Computational Modeling of Catecholamines Dysfunction in Alzheimer’s Disease at Pre-Plaque Stage
Abstract
Background:
Alzheimer’s disease (AD) etiopathogenesis remains partially unexplained. The main conceptual framework used to study AD is the Amyloid Cascade Hypothesis, although the failure of recent clinical experimentation seems to reduce its potential in AD research.
Objective:
A possible explanation for the failure of clinical trials is that they are set too late in AD progression. Recent studies suggest that the ventral tegmental area (VTA) degeneration could be one of the first events occurring in AD progression (pre-plaque stage).
Methods:
Here we investigate this hypothesis through a computational model and computer simulations validated with behavioral and neural data from patients.
Results:
We show that VTA degeneration might lead to system-level adjustments of catecholamine release, triggering a sequence of events leading to relevant clinical and pathological signs of AD. These changes consist first in a midfrontal-driven compensatory hyperactivation of both VTA and locus coeruleus (norepinephrine) followed, with the progression of the VTA impairment, by a downregulation of catecholamine release. These processes could then trigger the neural degeneration at the cortical and hippocampal levels, due to the chronic loss of the neuroprotective role of norepinephrine.
Conclusion:
Our novel hypothesis might contribute to the formulation of a wider system-level view of AD which might help to devise early diagnostic and therapeutic interventions.
INTRODUCTION
Alzheimer’s disease (AD) is a severely disabling neurodegenerative disorder leading to a progressive memory loss followed by worsening deficits in all cognitive domains, including language, visuospatial skills, and executive functions. Subtle non-cognitive alterations such as depression and apathy are also very common among people suffering from AD, starting from the early and middle stages, whereas motor impairments (e.g., dystonia, tremor) may appear in the later stages [1, 2]. Several hypotheses have been proposed to explain the causes of AD. The main histopathological findings related to AD are the abnormal accumulation of amyloid-β (Aβ) oligomers leading to plaque formation and the aggregation of hyperphosphorylated tau protein into neurofibrillary tangles. Both phenomena produce cytotoxic effects leading to cortical cell death [3, 4]. Another histopathological finding involves the degeneration of subcortical neuromodulatory nuclei. A common finding is, for example, the loss of cholinergic neurons in the nucleus basalis of Meynert (NbM) causing impairment in cholinergic neurotransmission in the cerebral cortex and other target areas involved in learning, memory, and emotional regulation (e.g., hippocampus and amygdala) [5–7], and leading to the deterioration of cognitive functions [8, 9]. Several data from postmortem studies and murine models of AD suggest degenerative histological abnormalities also in the main dopamine (DA) nuclei, the ventral tegmental area (VTA) and the substantia nigra pars compacta [10–12]. Pathological alterations of the meso-cortico-limbic circuit might contribute to cognitive and behavioral signs that may occur early in the disease progression while impairments of the meso-striatal circuit are associated to the development of extrapyramidal motor deficits usually occurring in the later stages of AD [13]. Another subcortical neuromodulatory nucleus affected in AD is the locus coeruleus (LC), the dorsal pontine nucleus that synthesizes norepinephrine (NE), involved in attention, memory, and various other aspects of cognition [14–16]. Extensive analysis of tau lesions in a large cohort, involving 2,332 non selected autopsy cases ranging in age from 1 to 100 years of normal and diseased brains, shows that abnormal (hyperphosphorylated) tau in a few neurons of the LC [17, 18] can appear very early in the AD progression. Overall, these data support a critical role of neuromodulatory dysregulation, in particular of catecholamines (DA and NE), in the AD-related pathophysiology [19].
However, the causes of these alterations, the relationship between them, and their potential role in the AD progression are important elements not yet elucidated [20]. Despite the large investments and research carried out within the Amyloid Cascade Hypothesis, it has not yet been possible to provide a unifying theory on the causal relations between Aβ oligomers formation, catecholamines dysregulation, and cognitive/non-cognitive impairments, suggesting the need of integrating these phenomena within a system-neuroscience approach [19].
In this regard, Nobili and colleagues [20] investigated the structural alterations of the midbrain dopaminergic system in a validated animal model of AD (Tg2576 mouse). They found an age-dependent dopaminergic neuron loss in the VTA at a stage when hyperphosphorylated tau tangles, Aβ-plaque deposition, or any sign of neurodegeneration in hippocampal and cortical regions involved in memory deficits has not yet occurred. The VTA degeneration results in a lower DA outflow in the nucleus accumbens and hippocampus and this is associated with dysfunctions in memory performance, cost-benefit decision-making, food reward processing, and depressive-like symptoms [21]. These preclinical results challenged clinical investigations aimed to correlate dopaminergic alterations with cognitive and non-cognitive alterations along the AD stages.
An MRI study corroborated this finding by showing a positive correlation between the VTA volume and both hippocampal size and memory performance in a cohort of patients compared with healthy controls [22], while other authors used functional brain magnetic resonance imaging (fMRI) at rest to investigate the VTA-driven modulation of connectivity in AD brains and its impact on behavioral symptoms [23]. Very recently, it has been reported a positive correlation of atrophy in VTA projecting areas with severity of depression, apathy, and anxiety in the prodromal phase of AD while no metabolic connectivity changes have been detected within nigrostriatal pathway [24].
In this paper, we propose a hypothesis to clarify the role of catecholamines dysregulation in the AD progression. In order to introduce the hypothesis in a detailed operational form capable of producing quantitative testable predictions, we formulate it through a neuro-computational model. This builds on a previous model, the Reinforcement Meta-Learner (RML), proposed by Silvetti and colleagues and validated with different datasets [25]. We chose this model because it simulates the dynamics involved in the modulation operated by the medial prefrontal cortex on two subcortical nuclei critically involved in AD development, the VTA [20, 22] and the LC [26]. Through the RML we articulate the hypothesis for which a primary loss of dopaminergic neurons in the VTA causes a dysregulation of catecholamine release (including NE), starting a cascade of complex effects that first lead to motivational and cognitive impairments during the early stages of the disease, and then evolve toward the Aβ oligomers formation and the tau protein aggregation into neurofibrillary tangles, possibly due to the chronic loss of the neuroprotective role of NE. To show this, we administered to the RML two different behavioral tasks while damaging the model component simulating the VTA, and compared the behavioral and neural dynamics of the model with those from human patients at the early stages of AD. Both the behavioral and neural dynamics of the model agree with data from human patients. This corroborates our hypothesis on the catecholamine role in AD pathogenesis and highlights the potential utility of the model to formulate a wider system-level view of AD and device new computer-based early diagnostic and therapeutic tools.
The Material and Methods section describes the equations composing the RML model and the simulations details (the interested reader can refer to [25] for a wider description of the RML and the broad range of neuroscience domains where it can be applied). The reader who is not interested in the technical details of the model can directly move to the Results section, where we provide a qualitative description of the model.
MATERIALS AND METHODS
RML equations
The RML architecture consists of four computational modules, two simulating part of the medial prefrontal cortex: the dorsal anterior cingulate cortex (dACCAct and dACCBoost) and two simulating the brainstem catecholamine nuclei (VTA and LC). Cortical and subcortical modules are reciprocally connected in order to generate a control loop that optimizes both catecholamines release (subcortical) and decision-making operations (cortical) while the RML interacts with the external environment (Fig. 1a). This machinery allows the optimization of neuromodulation supporting the cognitive and behavioral efficacy of the RML while it executes a task.
Fig.1
a) Overview of the RML model. This consists of two interacting systems: a state-action selection system (dACCBoost and dACCAct), based on reinforcement learning processes, and a parameter modulation system via catecholamine release (VTA and LC). The RML model can be connected to an external neural component (e.g., the model FROST representing the fronto-parietal network) that is targeted by the LC output (NE), while the entire system (RML + external component) interacts with the environment. Modified from [25]. b) The FROST model [28] simulates a fronto-parietal network for visuo-spatial WM (F, prefrontal neurons; P, parietal neurons). The RML-FROST integrated system can perform visuo-spatial WM tasks by optimizing prefrontal activity (F) via LC activity. c) Plot showing the gain field modulation by LC on one prefrontal (F) neuron, during a delayed matching-to-sample task. A stimulus is presented inside the receptive field of the neuron, followed by a delay period, and then by a second stimulus (target) in the same spatial position.
![a) Overview of the RML model. This consists of two interacting systems: a state-action selection system (dACCBoost and dACCAct), based on reinforcement learning processes, and a parameter modulation system via catecholamine release (VTA and LC). The RML model can be connected to an external neural component (e.g., the model FROST representing the fronto-parietal network) that is targeted by the LC output (NE), while the entire system (RML + external component) interacts with the environment. Modified from [25]. b) The FROST model [28] simulates a fronto-parietal network for visuo-spatial WM (F, prefrontal neurons; P, parietal neurons). The RML-FROST integrated system can perform visuo-spatial WM tasks by optimizing prefrontal activity (F) via LC activity. c) Plot showing the gain field modulation by LC on one prefrontal (F) neuron, during a delayed matching-to-sample task. A stimulus is presented inside the receptive field of the neuron, followed by a delay period, and then by a second stimulus (target) in the same spatial position.](https://content.iospress.com:443/media/jad/2020/77-1/jad-77-1-jad200276/jad-77-jad200276-g001.jpg)
Here we describe the RML functioning in its discrete-time implementation. All the free parameters of the model, reported in Table 1, were set as in Silvetti et al. [25] (see also this work for the dynamical version of the model). The software used for the simulations can be downloaded from the RML GitHub repository: https://github.com/AL458/RML. Data from human studies were extracted from the figures of their relative articles by means of WebPlotDigitizer (https://automeris.io/WebPlotDigitizer/).
Table 1
Parameters list and values of the model
Parameter | Value | Meaning | Equation |
ρ | 0.2 | TD-learning signal decay | 6a |
μ | 0.1 | DA dynamics | 6a |
τ | 0.6 | Softmax temperature | 2 |
α | 0.3 | low-pass filter meta-parameter | 5c–d |
β | 0.2 | Learning rate lower bound | 5a |
ω | 0.15 | Boosting cost | 6b |
We now describe the functioning and equations of each RML component.
dACCAct
The central equations in this module govern the state/action value updates:
(1a)
(1b)
(2)
dACCBoost
The dACCBoost module controls the parameters for cost and reward signals in equations 1–2 (dACCAct), via the modulation of the VTA and LC activity (boosting catecholamines). This is implemented by selecting the modulatory signal b (boost signal), by RL-based decision-making. In the model, different discrete boosting levels b are treated as actions. The dACCBoost updates the boost values vB(s, b), via the equation:
(3)
Equation 3 represents the value update of a specific boosting level b in the state s. The ACCBoost submodule probabilistically selects a boosting level based on expected values vB and temperature τ:
(4)
Control over learning rates: LC
The LC module also optimizes the learning rate parameters in the two dACC modules, λ and λB. The optimization of λ and λB solves the trade-off between stability and plasticity, increasing the learning speed when the environment changes and lowering it when the environment is simply noisy. In particular, λ and λB are computed as the ratio between the estimated variance of the state/action-value,
(5a)
To ensure numerical stability, λ ranges in (β, 1) where β is a free positive parameter. The variance is computed as:
(5b)
(5c)
The same low-pass filter is applied to the prediction error signal (δ) to obtain a running estimation of total variance
(5d)
Equations 5a–d are implemented independently for each of the two dACC modules, so that each module interacts with the LC to modulate its own learning rate parameter λ or λB.
VTA
The VTA module provides outcome-related signal DA to both dACC modules, either for action selection directed toward the environment (dACCAct) or for catecholamine boosting-level selection (dACCBoost). The DA signal afferent to the dACCAct is regulated as follows:
(6a)
The DA signal afferent to the dACCBoost is described by the following equation:
(6b)
Control over other brain areas
The RML uses the LC-based control signal (NE) to optimize performance of other modules simulating other brain regions. The dACCAct module implements a decision-making function in a domain-independent way, i.e., the activation of state/action channels can encode any decision process in other brain areas outside dACC. In addition, since optimization of any brain region improves behavioral performance, the dACCBoost can use LC signals to modulate any cortical area to optimize its performance. For example, we simulated a visuo-spatial working memory (WM) task (Simulation 1, below) by connecting the RML to an independently developed and published neural model that represents the fronto-parietal network for visual space representation.
Simulations
We administered to the RML both a visuo-spatial WM task (Simulation 1, Fig. 2a), and a decision-making task involving different levels of effort engagement (Simulation 2, Fig. 2b), in both a condition simulating the VTA degeneration and a control condition. We then compared the simulation results at both the behavioral and the neural levels with those deriving from human studies (see below). To mimic standard experimental paradigms as closely as possible, we repeated each simulation only 20 times (i.e., 20 simulated participants). This verified that the model could generate results with a large effect size (p-values, but not effect sizes, can be improved arbitrarily by running more simulated participants).
Fig.2
a) Delayed matching-to-sample task (Simulation 1). This task was administered to the RML-FROST system (Fig. 1b, c). The task difficulty (WM load) was simulated by varying from 1 to 30 s the delay between sample and target presentation (the figure only shows the case with 10 s). b) Effort task (Simulation 2). The RML chooses between two options (blue and red squares), a high effort option (thick black arrow moving the lever) leading to a large reward (HR option) and a low effort option (thin black arrow moving the lever) leading to a small reward (LR option).
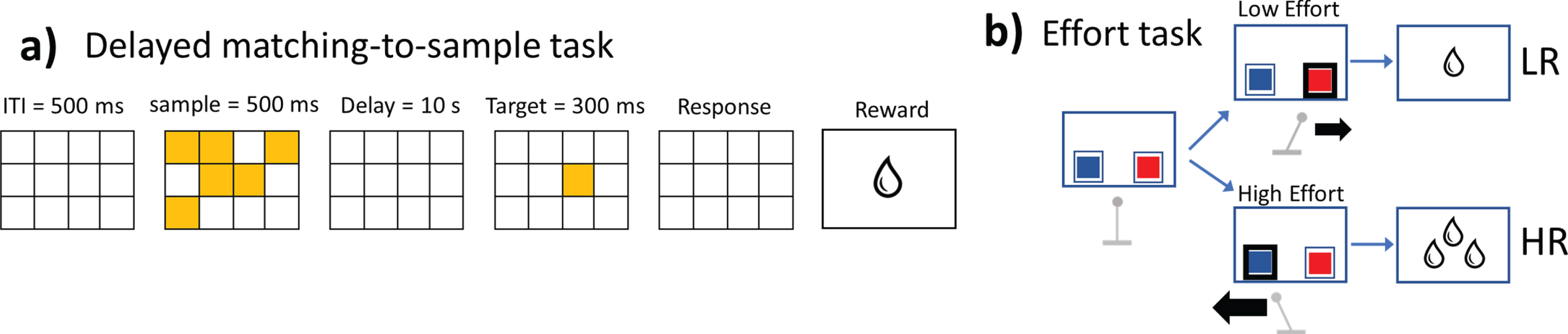
Simulation 1: Working memory impairment
In this simulation, the RML was administered a visuo-spatial delayed matching-to-sample task (Fig. 2a), in both normal and VTA-damaged conditions (DA levels reduced by 60%). In order to simulate this task, we integrated the RML with a recurrent neural network simulating visuo-spatial WM functions: The FROntal-Striatal-Thalamic model (FROST; Fig. 1b, c, [28]; see [25] for a mathematical description of this model and how it is connected to the RML). The FROST model is a recurrent neural network with frontal neurons (F) and parietal neurons (P), reciprocally interconnected and simulating visuo-spatial WM. The RML model is connected to the FROST model so that the dACC module can select one of the items retained in the F network, while the LC modulates F neurons activity (Fig. 1b, c). During the delayed matching-to-sample task (Fig. 2a), a pattern of visual items (stimuli) was presented inside the receptive fields of P neurons then a target item was presented after a delay. During the delay, spatial information was retained in F neurons thanks to the recurrent F-P activity. The RML read out the representations in the F layer of the FROST model and made a decision on whether the target matched or not one of the previously presented stimuli. Importantly, the RML also played a role of optimizer of the FROST performance as a function of memory load. This optimization process was implemented by gain modulation of F neurons by the NE signal afferent from the LC module (orange circuit in Fig. 1b, c), which increased the coding precision of spatial locations retained in F neurons. This mechanism simulates the effect of NE on prefrontal neurons [29]. One of the main features of the FROST model is that it can simulate the decrease of memory precision as a function of memory load, so that the higher the number of items to be retained in memory, the lower is the precision of their representation in its F cortical neurons (Fig. 1b). In this simulation, we also implemented the detrimental effect of the time delay on memory precision by decreasing the activity from the frontal cortex F as a function of time. We implemented this by making the gating signal A from Equation S20 in Silvetti et al. [25] decaying as a function of time:
The delayed matching-to-sample task implied different memory loads, namely a template of 4 items to be retained for 1, 10, or 30 s (Fig. 3a). We used a block design where we administered three blocks of 70 trials, each with one specific memory load. In 50% of trials, the probe matched with the template.
The statistical analysis of the results was conducted by a repeated measure 3×2 ANOVA (memory load by DA lesion). We estimated three design matrices related to three dependent variables, the first related to accuracy, the second related to reaction times (RTs), and the third related to the LC boosting by the dACCBoost module. The results were qualitatively compared with experimental results from both human controls and patients from [30]. Plot data from [30] were extracted through WebPlotDigitizer from Fig. 5 of the original article.
Fig.3
Neural and behavioural results from Simulation 1 (a–c), compared with experimental data from humans (d, e), related to the performance of MCI and control participants in a delay memory test. Blue plots indicate human or simulated controls, while red plots indicate human or simulated patients. a) LC module activation (arbitrary units, a.u.) as a function of task difficulty (delay duration). Error bars indicate s.e.m. b) Response accuracy as a function of difficulty (±s.e.m). c) RTs as a function of task difficulty (±s.e.m). d) Response accuracy in human participants (from [30]; number of recalled items±s.e.m) in a similar WM task. e) RTs in human participants (±s.e.m) from the same data set [30].
![Neural and behavioural results from Simulation 1 (a–c), compared with experimental data from humans (d, e), related to the performance of MCI and control participants in a delay memory test. Blue plots indicate human or simulated controls, while red plots indicate human or simulated patients. a) LC module activation (arbitrary units, a.u.) as a function of task difficulty (delay duration). Error bars indicate s.e.m. b) Response accuracy as a function of difficulty (±s.e.m). c) RTs as a function of task difficulty (±s.e.m). d) Response accuracy in human participants (from [30]; number of recalled items±s.e.m) in a similar WM task. e) RTs in human participants (±s.e.m) from the same data set [30].](https://content.iospress.com:443/media/jad/2020/77-1/jad-77-1-jad200276/jad-77-jad200276-g003.jpg)
Simulation 2: Motivational impairment in effort engagement tasks
In Simulation 2, we show how cortical-subcortical interactions between the dACC, VTA, and LC can drive optimal decision-making when effortful choices leading to large rewards (HR choice) compete with low effort choices leading to smaller rewards (LR choice) [31, 32]. To this purpose, we tested the RML with an experimental paradigm involving decision-making in effortful tasks where cost/benefit trade off must be optimized (Fig. 2b, see also Silvetti et al. [25]). Although this task is represented in Fig. 2b as involving physical effort (inspired from effort tasks in rodents [31]), from the computational viewpoint, it represents a class of tasks involving both physical or cognitive effort, as the central neuro-computational mechanisms subtending effort-based decision-making are shared across such different modalities. In Simulation 2a, we tested how a progressive VTA degeneration influences effort-based decision making and the subtending neural processes. We varied parametrically the entity of the VTA lesion and recorded, as dependent variables, the percentage of times in which the RML selected the optimal choice (HR), the percentage of times it refused to engage in the task (“Stay” choice), and the boosting signal generated by the dACCBoost module to modulate VTA and LC activity. The VTA degeneration was simulated by multiplying the DA signal (red circuit in Fig. 1a) by a real number within the interval 0.1 (90% of impairment) and 1 (no impairment). Costs for motor action (C in Equation 2) in this simulation were respectively 7 for the HR choice and 0.5 for the LR choice. Results were qualitatively compared with experimental results from both human controls and patients from [30]. Plot data from [30] were extracted by means of WebPlotDigitizer, respectively from Figs. 2 and 3 of the original articles. In Simulation 2b, we compared the LC activation as a function of three effort levels for HR choice (C values in Equation 2 were respectively 2, 5, and 40) in three different groups of simulated patients (Controls, mild cognitive impairment, and early AD), modeled by three levels of VTA impairment (respectively 0%, 50%, 90%). Also in this case, simulation results were qualitatively compared with experimental results from [30]. Plot data from [30] were extracted by means of WebPlotDigitizer software from Fig. 2 of the original article.
Simulation of VTA lesion
The VTA lesion was implemented by multiplying the output of the VTA module in the RML by a number in the range of (0, 1), for example a 60% VTA degeneration implied to multiply the VTA output (DA and DAB signals) by 0.4. For Simulation 1 (WM impairment), we used the dynamical version of the RML [25] and simulated the cortical-subcortical activity with a time resolution of 10 ms. In Simulation 2 (motivational impairment) we used the discrete version of the RML.
RESULTS
The reinforcement meta-learner model: A qualitative description
We investigated the consequences of dopaminergic neurons loss on catecholamine release, and thence on behavior, by using the Reinforcement Meta-Learner (RML) computational model [25]. This model describes the dynamical interactions between the catecholamine brainstem nuclei (LC and VTA) and the dorsal anterior cingulate cortex (dACC), simulating the optimal regulation of catecholamines release as a function of environmental conditions. The architecture of the model pivots on the biologically grounded idea that behavioral adaptation can be seen as a problem of optimization [33, 34] whose objective is to maximize the long-term reward while minimizing costs. In order to do so, animals need to learn not only how to optimally control their behavior but also how to control the internal variables that influence brain processes. For example, a foraging rat makes decisions about its movements in the environment and, at the same time, controls internal variables linked to activity engagement, to how much energy to spend in action performance (cost/benefit trade-off), and whether to take into account or not eventual changes in the surrounding environment (plasticity/stability trade-off) [35]. Learning to optimally control these internal variables is defined as meta-learning [36, 37].
The RML is built on the hypothesis that the mammalian brain performs meta-learning by optimizing the release of neuromodulators [38] as a function of task demands and expected rewards. Although this idea could be extended to any neuromodulator, for parsimony we decided to model the control of catecholamines (DA, and NE), a class of neuromodulators having strong and immediate influence on cognition and behavior. In our model, the release of catecholamines is regulated by a cortical-subcortical macro-circuit including the brainstem catecholamine nuclei (VTA and LC), the dACC, and their reciprocal connections [39–41]. The RML architecture (Fig. 1a) is based on two interacting loops connecting four computational modules: dACCAct, dACCBoost, VTA, and LC. An internal loop manages the interaction between the brainstem nuclei VTA and LC and the dACC modules (Fig. 1a, orange and red bidirectional arrows), whereas an external loop manages the interaction between the cortical modules and the external environment. The RML is aimed at minimizing both the costs of neuromodulators release (internal loop), and the costs of motor actions (external loop; e.g., the metabolic cost of climbing a stair) while optimizing performance (i.e., maximizing reward). In other words, we can describe the RML as an optimizer whose objective is to maximize the reward discounted by costs.
Although dACCAct and dACCBoost work in parallel and have the same objective of maximizing net reward, they are responsible respectively for value-based action selection (dACCAct part of the external loop in Fig. 1, Equation 1,2) and the modulation of LC and VTA activity (that is, the “boost”; dACCBoost is part of the internal loop in Fig. 1a, Equation 3, 4). Catecholamines affect the dynamics of the dACC modules in real time, i.e., while the model is interacting with the environment. In particular, they modulate the amount of cognitive and physical effort (by LC module, Equation 2) [42, 43] that the model exerts to execute a task, its plasticity for knowledge updating (learning rate, by the LC module, Equation 5a), and the magnitude of reward signals (by VTA module, Equation 6a,b). In agreement with recent experimental findings, the RML architecture assumes that, while DA influences effort-based decision-making, NE is directly involved in effort regulation [44]. The RML can be integrated with other computational models, e.g., to a WM model (see Simulation 1 and Fig. 1b, c), so that the efferent signal from the LC can modulate the dynamics of other simulated brain regions and optimize their performance [29] (Fig. 1a, b, orange arrows). Importantly (Simulation 1), the cortical-subcortical system modeled by the RML is strongly involved in the management of the WM functioning in primates. Indeed, neurophysiological findings have shown that LC neuromodulatory signal critically affects WM by improving coding precision in prefrontal neurons [45, 46], while a deficit in NE transmission in prefrontal neurons leads to WM impairment [47, 48]. Moreover, neuroimaging findings demonstrated an increase of dACC activity as a function of effort in WM tasks [49, 50]. Finally, it is worth stressing that the RML implements WM optimization as a form of cognitive effort, assuming that the VTA influences WM performance indirectly, by influencing the dACC function, which, in turn, modulates LC activity. For these reasons, and because LC activation is a major biological marker of both cognitive and physical effort [51, 52], the RML architecture is well suited to simulate how VTA degeneration interacts with LC-dependent effort in both physical and cognitive tasks.
The equations regulating the RML functioning are presented and discussed in the “Materials and Methods” section above (see [25]).
Simulation of two behavioral tasks
We disrupted the DA transmission in the RML to simulate VTA degeneration during the early stages of AD progression. While manipulating DA levels, we investigated the RML performance and dynamics in two domains that are particularly sensitive to the early stages of AD progression, i.e., WM and motivation to exert effort to get a reward. We investigated these domains by administering to the RML both a visuo-spatial WM task (Fig. 2a) and a decision-making task involving different levels of effort engagement (effort, Fig. 2b), while manipulating different levels of VTA degeneration. All the parameters and the task features used in these simulations are the same as those used in Silvetti et al. [25]. Simulation results were compared with data from human patients in early stages of AD progression.
Simulation 1: Working memory impairment
WM impairment is one of the earliest signs of AD. In this simulation we show how VTA degeneration can determine WM impairment by disrupting the functioning of the dACC, LC and VTA system. To reproduce WM dysfunctions the RML model was connected to the FROST model, a recurrent neural network simulating the fronto-parietal circuits involved in visuo-spatial WM [28] (Fig. 1b, c). As we show in Fig. 3a, the LC output increased as a function of delay duration (blue plot; main effect of memory load on LC output in controls: F(2,19) = 3,91, p = 0.0286), keeping the accuracy high (Fig. 3b, blue plot) and RTs low across different working memory loads (Fig. 3c, blue plot), as it happens also in healthy humans [30].
In case of VTA degeneration, both the RML-FROST behavior and its neural dynamics are impaired. At the behavioral level, this causes poor accuracy due to the necessity of high NE levels for this task (Fig. 3b, red plot; lesion main effect: F(1,19) = 22.15, p < 0.0001). Also the RTs worsened with VTA degeneration (Fig. 3c, red plot; lesion main effect: F(1,19) = 15.76, p < 0.0001). This was due to the consequent disruption of VTA-dACC-LC interaction, with the effect, at the neural level, of downregulating LC activity (Fig. 3a, red plot; main effect of DA lesion on LC output: F(1,19) = 19.37, p < 0.0001). This happened especially for high memory loads (lesion x memory-load interaction: F(2,38) = 4.93, p = 0.0125). In Fig. 3d and 3e, we show also that the simulated behavioral results are in agreement with those from human patients with mild cognitive impairment due to AD exhibiting a significant group effect and delay effect [30].
Simulation 2: Motivational impairment in effort engagement tasks
In this task, the RML could choose between high effort-high reward and low effort-low reward options. Although this experimental paradigm takes inspiration from rodent studies [31, 32, 44], where effort levels were manipulated in terms of physical effort, here it must be interpreted as a task involving indifferently either physical or cognitive effort, because, in the RML, the central neuro-computational mechanisms basing effort-based decision-making are shared across different effort modalities. Both human and nonhuman animal studies indicate that catecholamines are crucial to energize behavior and choose effortful actions when a large reward is available [31, 32]. Simulation results from this experimental paradigm are compared with those from patients performing a verbal short-term memory task (digit span).
Simulation 2a
Figure 4 shows both behavioral and neural results from the RML, as a function of progressive VTA degeneration, and compares them with those from human volunteers executing a task involving cognitive effort (digit span). At the behavioral level, when the VTA was intact, the RML exhibited an optimal behavior, preferring to exert an effort to get a large reward (Fig. 4a, 0% VTA degeneration). With the progression of the VTA degeneration, behavioral performance remained at first stable and then, when degeneration approached 50%, it dropped quickly. The percentage of trials where the RML refused to execute the task had similar dynamics. During the early VTA degeneration, the model kept a good level of commitment, followed by a rapid emergence of apathetic behavior (many refusals to engage) when the degeneration progressed (Fig. 4a, inner plot). The same performance pattern has been found in AD patients tested with a digit span task [53] every six months: here performance remained stable during the very early stages of the disease (first two assessments, at 0 and 6 months) and became significantly poorer from the third assessment (from 12 months) (Fig. 4b). At the neural level, this behavioral pattern emerges from the MPFC-brainstem adaptation to the VTA degeneration. Figure 4c shows an increase of LC activity, peaking at about 40% of VTA lesion, and dropping with further VTA degeneration (inverted U dynamics). This LC response was due to a compensatory mechanism where, during the initial stages of VTA degeneration, the dACCBoost compensated the drop of reward signal from the VTA by increasing the boosting signal promoting the release of both NE and DA. This compensatory mechanism can be interpreted in terms of the classic physiology concept of functional reserve. Afterwards, LC activity decreased, as the residual VTA output (conveying reward signal) was not enough to overcome the intrinsic cost of catecholamine boosting anymore. It is worth noting that with a severe VTA degeneration the LC output dropped below the baseline corresponding to an intact VTA, leading to a chronic downregulation of NE release, despite the LC was not lesioned. This result closely reproduces the LC activation (measured by pupil dilation [44, 54]) during a digit span task in human participants affected by different disease severities, showing a significant group effect of different cognitive impairments [55] (Fig. 4d).
Fig.4
Model behaviour and neural dynamics (±s.e.m.) in the execution of the Effort Task as a function of a progressive VTA lesion (left column), and comparison with human data (right column). a) At the behavioral level, the percentage of simulated optimal choices (HR) first decreased slowly and then dropped abruptly with the VTA damage progression. The inner plot shows the percentage of “Stay” choices (refusal to engage in the task): this remained low during the early stages of VTA degeneration, and increased quickly afterwards. b) Behavioral performance of AD patients at different assessment time points. This is in agreement with the simulation in panel ‘a’ (data from [53]) where performance remains good during the early disease stages and then it drops quickly. c) At the neural level, simulated LC response (arbitrary units, a.u.) followed an inverted U shape when VTA was progressively lesioned. With low VTA damage, the dACCBoost module compensated by promoting catecholamine release. As LC was not lesioned, this resulted in an over-activation that compensated VTA degeneration and kept behavioral performance stable (a, b); with a more severe VTA lesion, the LC under-activated, leading to abrupt performance drop (a, b). d) The same pattern was found in patients with progressive cognitive impairment [55] (data averaged over the effort dimension from panel f; MCI, mild cognitive impairment). Pupil dilation is used as a proxy for LC activation, see main text). e) LC simulated activation as a function of task difficulty (number of digits), in three different simulated populations: controls, MCI, and Early-AD. f) Pupil dilation (a proxy of LC activity) as a function of task difficulty (number of digits), in human control and patient groups (data from [55]).
![Model behaviour and neural dynamics (±s.e.m.) in the execution of the Effort Task as a function of a progressive VTA lesion (left column), and comparison with human data (right column). a) At the behavioral level, the percentage of simulated optimal choices (HR) first decreased slowly and then dropped abruptly with the VTA damage progression. The inner plot shows the percentage of “Stay” choices (refusal to engage in the task): this remained low during the early stages of VTA degeneration, and increased quickly afterwards. b) Behavioral performance of AD patients at different assessment time points. This is in agreement with the simulation in panel ‘a’ (data from [53]) where performance remains good during the early disease stages and then it drops quickly. c) At the neural level, simulated LC response (arbitrary units, a.u.) followed an inverted U shape when VTA was progressively lesioned. With low VTA damage, the dACCBoost module compensated by promoting catecholamine release. As LC was not lesioned, this resulted in an over-activation that compensated VTA degeneration and kept behavioral performance stable (a, b); with a more severe VTA lesion, the LC under-activated, leading to abrupt performance drop (a, b). d) The same pattern was found in patients with progressive cognitive impairment [55] (data averaged over the effort dimension from panel f; MCI, mild cognitive impairment). Pupil dilation is used as a proxy for LC activation, see main text). e) LC simulated activation as a function of task difficulty (number of digits), in three different simulated populations: controls, MCI, and Early-AD. f) Pupil dilation (a proxy of LC activity) as a function of task difficulty (number of digits), in human control and patient groups (data from [55]).](https://content.iospress.com:443/media/jad/2020/77-1/jad-77-1-jad200276/jad-77-jad200276-g004.jpg)
Simulation 2b
In Fig. 4e, we show how simulated mild cognitive impairment patients compensated VTA degeneration by increasing LC activation, while, in case of severe VTA damage (Early-AD), the LC activation dropped below the controls’ level (main effect of VTA degeneration: F(2,38) = 128.04, p < 0.00001). In case of severe VTA degeneration, the LC activation became no more responsive to effort (green plot in Fig. 4e; effort x VTA damage interaction: F(4,66) = 61.02, p < 0.00001). The same results have been documented in patients (Fig. 4f), showing both a main effect of group and an interaction group x memory load [30]. In both RML and humans, when the task demand became overwhelming the LC activation decreased (third effort level in Fig. 4e, f), regardless of the presence of pathology. In the RML, this is due to catecholamines release optimization, which leads to a neuromodulatory downregulation once the task becomes too hard.
DISCUSSION
Influence of VTA degeneration on LC modulation
Simulations 1 and 2 show how VTA degeneration disrupts the cortical-subcortical processes regulating catecholamine release as a function of environmental demand. In particular, the model suggests that VTA degeneration alters the control of LC in a nonlinear way. In the early stages of VTA degeneration, the dACC promotes catecholamine release, resulting in a partial VTA compensation and a higher (than normal) level of NE. LC temporary compensation defers performance drop (as described above, Fig. 4a, b) and it also counteracts the tendency of refusing to engage in the task (Fig. 4a), which can be interpreted as a resilience to apathy. With the progression of the VTA degeneration, NE level undergoes an “inverted U shape” dynamics leading to an initial increase of the LC activation followed by a chronic depression (Fig. 4c). The RML model allows an interpretation of this phenomenon as due to the continuous updating of the optimal point in the tradeoff between catecholamine upregulation (directed to preserve behavioral performance) and catecholamine downregulation (directed to save the cost of catecholamine release); however, in humans also the progressive degeneration of the LC itself may play a role, as proved in patients and AD mouse models [56–58]. The initial upregulation of catecholamines was found in patients at initial stages of AD, when the neuropsychological deficit emerges from clinical testing but is still not evident in everyday life, as patients can maintain a normal performance at the cost of a higher effort [55]. Moreover, the “inverted U shape” LC compensation, together with the progressive LC neurodegeneration, could explain why experimental data show either an increase or a decrease of NE levels in AD patients [59]. Indeed, based on our simulations, the LC output could augment or decrease depending on the disease progression [60]. These results are also in line with recent evidence from middle-aged cognitive normal adults indicating that task-evoked pupillary responses, revealing an LC-noradrenaline involvement, could be associated with AD polygenic risk scores (AD-PRSs) providing information for early screening of genetically at-risk individuals. In particular, in a digit span task, individuals with normal cognitive functions but high AD-PRSs exhibited a greater pupil dilation (effort) in a high (9-digit) cognitive load condition [61]. Figure 5 summarizes these processes [60].
Fig.5
Schema summarizing the interaction between dACC modules and catecholamine modules in the RML, during the progressive degeneration of VTA. Coronal brain sections indicating the possible presence of diffusive degeneration. a) In normal conditions, dACC modules make decisions and optimize catecholamines release to maximize performance (i.e., reward). b) In case of mild VTA degeneration, the dACC can compensate the VTA loss (DA: dopamine), by upregulating the boosting signal (functional reserve, ticker blue downward arrows). This compensates only partially the VTA degeneration (due to neural cells loss), but increases strongly the LC (NE: norepinephrine) output as the LC is not impaired, at least during early stages of the disease. This keeps behavioural performance close to normal, at least for easy tasks, but with a higher recruitment of catecholamines. c) When the VTA degeneration is severe the cost for compensation is too high, and therefore the dACC decreases the boosting signal (thinner downward arrows), causing a downregulation of both LC and an even stronger VTA output decrease. This causes the behavioural deficits described in Figs. 2 and 3, including the WM impairment due to the loss of LC function. d) Chronic catecholamines down-regulation leads to severe cognitive impairments and possibly to a lower clearance of Aβ peptide in LC target areas, thus contributing to the disease progression toward the plaque stage.
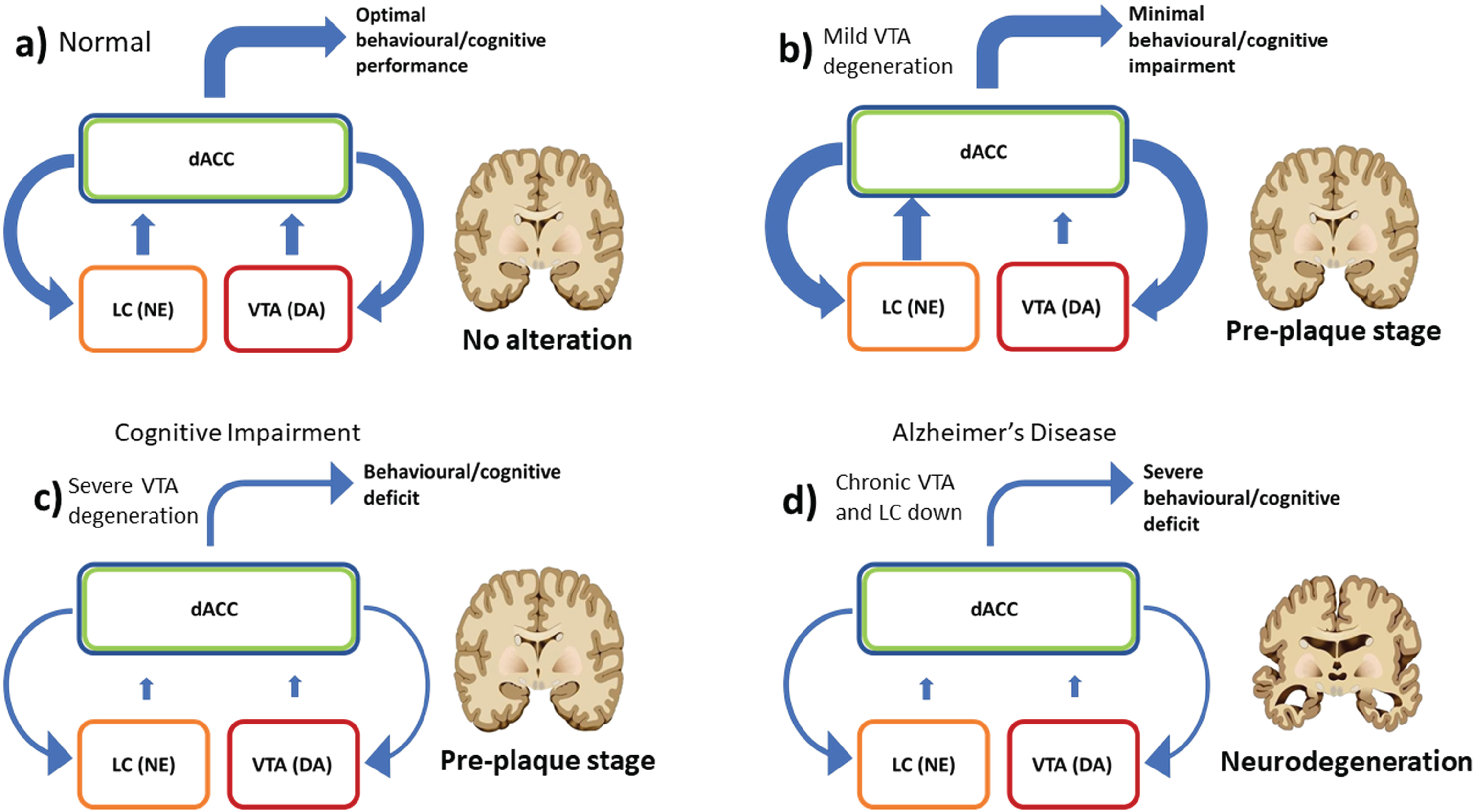
The simulations also show how the downregulation of both DA and NE following severe VTA degeneration causes the emergence of apathy, with a decreased willingness to perform a task (Fig. 4a). The model explains the emergence of apathy as related not only to the loss of the VTA function but also to the disruption of catecholamine control including NE and due to a primary VTA deficit (Fig. 5c, d).
The progressive catecholaminergic dysfunction associated with the degeneration of VTA and LC, and the resulting behavioral effects, agree with the experimental observations in rodent models [62–64]. Here experimental lesions in VTA produce altered locomotor activity, deficits in passive avoidance learning, and aggressive behavior, with all these effects often related to the size of the VTA lesion [62–64]. However, it should be considered that the behavioral alterations resulting from a focused damage of the VTA might differ from those resulting from a progressive degeneration involving a subset of dopaminergic VTA neurons as in AD. Moreover, a progressive degeneration of VTA neurons could gradually spread to other vulnerable brain areas such as LC, as demonstrated in the Tg2576 AD mouse model [20, 56].
Chronic LC downregulation as a risk factor of neurodegeneration
The simulations described above show how VTA degeneration might affect the modulation of catecholamine nuclei by the midfrontal cortex, up to a chronic downregulation of both DA and NE. In the long term, catecholamine downregulation might trigger a cascade of events leading to a stronger manifestation of the disease features. In particular, chronic depression of LC neurons activity contributes to producing abnormal (hyperphosphorylated) tau proteins in LC neurons [18] themselves, which can aggregate into neurofibrillary tangles [14, 17] and lead to permanent LC degeneration. The persistent lower NE produced by the loss of LC neurons has been associated with a lower clearance of Aβ peptide in LC target areas [65, 66], including amygdala (Amg) [67], hippocampus (Hip) [68], and PFC [69, 70]. This leads to an accumulation of Aβ plaques, leading to the AD-typical diffuse cortical atrophy [71] and a worsening of clinical symptoms (Fig. 5d). Aside from contributing to tauopathy and Aβ plaque formation, the decreased level of DA and NE might directly cause dysfunctions in the VTA and LC target areas [72–74], like Amg, Hip, and PFC, which are critically involved in AD development.
In the long run, the chronic malfunctioning of the VTA-LC system might also affect the functioning of NbM which receives dopaminergic input from VTA and noradrenergic input from LC, and provides the principal source of acetylcholine for PFC, Amg, and Hip [75–77]. At the early stages of pathology, most NbM neurons are unaffected by tauopathy and many of the affected neurons remain alive [78]. In this case, the AD symptoms might be mainly due to the processes discussed above, related to the effects of the VTA-LC malfunctioning on the cortical and limbic areas where they project. The prolonged malfunctioning of the VTA-LC system might increase the vulnerability to tauopathy and neurofibrillary degeneration of the cholinergic component of the NbM. Once developed, the tauopathy in these components might lead to the degeneration of the cholinergic axons, leading to the worsening of symptoms [79].
This discussion about the long-term effects of the catecholamine-frontal dysfunctions on a wider cortical-subcortical network is important for two reasons. Firstly, it provides a system-level perspective linking early catecholamine dysfunction to the typical histological findings in AD, together with the mechanistic description of cognitive impairment progression. This system-level perspective could be critical to develop better diagnostic approaches leading to address the challenges in AD research related to the lack of effective preventive strategies and reliable biomarkers for early diagnosis of the disease [80–82]. Secondly, it is critical to devise new therapies. AD is currently incurable and existing treatments produce only a modest and transient amelioration of symptoms [83]. To date, acetylcholinesterase inhibitors and memantine are the only drugs approved for its management. These drugs provide a symptomatic improvement but have a minor impact on the disease progression. The extensive investigation of the molecular and cellular pathogenesis in AD over the past few decades has provided significant progress in the understanding of the disease, stimulating the development of a number of novel strategies that seek to modify the disease progression. The major developments in this direction are the amyloid and tau-based therapeutics [84]. Aside from these approaches, the increased understanding of the mechanisms underlying AD pathogenesis and progression could also lead to the development of complementary therapeutic strategies. In this line, the results obtained in this article suggest that therapies involving also drugs enhancing DA transmission in early stages of the disease could provide better results with respect, for example, to drugs acting on norepinephrine alone that have been shown to give mixed results [17]. This proposal agrees with data supporting a role of dopaminergic drugs in AD therapy [85]. In particular, electrophysiological studies performed on AD patients showed positive effects of dopaminergic drugs on cortical synaptic plasticity mechanisms and on cognitive performances, suggesting the use of these drugs in the treatment of the disease [86–88].
In addition, the results of the simulations run with the model also suggest the investigation of the possible benefits of employing psychological interventions alongside drug-based treatments. In this respect, we have seen that the model introduces a subjective factor weighting the objective relevance of both rewards and costs received by the patient in daily life activities. This suggests the possibility of psychological interventions directed to lower the perceived subjective estimation of costs and increase the subjective estimation of gains to increase the probability of decisions in favor of coping and engagement. In turn this would lead to harvest the physiological benefits discussed above produced by enhanced catecholamine release. This view agrees with recent proposals to employ psychological interventions to treat depression and apathy symptoms as a way to contribute to prevent and treat AD [89, 90]
The hypothesis proposed in this paper agrees with the system-level perspective to the study of the brain according to which different behaviors are produced by the interplay of different parts of the brain rather than by specific areas working in isolation [91–94]. This view is in line with recent works proposing to study AD as a system-level disorder where various protective, age-related, and disease-promoting factors interact to produce the core mechanisms underlying the disease [80, 95, 96]. Recently, we have shown how this system-level approach could be critically used to explain in new ways the origin of different cortical-cerebellar-basal ganglia dysfunctions related to Tourette’s syndrome [97], ADHD [98], and Parkinson’s disease [99]. Similarly, in this article we have argued that a system-level approach could lead to view the AD early pathogenesis and development under a different perspective.
Concluding remarks
Both behavioral and neural data simulated by the model were consistent with recent experimental observations in patients and, thanks to the anatomo-functional plausibility of the RML, it was possible to formulate specific hypotheses on the neural mechanisms linking the VTA impairment to the disease progression and to the altered behavior. In this way it was possible to associate the neural-scale to the behavioral-scale features making the model suitable for: 1) quantify system-level dynamics through computer simulations, and bind them to the neural processes underlying AD pathogenesis at pre-plaques stage; 2) contribute to formulate a novel, integrated, view of the pathogenesis of AD, which might help to devise new computer simulation-based early diagnostics, for example by means of computational phenotyping techniques [100], and then new therapeutic interventions targeting the very early stages of the disease.
ACKNOWLEDGMENTS
MDA is financially supported by grants from Alzheimer’s Association, United States, (AARG-18-566270), and from Italian Ministry of Health (RF-2018-12365527). MS was funded from the European Union’s Horizon 2020 research and innovation programme under the Marie Skłodowska-Curie Grant Agreement No. 795919. GB received funds from the European Union’s Horizon 2020 Research and Innovation Programme, under grant agreement no 713010, project “GOAL-Robots - Goal-based Open-ended Autonomous Learning Robots”. GB dedicates his contribution to this work to his father Giuseppe, who was recently killed by Alzheimer’s disease after demanding life vicissitudes and depression.
Authors’ disclosures available online (https://www.j-alz.com/manuscript-disclosures/20-0276r2).
REFERENCES
[1] | Scheltens P ((2000) ) Aspects of Alzheimer’s disease. Lancet 355: , 1920. |
[2] | Waldemar G , Burns A ((2017) ), Alzheimer’s disease. Oxford University Press. |
[3] | Hardy J , Higgins G ((1992) ) Alzheimer’s disease: The amyloid cascade hypothesis. Science 256: , 184–185. |
[4] | Binder LI , Guillozet-Bongaarts AL , Garcia-Sierra F , Berry RW ((2005) ) Tau, tangles, and Alzheimer’s disease. Biochim Biophys Acta 1739: , 216–223. |
[5] | Hasselmo ME ((2006) ) The role of acetylcholine in learning and memory. Curr Opin Neurobiol 16: , 710–715. |
[6] | He Y , Zhu J , Huang F , Qin L , Fan W , He H ((2014) ) Age-dependent loss of cholinergic neurons in learning and memory-related brain regions and impaired learning in SAMP8 mice with trigeminal nerve damage. Neural Regeneration Res 9: , 1985–1994. |
[7] | Maurer SV , Williams CL ((2017) ) The cholinergic system modulates memory and hippocampal plasticity its interactions with non-neuronal cells. Front Immunol 8: , 1489. |
[8] | Whitehouse P , Price D , Struble R , Clark A , Coyle J , Delon M ((1982) ) Alzheimer’s disease and senile dementia: Loss of neurons in the basal forebrain. Science 215: , 1237–1239. |
[9] | Pinto T , Lanctôt KL , Herrmann N ((2011) ) Revisiting the cholinergic hypothesis of behavioral and psychological symptoms in dementia of the Alzheimer’s type. Ageing Res Rev 10: , 404–412. |
[10] | Burns JM , Galvin JE , Roe CM , Morris JC , McKeel DW ((2005) ) The pathology of the substantia nigra in Alzheimer disease with extrapyramidal signs. Neurology 64: , 1397–1403. |
[11] | Gibb WR , Mountjoy CQ , Mann DM , Lees AJ ((1989) ) The substantia nigra and ventral tegmental area in Alzheimer’s disease and Down’s syndrome. J Neurol Neurosurg Psychiatry 52: , 193–200. |
[12] | Storga D , Vrecko K , Birkmayer JG , Reibnegger G ((1996) ) Monoaminergic neurotransmitters, their precursors and metabolites in brains of Alzheimer patients. Neurosci Lett 203: , 29–32. |
[13] | Martorana A , Koch G ((2014) ) “Is dopamine involved in Alzheimer’s disease?”. Front Aging Neurosci 6: , 252. |
[14] | Weinshenker D ((2018) ) Long road to ruin: Noradrenergic dysfunction in neurodegenerative disease. Trends Neurosci 41: , 211–223 . |
[15] | Bondareff W , Mountjoy CQ , Roth M , Rossor MN , Iversen LL , Reynolds GP , Hauser DL ((1987) ) Neuronal degeneration in locus ceruleus and cortical correlates of Alzheimer disease. Alzheimer Dis Assoc Disord 1: , 256–262. |
[16] | Šimić G , Babić Leko M , Wray S , Harrington CR , Delalle I , Jovanov-Milošević N , Bažadona D , Buée L , de Silva R , Di Giovanni G , Wischik CM , Hof PR ((2017) ) Monoaminergic neuropathology in Alzheimer’s disease. Prog Neurobiol 151: , 101–138. |
[17] | Mather M , Harley CW ((2016) ) The locus coeruleus: Essential for maintaining cognitive function and the aging brain. Trends Cogn Sci 20: , 214–226. |
[18] | Braak H , Thal DR , Ghebremedhin E , Del Tredici K ((2011) ) Stages of the pathologic process in Alzheimer disease: Age categories from 1 to 100 years. J Neuropathol Exp Neurol 70: , 960–969. |
[19] | Henstridge CM , Hyman BT , Spires-Jones TL ((2019) ) Beyond the neuron-cellular interactions early in Alzheimer disease pathogenesis. Nat Rev Neurosci 20: , 94–108. |
[20] | Nobili A , Latagliata EC , Viscomi MT , Cavallucci V , Cutuli D , Giacovazzo G , Krashia P , Rizzo FR , Marino R , Federici M , De Bartolo P , Aversa D , Dell’Acqua MC , Cordella A , Sancandi M , Keller F , Petrosini L , Puglisi-Allegra S , Mercuri NB , Coccurello R , Berretta N , D’Amelio M ((2017) ) Dopamine neuronal loss contributes to memory and reward dysfunction in a model of Alzheimer’s disease. Nat Commun 8: , 14727. |
[21] | Ito R , Hayen A ((2011) ) Opposing roles of nucleus accumbens core and shell dopamine in the modulation of limbic information processing. J Neurosci 31: , 6001–6007. |
[22] | De Marco M , Venneri A ((2018) ) Volume and connectivity of the ventral tegmental area are linked to neurocognitive signatures of Alzheimer’s disease in humans. J Alzheimers Dis 63: , 167–180. |
[23] | Serra L , D’Amelio M , Di Domenico C , Dipasquale O , Marra C , Mercuri NB , Caltagirone C , Cercignani M , Bozzali M ((2018) ) In vivo mapping of brainstem nuclei functional connectivity disruption in Alzheimer’s disease. Neurobiol Aging 72: , 72–82. |
[24] | Iaccarino L , Sala A , Caminiti SP , Presotto L , Perani D , for the Alzheimer’s Disease Neuroimaging Initiative ((2020) ) In vivo MRI structural and PET metabolic connectivity study of dopamine pathways in Alzheimer’s disease. J Alzheimers Dis 75: , 1003–1016. |
[25] | Silvetti M , Vassena E , Abrahamse E , Verguts T ((2018) ) Dorsal anterior cingulate-brainstem ensemble as a reinforcement meta-learner. PLoS Comput Biol 14: , e1006370. |
[26] | Multani N , Anor CJ , Tang-Wai DF , Keren R , Fox S , Lang AE , Marras C , Tartaglia MC ((2016) ) Functional connectivity of the anterior cingulate cortex in Alzheimer’s disease, Parkinson’s disease and frontotemporal dementia. Alzheimers Dement 12: , P922. |
[27] | Kalman RE ((1960) ) A new approach to linear filtering and prediction problems. J Basic Eng 82: , 35. |
[28] | Ashby FG , Ell SW , Valentin VV , Casale MB ((2005) ) FROST: A distributed neurocomputational model of working memory maintenance. J Cogn Neurosci 17: , 1728–1743. |
[29] | Aston-Jones G , Cohen JD ((2005) ) An integrative theory of locus coeruleus-norepinephrine function: Adaptive gain and optimal performance. Annu Rev Neurosci 28: , 403–450. |
[30] | Alescio-Lautier B , Michel BF , Herrera C , Elahmadi A , Chambon C , Touzet C , Paban V ((2007) ) Visual and visuospatial short-term memory in mild cognitive impairment and Alzheimer disease: Role of attention. Neuropsychologia 45: , 1948–1960. |
[31] | Salamone JD , Cousins MS , Bucher S ((1994) ) Anhedonia or anergia? Effects of haloperidol and nucleus accumbens dopamine depletion on instrumental response selection in a T-maze cost/benefit procedure. Behav Brain Res 65: , 221–229. |
[32] | Walton ME , Groves J , Jennings KA , Croxson PL , Sharp T , Rushworth MFS , Bannerman DM ((2009) ) Comparing the role of the anterior cingulate cortex and 6-hydroxydopamine nucleus accumbens lesions on operant effort-based decision making. Eur J Neurosci 29: , 1678–1691. |
[33] | Frank MJ ((2004) ) By carrot or by stick: Cognitive reinforcement learning in parkinsonism. Science 306: , 1940–1943. |
[34] | Rushworth MFS , Behrens TEJ ((2008) ) Choice, uncertainty and value in prefrontal and cingulate cortex. Nat Neurosci 11: , 389–397. |
[35] | Muller TH , Mars RB , Behrens TE , O’Reilly JX ((2019) ) Control of entropy in neural models of environmental state. Elife 8: , e39404. |
[36] | Schweighofer N , Doya K ((2003) ) Meta-learning in reinforcement learning. Neural Netw 16: , 5–9. |
[37] | Wang JX , Kurth-Nelson Z , Kumaran D , Tirumala D , Soyer H , Leibo JZ , Hassabis D , Botvinick M ((2018) ) Prefrontal cortex as a meta-reinforcement learning system. Nat Neurosci 21: , 860–868. |
[38] | Doya K ((2002) ) Metalearning and neuromodulation. Neural Netw 15: , 495–506. |
[39] | Devinsky O , Morrell MJ , Vogt BA ((1995) ) Contributions of anterior cingulate cortex to behaviour. Brain 118: (Pt 1), 279–306. |
[40] | Margulies DS , Kelly AMC , Uddin LQ , Biswal BB , Castellanos FX , Milham MP ((2007) ) Mapping the functional connectivity of anterior cingulate cortex. Neuroimage 37: , 579–588. |
[41] | Gariano RF , Groves PM ((1988) ) Burst firing induced in midbrain dopamine neurons by stimulation of the medial prefrontal and anterior cingulate cortices. Brain Res 462: , 194–198. |
[42] | Vassena E , Silvetti M , Boehler CN , Achten E , Fias W , Verguts T ((2014) ) Overlapping neural systems represent cognitive effort and reward anticipation. PLoS One 9: , e91008. |
[43] | Croxson PL , Walton ME , O’Reilly JX , Behrens TEJ , Rushworth MFS ((2009) ) Effort-based cost-benefit valuation and the human brain. J Neurosci 29: , 4531–4541. |
[44] | Varazzani C , San-Galli A , Gilardeau S , Bouret S ((2015) ) Noradrenaline and dopamine neurons in the reward/effort trade-off: A direct electrophysiological comparison in behaving monkeys. J Neurosci 35: , 7866–7877. |
[45] | Arnsten AF , Steere JC , Hunt RD ((1996) ) The contribution of alpha 2-noradrenergic mechanisms of prefrontal cortical cognitive function. Potential significance for attention-deficit hyperactivity disorder. Arch Gen Psychiatry 53: , 448–455. |
[46] | Wang M , Ramos BP , Paspalas CD , Shu Y , Simen A , Duque A , Vijayraghavan S , Brennan A , Dudley A , Nou E , Mazer JA , McCormick DA , Arnsten AFT ((2007) ) Alpha2A-adrenoceptors strengthen working memory networks by inhibiting cAMP-HCN channel signaling in prefrontal cortex. Cell 129: , 397–410. |
[47] | Li BM , Mei ZT ((1994) ) Delayed-response deficit induced by local injection of the alpha 2-adrenergic antagonist yohimbine into the dorsolateral prefrontal cortex in young adult monkeys. Behav Neural Biol 62: , 134–139. |
[48] | Li B ((1999) ) Alpha-2 adrenergic modulation of prefrontal cortical neuronal activity related to spatial working memory in monkeys. Neuropsychopharmacology 21: , 601–610. |
[49] | Engström M , Landtblom AM , Karlsson T ((2013) ) Brain and effort: Brain activation and effort-related working memory in healthy participants and patients with working memory deficits. Front Hum Neurosci 7: , 140. |
[50] | Borst JP , Anderson JR ((2013) ) Using model-based functional MRI to locate working memory updates and declarative memory retrievals in the fronto-parietal network. Proc Natl Acad Sci U S A 110: , 1628–1633. |
[51] | Kahneman D ((1973) ) Attention and effort. Prentice Hall. |
[52] | Robison MK , Unsworth N ((2019) ) Pupillometry tracks fluctuations in working memory performance. Atten Percept Psychophys 81: , 407–419. |
[53] | Elosúa MR , Rosa Elosúa M , Peinado M , Contreras MJ , Manuel Reales J , Montoro PR ((2016) ) The suppression effect in visuospatial and verbal working memory span tasks in patients with Alzheimer’s disease: A 2-year follow-up study. Neurocase 22: , 426–435. |
[54] | Joshi S , Li Y , Kalwani RM , Gold JI ((2016) ) Relationships between pupil diameter and neuronal activity in the locus coeruleus, colliculi, and cingulate cortex. Neuron 89: , 221–234. |
[55] | Granholm EL , Panizzon MS , Elman JA , Jak AJ , Hauger RL , Bondi MW , Lyons MJ , Franz CE , Kremen WS ((2017) ) Pupillary responses as a biomarker of early risk for Alzheimer’s disease. J Alzheimers Dis 56: , 1419–1428. |
[56] | Guérin D , Sacquet J , Mandairon N , Jourdan F , Didier A ((2009) ) Early locus coeruleus degeneration and olfactory dysfunctions in Tg2576 mice. Neurobiol Aging 30: , 272–283. |
[57] | Betts MJ , Kirilina E , Otaduy MCG , Ivanov D , Acosta-Cabronero J , Callaghan MF , Lambert C , Cardenas-Blanco A , Pine K , Passamonti L , Loane C , Keuken MC , Trujillo P , Lüsebrink F , Mattern H , Liu KY , Priovoulos N , Fliessbach K , Dahl MJ , Maaß A , Madelung CF , Meder D , Ehrenberg AJ , Speck O , Weiskopf N , Dolan R , Inglis B , Tosun D , Morawski M , Zucca FA , Siebner HR , Mather M , Uludag K , Heinsen H , Poser BA , Howard R , Zecca L , Rowe JB , Grinberg LT , Jacobs HIL , Düzel E , Hämmerer D ((2019) ) Locus coeruleus imaging as a biomarker for noradrenergic dysfunction in neurodegenerative diseases. Brain 142: , 2558–2571. |
[58] | Liu Y , Rodenkirch C , Moskowitz N , Schriver B , Wang Q ((2017) ) Dynamic lateralization of pupil dilation evoked by locus coeruleus activation results from sympathetic, not parasympathetic, contributions. Cell Rep 20: , 3099–3112. |
[59] | Gannon M , Che P , Chen Y , Jiao K , Roberson ED , Wang Q ((2015) ) Noradrenergic dysfunction in Alzheimer’s disease. Front Neurosci 9: , 220. |
[60] | Hoogendijk WJ , Feenstra MG , Botterblom MH , Gilhuis J , Sommer IE , Kamphorst W , Eikelenboom P , Swaab DF ((1999) ) Increased activity of surviving locus ceruleus neurons in Alzheimer’s disease. Ann Neurol 45: , 82–91. |
[61] | Kremen WS , Panizzon MS , Elman JA , Granholm EL , Andreassen OA , Dale AM , Gillespie NA , Gustavson DE , Logue MW , Lyons MJ , Neale MC , Reynolds CA , Whitsel N , Franz CE ((2019) ) Pupillary dilation responses as a midlife indicator of risk for Alzheimer’s disease: Association with Alzheimer’s disease polygenic risk. Neurobiol Aging 83: , 114–121. |
[62] | Koob GF , Stinus L , Le Moal M ((1981) ) Hyperactivity and hypoactivity produced by lesions to the mesolimbic dopamine system. Behav Brain Res 3: , 341–359. |
[63] | Puciłowski O , Kostowski W , Bidziński A , Hauptmann M ((1982) ) Effect of 6-hydroxydopamine-induced lesions of A10 dopaminergic neurons on aggressive behavior in rats. Pharmacol Biochem Behav 16: , 547–551. |
[64] | Furlanetti LL , Coenen VA , Döbrössy MD ((2016) ) Ventral tegmental area dopaminergic lesion-induced depressive phenotype in the rat is reversed by deep brain stimulation of the medial forebrain bundle. Behav Brain Res 299: , 132–140. |
[65] | Heneka MT , Nadrigny F , Regen T , Martinez-Hernandez A , Dumitrescu-Ozimek L , Terwel D , Jardanhazi-Kurutz D , Walter J , Kirchhoff F , Hanisch U-K , Kummer MP ((2010) ) Locus ceruleus controls Alzheimer’s disease pathology by modulating microglial functions through norepinephrine. Proc Natl Acad Sci U S A 107: , 6058–6063. |
[66] | Kelly SC , He B , Perez SE , Ginsberg SD , Mufson EJ , Counts SE ((2017) ) Locus coeruleus cellular and molecular pathology during the progression of Alzheimer’s disease. Acta Neuropathol Commun 5: , 8. |
[67] | Asan E ((1998) ) The catecholaminergic innervation of the rat amygdala. Adv Anat Embryol Cell Biol 142: , 1–118. |
[68] | Segal M , Markram H , Richter-Levin G ((1991) ) Actions of norepinephrine in the rat hippocampus. Prog Brain Res 88: , 323–330. |
[69] | Chandler DJ , Gao W-J , Waterhouse BD ((2014) ) Heterogeneous organization of the locus coeruleus projections to prefrontal and motor cortices. Proc Natl Acad Sci U S A 111: , 6816–6821. |
[70] | Condés-Lara M ((1998) ) Different direct pathways of locus coeruleus to medial prefrontal cortex and centrolateral thalamic nucleus: Electrical stimulation effects on the evoked responses to nociceptive peripheral stimulation. Eur J Pain 2: , 15–23. |
[71] | Tiraboschi P , Hansen LA , Thal LJ , Corey-Bloom J ((2004) ) The importance of neuritic plaques and tangles to the development and evolution of AD. Neurology 62: , 1984–1989. |
[72] | McNamara CG , Dupret D ((2017) ) Two sources of dopamine for the hippocampus. Trends Neurosci 40: , 383–384. |
[73] | Sara SJ ((2009) ) The locus coeruleus and noradrenergic modulation of cognition. Nat Rev Neurosci 10: , 211–223. |
[74] | Jing Li J , Szkudlarek H , Renard J , Hudson R , Rushlow W , Laviolette SR ((2018) ) Fear memory recall potentiates opiate reward sensitivity through dissociable dopamine D1 versus D4 receptor-dependent memory mechanisms in the prefrontal cortex. J Neurosci 38: , 4543–4555. |
[75] | Gaykema RP , Zaborszky L ((1996) ) Direct catecholaminergic-cholinergic interactions in the basal forebrain. II. Substantia nigra-ventral tegmental area projections to cholinergic neurons. J Comp Neurol 374: , 555–577. |
[76] | Mesulam MM ((2013) ) Cholinergic circuitry of the human nucleus basalis and its fate in Alzheimer’s disease. J Comp Neurol 521: , 4124–4144. |
[77] | Smiley JF , Mesulam MM ((1999) ) Cholinergic neurons of the nucleus basalis of Meynert receive cholinergic, catecholaminergic and GABAergic synapses: An electron microscopic investigation in the monkey. Neuroscience 88: , 241–255. |
[78] | Mesulam M , Shaw P , Mash D , Weintraub S ((2004) ) Cholinergic nucleus basalis tauopathy emerges early in the aging-MCI-AD continuum. Ann Neurol 55: , 815–828. |
[79] | Liu AKL , Chang RCC , Pearce RKB , Gentleman SM ((2015) ) Nucleus basalis of Meynert revisited: Anatomy, history and differential involvement in Alzheimer’s and Parkinson’s disease. Acta Neuropathol 129: , 527–540. |
[80] | Wang J , Gu BJ , Masters CL , Wang YJ ((2017) ) A systemic view of Alzheimer disease — insights from amyloid-β metabolism beyond the brain. Nat Rev Neurol 13: , 612–623. |
[81] | Dubois B , Padovani A , Scheltens P , Rossi A , Dell’Agnello G ((2016) ) Timely diagnosis for Alzheimer’s disease: A literature review on benefits and challenges. J Alzheimers Dis 49: , 617–631. |
[82] | Olsson B , Lautner R , Andreasson U , Öhrfelt A , Portelius E , Bjerke M , Hölttä M , Rosén C , Olsson C , Strobel G , Wu E , Dakin K , Petzold M , Blennow K , Zetterberg H ((2016) ) CSF and blood biomarkers for the diagnosis of Alzheimer’s disease: A systematic review and meta-analysis. Lancet Neurol 15: , 673–684. |
[83] | Gao LB , Yu XF , Chen Q , Zhou D ((2016) ) Alzheimer’s disease therapeutics: Current and future therapies. Minerva Med 107: , 108–113. |
[84] | Anand R , Gill KD , Mahdi AA ((2014) ) Therapeutics of Alzheimer’s disease: Past, present and future. Neuropharmacology 76 Pt A: , 27–50. |
[85] | D’Amelio M , Puglisi-Allegra S , Mercuri N ((2018) ) The role of dopaminergic midbrain in Alzheimer’s disease: Translating basic science into clinical practice. Pharmacol Res 130: , 414–419. |
[86] | Martorana A , Mori F , Esposito Z , Kusayanagi H , Monteleone F , Codecà C , Sancesario G , Bernardi G , Koch G ((2009) ) Dopamine modulates cholinergic cortical excitability in Alzheimer’s disease patients. Neuropsychopharmacology 34: , 2323–2328. |
[87] | Martorana A , Di Lorenzo F , Esposito Z , Lo Giudice T , Bernardi G , Caltagirone C , Koch G ((2013) ) Dopamine D2-agonist Rotigotine effects on cortical excitability and central cholinergic transmission in Alzheimer’s disease patients. Neuropharmacology 64: , 108–113. |
[88] | Koch G , Di Lorenzo F , Bonnì S , Giacobbe V , Bozzali M , Caltagirone C , Martorana A ((2014) ) Dopaminergic modulation of cortical plasticity in Alzheimer’s disease patients. Neuropsychopharmacology 39: , 2654–2661. |
[89] | Diniz BS , Butters MA , Albert SM , Dew MA , Reynolds CF 3rd ((2013) ) Late-life depression and risk of vascular dementia and Alzheimer’s disease: Systematic review and meta-analysis of community-based cohort studies. Br J Psychiatry 202: , 329–335. |
[90] | Mendes-Silva AP , Pereira KS , Tolentino-Araujo GT , Nicolau E de S , Silva-Ferreira CM , Teixeira AL , Diniz BS ((2016) ) Shared biologic pathways between Alzheimer disease and major depression: A systematic review of microRNA expression studies. Am J Geriatr Psychiatry 24: , 903–912. |
[91] | Caligiore D , Pezzulo G , Baldassarre G , Bostan AC , Strick PL , Doya K , Helmich RC , Dirkx M , Houk J , Jörntell H , Lago-Rodriguez A , Galea JM , Chris Miall R , Popa T , Kishore A , Paul F M , Zucca R , Herreros I ((2016) ) Consensus paper: Towards a systems-level view of cerebellar function: The interplay between cerebellum, basal ganglia, and cortex. Cerebellum 16: , 203–229. |
[92] | Caligiore D , Borghi AM , Parisi D , Baldassarre G ((2010) ) TRoPICALS: A computational embodied neuroscience model of compatibility effects. Psychol Rev 117: , 1188–1228. |
[93] | Caligiore D , Fischer MH ((2013) ) Vision, action and language unified through embodiment. Psychol Res 77: , 1–6. |
[94] | Arbib MA , Bonaiuto JJ ((2016) ), From Neuron to Cognition Via Computational Neuroscience. MIT Press. |
[95] | Scheltens P , Blennow K , Breteler MMB , de Strooper B , Frisoni GB , Salloway S , Van der Flier WM ((2016) ) Alzheimer’s disease. Lancet 388: , 505–517. |
[96] | Ricciarelli R , Fedele E ((2017) ) The amyloid cascade hypothesis in Alzheimer’s disease: It’s time to change our mind. Curr Neuropharmacol 15: , 926–935. |
[97] | Caligiore D , Mannella F , Arbib MA , Baldassarre G ((2017) ) Dysfunctions of the basal ganglia-cerebellar-thalamo-cortical system produce motor tics in Tourette syndrome. PLoS Comput Biol 13: , e1005395. |
[98] | Silvetti M , Wiersema JR , Sonuga-Barke E , Verguts T ((2013) ) Deficient reinforcement learning in medial frontal cortex as a model of dopamine-related motivational deficits in ADHD. Neural Netw 46: , 199–209. |
[99] | Caligiore D , Helmich RC , Hallett M , Moustafa AA , Timmermann L , Toni I , Baldassarre G ((2016) ) Parkinson’s disease as a system-level disorder. NPJ Parkinsons Dis 2: , 16025. |
[100] | Schwartenbeck P , Friston K ((2016) ) Computational phenotyping in psychiatry: A worked example. eNeuro 3: , ENEURO.0049-16.2016. |