Memory-Related Frontal Brainwaves Predict Transition to Mild Cognitive Impairment in Healthy Older Individuals Five Years Before Diagnosis
Abstract
Background:
Early prognosis of high-risk older adults for amnestic mild cognitive impairment (aMCI), using noninvasive and sensitive neuromarkers, is key for early prevention of Alzheimer’s disease. We have developed individualized measures in electrophysiological brain signals during working memory that distinguish patients with aMCI from age-matched cognitively intact older individuals.
Objective:
Here we test longitudinally the prognosis of the baseline neuromarkers for aMCI risk. We hypothesized that the older individuals diagnosed with incident aMCI already have aMCI-like brain signatures years before diagnosis.
Methods:
Electroencephalogram (EEG) and memory performance were recorded during a working memory task at baseline. The individualized baseline neuromarkers, annual cognitive status, and longitudinal changes in memory recall scores up to 10 years were analyzed.
Results:
Seven of the 19 cognitively normal older adults were diagnosed with incident aMCI for a median 5.2 years later. The seven converters’ frontal brainwaves were statistically identical to those patients with diagnosed aMCI (n = 14) at baseline. Importantly, the converters’ baseline memory-related brainwaves (reduced mean frontal responses to memory targets) were significantly different from those who remained normal. Furthermore, differentiation pattern of left frontal memory-related responses (targets versus nontargets) was associated with an increased risk hazard of aMCI (HR = 1.47, 95% CI 1.03, 2.08).
Conclusion:
The memory-related neuromarkers detect MCI-like brain signatures about five years before diagnosis. The individualized frontal neuromarkers index increased MCI risk at baseline. These noninvasive neuromarkers during our Bluegrass memory task have great potential to be used repeatedly for individualized prognosis of MCI risk and progression before clinical diagnosis.
INTRODUCTION
Progression of Alzheimer’s disease (AD) and related dementia (ADRD), which involves synaptic, metabolic, and structural changes during brain aging, slowly lead to loss of cognition. Among the most common early symptoms of dementia are deficits in working memory and executive dysfunction as well as episodic memory deficits [1]. Working memory is the capacity to hold active information in one’s memory for immediate manipulation [2]. Currently, there are no effective disease-modifying treatments for clinical dementia, thus prevention is critical. By detecting amnestic mild cognitive impairment (aMCI), an early stage of dementia, clinical onset can be delayed through modification of lifestyle factors [3] and early interventions. Not all persons ever diagnosed with aMCI will necessarily develop clinical ADRD [4]. Recent results from the SPRINT-MIND trial suggest that intensive systolic blood pressure control in older adults with hypertension may delay progression of MCI to dementia [5].
Substantial progress has been made in detecting early pathological changes in living persons by using AD biomarkers with positron emission tomography (PET) imaging, Aβ in the cerebrospinal fluid (CSF) or plasma [6], and CSF and PET biomarkers for AD diagnosis [7]. However, many cognitively intact older adults perceive the procedures as invasive and expensive, and instead prefer noninvasive and cheap screening tools for assessing their risk of cognitive decline. If there is a risk for cognitive deficits, accessing sophisticated neuroimaging facilities for evaluation of dementia would be required. But in most cases, electrophysiological signals measured by electroencephalography (EEG), are noninvasive, affordable, and more suitable to healthy and cognitively intact older adults. EEG is a tool that measures the summations of neural postsynaptic potentials at the scalp and has been used for several decades. What is new here is applying memory-related potentials (averaged EEG signals during memory retrieval) sensitive to early synaptic changes in the brain to predict cognitive impairment at an individual level. Using a working memory task (modified delayed match-to-sample) during functional neuroimaging [8], we reported that memory-related cortical connectivity changes correlate with increased CSF AD biomarkers Aβ deposits and pTau in the brain. EEG network oscillations of brain activity have also been shown to act as a sensitive tool for evaluating neural dynamics of the mouse brain [9].
Averaged EEG signals, known as event-related potentials (ERPs), associated with cognitive events are a well-studied approach for indexing brain responses associated with memory and cognition. Specifically, ERPs measure synchronized synaptic neural activity associated with a cognitive event (e.g., memory retrieval). The science of using cognitive ERPs as predictive biomarkers for ADRD remains in its infancy; however, findings show that ERP signals during cognitive tasks, either in amplitude or in latency, are altered in patients with MCI and ADRD [10–14].
Previous studies show that memory task-induced responses in a network of brain regions such as the hippocampus, posterior cingulate cortex (PCC), and medial prefrontal cortex [15] are vulnerable to cognitive aging, preclinical AD [8], aMCI, and AD [12]. More importantly, these memory-induced brain responses also correlate with CSF Aβ42 (pg/ml) and pTau181 (pg/ml) in normal older adults [8]. Furthermore, Li et al. (2017) have identified brainwave patterns that are distinguishable among normal controls, aMCI, and AD brains using unified ERP criteria in conjunction with a short-term memory task (Fig. 2A) [12]. Using a delayed match-to-sample task (Fig. 1) in this study, we have reported left-frontal memory-related potentials as a ‘neuromarker’ for aMCI.
Fig.1
The Bluegrass memory paradigm is a modified delayed match-to-sample working memory task lasting approximately 18 minutes for each participant. In each memory trial (16 seconds), a participant was instructed to remember a presented sample target image (for 3 seconds) and then indicate whether each subsequent image (with a jittered delay 1.1–1.4 seconds) was a Match to the sample Target or a Nonmatch (Nontarget) by pressing a corresponding button (A or L key). EEG (64-channel NeuroScan) and memory performance were recorded. A new sample image was used in each trial. Individual images (either target or non-target) were tested two or three times per trial. Note on images: Sample Target = Target = Match; Non-match = Nontarget = Distractor during memory.
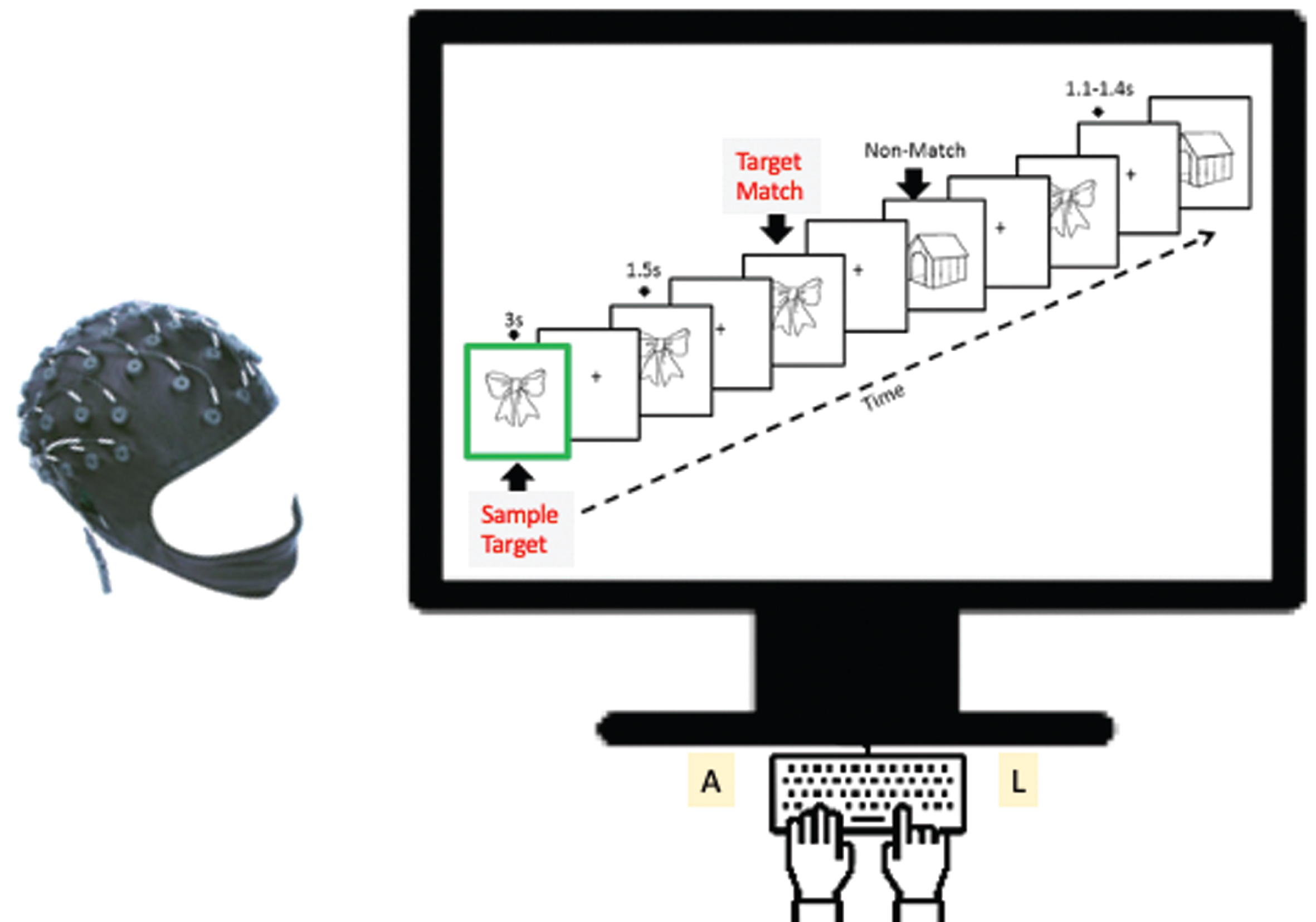
Fig.2
A. F753 is averaged left frontal memory-related potentials of F3, F5, and F7. The blue circle indicates location of left frontal sites from left to right F7, F5, and F3. Baseline memory-related potentials and topographical maps in Normal Cognition (NC) and patients with MCI groups (adapted from [12]). The yellow highlight indicates the differences (diff) of memory-related potentials (Target Match – Nontargets Nonmatch).
![A. F753 is averaged left frontal memory-related potentials of F3, F5, and F7. The blue circle indicates location of left frontal sites from left to right F7, F5, and F3. Baseline memory-related potentials and topographical maps in Normal Cognition (NC) and patients with MCI groups (adapted from [12]). The yellow highlight indicates the differences (diff) of memory-related potentials (Target Match – Nontargets Nonmatch).](https://content.iospress.com:443/media/jad/2021/79-2/jad-79-2-jad200931/jad-79-jad200931-g002.jpg)
Given these findings, the goal of the present study was to test whether individuals’ frontal brainwaves during a visual object working memory task could predict future cognitive status, i.e., incident diagnoses of aMCI. Our central hypothesis was that individuals who converted to MCI years later would have MCI-like memory-related ERP signatures at baseline compared to individuals who remained cognitively intact. We tested three likely predictors of cognitive impairment: 1) Reduced frontal responses to retrieval of memory-target (Target Match); 2) Enhanced frontal signals of distractors, i.e., memory-nontargets (Nontarget; Nonmatch), which reflect decline in frontal ability to filter task-irrelevant items; and 3) Cognitive impairment due to reduced differentiation of neural mechanisms associated with memory targets and distractors (i.e., differences of memory-related brainwaves).
MATERIALS AND METHODS
Participants and evaluations
Thirty-four community-dwelling older adults were recruited from the University of Kentucky Alzheimer’s Disease Center (UK-ADC) research volunteer cohort. Annual neuropsychological and clinical diagnostic procedures at the UK-ADC have been described elsewhere [17]. Each year, an older individual received several memory-related neuropsychological tests including the California Verbal Learning Test (CVLT) [18]. To note, aMCI is considered MCI due to AD pathologies and was assigned in accordance with the national standards [19]. Participants were evaluated with an annual detailed cognitive and clinical assessment by UK-ADC personnel, followed by a multidisciplinary consensus conference to determine their clinical diagnosis: 1) remains normal, no change; 2) conversion to MCI diagnosis by the examining physician is based on criteria of history and Clinical Dementia Rating Scale (CDR) score. In cases where memory was the dominant component of cognitive decline, the objective decline in memory test score 1.5 standard deviation below cutoff was subclassified as amnestic type MCI (aMCI); (3) criteria-based diagnosis of Alzheimer’s disease (AD) or other dementia [19].
In addition to the annual neuropsychological testing and clinical assessments at the UK-ADC, at baseline each participant underwent an EEG recording during the memory task in the Aging, Brain, and Cognition Laboratory in the Department of Behavioral Science, UK College of Medicine on a separate day [12]. Note that these cognitively intact normal older adults did not receive a diagnosis of Alzheimer’s neuropathology or disease based on standard CSF or PET biomarkers at baseline. One older individual who was MCI at age 67 at baseline did not show AD pathology in their autopsy at death at the age of 77. We have removed this individual from the aMCI group in this analysis.
All research activity was approved by the University of Kentucky Institutional Review Board and all participants provided written informed consent.
The task and memory-related potentials
The Bluegrass working memory task (Fig. 1) is a modified delayed match-to-sample task. For each memory trial, a participant holds the sample target image in his/her working memory and indicates whether each subsequent image is the sample target (Target match or Non-match) by pressing a button. EEG and memory performance, i.e., accuracy (%) and reaction times (milliseconds) were recorded during the visual working memory task. The Snodgrass and Vanderwart images are presented using E-prime (Psychology Software Tools, US).
EEG recording and EEG preprocessing
Scalp electrical signals were recorded using a 64-channel NeuroScan cap while the participants were engaged in a modified delayed match-to-sample task. These electrodes were referenced to a midline electrode during recording and re-referenced to the average of the right and left mastoid potentials offline. Two additional channels were used for monitoring horizontal and vertical eye movements. Impedance was maintained below 5 kΩ. EEG data were filtered using a band-pass of 0.05–40 Hz and sampled at a rate of 500 Hz. Each averaging epoch lasted 1,100 milliseconds, including 100 milliseconds prior to stimulus onset.
A regression algorithm implemented with NeuroScan software (Compumedics, Australia) was used to reduce the influence of eye blink artifact on the EEG recording. More details of EEG recording and analysis have been previously described [12]. Of the 34 participants who underwent EEG, one did not have data available for the memory task and was excluded, leaving 33 participants in the current study. Here we examined the predictivity of three different left frontal sites of F7, F5, and F3, where significant brainwave differences between CN and MCI patients were reported during the working memory task [12].
Statistical analysis
For participants with initially intact cognition, we assessed the association between ERPs and measures of both short-delayed recall and long-delayed recall. We used Pearson correlations based on the CVLT-II [18] standardized scores (i.e., age, sex, and education specific Z scores) for short- and long-delayed recall obtained at the time of the EEG and at last follow-up. Additionally, we used proportional hazards regression, with age as the time scale and no additional covariates, to estimate hazards of aMCI. Participants who were not diagnosed with incident aMCI were censored at their last UK-ADC assessment.
While 0.05 was used as the nominal significance level, we interpreted our results cautiously due to the small sample and number of comparisons made. Our primary focus was the pattern of results. All statistical analyses were performed in SAS 9.4®.
Analysis of covariance (ANCOVA) was used to assess mean group event-related potentials (ERPs) between groups using Prism 7 (http://www.graphpad.com). Adjustment covariates were age at the EEG assessment and years of education. Additionally, memory-related potentials were further tested using a nonparametric test (The Kruskal-Wallis test) using SPSS Statistics 26.
RESULTS
At baseline EEG, 14 carried a diagnosis of aMCI and 19 had normal cognition (NC) at the EEG (Table 1). After a median follow-up of 5.2 years (range 2.2–7.9 years), 7 out of 19 baseline NC had been diagnosed with incident aMCI.
Table 1
University of Kentucky Alzheimer’s Disease Center Participants Characteristics at Baseline
Normal Cognition (n = 12) | Incident MCI (n = 7) | Baseline MCI (n = 14) | |
Age (y) | 74.6±4.7 | 76.9±4.7 | 76.4±9.9 |
Sex (n, % female) | 8 (66.7) | 5 (71.4) | 4 (28.6) |
Education (y) | 16.9±2.6 | 16.0±3.1 | 16.8±2.1 |
MMSE (max = 30) | 29.4±0.7 | 28.6±1.0 | 27.6±2.3 |
CVLT-II Short Delay Free Recall (max = 16) | 11.8±3.4 | 7.7±3.9 | 7.3±2.7 |
CVLT-II Long Delay Free Recall (max = 16) | 12.7±2.6 | 8.0±3.5 | 6.3±4.1 |
MCI, mild cognitive impairment; MMSE, Mini-Mental State Examination; max, maximum; CVLT-II, California Verbal Learning Test-II.
We rechecked each incident aMCI individual’s CVLT and diagnosis data at baseline when they were normal. Despite their low scores on the CVLT recall tests, these 7 participants received a consensus diagnosis of normal cognition at the time of the testing and for multiple years afterward (e.g., approx. 8 years, 7 years, 4 years, 3 years, 4 years, 9 years, and 4 years). These CVLT scores indicate that they already had memory-related neural impairment, but overt clinical symptoms did not appear until years later.
Left frontal memory-related indicators as predictors
We previously reported a frontal memory-related brainwave signature: enhanced left frontal positive-going responses were associated with non-matching relative to matching targets during working memory in aMCI, but not in normal cognition [12]. Here, we examined each individual’s characteristics associated with multiple measures of frontal ERP (F7, F5, and F3; Fig. 2A), as well as a combined averaged left-frontal measure, F753 (averaged signals of three left frontal sites), of differentiation (Target match - Non match). Based on our previous work [12], ANOVA revealed significant adjusted mean differences in cognitive ERP between those who had aMCI at baseline and those who remained normal (Table 2).
Table 2
Mean (±SEM) Adjusted Left Frontal Memory-Related Potentials
Left Frontal Sites Predictor | Normal Cognition (n = 12) | Incident MCI (n = 7) | Baseline MCI (n = 14) |
F7_target | 5.27±0.81 | 2.74±1.06 | 1.46±0.77* |
F5_target | 5.67±0.88 | 3.42±1.16 | 1.99±0.84* |
F3_target | 6.25±0.95 | 4.19±1.25 | 2.62±0.91* |
F7_nontarget | 4.68±0.92 | 4.50±1.21 | 3.68±0.88 |
F5_nontarget | 5.01±0.95 | 5.32±1.24 | 3.87±0.90 |
F3_nontarget | 5.51±0.98 | 6.10±0.93 | 4.04±0.93 |
F753_diff | 0.66±0.58 | -1.86±0.75* | -1.84±0.56* |
F7_diff | 0.59±0.63 | -1.75±0.83* | -2.22±0.60* |
F5_diff | 0.65±0.60 | -1.90±0.79* | -1.88±0.57* |
F3_diff | 0.74±0.59 | -1.91±0.79* | -1.42±0.57* |
*Indicates significantly different from the least squares means (SEM) of Normal Cognition, adjusted for age at assessment and education. The_diff variables are created index diff = target – nontarget; F753 is not direct measurement but averaged signals from three left frontal sites, i.e., F7, F5, and F3 (see Fig. 2A).
In searching for effective neuromarkers predictors, we compared unadjusted multiple left frontal memory-related indicators (Fig. 2A) by diagnosis groups which are presented in Fig. 2B.
Fig.2
B. Distribution of memory-related potentials by groups: Normal cognition, incident MCI who were normal at baseline, and baseline MCI. Symbols (e.g.,+) are outlier individuals. The right most panel is not direct measures but combined indicators: F753 = mean of measures of F7, F5, and F3; diff = Target match – nonmatch, the more positive value of the diff, the more similar to younger and healthy direction. The negative diff values are MCI-like. The middle group (red) were normal at baseline, but diff values are MCI-like already.
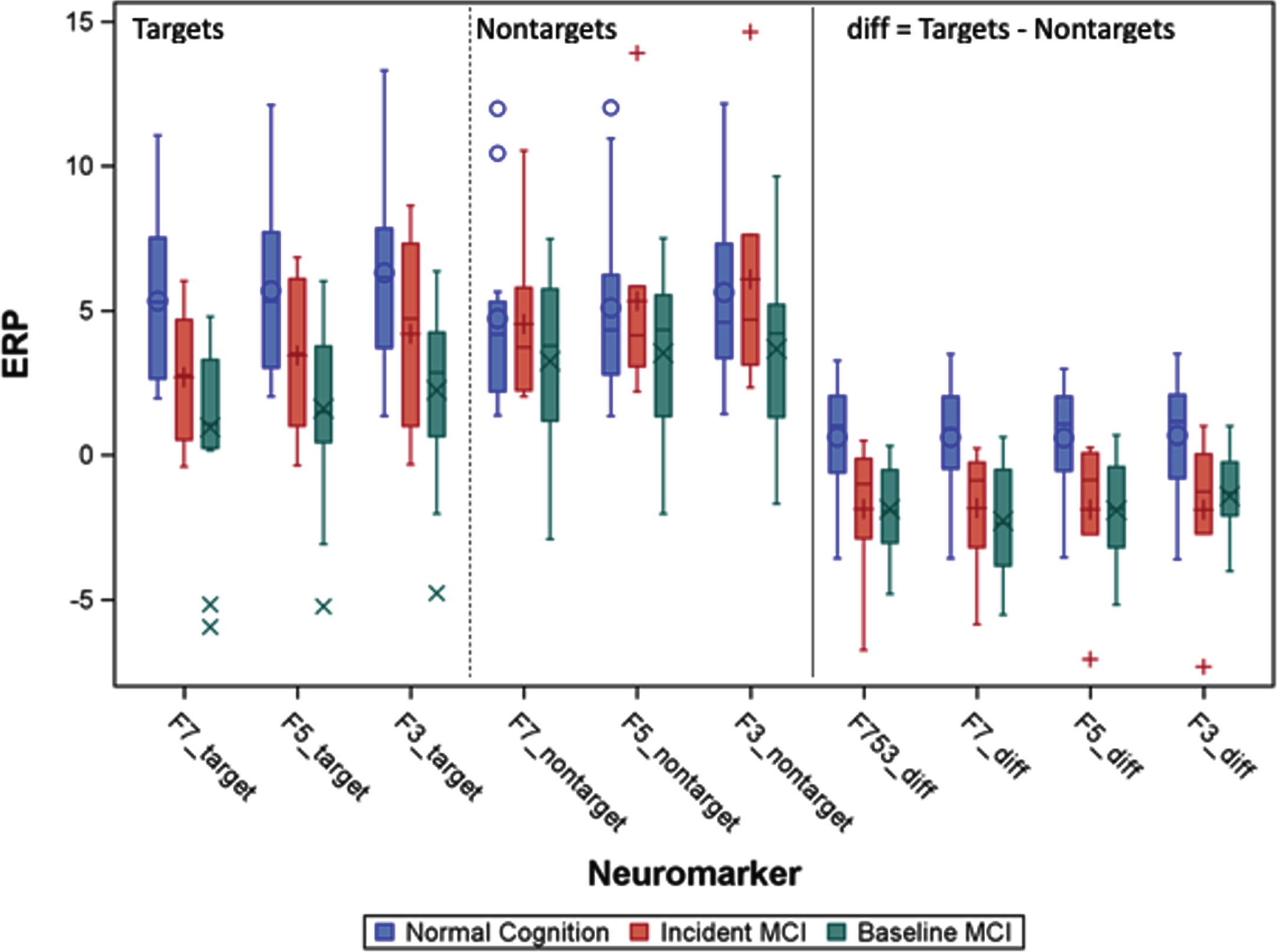
In addition to significant mean differences between those who remained cognitively normal versus those who developed aMCI, we also observed that memory-target responses differed. Differentiation of memory-related brainwaves produced remarkably consistent hazard ratios (HR) for incident MCI (Table 3; HR range 0.58–0.77), with significant associations for F753_diff, F7_diff, and F3_diff. Also striking was the consistent estimate of HRs around 1.00 for non-target ERPs.
Table 3
Pearson Correlation of Left Frontal Memory-related Neuromarkers and CVLT-II Delayed Recall at Baseline and Last Follow-Up (mean follow-up interval = 8.1 years)
Left Frontal Predictor | At Baseline Follow-Up | At Last | ||
Short-delay Recall | Long-delay Recall | Short-delay Recall | Long-delay Recall | |
F7_target | 0.56 (0.01) | 0.43 (0.07) | 0.44 (0.10) | 0.64 (0.01) |
F5_target | 0.64 (0.003) | 0.51 (0.03) | 0.43 (0.11) | 0.62 (0.01) |
F3_target | 0.61 (0.0005) | 0.43 (0.07) | 0.33 (0.23) | 0.57 (0.03) |
F7_nontarget | 0.27 (0.27) | 0.16 (0.33) | 0.19 (0.50) | 0.25 (0.37) |
F5_nontarget | 0.33 (0.17) | 0.24 (0.33) | 0.23 (0.42) | 0.30 (0.29) |
F3_nontarget | 0.33 (0.17) | 0.18 (0.47) | 0.17 (0.54) | 0.30 (0.28) |
F753_diff | 0.39 (0.16) | 0.31 (0.20) | 0.33 (0.23) | 0.53 (0.04) |
F7_diff | 0.39 (0.16) | 0.32 (0.19) | 0.36 (0.19) | 0.55 (0.03) |
F5_diff | 0.33 (0.17) | 0.29 (0.23) | 0.34 (0.22) | 0.54 (0.04) |
F3_diff | 0.34 (0.15) | 0.32 (0.18) | 0.29 (0.29) | 0.49 (0.06) |
All participants included in this analysis were cognitively intact at the time of the baseline EEG (N = 19). Results presented are r (p value). Values in Bold are significant. The_diff variable are created index diff = target – nontarget; F753 is averaged signals of three left frontal sites, i.e., F7, F5, and F3 (see Fig. 2A).
Frontal memory-related potentials were weakly to strongly correlated with both CVLT-II short- and long-delayed recall standardized scores obtained proximal to the EEG. Scores were significantly associated with multiple measures of Diff and Target, but not significantly associated with Nontarget (Table 3). Interestingly, ERPs were more strongly associated with long-delayed recall at the last follow-up than at the EEG for all measures (Table 3), with significant associations with Target and Diff, but not Nontarget. No ERP measures were significantly associated with short-delay recall at last follow-up, and all correlations were attenuated relative to the CVLT-II proximal to the EEG.
Individuals’ left frontal memory-related potentials
We further tested differences between memory-related potentials using the two frontal indicators (F753, and F5) in three types of individuals (Fig. 3). One-way ANOVA showed that the three types of individuals were different [F753, F(2, 32) = 6.802, p < 0.005; F5 F(2, 32) = 5.890, p < 0.01]. The planed comparisons between MCI converters versus those who remained normal were the most interesting.
Fig.3
The cognitively older healthy individuals at baseline (pink) who showed the MCI-like brainwaves at baseline converted to aMCI after 5.2 years. Baseline negative value signals risk for MCI in a normal older adult. A. Left frontal difference waves of memory target and nontargets (F753) are statistically significant between individual converters from non-converters (remain cognitively normal after averaged 10 years). Yet, similar memory-related ERP indexes between converters and baseline MCIs. Baseline negative value signals risk for MCI in a normal older adult. B. The left frontal (F5 site) responses to retrieval of memory-target in three types of individuals.
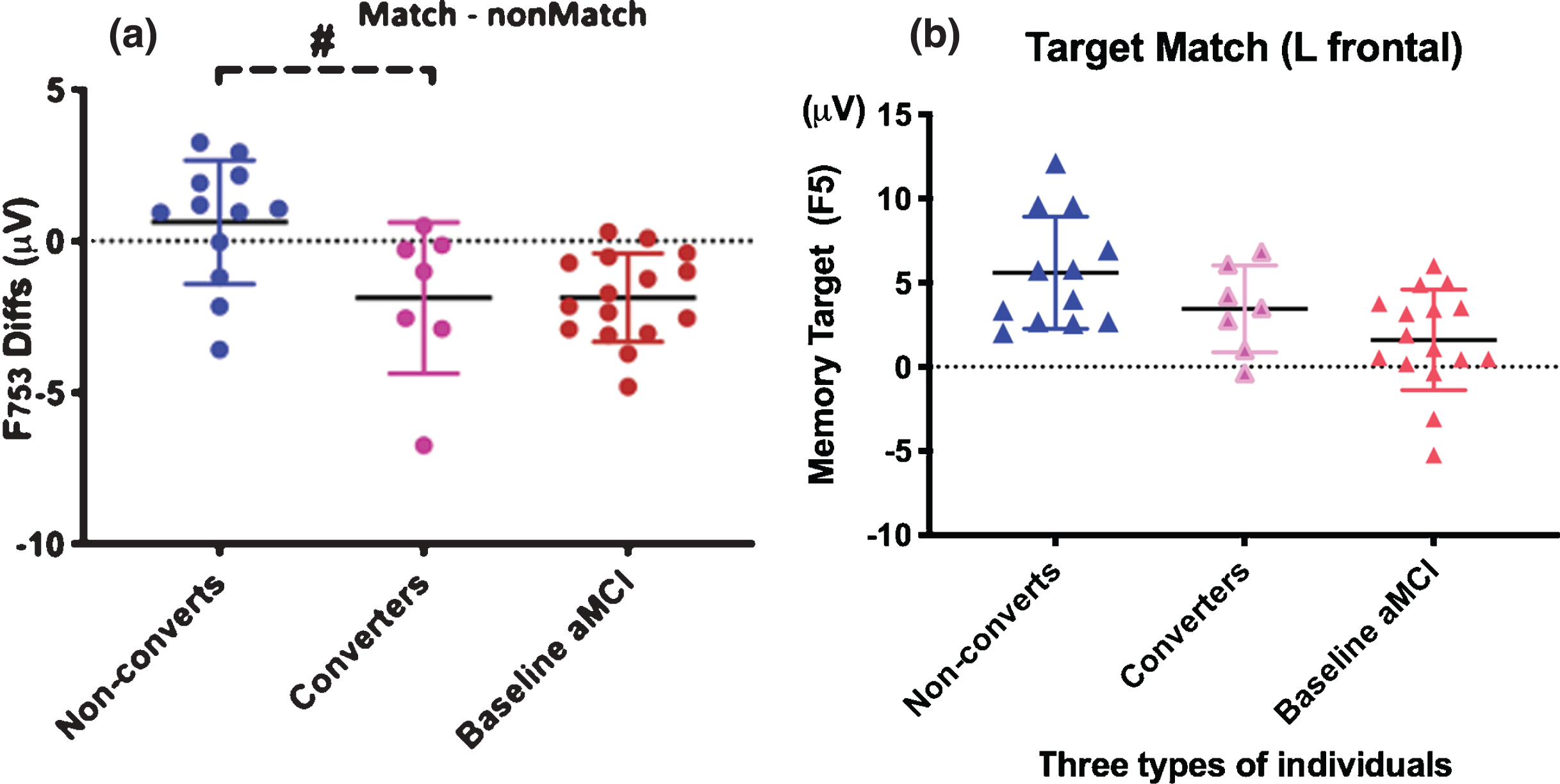
We found significant differences between converters’ and non-converters’ ERPs at baseline (Fig. 3A). The F753 difference waveforms of memory negative value indicates MCI-like high risk pattern (reduced target-related frontal responses). Older individuals who later converted to MCI showed MCI-like patterns at baseline compared to those who remained normal (F753 diff, p < 0.01; F5 p < 0.01).
The incident MCI converters’ patterns were not significantly different from those seen in individuals with MCI at baseline (p > 0.10), which means that the cognitively normal individuals who converted to MCI later already had the brainwave patterns similar to those of MCI patients at baseline. Interestingly, the F753 diff values, of almost all individuals with MCI at baseline and later MCI converters, were negative (below zero dash line in Fig. 3A).
Nonparametric analysis
Given the small sample of the converters, we further validate the results using nonparametric analysis. The Kruskal-Wallis test, a nonparametric (distribution free) test was conducted that provided evidence of a significant difference (p < 0.05) in at least one pair of groups in F753. Dunn’s pairwise tests were used for analyzing the three pairs of groups. There was a significant difference (p = 0.004, adjusted using the Bonferroni correction) between the baseline aMCI group and non-converters. There was no significant difference (adjusted p = 1.0) between the baseline aMCI group and converters. The difference between non-converts and converters did not reach the significance level (adjusted p = 0.102).
The same tests were used to analyze data in F5_Target, which showed similar results. A significant difference (p < 0.05) was found between baseline aMCI group and non-converts (adjusted p = 0.008), whereas no significant difference was found in the other two pairs of groups.
Hazard ratio analysis on risk of MCI
We also observed that all ERP measures of memory-target response (Target) and differentiation of memory-related brainwaves (Diff) produced hazard ratios (HR) for incident aMCI in the range 0.58–0.77 (Table 4), with statistically significant associations for F753_diff, F7_diff, and F3_diff. Also striking was the consistent estimate of HRs around 1.00 for nontarget ERPs (Nontarget). Thus, the best predictors for aMCI risk are the left frontal difference brainwaves.
Table 4
Left Frontal Cox Proportional Hazards Model Results for Hazard of MCI (Age as Timescale)
Left Frontal Predictor | 1-unit Increase in Predictor | 1-unit Decrease in Predictor |
F7_target | 0.65 (0.42, 1.01) | 1.54 (0.99, 2.38) |
F5_target | 0.58 (0.33, 1.01) | 1.72 (0.99, 3.03) |
F3_target | 0.67 (0.44, 1.01) | 1.49 (0.99, 2.27) |
F7_nontarget | 1.02 (0.80, 1.31) | 0.98 (0.76, 1.25) |
F5_nontarget | 1.04 (0.83, 1.29) | 0.96 (0.78, 1.20) |
F3_nontarget | 1.03 (0.82, 1.28) | 0.97 (0.78, 1.22) |
F753_diff | 0.68 (0.48, 0.97) | 1.47 (1.03, 2.08) |
F7_diff | 0.65 (0.45, 0.95) | 1.54 (1.05, 2.22) |
F5_diff | 0.77 (0.57, 1.05) | 1.30 (0.95, 1.75) |
F3_diff | 0.70 (0.50, 0.98) | 1.43 (1.02, 2.00) |
Results are Hazard Ratio with 95% Confidence Interval. Bolded results are statistically significant at the 0.05 level. The_diff variable are created index diff = target – nontarget; F753 is averaged signals of three left frontal sites, i.e., F7, F5, and F3.
DISCUSSION
Summary of key results
We previously reported that, at the baseline EEG assessment, the group means of left frontal visual memory-related potentials were different between participants with normal cognition and those with aMCI in carefully evaluated older adults [12]. We now show that cognitive neuromarkers among cognitively normal individuals who converted to aMCI during follow-up showed significantly different patterns of left frontal memory-related potentials compared to those who remained normal. In addition, we report that reduced left frontal memory-target related signals at baseline predicts later conversion to aMCI.
Our results indicate that brainwaves obtained during a specific working memory retrieval demonstrate an aMCI-like pattern in some cognitively normal older adults at an average of five years before aMCI conversion. These noninvasive and easy EEG neuromarkers allow repeated measures over time and may prove to be a useful prognostic measure of aMCI risk. Additionally, wireless, wearable, noninvasive, and cloud-based EEG measurements hold great promise in community clinics and future telehealth applications.
Our results support the central hypothesis that the aMCI converters’ memory-related brainwave patterns are similar to patients with aMCI even at the pre-clinical stage. This indicates that alteration of brainwaves underserving memory is present before clinical symptoms or definitive changes in cognitive tests. Since the memory-related neuromarkers were brain signals detected at the left frontal electrode sites, frontal responses at baseline in normal aged persons may be a “canary in the coal mine” indicating the presence of underlying changes affecting a particularly sensitive dynamic memory network. Future memory declines in these individuals would represent further degradation of other brain networks by advancing pathology. Among the three measures from three locations, the prediction using differences of memory targets vs nontargets separated individuals who remained cognitively intact versus those who become incidental MCIs or MCI at baseline (Fig. 2B). The frontal memory target-related potentials alone also showed some degrees of differences between converters and nonconverters.
The current results indicate that individualized neuromarkers based on brainwave patterns at pre-aMCI are predictive of aMCI conversion about 5 years later. The question remains, what exactly do these the memory-related neuromarkers measure?
Predicting MCI and risk for dementia using electrophysiological signals (reflecting synaptic functions) in the brain have been gaining momentum in recent decade [16]. The left frontal EEG during the working memory task is likely brain activity from known working memory network, which is also seen in the left frontal regions during the same task using functional magnetic resonance imaging (fMRI) [8, 20]. This memory-related brainwave pattern is possibly localized at left frontal BA 46 and BA 10, the classic working memory and executive control regions of the brain [21]. Interestingly, using language-based memory tasks, the frontal regions also show discriminative patterns between NC and MCI [10, 12–14]. Prediction of AD using the current left frontal memory-related markers is consistent with a previously reported successful prediction using an incidental repetition learning paradigm [14]. Seven elderly persons with normal cognition at the time of EEG recordings who showed subsequent cognitive decline or AD pathology at autopsy (n = 5, Braak stage = 2.8), were compared to 13 normal elderly who remained cognitively normal after ten years. The P600 congruous word repetition ERP effects (New minus Old congruous words) were significantly larger than those in Pre-AD. The prediction rate was 84% in identifying conversion to MCI or AD [14]. One difference between our visual memory task and others is that memory-related potential measures memory retrieval of explicit memory target and nontarget, while the repetition priming used by Olichney’s group measures a more implicit memory process.
Nature of the left frontal visual memory-related neuromarkers
The modified version of the delayed match-to-sample task has been used in monkey physiology and human neuroimaging studies on working memory. Our previous study has linked brain activity measured by functional MRI during this memory paradigm to distinct cortical regions known to be vulnerable to cognitive aging and impairment caused by preclinical AD [8, 22]. We reported that the left prefrontal memory-related potentials during the task are significantly different between NC, aMCI, and AD groups [12].
Here we tested three hypotheses on how the left frontal memory-related brainwaves predict future cognitive impairment: 1) Reduced memory-target (Target) related frontal responses; 2) Enhanced frontal signals of distractors (i.e. nontarget / nonmatch), which reflect decline in frontal ability to filter task-irrelevant items and correlate with faster memory decline; and 3) Cognitive impairment due to changes in both neural mechanisms reducing differentiation of memory-related brainwaves (diff = Target - Nontarget).
Our results revealed that the different brainwaves between memory Target and Nontargets in two left frontal electrodes (F7 or F3 in Table 4) or averaged from three left frontal sites (F753) are the best predictor (#3). Reduced brain responses to memory targets (#1) also predicted well. However, it was somewhat surprising that increased distraction (#2) typically seen in cognitive aging did not predict well. Our results suggest that the enhanced prefrontal response to memory targets (match) in the absence of stimuli during a delay period is the neuromarker of good maintenance during working memory. The neural responses reported in noninvasive human neuroimaging studies [19–22] are consistent with those obtained using invasive techniques in animals [24]. During target matches both show enhanced neural responses in the left frontal cortex and, to a lesser degree, in the temporal cortex. Elevated left frontal brain responses have also been reported by groups using fMRI during contextual memory retrieval tasks [25].
Importantly, our visual memory-related left frontal neuromarker is individual-based and noninvasive. Thus, the neuromarker offers great promise to become a dynamic indicator for aMCI that can be repeatedly used even within a short time period before explicit changes in memory performance or structural brain changes are seen. In addition, it is also a useful test that can be used across culture and languages.
The previous work with participants from UK-ADC longitudinal aging cohort identified increased loss of projections from the entorhinal cortex to the frontal and parietal regions with progression of AD [8]. This is important because aMCI is often a precursor to dementia [23, 27], and expresses different neural network function during resting-state [28], and during memory task [12] in our preclinical aging cohort. These neural afferents have been described as helping maintain working memory through short-term synaptic facilitation in animal models [29]. The Bluegrass memory task activates an enlarging network of brain regions as task difficulty increases. Greater network activation represents cognitive compensation related to the need to hold memory target online during working memory retrieval. The current report on altered brainwave patterns in normal persons who later develop MCI is consistent with other reports of EEG brain network changes in AD [30].
In summary, we show that a modified delayed match-to-sample task produces neuromarkers in normal persons that predict MCI up to 10 years before the conversion. A limitation of the current study is that the relatively small sample size does not allow a precise estimation of the associations we described. In addition, the older participants were selected in a non-random manner from the available population (normal subjects were simply asked if they would like to participate in this study as they appeared for their annual UK-ADC assessment). Thus, the participants were taken from a pool of research volunteers that are generally healthy and highly educated, which is not representative of the general US population. Nonetheless, this study provides a foundation for future validation in a large-scale longitudinal study.
To meet the urgent need for developing new non-invasive and affordable biomarkers to predict aMCI at the preclinical stage, we demonstrated that neuromarkers from the Bluegrass memory paradigm are more sensitive to neural and cognitive changes than conventional neuropsychological tests. With their large estimated effect size, our testing results suggest that individualized memory-related brainwave patterns in normal older adults predict aMCI an average of five years before conversion. This is significant because aMCI is strongly associated with the later development of AD. The noninvasive neuromarkers have great potential to be used repeatedly and longitudinally as an individualized risk assessment for prediction of MCI before clinical diagnosis.
Conclusions
Until now, non-invasive ‘neuromarkers’ predicting and monitoring risk for MCI have been lacking. EEG rhythms during resting-state and sleep are used as neuromarkers for clinical trials in AD. We followed the normal older adults over an average of eight years; here we report that memory-related potentials at baseline could serve as a useful marker to predict MCI about five years before MCI diagnosis. We reveal longitudinal validation that left frontal electrophysiological measures during working memory are effective in identifying MCI risk in cognitively normal older adults, which is consistent with several decades of neural evidence on monkey single-cell physiology acquired through invasive microelectrodes, and functional neuroimaging methods. Our findings provide new evidence that EEG during working memory shows great promise as a non-invasive and reliable predictor of cognitive decline.
AVAILABILITY OF DATA AND MATERIALS
The datasets of the present study are available from the corresponding author on request.
ACKNOWLEDGMENTS
We thank the volunteer participants of the UK-ADC, T. Shannon for database assistance, Y. Lin for nonparametric analysis, S. Borhani for Fig. 1 contribution, T. Venkatesan, J. Neal, B. Aghayeeabianeh, and M.L. Kasper for editing assistance. Supported by the United States National Institute of Health R56AG060608-01 and P30AG028383, and by Oak Ridge National Laboratory and UT-Battelle LLC for the US Department of Energy DE-AC05-00OR22725.
Authors’ disclosures available online (https://www.j-alz.com/manuscript-disclosures/20-0931r1).
REFERENCES
[1] | Kirova A-M , Bays RB , Lagalwar S ((2015) ) Working memory and executive function decline across normal aging, mild cognitive impairment, and Alzheimer’s disease. Biomed Res Int 2015: , 748212. |
[2] | Kumar S , Zomorrodi R , Ghazala Z , Goodman MS , Blumberger DM , Cheam A , Fischer C , Daskalakis ZJ , Mulsant BH , Pollock BG , Rajji TK ((2017) ) Extent of dorsolateral prefrontal cortex plasticity and its association with working memory in patients with Alzheimer disease. JAMA Psychiatry 74: , 1266–1274. |
[3] | Cummings JL , Isaacson RS , Schmitt FA , Velting DM ((2015) ) A practical algorithm for managing Alzheimer’s disease: What, when, and why? Ann Clin Transl Neurol 2: , 307–323. |
[4] | Abner EL , Kryscio RJ , Cooper GE , Fardo DW , Jicha GA , Mendiondo MS , Nelson PT , Smith CD , Van Eldik LJ , Wan L , Schmitt FA ((2012) ) Mild cognitive impairment: Statistical models of transition using longitudinal clinical data. Int J Alzheimers Dis 2012: , 1–9. |
[5] | SPRINT MIND Investigators for the SPRINT Research Group, Williamson JD , Pajewski NM , Auchus AP , Bryan RN , Chelune G , Cheung AK , Cleveland ML , Coker LH , Crowe MG , Cushman WC , Cutler JA , Davatzikos C , Desiderio L , Erus G , Fine LJ , Gaussoin SA , Harris D , Hsieh MK , Johnson KC , Kimmel PL , Tamura MK , Launer LJ , Lerner AJ , Lewis CE , Martindale-Adams J , Moy CS , Nasrallah IM , Nichols LO , Oparil S , Ogrocki PK , Rahman M , Rapp SR , Reboussin DM , Rocco MV , Sachs BC , Sink KM , Still CH , Supiano MA , Snyder JK , Wadley VG , Walker J , Weiner DE , Whelton PK , Wilson VM , Woolard N , Wright JT Jr , Wright CB ((2019) ) Effect of intensive vs standard blood pressure control on probable dementia. JAMA 321: , 553–561. |
[6] | Abner EL , Jicha GA , Shaw LM , Trojanowski JQ , Goetzl EJ ((2016) ) Plasma neuronal exosomal levels of Alzheimer’s disease biomarkers in normal aging. Ann Clin Transl Neurol 3: , 399–403. |
[7] | Mattsson N , Insel PS , Donohue M , Landau S , Jagust WJ , Shaw LM , Trojanowski JQ , Zetterberg H , Blennow K , Weiner W ; Alzheimer’s Disease Neuroimaging Initiative ((2015) ) Independent information form cerebrospinal fluid amyloid-β and flobetapir imaging in Alzheimer’s disease. Brain 138: (Pt 3), 772–783. |
[8] | Jiang Y , Huang H , Abner E , Broster LS , Jicha GA , Schmitt FA , Kryscio R , Andersen A , Powell D , Van Eldik L , Gold BT , Nelson PT , Smith C , Ding M ((2016) ) Alzheimer’s biomarkers are correlated with brain connectivity in older adults differentially during resting and task states. Front Aging Neurosci 8: , 15. |
[9] | Bero AW , Bauer AQ , Stewart FR , White BR , Cirrito JR , Raichle ME , Culver JP , Holtzman DM ((2012) ) Bidirectional relationship between functional connectivity and amyloid- deposition in mouse brain. J Neurosci 32: , 4334–4340. |
[10] | Chapman RM , McCrary JW , Gardner MN , Sandoval TC , Guillily MD , Reilly LA , DeGrush E ((2011) ) Brain ERP components predict which individuals progress to Alzheimer’s disease and which do not. Neurobiol Aging 32: , 1742–1755. |
[11] | Chapman RM , Gardner MN , Klorman R , Mapstone M , Porsteinsson AP , Antonsdottir IM , Kamalyan L ((2018) ) Temporospatial components of brain ERPs as biomarkers for Alzheimer’s disease. Alzheimers Dement (Amst) 10: , 604–614. |
[12] | Li J , Broster LS , Jicha GA , Munro NB , Schmitt FA , Abner E , Kryscio R , Smith CD , Jiang Y ((2017) ) A cognitive electrophysiological signature differentiates amnestic mild cognitive impairment from normal aging. Alzheimers Res Ther 9: , 3. |
[13] | Olichney JM , Yang JC , Taylor J , Kutas M ((2011) ) Cognitive event-related potentials: Biomarkers of synaptic dysfunction across the stages of Alzheimer’s disease. J Alzheimers Dis 26: , 215–228. |
[14] | Olichney JM , Pak J , Salmon DP , Yang J , Gahagan T , Nowacki R , Hansen L , Galasko D , Kutas M , Iragui-Madoz VJ ((2013) ) Abnormal P600 word repetition effect in elderly persons with preclinical Alzheimer’s disease. Cogn Neurosci 4: , 143–151. |
[15] | Fell J , Axmacher N ((2011) ) The role of phase synchronization in memory processes. Nat Rev Neurosci 12: , 105–118. |
[16] | Jackson CE , Snyder PJ ((2008) ) Electroencephalography and event-related potentials as biomarkers of mild cognitive impairment and mild Alzheimer’s disease. Alzheimers Dement 4: , S137–S143. |
[17] | Schmitt FA , Nelson PT , Abner E , Scheff S , Jicha GA , Smith C , Cooper G , Mendiondo M , Danner DD , Van Eldik LJ , Caban-Holt A , Lovell MA , Kryscio RJ ((2012) ) University of Kentucky Sanders-Brown healthy brain aging volunteers: Donor characteristics, procedures and neuropathology. Curr Alzheimer Res 9: , 724–733. |
[18] | Dean DC , Kramer JH , Kaplan E , Ober BA ((2000) ) California Verbal Learning Test: Second Edition. Pearson. https://www.pearsonassessments.com/store/usassessments/en/Store/Professional-Assessments/Cognition-%26-Neuro/California-Verbal-Learning-Test-%7C-Second-Edition/p/100000166html |
[19] | Albert MS , DeKosky ST , Dickson D , Dubois B , Feldman HH , Fox NC , Gamst A , Holtzman DM , Jagust WJ , Petersen RC , Snyder PJ , Carrillo MC , Thies B , Phelps CH ((2011) ) The diagnosis of mild cognitive impairment due to Alzheimer’s disease: Recommendations from the National Institute on Aging-Alzheimer’s Association workgroups on diagnostic guidelines for Alzheimer’s disease. Alzheimers Dement 7: , 270–279. |
[20] | Jiang Y , Haxby JV , Martin A , Ungerleider LG , Parasuraman R ((2000) ) Complementary neural mechanisms for tracking items in human working memory. Science 287: , 643–646. |
[21] | Guo C , Lawson AL , Jiang Y ((2007) ) Distinct neural mechanisms for repetition effects of visual objects. Neuroscience 149: , 747–759. |
[22] | Yu J , Li R , Jiang Y , Broster LS , Li J ((2016) ) Altered brain activities associated with neural repetition effects in mild cognitive impairment patients. J Alzheimers Dis 53: , 693–704. |
[23] | Parasuraman R , Jiang Y ((2012) ) Individual differences in cognition, affect, and performance: Behavioral, neuroimaging, and molecular genetic approaches. Neuroimage 59: , 70–82. |
[24] | McBride JC , Zhao X , Munro NB , Jicha GA , Schmitt FA , Kryscio RJ , Smith CD , Jiang Y ((2015) ) Sugihara causality analysis of scalp EEG for detection of early Alzheimer’s disease. Neuroimage Clin 7: , 258–265. |
[25] | Miller EK , Desimone R ((1994) ) Parallel neuronal mechanisms for short-term memory. Science 263: , 520–522. |
[26] | Rajah MN , Kromas M , Han JE , Pruessner JC ((2010) ) Group differences in anterior hippocampal volume and in the retrieval of spatial and temporal context memory in healthy young versus older adults. Neuropsychologia 48: , 4020–4030. |
[27] | Jicha GA , Abner E , Schmitt FA , Cooper GE , Stiles N , Hamon R , Carr S , Smith CD , Markesbery WR ((2008) ) Clinical features of mild cognitive impairment differ in the research and tertiary clinic settings. Dement Geriatr Cogn Disord 26: , 187–192. |
[28] | McBride J , Zhao X , Munro N , Smith C , Jicha G , Jiang Y ((2013) ) Resting EEG discrimination of early stage Alzheimer’s disease from normal aging using inter-channel coherence network graphs. Ann Biomed Eng 41: , 1233–1242. |
[29] | Mongillo G , Barak O , Tsodyks M ((2008) ) Synaptic theory of working memory. Science 319: , 1543–1546. |
[30] | Stam C , Jones B , Nolte G , Breakspear M , Scheltens P ((2007) ) Small-world networks and functional connectivity in Alzheimer’s disease. Cereb Cortex 17: , 92–99. |