Data-Driven Modeling of Knowledge Assemblies in Understanding Comorbidity Between Type 2 Diabetes Mellitus and Alzheimer’s Disease
Abstract
Background:
Recent studies have suggested comorbid association between Alzheimer’s disease (AD) and type 2 diabetes mellitus (T2DM) through identification of shared molecular mechanisms. However, the inference is pre-dominantly literature-based and lacks interpretation of pre-disposed genomic variants and transcriptomic measurables.
Objective:
In this study, we aim to identify shared genetic variants and dysregulated genes in AD and T2DM and explore their functional roles in the comorbidity between the diseases.
Methods:
The genetic variants for AD and T2DM were retrieved from GWAS catalog, GWAS central, dbSNP, and DisGeNet and subjected to linkage disequilibrium analysis. Next, shared variants were prioritized using RegulomeDB and Polyphen-2. Afterwards, a knowledge assembly embedding prioritized variants and their corresponding genes was created by mining relevant literature using Biological Expression Language. Finally, coherently perturbed genes from gene expression meta-analysis were mapped to the knowledge assembly to pinpoint biological entities and processes and depict a mechanistic link between AD and T2DM.
Results:
Our analysis identified four genes (i.e., ABCG1, COMT, MMP9, and SOD2) that could have dual roles in both AD and T2DM. Using cartoon representation, we have illustrated a set of causal events surrounding these genes which are associated to biological processes such as oxidative stress, insulin resistance, apoptosis and cognition.
Conclusion:
Our approach of using data as the driving force for unraveling disease etiologies eliminates literature bias and enables identification of novel entities that serve as the bridge between comorbid conditions.
INTRODUCTION
In recent years, comorbidities are inspected with a different perspective. The new route in understanding possible comorbidities has changed from classical approaches that use magnitude, severity, patterns, and burden to comparing disease associated events, pathways, and maps [1, 2]. By establishing comorbid associations from assessment scores of these aspects, classical approaches fail to explain the biology underlying diseases. Hence, biological entities such as genes, proteins, and miRNAs and their involvement in biological processes and pathways have been studied to unravel insights about comorbidity.
The possible association between type 2 diabetes mellitus (T2DM) and Alzheimer’s disease (AD) has enticed significant interest from the scientific community since the identification of typical events of T2DM in AD and vice-versa. For instance, the brains of AD patients are reported to exhibit T2DM-related mechanisms including impaired insulin signaling, insulin resistance, and impaired glucose metabolism [3]. Moreover, hyperphosphorylated microtubule associated protein tau (MAPT) leading to formation of neurofibrillary tangles (NFTs), one of the hallmarks of AD, is a consequence of abnormal glycogen synthase kinase 3 beta activity in the insulin signaling pathway [4]. On the other hand, the presence of abnormally processed islet amyloid polypeptide in pancreas of T2DM patients mimics amyloid-β protein precursor (AβPP)-derived deleterious amyloid-β (Aβ) in AD brains [5]. In addition to these, the comorbid link between T2DM and AD has been established through several studies reporting shared biological processes such as oxidative stress, mitochondrial dysfunction, inflammation, and advanced glycation end products [6].
While most of the speculations are based on individual experiments, studies, or review articles, the putative mechanisms explaining the comorbidity are still unknown. To address this issue, disease-specific knowledge assemblies are created by systematic retrieval of biological information from literature and compared for identifying shared pathophysiological mechanisms. In this regard, Kodamullil et al. (2015) have undertaken a systems biology approach to create cause-and-effect models and proposed single nucleotide polymorphism (SNP)-based mechanisms as the link between the diseases. This is one of the first and few studies that mechanistically depicts and compares disease etiologies [7]. A broader scenario representing mechanistic crosstalk between several pathways such as insulin signaling, neurotrophin signaling, inflammatory regulators, and MTOR signaling in AD and T2DM was demonstrated in our previous work [8]. Interestingly, we have also suggested that metformin, an FDA approved T2DM drug, could be one of the risk factors for developing AD in old age of the diabetic patients. Through this study, the consideration of metformin in drug repositioning in AD has been questioned by depicting the role of metformin in contributing to augment characteristic features of AD such as neuroinflammation, formation of Aβ, and NFTs. Therefore, the hypothesis of drug-induced comorbidity cannot be ruled out. In this context, prolonged use of anti-psychotic drugs has been previously reported to induce symptoms of Parkinson’s disease (PD) [9–11]. The authors have rationalized this assumption by identifying blocked dopamine receptors and calcium channels by the drugs, both of which are impaired in PD. However, the postulation about this aspect of drugs in inducing a disease as a side-effect is still at its infancy.
The prevalence of study bias, which eventually leads to literature bias, is due to the fact that proteins with known biomedical functions and associated signaling pathways are studied recurrently [12, 13]. And because knowledge assemblies massively depend on literature resource, they inherit pre-existing bias. Therefore, chances are higher that literature aided inferences could represent biased knowledge. Taking this into consideration, Naz et al. (2017) have analyzed genomic data and performed functional assessment of prioritized SNPs using literature to depict stress-induced comorbid association in AD and PD [14]. This approach not only eliminates biasedness of over-representation of well-known biological entities and processes, but also identifies new genes and associated events which can serve as putative drug targets and drugable mechanisms. In this study, we have implemented a similar strategy in deciphering the comorbid link between T2DM and AD. The genomic data (i.e., SNPs) for AD and T2DM were fetched from curated public databases and subjected to linkage disequilibrium (LD) analysis. After filtering for shared SNPs in both diseases and prioritizing them based on their relevance to the diseases, we constructed cause-and-effect computable, network models using Biological Expression Language (BEL) [15]. The language enables conversion of unstructured textual information from literature into structured computer-readable triples (i.e., subject-predicate-object). The parsing and compilation of several triples after syntactic and semantic validation generates network models, which are also known as knowledge assemblies. Next, we added the dimension of high-throughput data as the driving force of our analysis by mapping differentially expressed genes to our knowledge assemblies. Finally, a mechanistic graph tailored by analysis of genomic and transcriptomic data was created from the knowledge assembly to explain the comorbid link between T2DM and AD.
MATERIALS AND METHODS
Firstly, a knowledge assembly embedding prioritized SNP was created from the literature. To this knowledge assembly, we mapped expression profiles from our gene expression analysis. Finally, we filtered the knowledge assembly with those genes which were consistently perturbed. The overall methodology implemented in this study can be divided into 1) data collection, 2) data analysis, and 3) interpretation. Firstly, we collected SNPs and gene expression (GE) datasets for AD and T2DM from freely accessible public databases (i.e., GWAS Catalog [16], GWAS Central [17], dbSNP [18], and DisGeNET [19]). Secondly, for SNP data, we conducted LD analysis followed by identification of shared SNPs and their prioritization using Polyphen-2 [20] and RegulomeDB [21]. Likewise, for GE datasets, we performed differential GE analysis followed by meta-analysis of AD and T2DM datasets. Lastly, we built a literature-derived knowledge assembly representing the results of the SNP analysis and mapped expression profiles of genes from the meta-analysis. A schematic diagram illustrating the methodology is shown in Fig. 1 and described in detail in the following sections.
Fig. 1
A schematic representation of the implemented workflow: The steps involved are 1) collection of genomic and gene expression data from open and freely accessible databases; 2) analysis of data using available tools and packages; and 3) construction of literature derived knowledge assembly and comorbid interpretation.
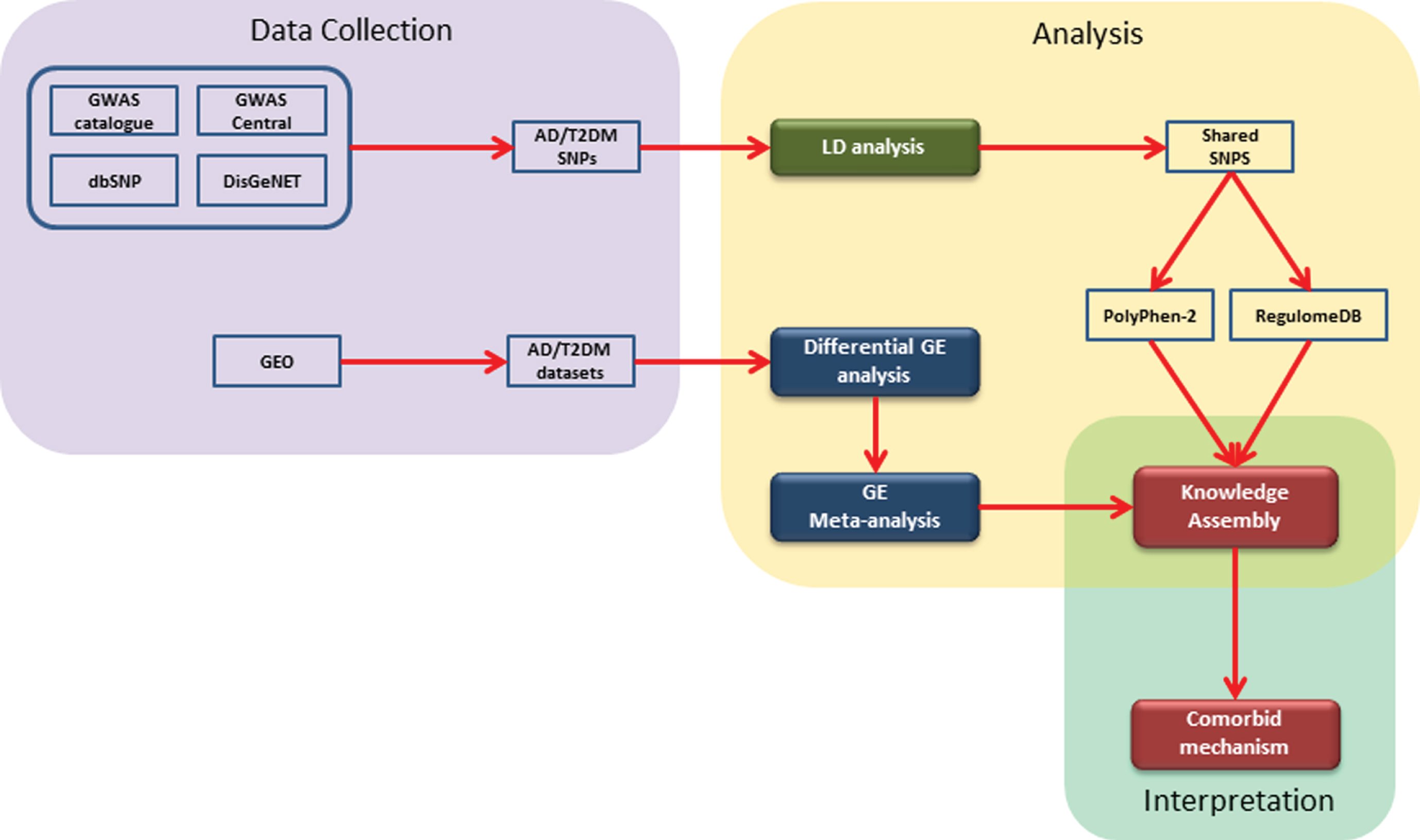
Retrieval of AD and T2DM SNPs from curated SNP databases
We retrieved a total of 1,130, 1,516, 1,420, and 1,062 SNPs associated to AD from GWAS Catalog, GWAS Central, dbSNP, and DisGeNET, respectively. Similarly, we extracted 1,791, 1,069, 1,865, and 1,522 SNPs associated to T2DM from GWAS Catalog, GWAS Central, dbSNP, and DisGeNET, respectively. To ensure the accuracy of our search, we queried dbSNP, GWAS central, and DisGeNET with corresponding Medical Subject Headings (MeSH) identifiers of AD (i.e., D000544) and T2DM (i.e., D003924). Likewise, we used Experimental Ontology Factor (EFO) identifiers of AD (i.e., EFO_0000249) and T2DM (i.e., EFO_0001360) for GWAS Catalog.
Linkage disequilibrium analysis and SNP prioritization
Using a total of 5,128 and 6,247 SNPs associated to AD and T2DM, respectively, we performed a LD analysis using the R-package haploR [22]. The function queryHaploreg was used with the default r2 threshold of 0.8 to perform the analysis. This yielded 77,486 SNPs in AD and 130,807 SNPs in T2DM. Out of these, 3,572 SNPs were shared between the diseases. Next, depending on whether the SNPs occur in coding or non-coding region of the gene, we used two databases to functionally annotate these shared SNPs. The impact of the SNPs located in the coding region and the resulting amino acid mutation along with the prediction, either benign or possibly damaging, was assessed using Polyphen-2. Likewise, the assessment of the SNPs in the regulatory region (non-coding) was performed using the function queryRegulome from R-package haploR. Subsequently, we prioritized them using RegulomeDB scores based on current ENCODE releases, Chromatin States from the Roadmap Epigenome Consortium as well as updates to DNase footprinting, Position Weight Matrix for TF binding, and DNA Methylation, and ENSEMBL SNP’s functional consequences [21, 23].
Literature corpus and cause-and-effect model using Biological Expression Language
The functional annotation of SNPs using Polyphen-2 and RegulomeDB helps in prioritization of SNPs. Nonetheless, these databases lack their putative roles in a disease context. In this study, we aimed at depicting mechanistic causal graphs embedding prioritized SNPs and their corresponding genes. This was achieved by building a comprehensive knowledge assembly using MEDLINE as the source of literature. The MeSH terms “Alzheimer Disease” and “Diabetes Mellitus, Type 2” were used to query PubMed (Date:02-12-2019) to create separate literature corpus of both diseases. The total number of articles for AD and T2DM were 90,215 and 127,020, respectively. Furthermore, through text mining, we created literature corpora that only contained shared SNPs and genes from LD analysis. The new corpus corresponding to AD and T2DM had a total of 14,293 and 9,032 articles, respectively. Next, we used BEL to capture causal and correlative relationships between the entities from the corpora. The language serves as an efficient platform to create computable knowledge assemblies by compiling relationships which are formulated in the form of triples. The conversion of regular text to BEL was assisted by BELIEF, a semi-automatic workflow to systematically extract BEL relationships from the corpus [24]. The outputs of the BELIEF workflow were manually curated to ensure high quality of the BEL relationships and then compiled using PyBEL for visualization [25].
Meta-analysis of gene expression datasets
In this study, our objective is to perform functional assessment of shared SNPs between AD and T2DM with the help of literature derived knowledge assemblies. In the Introduction section, we have already mentioned the possible bias that results from a purely literature-based construction of knowledge assemblies. Therefore, in order to tackle this issue, we mapped and investigated genes with consistent patterns of perturbed expressions to the knowledge assembly as such genes are more likely to be important in disease pathophysiology. A total of 14 GE datasets, 7 each for AD and T2DM, were selected from GEO (Gene Expression Omnibus). The selection of the datasets was done based on the criterion that the samples must be from humans (i.e., patients) diagnosed with AD or T2DM. Moreover, we did not consider datasets that used cell lines, induced medical conditions, animal models and modified genes or environments for expression analysis. The datasets were analyzed with GEO2R tool to identify differentially expressed genes in both diseases [26]. However, because expression patterns of the same disease are inconsistent and non-reproducible [27, 28], we performed a meta-analysis of the AD and T2DM GE datasets independently. This was achieved by using MetaVolcanoR, an R package with an algorithm based on voting approach and p-values of differentially expressed genes [29]. This allowed us to identify consistent patterns of perturbed gene expression across all the datasets. A brief description of each of the datasets is provided in Supplementary File 1.
RESULTS
Linkage disequilibrium analysis
The distribution analysis of 3,572 shared SNPs revealed that chromosome 1 had the highest number of SNPs, i.e., 495, followed by chromosome 17 (295 SNPs) and chromosome 8 (289 SNPs). The distribution of SNPs over all the chromosomes is shown in Supplementary File 2. The shared SNPs were mapped to 236 genes and the top 5 genes with the highest number of SNPS were lipoprotein lipase (LPL) (CHR 8, 153 SNPs), ubiquitin conjugating enzyme E2 D3 (UBE2D3) (CHR 4, 128 SNPs), leptin receptor (LEPR) (CHR 1, 116 SNPs), FTO alpha-ketoglutarate dependent dioxygenase (FTO) (CHR 16, 94 SNPs), and EF-hand calcium binding domain 5 (EFCAB5) (CHR 17, 86 SNPs). The full list of number of SNPs per each gene is provided in Supplementary File 3.
Assessment of SNPs with Polyphen-2 and RegulomeDB
A total of 64 SNPs, mapped to 50 genes, were identified by Polyphen-2 to be responsible for amino acid substitutions in their corresponding proteins. Out of these, 50 mutations were predicted to be benign while the remaining 14 mutations were predicted to be possibly damaging. Interestingly, mutations in the few well-characterized genes in AD and T2DM such as apolipoprotein E (APOE), brain derived neurotrophic factor (BDNF), and insulin receptor substrate 1 (IRS1) were classified as “possibly damaging”. The full list of Polyphen-2 output is provided in Supplementary File 4. Likewise, a total of 127 SNPs, mapped to 52 genes, were identified by RegulomeDB to be located in the functional region of their corresponding genes. This was indicated by the scores ranging between 1a and 1f. Genes such as APOE, translocase of outer mitochondrial membrane 40 (TOMM40), and interleukin 6 (IL6) were among the examples for the genes that were mapped to the 127 SNPs. The full list of RegulomeDB output is provided in Supplementary File 5.
Results from meta-analysis of GE datasets
The meta-analysis of AD datasets showed 206 genes to exhibit consistent patterns of perturbed expression, where 49 genes were underexpressed and 157 genes were overexpressed. Similarly, in T2DM, a total of 142 genes regulated persistently, with 13 genes showing downregulation and 129 genes that were consistently upregulated. Out of these, 3 genes, i.e., interferon gamma inducible protein 16 (IFI16), syntrophin beta 2 (SNTB2), and laminin subunit alpha 4 (LAMA4) were found to be overexpressed in meta-analyses of AD and T2DM. The full list of differentially expressed genes and plots showing expression patterns of each datasets are provided in Supplementary Files 6 and 7, respectively. The implementation of GE meta-analysis after differential GE analysis is justified by our findings that the number of coherently perturbed genes reduced with increasing number of GE datasets. This implies the ability of GE meta-analysis to yield robustness and convergence of expression patterns.
Comorbidity in AD and T2DM explained by mechanistic BEL graphs
The knowledge assemblies representing AD and T2DM were combined to investigate the role of shared SNPs and their corresponding genes along with consistently perturbed genes from our meta-analyses. The merged network had a total of 692 nodes and 1,793 edges. The top 5 biological processes based on highest degree of node centrality were insulin resistance, inflammatory response, aggregation of Aβ, apoptotic process, and oxidative stress. Similarly, cystatin C (CST3), BDNF, peroxisome proliferator activated receptor gamma (PPARG), MAPT, and LEPR were the top 5 genes in the network. We mapped persistently perturbed genes from the meta-analyses to the network and used them as driving force of our comorbid analysis. The rationale supporting this implementation are 1) abnormal expression of genes and their activities mutilate biological processes and pathways and thus are responsible for manifesting disease characteristics, and 2) it overcomes the risk of representing biased knowledge. A mechanistic graph embedding corresponding genes of our SNP analysis and abnormally expressed genes is shown in Fig. 2 It had a total of 41 nodes and 45 edges and comprised of 4 genes (i.e., cholinergic receptor nicotinic alpha 3 subunit (CHRNA3) (CHR 15, 6 SNPs), catechol-O-methyltransferase (COMT) (CHR 22, 4 SNPs), nuclear receptor subfamily 1 group H member 3 (NR1H3) (CHR 11, 13 SNPs), and transforming growth factor beta 1 (TGFB1) (CHR 19, 4 SNPs) sharing 27 SNPs between AD and T2DM.
Fig. 2
Mechanistic comorbid association: The cartoon diagram represents causal interactions between entities and events led by prioritized SNPs, their corresponding genes and consistently dysregulated genes. Genes such as COMT, MMP9, ABCG1, and SOD2 were identified to contribute to biological processes such as oxidative stress, apoptosis and cognition, all of which are associated with T2DM and AD. Since the onset of T2DM takes place earlier than AD, this diagram is inclined toward AD-related phenomena (i.e., NFT aggregation, synaptic plasticity, etc.) which are influenced by downstream T2DM-related genes.
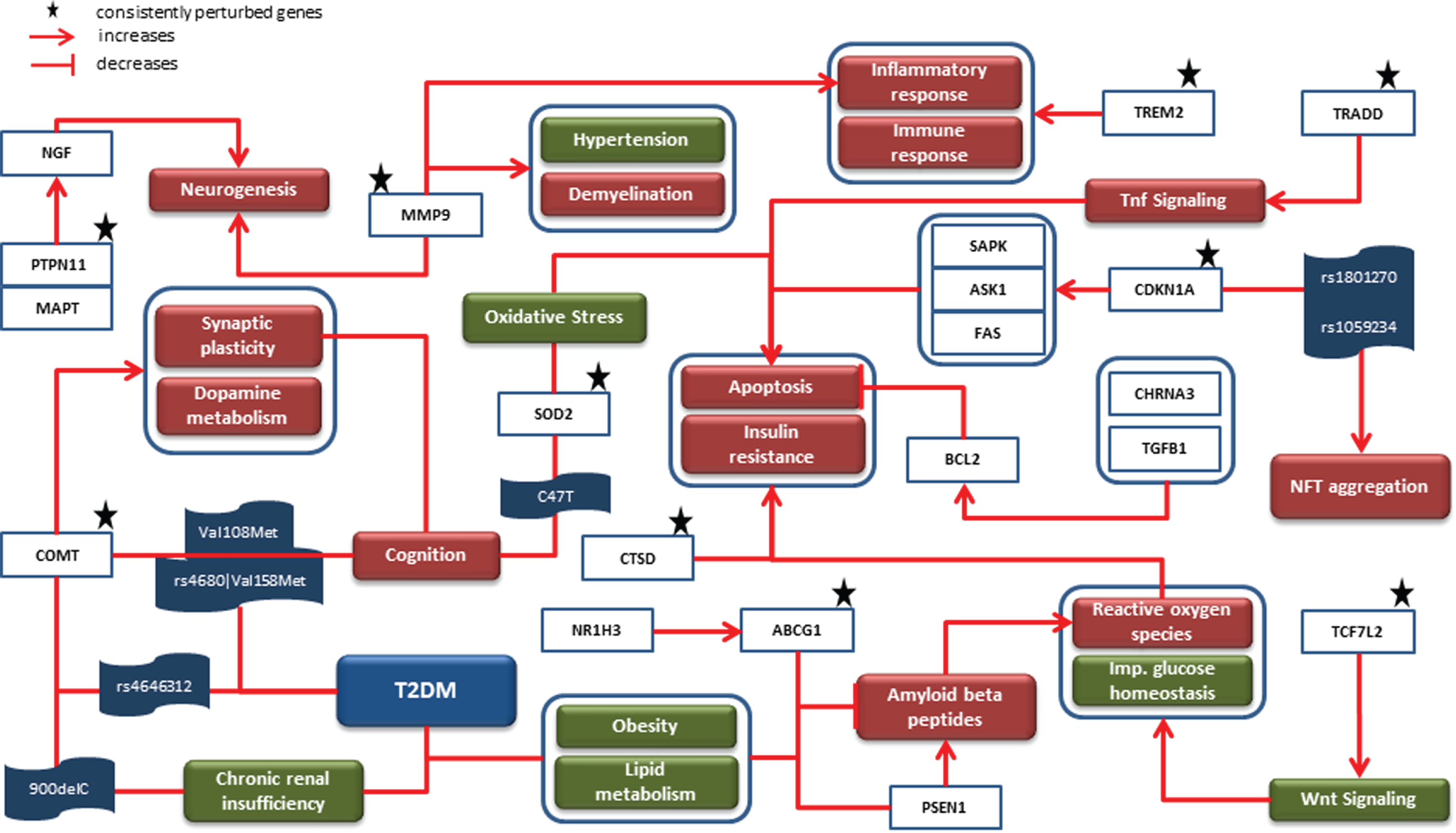
As shown in Fig. 2, COMT is known to influence synaptic plasticity and dopamine metabolism, both of which are associated with cognition. In this context, two point mutations (i.e., Val108Met and rs4680 –>Val158Met) in this gene were identified to be predictors of cognition scores in AD patients through independent studies [30, 31]. Interestingly, the latter mutation along with rs4646312 in COMT has been associated with T2DM [32]. Also, the 900delC variant form in COMT correlates to chronic renal insufficiency in T2DM [33]. Along the same lines, C47T variant in superoxide dismutase 2 (SOD2) is associated with cognition [34]. Likewise, ATP binding cassette subfamily G member 1 (ABCG1), which is upregulated by NR1H3 [35], has been linked with T2DM because of its involvement in obesity and lipid metabolism [36, 37]. In AD, ABCG1 is reported to inhibit the process of formation of Aβ through inhibition of presenilin 1 (PSEN1) [38]. The amyloid hypothesis in AD considers PSEN1 as one of the two important enzymes that is responsible for abnormal cleaving of AβPP [39], the other being beta-secretase 1 (BACE1) [40]. The accumulated Aβ leading to production of reactive oxygen species (ROS) which further increases oxidative stress to consequently trigger apoptosis is well understood through several studies in AD [41, 42]. Similarly, transcription factor 7 like 2 (TCF7L2)-activated Wnt signaling has been reported to generate ROS and impair glucose homeostasis [43]. The excess of ROS thus produced is detrimental as it results in insulin resistance [44]. Moreover, another study has identified dysfunctional cathepsin D (CTSD) to increase both insulin resistance and apoptosis [45, 46]. In addition, SOD2 induced oxidative stress [47], TNFRSF1A associated via death domain (TRADD) activated Tnf signaling, and cyclin dependent kinase inhibitor 1A (CDKN1A) activated mitogen-activated protein kinase 9 (MAPK9) [48], mitogen-activated protein kinase kinase kinase 5 (MAP3K5), and Fas cell surface death receptor (FAS) also lead to apoptosis [49]. In contrast, the suppression of apoptosis takes place through CHRNA3 [50] and TGFB1 activated BCL2 apoptosis regulator (BCL2) [51]. The identification of two SNPs (i.e., rs1801270 and rs1059234) in CDKN1A positively correlated with NFT aggregation in AD patients [49]. The binding of protein tyrosine phosphatase non-receptor type 11 (PTPN11) and MAPT promotes neurogenesis by activating nerve growth factor [52]. Besides, matrix metallopeptidase 9 (MMP9) is also suggested to play a part in neurogenesis and other biological processes such as hypertension, demyelination, and inflammatory response [53, 54]. Lastly, triggering receptor expressed on myeloid cells 2 (TREM2) activity is found to influence inflammatory and immune response [55].
DISCUSSION
In this study, we formulated an integrative approach of combining data and knowledge to unravel new insights about the possible association between AD and T2DM. Our data-driven modeling of knowledge assemblies represents highly specialized knowledge on comorbidities. While most of the data analytics workflow end up in gene set enrichment analysis, our approach has opened up a new avenue of mechanism-centric interpretation of data. We have used two different data modalities to guide the extraction process of relevant literature knowledge. Firstly, we identified shared SNPs between AD and T2DM from curated resources and built a knowledge assembly around prioritized SNPs and their corresponding genes. Although literature can also be used as a source of SNP information, it is important to note that we have considered only curated databases for retrieval of SNPs. This decision can be explained by the fact that curated databases ensure association between a SNP and a disease with a given statistical significance (i.e., p-value). Unlike curated databases, some SNPs mentioned in the literature might not have any association with a disease because the statistical power of association is below par [56–59]. Therefore, by including such SNPs, we would be adding possible false positives in our analysis and, thus, diminishing the quality of the results. Secondly, as we are aware of the literature bias in knowledge assemblies, we identified consistently perturbed genes by conducting a GE meta-analysis and used these signals to mechanistically link AD and T2DM. Our results illustrate that genes such as COMT, MMP9, SOD2, and ABCG1, which do not belong to the realm of well-known genes in AD and T2DM, are involved in important biological processes of both diseases. This suggests dysfunctional activities of these genes could be the bridge between these diseases. Moreover, our findings endorse and strengthen the proposition of AD and T2DM comorbidity suggested by epidemiological, preclinical, and pathophysiology studies by identifying novel genes.
The genetic variants of AD and T2DM amassed in our study are readily explorable and bear the potential to yield new insights. For instance, genomic loci dependent SNPs can be functionally assessed to uncover their roles in the underlying comorbid mechanisms. This would enable identification of “genomic hotspots” that are closely associated to AD and T2DM. However, this study does not address this aspect due to time constraints and it is out-of-scope of our objectives. Also, we have not considered the role of epigenetic modifications in the comorbid association between AD and T2DM. Nonetheless, our knowledge assemblies can be used as the starting point for assimilating epigenetic modifications concerning AD and T2DM.
ACKNOWLEDGMENTS
This work was funded by Bundesministerium für Bildung und Forschung (BMBF, e:Med initiative COMMITMENT (grant number: 01ZX1904C)). The authors would also like to acknowledge the financial support from the B-IT foundation that sponsors part of the academic work in our department.
Authors’ disclosures available online (https://www.j-alz.com/manuscript-disclosures/20-0752r1).
SUPPLEMENTARY MATERIAL
[1] The supplementary material is available in the electronic version of this article: https://dx.doi.org/10.3233/JAD-200752.
REFERENCES
[1] | Haregu TN , Oldenburg BF , Setswe G , Elliott J ((2012) ) Perspectives, constructs and methods in the measurement of multimorbidity and comorbidity: A critical review. Internet J Epidemiol 10: , 1–11. |
[2] | Pugazhenthi S , Qin L , Reddy PH ((2017) ) Common neurodegenerative pathways in obesity, diabetes, and Alzheimer’s disease. Biochim Biophys Acta Mol Basis Dis 1863: , 1037–1045. |
[3] | de la Monte SM , Wands JR ((2008) ) Alzheimer’s disease is type 3 diabetes—evidence reviewed. J Diabetes Sci Technol 2: , 1101–1113. |
[4] | de la Monte SM , Chen GJ , Rivera E , Wands JR ((2003) ) Neuronal thread protein regulation and interaction with microtubule-associated proteins in SH-Sy5y neuronal cells. Cell Mol Life Sci C 60: , 2679–2691. |
[5] | Yashin AI , Fang F , Kovtun M , Wu D , Duan M , Arbeev K , Akushevich I , Kulminski A , Culminskaya I , Zhbannikov I , Tashkin A , Stallard E , Ukraintseva S ((2018) ) Hidden heterogeneity in Alzheimer’s disease: Insights from genetic association studies and other analyses. Exp Gerontol 107: , 148–160. |
[6] | Akter K , Lanza EA , Martin SA , Myronyuk N , Rua M , Raffa RB ((2011) ) Diabetes mellitus and Alzheimer’s disease: Shared pathology and treatment? Br J Clin Pharmacol 71: , 365–376. |
[7] | Kodamullil AT , Younesi E , Naz M , Bagewadi S , Hofmann-Apitius M ((2015) ) Computable cause-and-effect models of healthy and Alzheimer’s disease states and their mechanistic differential analysis. Alzheimers Dement 11: , 1329–1339. |
[8] | Karki R , Kodamullil AT , Hofmann-Apitius M ((2017) ) Comorbidity analysis between Alzheimer’s disease and type 2 diabetes mellitus (T2DM) based on shared pathways and the role of T2DM drugs. J Alzheimers Dis 60: , 721–731. |
[9] | Bohlega SA , Al-Foghom NB ((2013) ) Drug-induced Parkinson’s disease. A clinical review. Neurosci 18: , 215–221. |
[10] | Shin HW , Chung SJ ((2012) ) Drug-induced Parkinsonism. J Clin Neurol 8: , 15–21. |
[11] | Bashford G , Bradd P ((1996) ) Drug-induced Parkinsonism associated with dysphagia and aspiration: A brief report. J Geriatr Psychiatry Neurol 9: , 133–135. |
[12] | Schaefer MH , Serrano L , Andrade-Navarro MA ((2015) ) Correcting for the study bias associated with protein–protein interaction measurements reveals differences between protein degree distributions from different cancer types. Front Genet 6: , 260. |
[13] | Charitou T , Bryan K , Lynn DJ ((2016) ) Using biological networks to integrate, visualize and analyze genomics data. Genet Sel Evol 48: , 27. |
[14] | Naz M , Younesi E , Hofmann-Apitius M ((2017) ) Systematic analysis of GWAS data reveals genomic hotspots for shared mechanisms between neurodegenerative diseases. J Alzheimers Dis Park 7: , 460–2161. |
[15] | Slater T , Song D ((2012) ) Saved by the BEL: Ringing in a common language for the life sciences. Drug Discov World Fall 2012: , 75–80. |
[16] | Buniello A , MacArthur JAL , Cerezo M , Harris LW , Hayhurst J , Malangone C , McMahon A , Morales J , Mountjoy E , Sollis E , Suveges D , Vrousgou O , Whetzel PL , Amode R , Guillen JA , Riat HS , Trevanion SJ , Hall P , Junkins H , Flicek P , Burdett T , Hindorff LA , Cunningham F , Parkinson H ((2019) ) The NHGRI-EBI GWAS catalog of published genome-wide association studies, targeted arrays and summary statistics 2019. Nucleic Acids Res 47: , D1005–D1012. |
[17] | Beck T , Shorter T , Brookes AJ ((2020) ) GWAS central: A comprehensive resource for the discovery and comparison of genotype and phenotype data from genome-wide association studies. Nucleic Acids Res 48: , D933–D940. |
[18] | Smigielski EM , Sirotkin K , Ward M , Sherry ST ((2000) ) dbSNP: A database of single nucleotide polymorphisms. Nucleic Acids Res 28: , 352–355. |
[19] | Piñero J , Ramírez-Anguita JM , Saüch-Pitarch J , Ronzano F , Centeno E , Sanz F , Furlong LI ((2019) ) The DisGeNET knowledge platform for disease genomics: 2019 update. Nucleic Acids Res 48: , D845–D855. |
[20] | Adzhubei IA , Schmidt S , Peshkin L , Ramensky VE , Gerasimova A , Bork P , Kondrashov AS , Sunyaev SR ((2010) ) A method and server for predicting damaging missense mutations. Nat Methods 7: , 248–249. |
[21] | Boyle AP , Hong EL , Hariharan M , Cheng Y , Schaub MA , Kasowski M , Karczewski KJ , Park J , Hitz BC , Weng S , Cherry JM , Snyder M ((2012) ) Annotation of functional variation in personal genomes using RegulomeDB. Genome Res 22: , 1790–1797. |
[22] | Zhbannikov IY , Arbeev K , Ukraintseva S , Yashin AI ((2017) ) haploR: An R package for querying web-based annotation tools. F1000Res 6: , 97. |
[23] | Aken BL , Ayling S , Barrell D , Clarke L , Curwen V , Fairley S , Fernandez Banet J , Billis K , García Girón C , Hourlier T , Howe K , Kähäri A , Kokocinski F , Martin FJ , Murphy DN , Nag R , Ruffier M , Schuster M , Tang YA , Vogel JH , White S , Zadissa A , Flicek P , Searle SMJ ((2016) ) The Ensembl gene annotation system. Database (Oxford) 2016: , baw093. |
[24] | Fluck J , Madan S , Ansari S , Szostak J , Hoeng J , Zimmermann M , Hofmann-Apitius M , Peitsch MC ((2014) ) BELIEF-A semiautomatic workflow for BEL network creation. In Proceedings of the 6th International Symposium on Semantic Mining in Biomedicine, pp. 109-113. |
[25] | Hoyt CT , Konotopez A , Ebeling C ((2018) ) PyBEL: A computational framework for biological expression language. Bioinformatics 34: , 703–704. |
[26] | Clough E , Barrett T ((2016) ) The gene expression omnibus database. Methods Mol Biol 1418: , 93–110. |
[27] | Jafari P , Azuaje F ((2006) ) An assessment of recently published gene expression data analyses: Reporting experimental design and statistical factors. BMC Med Inform Decis Mak 6: , 27. |
[28] | Ntzani EE , Ioannidis JP ((2003) ) Predictive ability of DNA microarrays for cancer outcomes and correlates: An empirical assessment. Lancet 362: , 1439–1444. |
[29] | Prada C , Lima D , Nakaya H ((2020) ) MetaVolcanoR: Gene expression meta-analysis visualization tool. R Packag. version 1.1. |
[30] | Lanni C , Garbin G , Lisa A , Biundo F , Ranzenigo A , Sinforiani E , Cuzzoni G , Govoni S , Ranzani GN , Racchi M ((2012) ) Influence of COMT Val158Met polymorphism on Alzheimer’s disease and mild cognitive impairment in Italian patients. J Alzheimers Dis 32: , 919–926. |
[31] | Perkovic MN , Strac DS , Tudor L , Konjevod M , Erjavec GN , Pivac N ((2018) ) Catechol-O-methyltransferase, cognition and Alzheimer’s disease. Curr Alzheimer Res 15: , 408–419. |
[32] | Xiu L , Lin M , Liu W , Kong D , Liu Z , Zhang Y , Ouyang P , Liang Y , Zhong S , Chen C , Jin X , Fan X , Qin J , Zhao X , Rao S , Ding Y ((2015) ) Association of DRD3, COMT, and SLC6A4 gene Polymorphisms with type 2 diabetes in Southern Chinese: A hospital-based case-control study. Diabetes Technol Ther 17: , 580–586. |
[33] | Prasad P , Kumar KM , Ammini AC , Gupta A , Gupta R , Thelma BK ((2008) ) Association of dopaminergic pathway gene polymorphisms with chronic renal insufficiency among Asian Indians with type-2 diabetes. BMC Genet 9: , 26. |
[34] | Gamarra D , Elcoroaristizabal X , Fernández-Martínez M , de Pancorbo MM ((2015) ) Association of the C47T polymorphism in SOD2 with amnestic mild cognitive impairment and Alzheimer’s disease in carriers of the APOEɛ4 allele. Dis Markers 2015: , 746329. |
[35] | Natunen T , Martiskainen H , Sarajärvi T , Helisalmi S , Pursiheimo JP , Viswanathan J , Laitinen M , Mäkinen P , Kauppinen T , Rauramaa T , Leinonen V , Alafuzoff I , Haapasalo A , Soininen H , Hiltunen M ((2013) ) Effects of NR1H3 genetic variation on the expression of liver X receptor α and the progression of Alzheimer’s disease. PLoS One 8: , e80700. |
[36] | Choromanska B , Mysliwiec P , Hady HR , Dadan J , Mysliwiec H , Bonda T , Chabowski A , Miklosz A ((2019) ) The implication of adipocyte ATP-binding cassette A1 and G1 transporters in metabolic complications of obesity. J Physiol Pharmacol 70: , doi: 10.26402/jpp.2019.1.14 |
[37] | Churm R , Caplin S , Barry J , Davies JS , Stephens JW , Prior SL ((2019) ) Acyl-ghrelin mediated lipid retention and inflammation in obesity-related type 2 diabetes. Mol Cell Endocrinol 481: , 8–13. |
[38] | Sano O , Tsujita M , Shimizu Y , Kato R , Kobayashi A , Kioka N , Remaley AT , Michikawa M , Ueda K , Matsuo M ((2016) ) ABCG1 and ABCG4 suppress γ-secretase activity and amyloid β production. PLoS One 11: , e0155400. |
[39] | Selkoe DJ ((1994) ) Cell biology of the amyloid beta-protein precursor and the mechanism of Alzheimer’s disease. Annu Rev Cell Biol 10: , 373–403. |
[40] | Cai H , Wang Y , McCarthy D , Wen H , Borchelt DR , Price DL , Wong PC ((2001) ) BACE1 is the major beta-secretase for generation of Abeta peptides by neurons. Nat Neurosci 4: , 233–234. |
[41] | Cai Z , Zhao B , Ratka A ((2011) ) Oxidative stress and β-amyloid protein in Alzheimer’s disease. Neuromolecular Med 13: , 223–250. |
[42] | Loh KP , Huang SH , De Silva R , Tan BK , Zhu YZ ((2006) ) Oxidative stress: Apoptosis in neuronal injury. Curr Alzheimer Res 3: , 327–337. |
[43] | Yoon JC , Ng A , Kim BH , Bianco A , Xavier RJ , Elledge SJ ((2010) ) Wnt signaling regulates mitochondrial physiology and insulin sensitivity. Genes Dev 24: , 1507–1518. |
[44] | Zhai L , Ballinger SW , Messina JL ((2011) ) Role of reactive oxygen species in injury-induced insulin resistance. Mol Endocrinol 25: , 492–502. |
[45] | Nowak C , Sundström J , Gustafsson S , Giedraitis V , Lind L , Ingelsson E , Fall T ((2016) ) Protein biomarkers for insulin resistance and type 2 diabetes risk in two large community cohorts. Diabetes 65: , 276–284. |
[46] | Di YQ , Han XL , Kang XL , Wang D , Chen CH , Wang JX , Zhao XF ((2020) ) Autophagy triggers CTSD (cathepsin D) maturation and localization inside cells to promote apoptosis. Autophagy, doi: 10.1080/15548627.2020.1752497 |
[47] | Pias EK , Ekshyyan OY , Rhoads CA , Fuseler J , Harrison L , Aw TY ((2003) ) Differential effects of superoxide dismutase isoform expression on hydroperoxide-induced apoptosis in PC-12 cells. J Biol Chem 278: , 13294–13301. |
[48] | Zhao M , Cribbs DH , Anderson AJ , Cummings BJ , Su JH , Wasserman AJ , Cotman CW ((2003) ) The induction of the TNFalpha death domain signaling pathway in Alzheimer’s disease brain. Neurochem Res 28: , 307–318. |
[49] | Yates SC , Zafar A , Rabai EM , Foxall JB , Nagy S , Morrison KE , Clarke C , Esiri MM , Christie S , Smith AD , Nagy Z ((2015) ) The effects of two polymorphisms on p21cip1 function and their association with Alzheimer’s disease in a population of European descent. PLoS One 10: , e0114050. |
[50] | Zhang XL , Qi XL , Ren JM , Wu CX , Guan ZZ ((2013) ) Effects of α3 neuronal nicotinic acetylcholine receptor on cell apoptosis and p38 MAPK signal transduction pathway in SH-SY5Y cells. Zhonghua Bing Li Xue Za Zhi 42: , 116–120. |
[51] | Tan J , Town T , Placzek A , Kundtz A , Yu H , Mullan M ((1999) ) Bcl-X(L) inhibits apoptosis and necrosis produced by Alzheimer’s beta-amyloid1-40 peptide in PC12 cells. Neurosci Lett 272: , 5–8. |
[52] | Kim Y , Liu G , Leugers CJ , Mueller JD , Francis MB , Hefti MM , Schneider JA , Lee G ((2019) ) Tau interacts with SHP2 in neuronal systems and in Alzheimer’s disease brains. J Cell Sci 132: , jcs229054. |
[53] | Kaminari A , Tsilibary EC , Tzinia A ((2018) ) A new perspective in utilizing MMP-9 as a therapeutic target for Alzheimer’s disease and type 2 diabetes mellitus. J Alzheimers Dis 64: , 1–16. |
[54] | Kostov K , Blazhev A , Atanasova M , Dimitrova A ((2016) ) Serum concentrations of endothelin-1 and matrix metalloproteinases-2, -9 in pre-hypertensive and hypertensive patients with type 2 diabetes. Int J Mol Sci 17: , 1182. |
[55] | Misra A , Chakrabarti SS , Gambhir IS ((2018) ) New genetic players in late-onset Alzheimer’s disease: Findings of genome-wide association studies. Indian J Med Res 148: , 135–144. |
[56] | Sajovic J , Cilenšek I , Mankoč S , Tajnšek Š , Kunej T , Petrovič D , Petrovič MG ((2019) ) Vascular endothelial growth factor (VEGF)-related polymorphisms rs10738760 and rs6921438 are not risk factors for proliferative diabetic retinopathy (PDR) in patients with type 2 diabetes mellitus (T2DM). Bosn J Basic Med Sci 19: , 94. |
[57] | Završnik M , Letonja J , Makuc J , Šeruga M , Cilenšek I , Petrovič D ((2018) ) Interleukin-4 (IL4) -590C/T (rs2243250) gene polymorphism is not associated with diabetic nephropathy (DN) in Caucasians with type 2 diabetes mellitus (T2DM). Bosn J Basic Med Sci 18: , 347–351. |
[58] | Bey K , Wolfsgruber S , Karaca I , Wagner H , Lardenoije R , Becker J , Milz E , Kornhuber J , Peters O , Frölich L , Hüll M , Rüther E , Wiltfang J , Riedel-Heller S , Scherer M , Jessen F , Maier W , van den Hove DL , Rutten BP , Wagner M , Ramirez A ((2016) ) No association of the variant rs11887120 in DNMT3A with cognitive decline in individuals with mild cognitive impairment. Epigenomics 8: , 593–598. |
[59] | Zeng F , Deng YP , Yi X , Cao HY , Zou HQ , Wang X , Liang CR , Wang YR , Zhang LL , Gao CY , Xu ZQ , Lian Y , Wang L , Zhou XF , Zhou HD , Wang YJ ((2013) ) No association of SORT1 gene polymorphism with sporadic Alzheimer’s disease in the Chinese Han population. Neuroreport 24: , 464–468. |