Pilot Evaluation of the Unsupervised, At-Home Cogstate Brief Battery in ADNI-2
Abstract
Background:
There is a need for feasible, scalable assessments to detect cognitive impairment and decline. The Cogstate Brief Battery (CBB) is validated for Alzheimer’s disease (AD) and in unsupervised and bring your own device contexts. The CBB has shown usability for self-completion in the home but has not been employed in this way in a multisite clinical trial in AD.
Objective:
The objective of the pilot was to evaluate feasibility of at-home, self-completion of the CBB in the Alzheimer’s Disease Neuroimaging Initiative (ADNI) over 24 months.
Methods:
The CBB was included as a pilot for cognitively normal (CN) and mild cognitive impairment (MCI) participants in ADNI-2, invited to take the assessment in-clinic, then at at-home over a period of 24 months follow-up. Data were analyzed to explore acceptability/usability, concordance of in-clinic and at-home assessment, and validity.
Results:
Data were collected for 104 participants (46 CN, 51 MCI, and 7 AD) who consented to provide CBB data. Subsequent analyses were performed for the CN and MCI groups only. Test completion rates were 100%for both the first in-clinic supervised and first at-home unsupervised assessments, with few repeat performances required. However, available follow-up data declined sharply over time. Good concordance was seen between in-clinic and at-home assessments, with non-significant and small effect size differences (Cohen’s d between -0.04 and 0.28) and generally moderate correlations (r = 0.42 to 0.73). Known groups validity was also supported (11/16 comparisons with Cohen’s d≥0.3).
Conclusion:
These data demonstrate the feasibility of use for the CBB for unsupervised at-home, testing, including MCI groups. Optimal approaches to the application of assessments to support compliance over time remain to be determined.
INTRODUCTION
The worldwide prevalence of cognitive dysfunction and dementia due to Alzheimer’s disease (AD) is increasing with aging populations. While the rapid development of amyloid and tau biomarkers is improving identification of AD biology, there remains a need for feasible, scalable assessments (e.g., brief, low burden/complexity, self-administered, and low cost) that can both detect mild cognitive impairment (MCI) and dementia, as well as track cognitive decline throughout the AD continuum. The Cogstate Brief Battery (CBB) is a computerized cognitive test battery, validated across multiple clinical stages of AD and related dementias (noting that cognitive impairment may have many different causes) and adapted for use in both unsupervised and bring your own device (BYOD) assessment contexts [1–5]. The CBB assesses the domains of processing speed, attention, visual learning, and working memory and has acceptable stability and test-retest reliability with minimal practice effects at short test-retest intervals in groups of healthy controls and in patients at various stages of cognitive impairment and dementia [1, 3, 6]. Clinical research studies show that performance on the memory and working memory tests from CBB declines in both the preclinical and prodromal stages of AD and cross-sectional design studies show that substantial impairments on these same tests in individuals with clinically classified MCI (Hedge’s g effect size = 2.2) and AD dementia (Hedge’s g effect size = 3.3), and high classification accuracy (AUC = 0.91 for MCI and 0.99 for AD) [2, 3]. Data from the Australian Imaging, Biomarker & Lifestyle Flagship Study of Ageing (AIBL) have shown decline over 72 months of follow-up on measures from the CBB, dependent on CDR Global score and amyloid status. These data indicate that in individuals with very mild dementia, who also have amyloid-β (Aβ)+biomarker confirmation, changes were primarily evident in learning and working memory, and were associated with hippocampal volume loss [7]. Studies investigating AD relevant biomarker correlates of CBB outcome measures have been published, with the majority finding an association with amyloid status [8]. Modest associations with other biomarkers have also been seen including hippocampal volume (measured by magnetic resonance imaging), fluorodeoxyglucose-positron emission tomography (FDG-PET), and amyloid PET in the Mayo Clinic Study of Aging (MCSA), and Aβ42 and phosphorylated-tau (p-tau) ratio measured in cerebrospinal fluid in the Wisconsin Registry for Alzheimer’s Prevention [8, 9].
The CBB cognitive tests have also been shown to have high acceptability and usability when used by older adults in unsupervised or remote contexts, such as on personal computers in their homes [4]. Although as yet, there has not been detailed examination of the equivalence of performance on the CBB between in-clinic and at home assessments in the context of a multisite clinical trial. The Alzheimer’s Disease Neuroimaging Initiative 2 (ADNI-2) study is a continuation of the previous ADNI studies, with the overall goal of validating biomarkers for AD clinical trials. ADNI is an observational study, designed to collect data relevant to the planning and conduct of AD clinical trials, and aims to inform the neuroscience of AD, identify diagnostic and prognostic markers, and outcome measures that can be used in clinical trials, and to help develop effective clinical trial scenarios. To explore the potential of unsupervised, at-home cognitive testing, the CBB was included as a pilot component of ADNI-2. The first aim of this was to determine the feasibility and acceptability of unsupervised, at-home CBB cognitive testing in ADNI. The second aim was to explore concordance between the in-clinic (baseline), supervised and the first follow-up at-home, unsupervised assessment. The third aim was to explore CBB performance in CN versus MCI populations.
MATERIALS AND METHODS
Participants
Data used in the preparation of this article were obtained from the Alzheimer’s Disease Neuroimaging Initiative (ADNI) database (http://adni.loni.usc.edu). ADNI was launched in 2003 as a public-private partnership, led by Principal Investigator Michael W. Weiner, MD. All procedures were in accordance with the ethical standards of the institutional and/or national research committee and with the Declaration of Helsinki or comparable ethical standards. The study was approved by the institutional review boards of all the participating institutions, and informed written consent was obtained from all participants at each site. ADNI-2 is a non-randomized natural history non-treatment study with a planned sample size of approximately 650 newly enrolled subjects, across approximately 55 sites from the United States and Canada. In the context of the pilot evaluation of the CBB, a subset of 189 CN and MCI study participants at selected sites were offered the opportunity to complete the CBB both in-clinic and at-home as an “optional addendum study”, in addition to participation in ADNI-2. This was a self-selecting sample with a subset of both ADNI sites and participants at those sites choosing to take up the offer of participation. Participants were invited to take the CBB while supervised on a computer located at the clinic, during one of their regularly scheduled visits and were also instructed to log-in and take the CBB at-home, unsupervised and using any device (BYOD) within 2 weeks and at 6 months, 12 months, 18 months, and 24 months. Of the 189 ADNI-2 invited participants, 55%(104) consented to undertake the CBB assessments.
Cognitive and clinical assessments
The CBB was scheduled to be completed at an initial (baseline) in-clinic evaluation, where performance was supervised; and could additionally be completed at up to five unsupervised, at-home follow-up time-points of 1-14 days, 6 months, 12 months, 18 months, and 24 months.
For both in-clinic and at-home assessments, the CBB was completed via a web browser (Firefox, Internet Explorer, Google Chrome, or Safari), with participants directed to an ADNI website and required to complete their cognitive testing in one sitting, on a desktop or laptop computer. Tests are downloaded, completed locally on the testing device, and then uploaded, to minimize any impact of internet connectivity. For the unsupervised version of the CBB, the tests remain exactly the same but the design and implementation of the instructions and delivery have been modified using a shaping approach to ensure individuals understand the context for decisions and response requirements prior to their beginning a test [5]. Participants were given instruction in accessing the tests at-home and unsupervised but could also receive additional support from the sites or from friends and family members. Additionally, test supervisors were able to provide comments related to the CBB describing any issues and observations, whether their own or raised by participants, to generate information that might be of relevance to supporting and improving the CBB assessments.
The CBB has a game-like interface which uses playing card stimuli and requires participants to provide “Yes” or “No” responses. It consists of four tests: Detection (DET), Identification (IDN), One Card Learning (OCL), and One-Back (ONB) [10].
DET is a simple reaction time test that measures psychomotor function. In this test, the participant is required to press the ‘Yes’ key as quickly as possible when the central card turns face-up (constituting 1 trial). Correct responses following an anticipatory response are ignored. The face-up card displayed is always the same joker card.
IDN is a choice reaction time test that measures visual attention. This test is presented similarly to DET, with instructions indicating the participant should respond ‘Yes’ if the face-up card is red, or ‘No’ if it is not red. The cards displayed are red or black joker cards. Joker cards are used to ensure that playing cards presented in the next test were not previously seen in the same testing session.
OCL is a continuous visual learning test that assesses visual recognition/pattern separation. This test is similar in presentation to the IDN test, with instructions indicating the participant should respond ‘Yes’ if the face-up card has appeared in the test before, and ‘No’ if it has not yet appeared. Normal playing cards of both colors and the four suits are displayed (without joker cards).
ONB assesses working memory using the N-back paradigm and is similar in presentation to the OCL test, with instructions indicating the participant should respond ‘Yes’ if the face-up card is exactly the same as the card presented immediately prior, or ‘No’ if it is not the same. Normal playing cards are again used.
For each test, the accuracy of performance was defined by the number of correct responses made (i.e., true positive and true negative), expressed as a proportion of the total trials attempted. An arcsine transformation was then applied to normalize the distribution. The speed of performance was defined in terms of the average reaction time (RT; milliseconds) for correct responses. A base 10 logarithmic transformation was then applied to normalize the distributions of mean RT.
A small subset of additional ADNI data for the participants including age, Montreal Cognitive Assessment (MoCA) total score, Alzheimer’s Disease Assessment Scale –Cognitive Subscale (ADAS-Cog) total score, and CDR (Clinical Dementia Rating) Global score was also obtained.
Statistical analyses
Data analyses occurred in four stages. First, all data collected were summarized by diagnosis at baseline (CN, MCI, AD dementia) for each time-point. The AD dementia patients were removed from the subsequent analyses, since the pilot study had not intended to recruit this group and the number recruited was very small for the purpose of evaluating feasibility. Second, acceptability and usability of each CBB test was evaluated according to a human computer interface (HCI) approach [5]. HCI acceptability was operationalized as the amount and nature of missing test data within CBB attempts (i.e., ‘completion’). HCI usability was operationalized as the participants’ ability to adhere to the requirements of each test (i.e., ‘performance’ or error). Provided a test was complete, the additional performance check was applied to ensure the test was understood in accordance with the test requirements (see Table 3 for completion and performance criteria). If a test did not meet either the completion or performance criteria, it was automatically re-administered at the end of the battery, up to a maximum of three times with the instruction “We would now like you to try some of the same tests again”. All tests could be abandoned at any point. Third, analyses were conducted to evaluate the level of concordance between the in-clinic, supervised and the first follow-up at-home, unsupervised assessment (1–14 days) using Cohen’s d effect size, and intraclass correlation coefficient (ICC). Fourth, known-groups validity for CN versus MCI, was assessed using independent samples t-tests and Cohen’s d effect size; and construct validity evaluated via correlation (Pearson’s r) with demographic and clinical characteristics. Per the ADNI procedures manual, many demographic, clinical, and biomarker parameters are available, and so a small but representative subset (age, MoCA total score, ADAS-Cog total score, and CDR Global score) were explored here. Eight outcome measures were derived from the CBB for the analyses of test performance data (Table 2). Prior studies have shown that speed (reaction time) has optimal metric properties for the DET, IDN, and ONB tests, and accuracy for OCL. However, accuracy for ONB is useful for some populations with cognitive impairment and where there are not prominent ceiling effects, including AD, and so this outcome measure was included. Furthermore, composite outcomes for psychomotor function/attention, learning/working memory, and processing speed were derived as averaged z-scores standardized using normative data.
Table 3
Demographic and clinical characteristics
Cognitively normal (CN) | Mild cognitive impairment (MCI) | Alzheimer’s disease dementia (AD) | |
N | 46 | 51 | 7 |
Sex | |||
Female, N (%) | 27 (58.7%) | 22 (43.1%) | 2 (28.6%) |
Male, N (%) | 19 (41.3%) | 29 (56.9%) | 5 (71.4%) |
Age, mean (SD) | 75.65 (6.65) | 75.98 (8.43) | 76.29 (7.02) |
MMSE, mean (SD) | 28.87 (1.22) | 27.67 (2.04) | 24.43 (2.70) |
CDR Global, mean (SD) | 0.06 (0.16) | 0.39 (0.23) | 0.71 (0.27) |
GDS, mean (SD) | 1.11 (1.27) | 2.45 (2.53) | 1.86 (1.35) |
FAQ, mean (SD) | 0.27 (1.64) | 2.34 (3.19) | 13.29 (6.45) |
MoCA Total, mean (SD) | 25.80 (2.98) | 23.53 (3.18) | 17.71 (5.09) |
ADAS-Cog, mean (SD) | 4.96 (2.91) | 8.69 (4.04) | 18.57 (7.64) |
Initial supervised, in-clinic assessment (baseline). MMSE, Mini-Mental Status Exam; CDR, Clinical Dementia Rating; FAQ, Functional Activities Questionnaire; MoCA, Montreal Cognitive Assessment; ADAS-Cog, Alzheimer’s Disease Assessment Scale –Cognitive Subscale; GDS, Geriatric Depression Scale.
Table 2
Cogstate Brief Battery tests and outcome measures
Test | Abbreviation | Domain | Paradigm | Completion criterion | Performance criterion | Outcome measures | Range |
Detection | DET speed | Psychomotor function | Simple reaction time | ≥100%of trial responses | ≥70%accuracy | reaction time in ms (speed), normalized by log10 transformation | 0 to 3.69* |
Identification | IDN speed | Attention | Choice reaction time | ≥100%of trial responses | ≥70%accuracy | reaction time in ms (speed), normalized by log10 transformation | 0 to 3.69* |
One Card Learning | OCL accuracy | Visual learning | Pattern separation | ≥100%of trial responses | ≥40%accuracy | proportion of correct answers (accuracy), normalized by arcsine square-root transformation | 0–1.57 |
One Back | ONB speed | Working memory | N-back | ≥100%of trial responses | ≥50%accuracy | reaction time in ms (speed), normalized by log10 transformation | 0 to 3.69* |
ONB accuracy | proportion of correct answers (accuracy), normalized by arcsine square-root transformation | 0–1.57 | |||||
Psychomotor function and attention | DET/IDN speed | Composite | Composite | 2/2 test instances available | 2/2 test instances available | average of z-scores for DET and IDN speed | –5 to 5 |
Learning and working memory | LWM accuracy | Composite | Composite | 2/2 test instances available | 2/2 test instances available | average of z-scores for OCL and ONB accuracy | –5 to 5 |
Learning and working memory processing speed | OCL/ONB speed | Composite | Composite | 2/2 test instances available | 2/2 test instances available | average of z-scores for OCL accuracy and ONB speed | –5 to 5 |
*Reaction times longer than 5 s (i.e., log10 [5000]) are excluded as reflecting responses that are abnormally slow.
Effect size data were interpreted qualitatively as d < 0.2 ‘trivial’, d≥0.2 to < 0.5 ‘small’, d≥0.5 to < 0.8 ‘medium’, and d≥0.8 ‘large’. Correlations were interpreted qualitatively as r≥0 to < 0.1 ‘negligible’, r≥0.1 to < 0.4 ‘weak’, r≥0.4 to < 0.7 ‘moderate’, r≥0.7 to < 0.9 ‘strong’, and r≥0.9 to = 1 ‘very strong’.
RESULTS
Participants
Data were collected for 104 participants at the initial in-clinic (baseline) assessment (49.0%Female; mean age 75.9 years (SD 7.53), range 59–97) (Table 3). Of these, there were 46 CN participants, 51 MCI, and 7 AD. At follow-up 1 (1–14 days), data were available for 77 CN and MCI participants (79.4%), dropping to 37.1%at 6 months, 13.4%at 12 months, 5.2%at 18 months, and < 1%at 24 months (Table 1). The average time required to complete the CBB was 17.2 minutes (SD 3.90) at the in-clinic (baseline) assessment and 15.9 min (SD 4.32) for the first at-home follow-up.
Table 1
Number of participants with CBB data at each assessment time-point
All | CN | MCI | AD | |
Offered Participation | 189 | . | . | . |
Consented to Participate | 104 | . | . | . |
In-clinic (baseline) | 104 | 46 | 51 | 7 |
1–14 days (at-home) | 80 | 37 | 40 | 3 |
6 months (at-home) | 37 | 20 | 16 | 1 |
12 months (at-home) | 13 | 9 | 4 | 0 |
18 months (at-home) | 5 | 5 | 0 | 0 |
24 months (at-home) | 1 | 1 | 0 | 0 |
Test usability and acceptability
Completion and performance pass rates were high, with 100%pass rates for all CN and MCI participants.
The rates of repeat test performance triggered by completion or performance check failures were low, with only OCL having a second assessment in the CN participants (2.3%supervised and 2.7%unsupervised). For the MCI participants, more repeats were required, with a range of 0%(DET supervised) to 8.0%(OCL supervised) requiring a second attempt, and only OCL supervised (2%) requiring a third attempt (Table 4).
Table 4
Completion rates and number of test attempts for initial in-clinic and first at-home assessments
Test | Setting | Completion Pass Rate | Performance Check Pass Rate | Number of Attempts to Fulfill Criteria | |||||||
1 | 2 | 3 | |||||||||
CN | MCI | CN | MCI | CN | MCI | CN | MCI | CN | MCI | ||
DET | In-clinic | 46 (100%) | 51 (100%) | 46 (100%) | 51 (100%) | 46 (100%) | 51 (100%) | 0 (0%) | 0 (0%) | 0 (0%) | 0 (0%) |
At-home | 36 (100%) | 40 (100%) | 36 (100%) | 40 (100%) | 36 (100%) | 37 (92.5%) | 0 (0%) | 3 (7.5%) | 0 (0%) | 0 (0%) | |
IDN | In-clinic | 46 (100%) | 51 (100%) | 46 (100%) | 51 (100%) | 46 (100%) | 49 (96.1%) | 0 (0%) | 2 (3.9%) | 0 (0%) | 0 (0%) |
At-home | 37 (100%) | 40 (100%) | 37 (100%) | 40 (100%) | 37 (100%) | 39 (97.5%) | 0 (0%) | 1 (2.5%) | 0 (0%) | 0 (0%) | |
OCL | In-clinic | 46 (100%) | 51 (100%) | 46 (100%) | 51 (100%) | 45 (97.7%) | 44 (90.0%) | 1 (2.3%) | 4 (8.0%) | 0 (0%) | 1 (2.0%) |
At-home | 36 (100%) | 40 (100%) | 36 (100%) | 40 (100%) | 35 (97.3%) | 37 (92.5%) | 1 (2.7%) | 3 (7.5%) | 0 (0%) | 0 (0%) | |
ONB | In-clinic | 46 (100%) | 51 (100%) | 46 (100%) | 51 (100%) | 46 (100%) | 49 (96.0%) | 0 (0%) | 2 (4.0%) | 0 (0%) | 0 (0%) |
At-home | 36 (100%) | 40 (100%) | 36 (100%) | 40 (100%) | 36 (100%) | 39 (97.5%) | 0 (0%) | 1 (2.5%) | 0 (0%) | 0 (0%) |
Data for AD dementia patients was removed (N = 7).
Concordance between in-clinic and at-home assessments
The range of effect size differences between the in-clinic baseline and first at-home follow-up assessments for the CN and MCI groups was –0.04 to 0.28 and there was substantial overlap of 95%CIs in all cases (Table 4). The largest of these differences (0.28) reflected slower performance for the processing speed and attention z-score composite (DET/IDN) for the at-home follow-up assessment versus in-clinic, in the MCI participants only (Table 5). A moderate (r = 0.42 to 0.73) and statistically significant (p < 0.001) association was evident between the in-clinic baseline and at-home follow-up for all outcome measures, with the exception of ONB accuracy (r = 0.22; p = 0.003) where a restricted range and high proportion of instances of performance near to or at ceiling were evident in the data (Fig. 1E). The strongest association (r = 0.73; p < 0.001) was observed for DET speed (Fig. 1A).
Table 5
In-clinic and at-home assessment and between group differences
Outcome | Group | N | In-clinic (baseline) | At-home (1–14 days) | In-clinic versus At-home Cohen’s d | CN versus MCI (In-clinic) p, Cohen’s d | CN versus MCI (At-home) p, Cohen’s d | ||
Mean (SD) | 95%CI | Mean (SD) | 95%CI | ||||||
DET speed | CN | 36 | 2.61 (0.14) | 2.57, 2.66 | 2.62 (0.11) | 2.58, 2.65 | d = –0.04 | p = 0.75, | p = 0.19, |
MCI | 40 | 2.62 (0.12) | 2.59, 2.66 | 2.65 (0.15) | 2.61, 2.7 | d = –0.27 | d = –0.08 | d = –0.30 | |
IDN speed | CN | 37 | 2.77 (0.10) | 2.74, 2.8 | 2.75 (0.07) | 2.73, 2.78 | d = 0.24 | p = 0.71, | p = 0.028, |
MCI | 40 | 2.78 (0.07) | 2.76, 2.8 | 2.79 (0.07) | 2.77, 2.81 | d = –0.17 | d = –0.09 | d = –0.51 | |
OCL accuracy | CN | 36 | 0.96 (0.11) | 0.93, 1 | 0.97 (0.11) | 0.93, 1 | d = –0.07 | p = 0.020, | p = 0.079, |
MCI | 40 | 0.90 (0.11) | 0.87, 0.94 | 0.92 (0.10) | 0.89, 0.95 | d = –0.14 | d = 0.55 | d = 0.41 | |
ONB speed | CN | 36 | 2.95 (0.08) | 2.92, 2.98 | 2.94 (0.09) | 2.91, 2.97 | d = 0.08 | p = 0.012 | p = 0.028, |
MCI | 40 | 3.00 (0.10) | 2.97, 3.03 | 2.99 (0.09) | 2.96, 3.01 | d = 0.21 | d = –0.60 | d = –0.51 | |
ONB accuracy | CN | 36 | 1.33 (0.18) | 1.27, 1.39 | 1.35 (0.13) | 1.3, 1.39 | d = –0.09 | p = 0.34 | p = 0.34, |
MCI | 40 | 1.29 (0.18) | 1.23, 1.35 | 1.31 (0.17) | 1.26, 1.37 | d = –0.11 | d = 0.22 | d = 0.22 | |
DET/IDN speed | CN | 37 | –0.38 (1.23) | –0.78, 0.02 | –0.21 (0.89) | –0.5, 0.07 | d = –0.18 | p = 0.997, | p = 0.086, |
MCI | 40 | –0.38 (0.88) | –0.65, –0.11 | –0.58 (0.95) | –0.87, –0.29 | d = 0.28 | d = –0.001 | d = 0.40 | |
OCL/ONB speed | CN | 36 | 0.03 (0.64) | –0.18, 0.24 | 0.08 (0.66) | –0.14, 0.3 | d = –0.11 | p = 0.003, | p = 0.008, |
MCI | 40 | –0.44 (0.68) | –0.65, –0.23 | –0.30 (0.55) | –0.47, –0.13 | d = –0.22 | d = 0.71 | d = 0.62 | |
LWM accuracy | CN | 36 | 0.02 (0.82) | –0.25, 0.28 | 0.10 (0.59) | –0.09, 0.29 | d = –0.11 | p = 0.061, | p = 0.11, |
MCI | 40 | –0.33 (0.78) | –0.57, –0.09 | –0.19 (0.84) | –0.45, 0.07 | d = –0.15 | d = 0.44 | d = 0.37 |
N reflects available data at first, at-home, unsupervised assessment; Data for AD dementia patients was removed (N = 3 completed an at-home assessment 1–14 days post the in-clinic baseline); p-values from independent samples t-tests; In-clinic data is for the baseline and at-home data is for the first follow-up, 1–14 days later.
Fig. 1
Scatterplots for reliability between in-clinic and at-home (FU1) assessments. A) DET speed. B) IDN speed. C) OCL accuracy. D) ONB speed. E) ONB accuracy. F) DET/IDN speed. G) LWM accuracy. H) OCL/ONB speed.
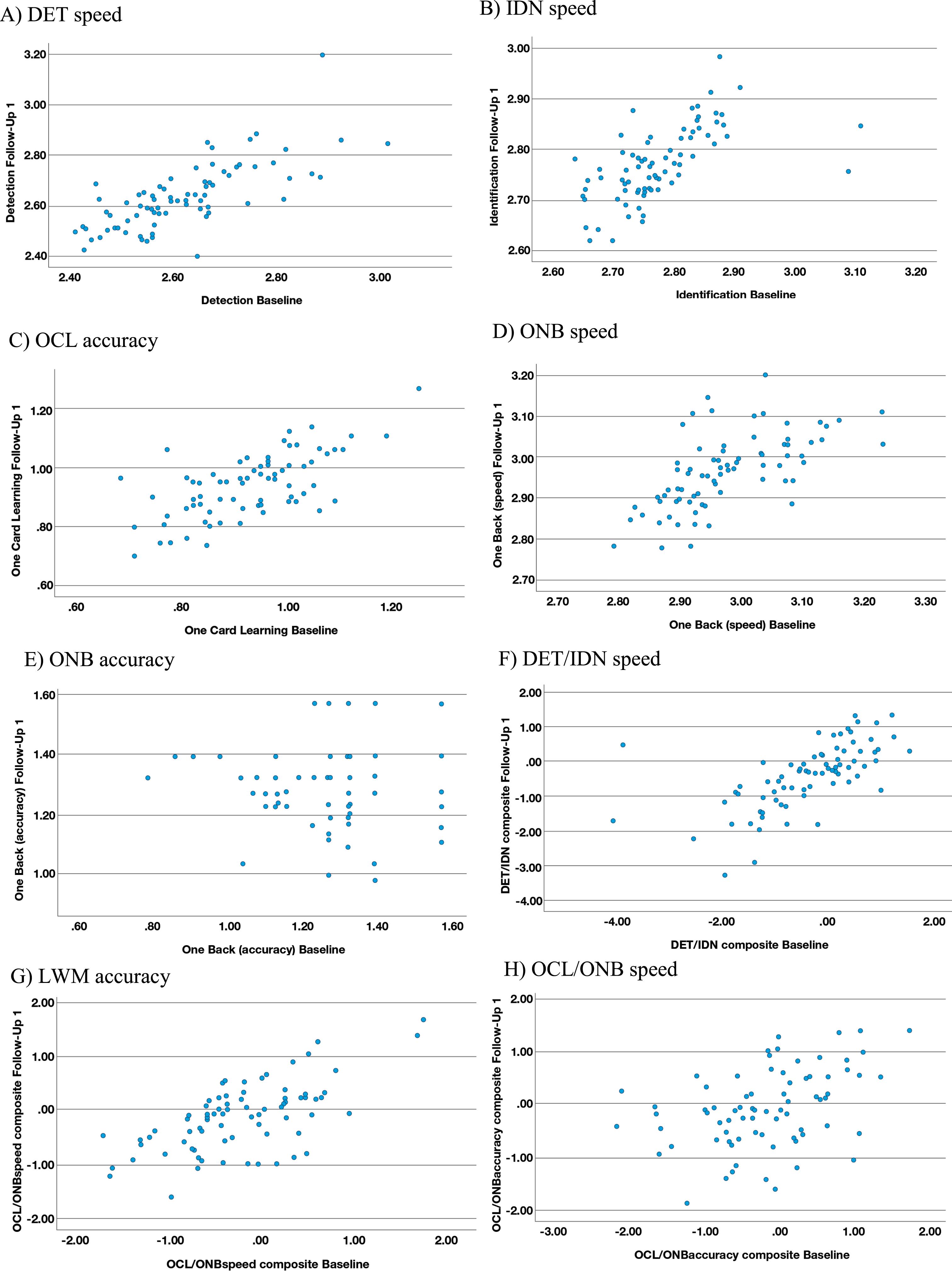
Known groups validity
At both the in-clinic baseline and first at-home follow-up assessments, test performance was poorer for all test outcome measures for the MCI versus the CN group, with the exception of the in-clinic assessment for the DET/IDN speed composite, where the groups were not different (p = 0.997, ES = –0.001). Across the outcome measures 6/16 differences were statistically significant (p≤0.05) and 11/16 showed relevant effect size of impairment (Cohen’s d≥0.3), with a range of –0.3 (DET at-home) to 0.71 (OCL/ONB speed in-clinic). Consistent with this, the three z-score composites derived using normative data showed expected effect size impairment for the MCI group versus age matched norms in the range 0.33 to 0.44 at the in-clinic baseline. Correspondingly, there was no impairment in the CN group (Cohen’s d≤0.1) for OCL/ONB speed and the Learning and working memory accuracy composite (LWM accuracy). However, the DET/IDN speed composite showed some evidence for impairment in the CN group, which was comparable to the MCI group at the in-clinic baseline, as noted (Table 5).
Construct validity evaluated in the pooled CN and MCI data via correlation with age, ADAS-Cog total score, MOCA total scores, and CDR Global score at the in-clinic baseline, was evident for several of the CBB outcome measures (Table 6). For all outcome measures the association with age was in the direction of poorer performance with increasing age. This relationship with age was statistically significant for 7/8 CBB outcome measures (p≤0.32) with a range of r = 0.21 to r = 0.34. For the relationship with ADAS-Cog and MoCA there were only four associations where r was≥0.4 and supportive of construct validity: 1) OCL arcsine accuracy with ADAS-Cog (r = –0.49, p < 0.001); 2) OCL arcsine accuracy with MoCA (r = 0.52, p < 0.001); 3) OCL/ONB z-score composite speed with ADAS-Cog (r = –0.44, p < 0.001); and 4) OCL/ONB z-score composite reaction time speed with MoCA (r = 0.48, p < 0.001). No correlation≥0.4 was observed between the CDR and CBB, with the strongest correlation being for OCL arcsine accuracy (r = –0.35, p = 0.001).
Table 6
Construct validity
Outcome | Age | ADAS-Cog Total Score | MOCA Total Score | CDR Global Score |
DET speed | r = 0.20, p = 0.06 | r = 0.21, p = 0.043 | r = –0.33, p = 0.001 | r = 0.03, p = 0.78 |
IDN speed | r = 0.25, p = 0.014 | r = 0.26, p = 0.010 | r = –0.34, p = 0.001 | r = –0.05, p = 0.62 |
OCL accuracy | r = –0.27, p = 0.009 | r = –0.50, p < 0.001 | r = 0.55, p < 0.001 | r = –0.35, p = 0.001 |
ONB speed | r = 0.31, p = 0.002 | r = 0.23, p = 0.030 | r = –0.27, p = 0.009 | r = 0.10, p = 0.33 |
ONB speed | r = –0.31, p = 0.002 | r = –0.18, p = 0.09 | r = 0.28, p = 0.007 | r = 0.06, p = 0.59 |
DET/IDN speed | r = –0.12, p = 0.26 | r = –0.24, p = 0.017 | r = 0.34, p = 0.001 | r = 0.01, p = 0.89 |
OCL/ONB speed | r = –0.22, p = 0.041 | r = –0.45, p < 0.001 | r = 0.48, p < 0.001 | r = –0.31, p = 0.002 |
LWM accuracy | r = –0.26, p = 0.013 | r = –0.38, p < 0.001 | r = 0.47, p < 0.001 | r = –0.16, p = 0.13 |
Bolded values are Pearson’s r≥0.4. MMSE, Mini-Mental Status Exam; CDR, Clinical Dementia Rating; FAQ, Functional Activities Questionnaire; MoCA, Montreal Cognitive Assessment; ADAS-Cog, Alzheimer’s Disease Assessment Scale –Cognitive Subscale; GDS, Geriatric Depression Scale; LWM, Learning and Working Memory.
DISCUSSION
The results of this study indicate that the four tests from the CBB have high acceptability and usability when administered to CN and MCI older adults in both an in-clinic, supervised settings and an unsupervised at home setting. Test completion and performance criteria were met for 100%of the initial in-clinic baseline and first at-home follow-ups. Repeated attempts at the individual tests exceeded 5%in only three cases, which were all seen for the MCI group, suggesting that the ability to have a further test attempt is of clear value in generating additional, valid performance data. Prior studies have supported the feasibility of at-home assessment in older adults using the CBB tests, suggesting a large majority of individuals will successfully complete at least one assessment, though with increasingly lower numbers completing multiple or longer-term follow-up assessments [4, 11].
Despite the high acceptability associated with test attempts, there was a large drop out from the prospective part of the study over the 2 years, with the number of ADNI participants completing the CBB declining sharply over the assessment period, falling from around 80%at 1–14 days, to around 13%at one year. The rapid loss of participants from online longitudinal prospective studies is a common feature of studies depending solely on remote assessment of clinical or cognitive symptoms (e.g., mPower study in Parkinson’s disease) [12] and a previous remote, unsupervised study of CBB test completion found 95%of older adults successfully completed a valid baseline assessment, 67%3 month, and 43%12 month follow-ups [4]. This may be contrasted with supervised use in clinical trials over short-term follow-up, where no systematic issues with missing data have been observed, even in AD dementia [13–15] and successful data collection in longer-term registries, e.g., AIBL and MCSA, with the latter including unsupervised at-home assessment [7, 16]. This suggests that if remote assessments are to be used successfully to understand clinical disease progression, strategies will have to be implemented to encourage and support both sites and participants to remain engaged and compliant in the studies. As this study was a pilot, assessments with the CBB were not part of the main study protocol, therefore no formal reminders or participant follow-up was given. This absence may have reduced perceptions of the value of the remote cognitive tests. The tests themselves, as with other clinical assessments may not hold value as entertaining or engaging, they do not provide health information in the form of feedback to participants, or other benefit such as brain training, and were not mandated. Therefore, clear instructions for site staff, engagement of sites and patients in the value and importance of data, and ongoing support, engagement and reminders would all be important features of future studies utilizing at-home, unsupervised assessment.
The level of concordance between the in-clinic (baseline) at first at-home assessment was high. Across the outcome measures the largest effect size difference was 0.28 and 11/16 comparisons had an effect size difference < 0.2, suggesting differences were generally trivial to small. Additionally, there was substantial overlap in estimates of variability. The two assessment time-points were correlated in the range of r = 0.42 to 0.73, except for ONB accuracy (r = 0.22), which was most likely due to the presence of an expected range restriction and ceiling effect in the sample for this outcome measure. It should be noted that the study was not designed or intended as an equivalence study and did not counterbalance the order of assessment of at-home and in-clinic and so it is possible that there were sequential effects on test performance such as familiarization. For this reason, the inclusion of pre-baseline ‘practice’ assessments for cognitive tests is a common recommendation for studies with sequential assessment [17, 18]. Additionally, the in-clinic assessment was supervised, whilst the at-home assessment was not, perhaps introducing factors such as a ‘white-coat’ effect. Despite this, established criteria for equivalence (ICC≥0.7 and mean difference Cohen’s d < 0.2) [19] were met in some cases. Two previous published studies using the CBB tests have suggested that there is not a strong effect of unsupervised assessment or test environment [5, 20]. Analyzing data from the MCSA, Stricker et al. concluded that the location where the CBB was completed (in-clinic or at-home) had an important impact on performance. However, this was a self-selecting sample where participants chose their preferred setting, so an element of bias beyond the controlled for differences in age, education, number of sessions completed, and duration of follow-up, cannot be discounted [16]. Additional studies specifically designed to assess equivalence are needed to fully resolve these questions as well as the influence of setting (in-clinic versus at-home) and supervision (supervised versus unsupervised), since remote supervised assessment could be proctored using telephone or video call. Importantly, equivalence may not always be relevant, since many studies will be designed to avoid the potential for noise or confounding that could be introduced by changing elements such as setting or supervision by keeping this fixed.
Individuals with MCI consistently performed more poorly on the CBB outcome measures than CN participants, with the largest (> 0.5) and most consistent effect size differences observed for the OCL accuracy, ONB speed, and the OCL/ONB speed and LWM accuracy composites. These effect sizes are smaller than the usual criteria defining MCI and also what has been seen previously for the CBB [2, 21]. Further work is required to explore the extent to which such findings may reflect characteristics of the ADNI-2 CBB sample from this pilot study.
Evidence for construct validity against ADAS-Cog and MoCA was seen for OCL accuracy and OCL/ONB speed, which may reflect the relatively greater focus of these two clinical tests on aspects of memory, but the lesser contribution of psychomotor speed, attention, and working memory. Notable correlations with the CDR Global score were not observed though. Prior data has shown that in MCI and AD dementia patients, a stronger relationship was observed between the CDR sum of boxes and LWM accuracy (r = 0.76) than for DET/IDN speed (r = 0.58) [2].
From the additional qualitative feedback obtained from test supervisors and participants, two issues had somewhat greater prominence, which were difficulty accessing the website (reported on 10 occasions by test supervisors and three occasions by participants) and difficulty remembering the D and K buttons on their own computers as “No” and “Yes” respectively (reported on five occasions by test supervisors and two occasions by participants). Other issues were infrequent (≤4 instances in total). Website access, perhaps driven by specific browser requirements is an important barrier to entry for web-based studies, and the ability to check for browser and/or support study participants, e.g., with browser updates should be considered during trial planning. Difficulty remembering key positioning might reflect cognitive impairment leading to poorer test outcomes and given the high levels of complete and appropriate performance, may not require any specific action.
There are several important limitations of the present study, especially given the pilot nature. These include the self-selected sample of participants, the post-hoc nature of some of the analyses, the relatively small sample size (especially for assessments at 6 months or later after baseline), and the design, which did not attempt to counterbalance the in-clinic and at-home assessment. Additionally, more data regarding both those participants who did not consent to participate in the CBB assessment, and those dropping out from the pilot as well as a more comprehensive approach to collecting participant experience data, would have been informative. This could include some ability to remotely supervise assessments to gain further insight into conduct of the assessments.
The CBB may have advantages versus traditional neuropsychological assessment tools, including its relative brevity and the ability for remote, unsupervised assessment in very large and geographically dispersed populations; however, in contrast to more detailed evaluations, the CBB does not assess some cognitive domains and test paradigms that may be of particular value to clinical research and clinical trials in AD, for example, visuo-perceptive, or visuo-constructional abilities and verbal memory. These potential limitations of the CBB must be weighed against more traditional tests such as auditory verbal learning, which may require > 30 min with a delayed recall/recognition component, as well as a trained and qualified expert to administer and score the assessment.
Important future directions include consideration of enhancements to the assessments that may further support test completion including ease of access and understanding of test requirements. Perhaps most importantly though, measures to support and increase compliance for longer-term follow-up are needed. This could include a system of alerts and reminders operating within or external to the CBB itself, as well as exploration of techniques specifically focused on issues of compliance, and retention as they relate to remote, unsupervised cognitive assessment.
In conclusion, these pilot data are supportive of the feasibility of the CBB in both CN and MCI individuals at initial in-clinic (baseline), supervised, and at-home (1–14 days follow-up), unsupervised contexts. These initial assessment data also give support to maintained validity and reliability in these two contexts. The CBB is currently part of the ADNI-3 study, which will further confirm validity and reliability in a larger sample and provide additional opportunities to evaluate sensitivity to disease progression, association with biomarker data, and predictive validity. The ability to conduct these assessments at-home and unsupervised, provides opportunities for additional data collection, that may provide new clinical insights, whilst also lowering patient and site burden. Optimal approaches to supporting the delivery and conduct of such assessments for longer-term follow-up, including the relative importance of setting, supervision, and other factors, remains to be determined.
ACKNOWLEDGMENTS
Data collection and sharing for this project was funded by the Alzheimer’s Disease Neuroimaging Initiative (ADNI) (National Institutes of Health Grant U01 AG024904) and DOD ADNI (Department of Defense award number W81XWH-12-2-0012). ADNI is funded by the National Institute on Aging, the National Institute of Biomedical Imaging and Bioengineering, and through generous contributions from the following: AbbVie, Alzheimer’s Association; Alzheimer’s Drug Discovery Foundation; Araclon Biotech; BioClinica, Inc.; Biogen; Bristol-Myers Squibb Company; CereSpir, Inc.; Cogstate; Eisai Inc.; Elan Pharmaceuticals, Inc.; Eli Lilly and Company; EuroImmun; F. Hoffmann-La Roche Ltd and its affiliated company Genentech, Inc.; Fujirebio; GE Healthcare; IXICO Ltd.; Janssen Alzheimer Immunotherapy Research & Development, LLC.; Johnson & Johnson Pharmaceutical Research & Development LLC.; Lumosity; Lundbeck; Merck & Co., Inc.; Meso Scale Diagnostics, LLC.; NeuroRx Research; Neurotrack Technologies; Novartis Pharmaceuticals Corporation; Pfizer Inc.; Piramal Imaging; Servier; Takeda Pharmaceutical Company; and Transition Therapeutics. The Canadian Institutes of Health Research is providing funds to support ADNI clinical sites in Canada. Private sector contributions are facilitated by the Foundation for the National Institutes of Health (http://www.fnih.org). The grantee organization is the Northern California Institute for Research and Education, and the study is coordinated by the Alzheimer’s Therapeutic Research Institute at the University of Southern California. ADNI data are disseminated by the Laboratory for Neuro Imaging at the University of Southern California.
Ethical approval (humans): As per ADNI protocols, all procedures performed in studies involving human participants were in accordance with the ethical standards of the institutional and/or national research committee and with the Declaration of Helsinki or comparable ethical standards. The study was approved by the institutional review boards of all the participating institutions, and informed written consent was obtained from all participants at each site. More details can be found at http://adni.loni.usc.edu/.
Authors’ disclosures available online (https://www.j-alz.com/manuscript-disclosures/21-0201r2).
REFERENCES
[1] | Hammers D , Spurgeon E , Ryan K , Persad C , Barbas N , Heidebrink J , Darby D , Giordani B ((2012) ) Validity of a brief computerized cognitive screening test in dementia. J Geriatr Psychiatry Neurol 25: , 89–99. |
[2] | Maruff P , Lim YY , Darby D , Ellis KA , Pietrzak RH , Snyder PJ , Bush AI , Szoeke C , Schembri A , Ames D , Masters CL ((2013) ) Clinical utility of the Cogstate Brief Battery in identifying cognitive impairment in mild cognitive impairment and Alzheimer’s disease. BMC Psychol 1: , 30. |
[3] | Lim YY , Maruff P , Pietrzak RH , Ellis KA , Darby D , Ames D , Harrington K , Martins RN , Masters CL , Szoeke C , Savage G , Villemagne VL , Rowe CC ((2014) ) Aβ and cognitive change: Examining the preclinical and prodromal stages of Alzheimer’s disease. Alzheimers Dement 10: , 743–751.e1. |
[4] | Darby DG , Fredrickson J , Pietrzak RH , Maruff P , Woodward M , Brodtmann A ((2014) ) Reliability and usability of an internet-based computerized cognitive testing battery in community-dwelling older people. Comput Human Behav 30: , 199–205. |
[5] | Perin S , Buckley RF , Pase MP , Yassi N , Lavale A , Wilson PH , Schembri A , Maruff P , Lim YY ((2020) ) Unsupervised assessment of cognition in the Healthy Brain Project: Implications for web-based registries of individuals at risk for Alzheimer’s disease. Alzheimers Dement (N Y) 6: , e12043. |
[6] | Lim YY , Jaeger J , Harrington K , Ashwood T , Ellis KA , Stoffler A , Szoeke C , Lachovitzki R , Martins RN , Villemagne VL , Bush A , Masters CL , Rowe CC , Ames D , Darby D , Maruff P ((2013) ) Three-month stability of the CogState Brief Battery in healthy older adults, mild cognitive impairment, and Alzheimer’s disease: Results from the Australian Imaging, Biomarkers, and Lifestyle-Rate of Change Substudy (AIBL-ROCS). Arch Clin Neuropsychol 28: , 320–330. |
[7] | Lim YY , Villemagne VL , Laws SM , Pietrzak RH , Ames D , Fowler C , Rainey-Smith S , Snyder PJ , Bourgeat P , Martins RN , Salvado O , Rowe CC , Masters CL , Maruff P ((2016) ) Performance on the Cogstate Brief Battery is related to amyloid levels and hippocampal volume in very mild dementia. J Mol Neurosci 60: , 362–370. |
[8] | Racine AM , Clark LR , Berman SE , Koscik RL , Mueller KD , Norton D , Nicholas CR , Blennow K , Zetterberg H , Jedynak B , Bilgel M , Carlsson CM , Christian BT , Asthana S , Johnson SC ((2016) ) Associations between performance on an Abbreviated CogState Battery, other measures of cognitive function, and biomarkers in people at risk for Alzheimer’s disease. J Alzheimers Dis 54: , 1395–1408. |
[9] | Mielke MM , Weigand SD , Wiste HJ , Vemuri P , Machulda MM , Knopman DS , Lowe V , Roberts RO , Kantarci K , Rocca WA , Jack CR , Petersen RC ((2014) ) Independent comparison of CogState computerized testing and a standard cognitive battery with neuroimaging. Alzheimers Dement 10: , 779–789. |
[10] | Lim YY , Ellis KA , Harrington K , Ames D , Martins RN , Masters CL , Rowe C , Savage G , Szoeke C , Darby D , Maruff P , the AIBL Research Group ((2012) ) Use of the CogState Brief Battery in the assessment of Alzheimer’s disease related cognitive impairment in the Australian Imaging, Biomarkers and Lifestyle (AIBL) study. J Clin Exp Neuropsychol 34: , 345–358. |
[11] | Rentz DM , Dekhtyar M , Sherman J , Burnham S , Blacker D , Aghjayan SL , Papp K V , Amariglio RE , Schembri A , Chenhall T , Maruff P , Aisen P , Hyman BT , Sperling RA ((2016) ) The feasibility of at-home iPad cognitive testing for use in clinical trials. J Prev Alzheimers Dis 3: , 8–12. |
[12] | Bot BM , Suver C , Neto EC , Kellen M , Klein A , Bare C , Doerr M , Pratap A , Wilbanks J , Dorsey ER , Friend SH , Trister AD ((2016) ) The mPower study, Parkinson disease mobile data collected using ResearchKit. Sci Data 3: , 160011. |
[13] | Grove R , Harrington C , Mahler A , Beresford I , Maruff P , Lowy M , Nicholls A , Boardley R , Berges A , Nathan P , Horrigan J ((2014) ) A randomized, double-blind, placebo-controlled, 16-week study of the H3 receptor antagonist, GSK239512 as a monotherapy in subjects with mild-to-moderate Alzheimer’s disease. Curr Alzheimer Res 11: , 47–58. |
[14] | Maher-Edwards G , De’Ath J , Barnett C , Lavrov A , Lockhart A ((2015) ) A 24-week study to evaluate the effect of rilapladib on cognition and cerebrospinal fluid biomarkers of Alzheimer’s disease. Alzheimers Dement (N Y) 1: , 131–140. |
[15] | Scheltens P , Hallikainen M , Grimmer T , Duning T , Gouw AA , Teunissen CE , Wink AM , Maruff P , Harrison J , Van Baal CM , Bruins S , Lues I , Prins ND ((2018) ) Safety, tolerability and efficacy of the glutaminyl cyclase inhibitor PQ912 in Alzheimer’s disease: Results of a randomized, double-blind, placebo-controlled phase 2a study. Alzheimer’s Res Ther 10: , 107. |
[16] | Stricker NH , Lundt ES , Alden EC , Albertson SM , Machulda MM , Kremers WK , Knopman DS , Petersen RC , Mielke MM ((2020) ) Longitudinal comparison of in clinic and at home administration of the Cogstate Brief Battery and demonstrated practice effects in the Mayo Clinic Study of Aging. J Prev Alzheimers Dis 7: , 21–28. |
[17] | Falleti MG , Maruff P , Collie A , Darby DG ((2006) ) Practice effects associated with the repeated assessment of cognitive function using the CogState Battery at 10-minute, one week and one month test-retest intervals. J Clin Exp Neuropsychol 28: , 1095–1112. |
[18] | Goldberg TE , Harvey PD , Wesnes KA , Snyder PJ , Schneider LS ((2015) ) Practice effects due to serial cognitive assessment: Implications for preclinical Alzheimer’s disease randomized controlled trials. Alzheimers Dement (Amst) 1: , 103–111. |
[19] | Coons SJ , Gwaltney CJ , Hays RD , Lundy JJ , Sloan JA , Revicki DA , Lenderking WR , Cella D , Basch E ((2009) ) Recommendations on evidence needed to support measurement equivalence between electronic and paper-based Patient-Reported Outcome (PRO) measures: ISPOR ePRO Good Research Practices Task Force Report. Value Health 12: , 419–429. |
[20] | Cromer JA , Harel BT , Yu K , Valadka JS , Brunwin JW , Crawford CD , Mayes LC , Maruff P ((2015) ) comparison of cognitive performance on the Cogstate Brief Battery when taken in-clinic, in-group, and unsupervised. Clin Neuropsychol 29: , 542–558. |
[21] | Albert MS , DeKosky ST , Dickson D , Dubois B , Feldman HH , Fox NC , Gamst A , Holtzman DM , Jagust WJ , Petersen RC , Snyder PJ , Carrillo MC , Thies B , Phelps CH ((2011) ) The diagnosis of mild cognitive impairment due to Alzheimer’s disease: Recommendations from the National Institute on Aging-Alzheimer’s Association workgroups on diagnostic guidelines for Alzheimer’s disease. Alzheimers Dement 7: , 270–279. |