C , Gillespie AK , Yoon SY , Wang MM , Zhang O , Knoferle J , Rubenstein JL , Alvarez-Buylla A , Huang Y ((2014) ) Inhibitory interneuron progenitor transplantation restores normal learning and memory in ApoE4 knock-in mice without or with Abeta accumulation. J Neurosci 34: , 9506–9515. |
[65] | Tong LM , Yoon SY , Andrews-Zwilling Y , Yang A , Lin V , Lei H , Huang Y ((2016) ) Enhancing GABA signaling during middle adulthood prevents age-dependent GABAergic interneuron decline and learning and memory deficits in ApoE4 mice. J Neurosci 36: , 2316–2322. |
[66] | Yin J , Nielsen M , Carcione T , Li S , Shi J ((2019) ) Apolipoprotein E regulates mitochondrial function through the PGC-1alpha-sirtuin 3 pathway. Aging (Albany NY) 11: , 11148–11156. |
[67] | Yin J , Nielsen M , Li S , Shi J ((2019) ) Ketones improves Apolipoprotein E4-related memory deficiency via sirtuin 3. Aging (Albany NY) 11: , 4579–4586. |
[68] | Davies SS , Bodine C , Matafonova E , Pantazides BG , Bernoud-Hubac N , Harrison FE , Olson SJ , Montine TJ , Amarnath V , Roberts LJ , 2nd ((2011) ) Treatment with a gamma-ketoaldehyde scavenger prevents working memory deficits in hApoE4 mice. J Alzheimers Dis 27: , 49–59. |
[69] | Harris FM , Brecht WJ , Xu Q , Tesseur I , Kekonius L , Wyss-Coray T , Fish JD , Masliah E , Hopkins PC , Scearce-Levie K , Weisgraber KH , Mucke L , Mahley RW , Huang Y ((2003) ) Carboxyl-terminal-truncated apolipoprotein E4 causes Alzheimer’s disease-like neurodegeneration and behavioral deficits in transgenic mice. Proc Natl Acad Sci U S A 100: , 10966–10971. |
[70] | Shenk JC , Liu J , Fischbach K , Xu K , Puchowicz M , Obrenovich ME , Gasimov E , Alvarez LM , Ames BN , Lamanna JC , Aliev G ((2009) ) The effect of acetyl-L-carnitine and R-alpha-lipoic acid treatment in ApoE4 mouse as a model of human Alzheimer’s disease. J Neurol Sci 283: , 199–206. |
[71] | Thomas R , Zuchowska P , Morris AW , Marottoli FM , Sunny S , Deaton R , Gann PH , Tai LM ((2016) ) Epidermal growth factor prevents APOE4 and amyloid-beta-induced cognitive and cerebrovascular deficits in female mice. Acta Neuropathol Commun 4: , 111. |
[72] | Champagne D , Dupuy JB , Rochford J , Poirier J ((2002) ) Apolipoprotein E knockout mice display procedural deficits in the Morris water maze: Analysis of learning strategies in three versions of the task. Neuroscience 114: , 641–654. |
[73] | Oitzl MS , Mulder M , Lucassen PJ , Havekes LM , Grootendorst J , de Kloet ER ((1997) ) Severe learning deficits in apolipoprotein E-knockout mice in a water maze task. Brain Res 752: , 189–196. |
[74] | Ruiz-Roso MB , Echeverry-Alzate V , Ruiz-Roso B , Quintela JC , Ballesteros S , Lahera V , de Las Heras N , Lopez-Moreno JA , Martin-Fernandez B ((2018) ) Low phytanic acid-concentrated DHA prevents cognitive deficit and regulates Alzheimer disease mediators in an ApoE(-/-) mice experimental model. Nutrients 11: , 11. |
[75] | Tan Y , Nie S , Zhu W , Liu F , Guo H , Chu J , Cao XB , Jiang X , Zhang Y , Li Y ((2016) ) 7,8-dihydroxyflavone ameliorates cognitive impairment by inhibiting expression of tau pathology in ApoE-knockout mice. Front Aging Neurosci 8: , 287. |
[76] | Yin J , Turner GH , Coons SW , Maalouf M , Reiman EM , Shi J ((2014) ) Association of amyloid burden, brain atrophy and memory deficits in aged apolipoprotein epsilon4 mice. Curr Alzheimer Res 11: , 283–290. |
[77] | Lin AL , Jahrling JB , Zhang W , DeRosa N , Bakshi V , Romero P , Galvan V , Richardson A ((2017) ) Rapamycin rescues vascular, metabolic and learning deficits in apolipoprotein E4 transgenic mice with pre-symptomatic Alzheimer’s disease. J Cereb Blood Flow Metab 37: , 217–226. |
[78] | Sarica A , Cerasa A , Quattrone A ((2017) ) Random forest algorithm for the classification of neuroimaging data in Alzheimer’s disease: A systematic review. Front Aging Neurosci 9: , 329. |
[79] | Chen X , Ishwaran H ((2012) ) Random forests for genomic data analysis. Genomics 99: , 323–329. |
[80] | Castellano JM , Kim J , Stewart FR , Jiang H , DeMattos RB , Patterson BW , Fagan AM , Morris JC , Mawuenyega KG , Cruchaga C , Goate AM , Bales KR , Paul SM , Bateman RJ , Holtzman DM ((2011) ) Human apoE isoforms differentially regulate brain amyloid-beta peptide clearance. Sci Transl Med 3: , 89ra57. |
[81] | Cantuti-Castelvetri L , Fitzner D , Bosch-Queralt M , Weil MT , Su M , Sen P , Ruhwedel T , Mitkovski M , Trendelenburg G , Lutjohann D , Mobius W , Simons M ((2018) ) Defective cholesterol clearance limits remyelination in the aged central nervous system. Science 359: , 684–688. |
[82] | Hudry E , Klickstein J , Cannavo C , Jackson R , Muzikansky A , Gandhi S , Urick D , Sargent T , Wrobleski L , Roe AD , Hou SS , Kuchibhotla KV , Betensky RA , Spires-Jones T , Hyman BT ((2019) ) Opposing roles of apolipoprotein E in aging and neurodegeneration. Life Sci Alliance 2: , e201900325. |
[83] | Yassine HN , Finch CE ((2020) ) APOE alleles and diet in brain aging and Alzheimer’s disease. Front Aging Neurosci 12: , 150. |
[84] | Yamazaki Y , Zhao N , Caulfield TR , Liu CC , Bu G ((2019) ) Apolipoprotein E and Alzheimer disease: Pathobiology and targeting strategies. Nat Rev Neurol 15: , 501–518. |
[85] | Liu CC , Zhao N , Fu Y , Wang N , Linares C , Tsai CW , Bu G ((2017) ) ApoE4 accelerates early seeding of amyloid pathology. Neuron 96: , 1024–1032 e1023. |
[86] | Xu Q , Bernardo A , Walker D , Kanegawa T , Mahley RW , Huang Y ((2006) ) Profile and regulation of apolipoprotein E (ApoE) expression in the CNS in mice with targeting of green fluorescent protein gene to the ApoE locus. J Neurosci 26: , 4985–4994. |
[87] | Fuentes D , Fernandez N , Garcia Y , Garcia T , Morales AR , Menendez R ((2018) ) Age-related changes in the behavior of apolipoprotein E knockout mice. Behav Sci (Basel) 8: , 33. |
[88] | Barnhart CD , Yang D , Lein PJ ((2015) ) Using the Morris water maze to assess spatial learning and memory in weanling mice. PLoS One 10: , e0124521. |
[89] | Bromley-Brits K , Deng Y , Song W (2011) Morris water maze test for learning and memory deficits in Alzheimer’s disease model mice. J Vis Exp, 2920. |
[90] | Hanson AJ , Craft S , Banks WA ((2015) ) The APOE genotype: Modification of therapeutic responses in Alzheimer’s disease. Curr Pharm Des 21: , 114–120. |
[91] | Cao J , Hou J , Ping J , Cai D ((2018) ) Advances in developing novel therapeutic strategies for Alzheimer’s disease. Mol Neurodegener 13: , 64. |
[92] | Alvarez XA , Mouzo R , Pichel V , Perez P , Laredo M , Fernandez-Novoa L , Corzo L , Zas R , Alcaraz M , Secades JJ , Lozano R , Cacabelos R ((1999) ) Double-blind placebo-controlled study with citicoline in APOE genotyped Alzheimer’s disease patients. Effects on cognitive performance, brain bioelectrical activity and cerebral perfusion. Methods Find Exp Clin Pharmacol 21: , 633–644. |
[93] | Famenini S , Rigali EA , Olivera-Perez HM , Dang J , Chang MT , Halder R , Rao RV , Pellegrini M , Porter V , Bredesen D , Fiala M ((2017) ) Increased intermediate M1-M2 macrophage polarization and improved cognition in mild cognitive impairment patients on omega-3 supplementation. FASEB J 31: , 148–160. |
[94] | Cummings JL , Zhong K , Kinney JW , Heaney C , Moll-Tudla J , Joshi A , Pontecorvo M , Devous M , Tang A , Bena J ((2016) ) Double-blind, placebo-controlled, proof-of-concept trial of bexarotene Xin moderate Alzheimer’s disease. Alzheimers Res Ther 8: , 4. |
[95] | Salloway S , Sperling R , Fox NC , Blennow K , Klunk W , Raskind M , Sabbagh M , Honig LS , Porsteinsson AP , Ferris S , Reichert M , Ketter N , Nejadnik B , Guenzler V , Miloslavsky M , Wang D , Lu Y , Lull J , Tudor IC , Liu E , Grundman M , Yuen E , Black R , Brashear HR , Bapineuzumab 301 and 302 Clinical Trial Investigators ((2014) ) Two phase 3 trials of bapineuzumab in mild-to-moderate Alzheimer’s disease. N Engl J Med 370: , 322–333. |