Which Risk Factors Causally Influence Dementia? A Systematic Review of Mendelian Randomization Studies
Abstract
Background:
Numerous risk factors for dementia are well established, though the causal nature of these associations remains unclear.
Objective:
To systematically review Mendelian randomization (MR) studies investigating causal relationships between risk factors and global cognitive function or dementia.
Methods:
We searched five databases from inception to February 2017 and conducted citation searches including MR studies investigating the association between any risk factor and global cognitive function, all-cause dementia or dementia subtypes. Two reviewers independently assessed titles and abstracts, full-texts, and study quality.
Results:
We included 18 MR studies investigating education, lifestyle factors, cardiovascular factors and related biomarkers, diabetes related and other endocrine factors, and telomere length. Studies were of predominantly good quality, however eight received low ratings for sample size and statistical power. The most convincing causal evidence was found for an association of shorter telomeres with increased risk of Alzheimer’s disease (AD). Causal evidence was weaker for smoking quantity, vitamin D, homocysteine, systolic blood pressure, fasting glucose, insulin sensitivity, and high-density lipoprotein cholesterol. Well-replicated associations were not present for most exposures and we cannot fully discount survival and diagnostic bias, or the potential for pleiotropic effects.
Conclusions:
Genetic evidence supported a causal association between telomere length and AD, whereas limited evidence for other risk factors was largely inconclusive with tentative evidence for smoking quantity, vitamin D, homocysteine, and selected metabolic markers. The lack of stronger evidence for other risk factors may reflect insufficient statistical power. Larger well-designed MR studies would therefore help establish the causal status of these dementia risk factors.
INTRODUCTION
According to recent estimates and projections, 35.6 million older adults across the globe suffered from dementia in 2010, and 115 million will have dementia in 2050 [1]. Currently there are no disease modifying treatments, but if we reduced the prevalence of seven modifiable risk factors (diabetes, midlife hypertension, midlife obesity, depression, physical inactivity, smoking, low education) by 10% per decade, 8.8 million cases of Alzheimer’s disease (AD) may potentially be prevented worldwide in 2050 [2]. This illustrates the potential of dementia prevention but relies on the assumption that these risk factors are causally associated. A number of dementia risk factors have been identified in epidemiological research. However, these observational findings are challenged by the inability to distinguish cause from effect and confounders inducing (or masking) an association. Randomized controlled trials (RCTs) are not a viable option for establishing causal effects where there is a long latency from exposure to disease onset, or it is unethical to administer (or withdraw) an exposure. Mendelian randomization (MR) is an alternative approach which uses genetic variation as an instrument to assign participants to exposure groups, akin to arms of a naturally occurring randomized controlled trial [3, 4]. As an individual’s genotype is randomly assigned at conception, it cannot be altered by either the outcome of interest (reverse causation) or confounders, resolving the two main issues in observational studies. Genetic variants are selected as instrumental variables (IVs) when they are robustly associated with the exposure, with the fundamental assumption that they are associated with the outcome through the exposure (Fig. 1). It is assumed that there is no direct path between the genetic instrument and the outcome. As MR can be a powerful tool to examine causality, we conducted a systematic review of MR studies investigating causal relationships between risk factors and global cognitive function or dementia.
Fig. 1
Schematic of the principles of Mendelian randomization. Mendelian randomization can be used to test for a causal relationship between a risk factor and outcome, indicated here with the red arrow. A genetic instrument (e.g., a single nucleotide polymorphism) associated with the risk factor (blue arrow) can be used as an instrumental variable to effectively randomly assign individuals to exposure groups. Reverse causation can be excluded as it is not possible for the outcome to influence a genotype which is established at conception. One important assumption is that there is no association between the genetic instrument and the outcome except via the risk factor (i.e., the dashed arrow does not exist).
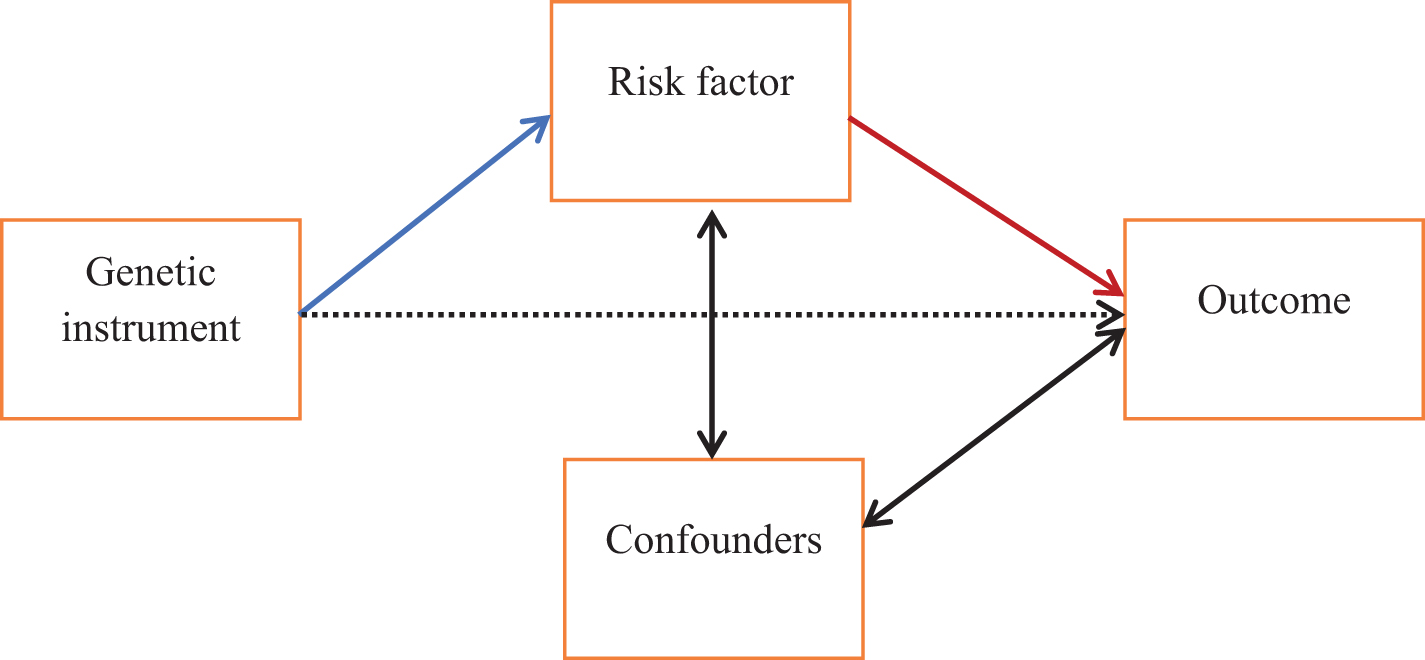
METHODS
We conducted this systematic review following general principles outlined by the Centre for Reviews and Dissemination [5].
Search strategy
Following a pre-defined protocol (Supplementary Methods), an information specialist (AB) developed a search strategy in collaboration with dementia and methods experts (EK, IL, DJL, JTC, KL, EHy) including subject headings and free text terms relevant to dementia, cognition and MR. The search strategy developed for Medline is provided in Supplementary Figure 1. We searched five databases from inception to 16 February 2017 with no language restrictions: Medline, Embase, PsycINFO (via OvidSP), BIOSIS Citation Index (via Web of Science) and the Cochrane Central Register of Controlled Trials (Central). We also conducted forward and backward citation searches of included publications via Web of Science and Scopus.
Study selection and data extraction
We included studies using MR to investigate the association between any risk factor and all-cause dementia, dementia subtypes, global cognitive function, or cognitive impairment. When selecting studies for inclusion we broadened the definition of MR by Lawlor and colleagues [6] to include any study that uses genetic variation as a proxy for an exposure to make causal inferences. Therefore, we retained studies that did not quantify the causal relationship but investigated the effect of a genetic IV on the outcome. Non-genetic studies, genetic studies other than MR, studies with outcomes not directly related to the clinical syndrome of dementia (e.g., neuroimaging, domain specific cognitive tests) and animal studies were excluded. We also excluded case reports, narrative reviews, letters, editorials, opinions and conference abstracts. Two reviewers (EK, IL) independently screened titles and abstracts using pre-defined inclusion and exclusion criteria. Full-texts were also screened independently by the same two reviewers and discussed with a third reviewer with particular expertise in MR (EHa). Key data were extracted by one reviewer (EK) and checked by two reviewers (EHa, IL). Where exposures were tested with multiple IVs, results based on the strongest IV (ranked by F statistics) were considered as primary analyses and results using alternative IVs are included in Additional files. If results from multiple cohorts were combined, we extracted only pooled findings. We also tried to contact corresponding authors of studies where relevant data was not fully reported [7–9] and received additional data for one study [9].
Risk of bias
The risk of bias of included studies was assessed independently by two reviewers (EK, EHa) with discrepancies resolved by discussion using the Q-Genie tool [10], developed and validated specifically to measure the quality of genetic studies with 11 items assessing study rationale, risk of bias, statistical power and appropriateness of conclusions on a seven-point Likert scale [10].
Data synthesis
Extracted data were synthesized narratively because meta-analyses could not be conducted due to a wide range of exposures, IVs, outcomes, and statistical methods used in included studies. Main findings were categorized by exposure. Studies varied in their approaches to multiple testing, in part due to differing numbers of exposures and outcomes tested. For consistency we presented uncorrected results and considered p values < 0.05 statistically significant. Where applicable, we indicated which results were adjusted for multiple testing in the original publication.
RESULTS
We identified 315 records from electronic database searches. After removing duplicates (114 records), we excluded 152 records based on the title and abstract screening. We reviewed full-texts of 22 articles, 17 of which met our inclusion criteria. We identified one additional study [8] via backward citation searches of the 17 included studies, so in total 18 studies were eligible for inclusion in our systematic review (Supplementary Figure 2). Key characteristics of included studies are shown in Supplementary Table 1. These focused on a wide range of exposures: education [11, 12], lifestyle factors [12–16], cardiovascular factors and related biomarkers [7–9, 12, 17–19], diabetes related and other endocrine factors [12, 20–24], and telomere length [25], and dementia-related outcomes: global cognitive function [8, 9, 14, 15, 20, 22, 24], cognitive impairment [13], dementia probability [11, 17, 21], all-cause dementia [7] and AD [12, 16–19, 21, 23, 25]. While the majority of studies were based on populations of European ancestry, three were Asian [14, 22, 24] and one was mixed [19]. Three studies [13, 14, 22] included only men, one only women [24]. Analytic sample sizes ranged from 570 [7] to 54,162 [12, 16, 21, 23, 25], and all studies involved middle-aged and/or older participants. There was variety in the statistical approaches employed for MR: 15 studies derived causal estimates by either two-stage least squares regression [7, 14, 15, 20–22], ratio of coefficients [11, 18, 19, 24], inverse-variance weighted combination of summary statistics from a genome-wide association study (GWAS) [12, 17, 21, 23, 25] or weighted generalized linear regression for correlated single nucleotide polymorphisms (SNPs) [16]. Three studies only performed an association analysis of the genetic instrument on the outcome [8, 9, 13]. The studies used either a single genetic variant [8, 9, 13–15], a combination of genetic variants, sometimes combined into a genetic risk score (GRS) [11, 12, 16–24], or both [7, 25] as IVs. The majority of included studies were of good overall quality, with three studies [7, 8, 13] rated as moderate (Supplementary Table 2). However, eight studies [7, 8, 11, 13, 14, 20, 22, 24] had low ratings (<4/7) regarding the sample size and statistical power. Nine studies performed multiple tests, either considering multiple risk factors, IVs or outcomes [7, 9, 12, 16–18, 21, 23, 24] however adjustment for multiple comparisons was rare and handling of missing phenotypic data often not addressed.
Education
Two studies examined genetic evidence for the causal association between education and dementia probability [11] or AD [12]. The causal estimates were in the expected direction with lower dementia probability [11] or odds of AD [12] for each year of education or completion of university, though these associations were not statistically significant (p > 0.10 for all comparisons; Table 1).
Table 1
Results of included studies investigating education and lifestyle factors
Study | Methods | Results |
---|---|---|
Exposure (analytic n/casesa) | MR estimate (95% CI) | |
Nguyen et al., 2016 [11] Education (7,981) | GRS is weighted sum of risk alleles (R2 = 0.11%); separate-sample 2SLSb | Dementia probability IV estimated change in dementia probability per education year = −0.011 (−0.024, 0.002), p = 0.11 |
Østergaard et al., 2015 [12] Education, smoking (54,162 / 17,008) | Inverse-variance weighted combination of summary statisticsc | AD IV estimated OR per unit in log odds of university completion = 0.95 (0.67, 1.34), p = 0.752 IV estimated OR per education year = 0.71 (0.48, 1.06), p = 0.097 IV estimated OR per unit in log odds of smoking initiation = 0.70 (0.37, 1.33), p = 0.278 IV estimated OR per 10 cigarettes/day = 0.67 (0.51, 0.89), p = 6.5×10−3 |
North et al., 2015 [15] Smoking (13,004d) | 2SLS regression within each cohort and combined with a random effects meta-analysis | General fluid cognition IV estimated difference between current and ex-smoker = 0.029 (−1.394, 1.453) |
Almeida et al., 2014 [13] Alcohol (3,542 / 502) | Association test of IV on outcome | Cognitive impairment OR for AA versus GG in rs1229984 = 1.35 (0.29, 6.27) OR for GA versus GG in rs1229984 = 1.05 (0.71, 1.55) |
Au Yeung et al., 2012 [14] Alcohol (2,284) | 2SLS regression; R2 = 3% | Global cognition IV estimated change per 10g ethanol/day = 0.06 (−0.22, 0.34) |
Kwok et al., 2016 [16] Coffee (54,162 / 17,008) | Weighted generalized linear regression for correlated SNPse | AD IV estimated OR per cup per day = 1.17 (0.96, 1.43)f |
AD, Alzheimer’s disease; CI, Confidence Interval; GRS, genetic risk score; IV, instrumental variable; MR, Mendelian randomization; OR, odds ratio; R2, percentage of variance explained; 2SLS, two-stage least squares. aReported for binary outcomes only. bAdjusted for sex, age at first outcome assessment, age2, early life socioeconomic status, population eigenvectors. cStudy applied a Bonferroni corrected significance threshold of p < 3.8×10−3. dTotal number of participants with age, sex, genotype, smoking status and general fluid intelligence factor, and restricted to those included also in the observational analysis. eBonferroni corrected significance level of 0.002. fIV estimated OR per cup per day = 1.29 (0.82, 2.03) after exclusion of 4 SNPs associated with body weight or lipids and 2 SNPs previously not associated with coffee.
Lifestyle factors
Two studies [12, 15] investigated causal effects of smoking in participants of European ancestry. North and colleagues [15] used IVs from CHRNA5 or CHRNA3 and reported no evidence for a causal association between current smokers and global fluid cognition compared to former smokers. Østergaard and colleagues [12] found no evidence for a causal association between smoking initiation (BDNF) and odds of AD. However, elevated smoking quantity (CHRNA3, LOC100188947 and CYP2A6) was significantly associated with lower odds of AD [12] (Table 1).
Two studies conducted in men only evaluated the association between alcohol consumption and cognitive impairment [13] or global cognition [14]. One study [13] based on participants of European ancestry found no association with cognitive impairment for rs1229984 in ADH1B where the minor allele (A) has previously been associated with lower alcohol consumption [26]. There was also no evidence for a causal association in the other study of global cognition conducted in Chinese men using rs671 in ALDH2 as the IV [14] (Table 1).
One study [16] investigating coffee consumption found no genetic evidence supporting a causal association with odds of AD (Table 1).
Cardiovascular factors and related biomarkers
Three studies [12, 17, 18] investigated cardiovascular factors and their association with dementia risk. Mukherjee and colleagues [17] examined whether there is a causal link between body mass index (BMI) and either dementia probability or AD. Neither BMI nor any of the mechanism-specific aspects of obesity (e.g., adipogenesis, appetite or cardiopulmonary processes) were significantly associated with dementia probability [17] (Table 2). While there was also no significant association with BMI, the group of “unspecific cellular processes” was statistically significantly associated with odds of AD [17]. Similarly, Østergaard and colleagues [12], using an IV derived from a largely overlapping set of SNPs, did not find evidence to support a causal association between BMI and AD (Table 2).
Table 2
Results of included studies investigating cardiovascular factors and related biomarkers
Study Exposure (analytic n/casesa) | Methods | Results MR estimate (95% CI) |
---|---|---|
Mukherjee et al., 2015 [17] BMI (dementia probability: 8,403; AD: 30,146 / 13,256) | GRS is weighted sum of alleles (R2 ∼1%); association test of GRS on outcome for ADGC and HRS; inverse variance weighted summary statistic for GERAD; ADGC and GERAD results combined with fixed effects meta-analysisb | Dementia probability IV estimated OR per BMI GRS unit = 1.00 (0.75, 1.32), p = 0.98 IV estimated OR per BMI adipogenesis GRS unit = 1.05 (0.42, 2.62), p = 0.92 IV estimated OR per BMI appetite GRS unit = 1.02 (0.72, 1.44), p = 0.92 IV estimated OR per BMI cardiopulmonary processes GRS unit = 1.09 (0.42, 2.83), p = 0.86 IV estimated OR per BMI unspecified cellular processes GRS unit = 0.87 (0.46, 1.66), p = 0.68 AD IV estimated OR per BMI GRS unit = 0.95 (0.91, 1.00), p = 0.058 IV estimated OR per BMI adipogenesis GRS unit = 1.07 (0.90, 1.27), p = 0.450 IV estimated OR per BMI appetite GRS unit = 0.96 (0.90, 1.02), p = 0.160 IV estimated OR per BMI cardiopulmonary processes GRS unit = 1.21 (1.00, 1.47), p = 0.055 IV estimated OR per BMI unspecified cellular processes GRS unit = 0.81 (0.74, 0.90), p = <0.001 |
Østergaard et al., 2015 [12] BMI, SBP, total cholesterol, HDL, LDL, triglycerides (54,162 / 17,008) | Inverse-variance weighted combination of summary statisticsc | AD IV estimated OR per BMI SD = 0.99 (0.80, 1.19), p = 0.779 IV estimated OR per SBP SD = 0.75 (0.62, 0.91), p = 3.4×10−3 IV estimated OR per total cholesterol SDd = 1.94 (1.79, 2.10), p = 3.1×10−56 IV estimated OR per HDL SDe = 0.75 (0.69, 0.82), p = 1.0×10−11 IV estimated OR per LDL SDf = 2.31 (2.12, 2.50), p = 3.0×10−87 IV estimated OR per triglycerides SD = 0.96 (0.87, 1.07), p = 0.482 |
Proitsi et al., 2014 [18] Total cholesterol, HDL, LDL, triglycerides | GRS is standardized weighted sum of risk alleles (total cholesterol full GRS R2 = 3.59%, specific GRS R2 = 0.31%; HDL full GRS R2 = 4.19%, specific GRS R2 = 0.02%; LDL full GRS R2 = 1.83%, specific GRS R2 = 0.28%; triglycerides full GRS R2 = 4.34%, specific GRS R2 = 0.50%); where GRS risk prediction R2≥1.5% ratio of coefficients methodg; where GRS risk prediction R2 < 1.5% inverse-variance weighted combination of summary statistics. Results from each cohort were combined with inverse-variance fixed effects meta-analysis | AD IV estimated OR per total cholesterol GRS unit (full GRS) = 0.954 (0.76, 1.21), p = 0.688 IV estimated OR per total cholesterol GRS unit (trait specific GRS) = 1.036 (0.99, 1.09), p = 0.144 IV estimated OR per HDL GRS unit (full GRS) = 1.005 (0.82, 1.24), p = 0.962 IV estimated OR per HDL GRS unit (trait specific GRS) = 1.463 (1.05, 2.04), p = 0.023 IV estimated OR per LDL GRS unit (full GRS) = 0.901 (0.65, 1.25), p = 0.530 IV estimated OR per LDL GRS unit (trait specific GRS) = 0.572 (0.24, 1.34), p = 0.199 IV estimated OR per triglycerides GRS unit (full GRS) = 1.104 (0.89, 1.37), p = 0.362 IV estimated OR per triglycerides GRS unit (trait specific GRS) = 0.872 (0.46, 1.65), p = 0.676 |
Cruchaga et al., 2012 [7] CSF APOE levels (570 / 146) | 2SLS regressionh; R2 = 8.2% | Dementia severity at baseline APOE p = 3.06×10−5 Dementia severity at 36 months APOE p = 2.06×10−6 Case-control status for AD at baseline significant associations per CSF APOE levels |
Marioni et al., 2011 [8] Fibrinogen (4,248) | Association of IV on outcome in each cohort were combined with inverse variance meta-analysisi | General cognitive factor rs2227412 (GA versus GG and AA) p = 0.14 |
Quinn et al., 2015 [9] D-dimer, fibrinogen, plasminogen activator inhibitor, von Willebrand factor (12,757–13,142) | Association test of IV on outcomej; (D-dimer R2 = 1.8%; fibrinogen R2 = 2%; plasminogen activator inhibitor R2 = 3.7%; von Willebrand factor; R2 = 13%) | General cognitive factork Difference between GT and GG at rs12029080 (D-dimer) = −0.010 (−0.073; 0.054), p = 0.765 Difference between TT and GG at rs12029080 (D-dimer) = 0.002 (−0.061; 0.065), p = 0.951 Difference between AG and AA at rs1800789 (fibrinogen) = −0.022 (−0.115; 0.071), p = 0.646 Difference between GG and AA at rs1800789 (fibrinogen) = −0.040 (−0.132; 0.052), p = 0.394 Difference between AG and AA at rs2227631 (plasminogen activator inhibitor) = 0.029 (−0.008; 0.065), p = 0.121 Difference between GG and AA at rs2227631 (plasminogen activator inhibitor) = 0.036 (−0.016; 0.087), p = 0.172 Difference between GA and GG at rs1063857 (von Willebrand factor) = −0.05 (−0.055; 0.045), p = 0.832 Difference between AA and GG at rs1063857 (von Willebrand factor) = 0.006 (−0.047; 0.058), p = 0.824 |
Hu et al., 2016 [19] Homocysteine (9,397 / 4,120) | Ratio of coefficients method | AD IV estimated OR per SD unit of homocysteine (overall) = 3.37 (1.90, 5.95), p = 2.9×10−5 IV estimated OR per SD unit of homocysteine (Caucasian) = 1.67 (0.95, 2.95), p = 0.75 IV estimated OR per SD unit of homocysteine (Asian) = 6.69 (2.13, 20.99), p = 0.0011 IV estimated OR per SD unit of homocysteine (other) = 12.6 (3.15, 50.61), p = 3.4×10−3 |
AD, Alzheimer’s disease; ADGC, Alzheimer’s Disease Genetics Consortium; APOE, apolipoprotein E; BMI, body mass index; CI, confidence interval; CSF, cerebrospinal fluid; GERAD, Genetic and Environmental Risk for Alzheimer’s Disease consortium; GRS, genetic risk score; HDL, high-density lipoprotein cholesterol; IV, instrumental variable; LDL, low-density lipoprotein cholesterol; MR, Mendelian randomization; OR, odds ratio; SBP, systolic blood pressure; SD, standard deviation; 2SLS, two-stage least squares. aReported for binary outcomes only. bADGC models adjusted for study, 3 genetic principal components; HRS models adjusted for 6 genetic principal components, age and sex. cStudy applied a Bonferroni corrected significance threshold of p < 3.8×10−3. dIV estimated OR per total cholesterol SD = 1.04; 95% CI: 0.95–1.13, p = 0.84 after exclusion of SNPs associated with AD. eIV estimated OR per HDL SD = 1.01, 95% CI: 0.93–1.09, p = 0.87 after exclusion of SNPs associated with AD. fIV estimated OR per LDL SD = 1.07, 95% CI: 0.98–1.17, p = 0.14 after exclusion of SNPs associated with AD. gAll analyses of GRS on AD adjusted for the first 4 genetic principal components, additional adjustment for genotyping batch in Institute of Psychiatry Plus data only. hAdjustment strategy unclear. iAdjusted for age and sex. jAdjusted for age, sex, Mill Hill test, household income, education, blood pressure, heart rate, body mass index, waist-hip ratio, alcohol consumption, smoking, vascular and cognitive comorbidity history. kEstimates (except for fibrinogen) provided from the authors at request.
Østergaard and colleagues [12] also investigated systolic blood pressure (SBP) reporting that higher SBP significantly lowered the odds of AD (Table 2), a finding that survived Bonferroni correction for the multiple exposures they considered.
Two studies [12, 18] examined the link between total cholesterol, high-density lipoprotein cholesterol (HDL), low-density lipoprotein cholesterol (LDL), triglycerides and AD. Primary analyses conducted by Østergaard and colleagues [12] found statistically significant associations between both higher total cholesterol and LDL and higher odds of AD whereas higher HDL was linked with lower odds of AD. However, these associations were no longer significant after excluding rs6857 near APOE and rs1883025 from ABCA1 (both associated with AD) from the GRS IV, suggesting the initial results may be driven by pleiotropic effects of these excluded SNPs [12]. The study by Proitsi and colleagues [18] found no significant associations between total cholesterol, HDL, or LDL and odds of AD based on either individual level data (Table 2) or summary data (Supplementary Table 3). When instrumented by phenotype specific GRSs (limited to SNPs only associated with one exposure) calculated with summarized GWAS results, higher HDL was significantly associated with higher odds of AD but there was no link with either total cholesterol or LDL [18] (Table 2). This significant relationship may also be explained by pleiotropic effects of rs1883025, which was excluded in sensitivity analyses by Østergaard and colleagues due to its association with AD [12]. Taken together, there was no consistent evidence for a causal association between cholesterol levels and AD. Further, there was also no evidence of a causal association between triglycerides and odds of AD in either study [12, 18] (Table 2 and Supplementary Table 3).
APOE genotype is a well-known genetic risk factor for AD [27, 28]. Cruchaga and colleagues [7] investigated the causal effects of cerebrospinal APOE protein levels using MR and reported significant associations [7] with three related outcomes (dementia severity at baseline and 3-year follow-up, and baseline AD status). However, they did not provide estimates of the effect size or confidence intervals to allow for a more detailed evaluation of their findings (Table 2 and Supplementary Table 3).
Two studies [8, 9] examined fibrinogen in relationship to global cognition with neither study finding genetic evidence for a causal relationship. In addition to fibrinogen, Quinn and colleagues [9] also investigated D-dimer, plasminogen activator inhibitor and von Willebrand factor but found no evidence for an association between any of the IVs for these measures and global cognition (Table 2).
One study [19] investigating causal effects of homocysteine in a heterogeneous population of mixed ancestry found that increased homocysteine levels were associated with increased odds of AD. However, the association was no longer statistically significant when the sample was restricted to Caucasians (Table 2), which is likely important as the effects of the IV on AD were estimated in a European population.
Diabetes related and other endocrine factors
Two studies [12, 21] examined the causal relationship between type 2 diabetes (T2D) or related phenotypes and odds of AD using the International Genomics of Alzheimer’s Project (IGAP) GWAS results. One of these studies [21] also investigated the link with dementia probability in the Health and Retirement Study (HRS). Although they selected different sets of SNPs for the T2D GRS (49 [12] versus 39 [21] SNPs), neither study [12, 21] found a significant association with T2D. There was also little evidence to support the relationship with T2D related phenotypes, as the nominally significant finding by Walter and colleagues [21] for insulin sensitivity did not survive Bonferroni multiple testing correction (p = 0.08). However, higher fasting glucose was significantly associated with increased odds of AD after excluding one SNP (rs11039149) which is possibly pleiotropically associated with AD [12] (Table 3).
Table 3
Results of included studies investigating diabetes related and other endocrine factors, and telomere length
Study Exposure (analytic n/casesa) | Methods | Results MR estimate (95% CI) |
---|---|---|
Østergaard et al., 2015 [12] T2D, fasting glucose, insulin resistance (54,162 / 17,008) | Inverse-variance weighted combination of summary statisticsb | AD IV estimated OR per unit in log odds of T2D = 1.02 (0.97, 1.07), p = 0.535 IV estimated OR per fasting glucose SD = 1.12 (0.97, 1.30), p = 0.112c IV estimated OR per log-fasting insulin SD = 1.32 (0.88, 1.98), p = 0.177> |
Walter et al., 2016 [21] T2D (dementia probability: 8,501; AD: 54,162 / 17,008) | GRS is weighted sum of risk alleles converted to a probability predicting dementia probability (logit) T2D GRS R2 = 1.98% adiposity GRS R2 = 0.09% β-cell function GRS R2 = 1.28% insulin sensitivity GRS R2 = 0.29% other GRS R2 = 0.30%; 2SLS regression for dementia probabilityd; inverse-variance weighted combination of summary statistics for AD | Dementia probability (logit) IV estimated change per predicted risk of T2D = 0.04 (−0.92, 1.01) IV estimated change per predicted risk of T2D (adiposity SNPs) = 2.23 (−2.12, 6.59) IV estimated change per predicted risk of T2D (β-cell function SNPs) = −0.49 (−1.70, 0.73) IV estimated change per predicted risk of T2D (insulin sensitivity SNPs) = 1.59 (−0.99, 4.18) IV estimated change per predicted risk of T2D (other SNPs) = 0.21 (−2.28, 2.71) AD IV estimated OR per unit in log odds of T2D = 1.01 (0.96, 1.06), p = 0.79 IV estimated OR per unit in log odds of T2D (adiposity SNPs) = 0.93 (0.74, 1.15), p = 0.49 IV estimated OR per unit in log odds of T2D (β-cell function SNPs) = 1.00 (0.94, 1.07), p = 0.89 IV estimated OR per unit in log odds of T2D (insulin sensitivity SNPs) = 1.17 (1.02, 1.34), p = 0.02e IV estimated OR per unit in log odds of T2D (other SNPs) = 0.90 (0.79, 1.04), p = 0.14 |
Kueider et al., 2016 [20] Vitamin D (848) | GRS is sum of minor alleles; 2SLS regressionf | Global cognition IV estimated change per ng/mL of 25(OH)D = 0.01 (−0.01, 0.06), p = 0.41 |
Mokry et al., 2016 [23] Vitamin D (54,162 / 17,008) | Inverse-variance weighted combination of summary statistics; R2 = 2.44% | AD IV estimated OR per SD decrease in natural log 25(OH)D = 1.25 (1.03, 1.51), p = 0.02g IV estimated OR per SD decrease in natural log 25(OH)D (metabolism SNPs) = 1.46 (1.03, 2.07), p = 0.03 IV estimated OR per SD decrease in natural log 25(OH)D (synthesis SNPs) = 1.17 (0.93, 1.46), p = 0.17 |
Zhao et al., 2016 [22] Testosterone (4,122) | 2SLS regression; R2 = 4.1% | Global cognition IV estimated change per testosterone nmol/L = 0.06 (−0.002, 0.12), p = 0.06 |
Au Yeung et al., 2016 [24] 17β-estradiol (3,066) | Ratio of coefficients; R2 = 4.9%; | Global cognition IV estimated change per log 17β-estradiol pmol/L = 0.39 (−0.87, 1.65) |
Zhan et al., 2016 [25] Telomere length (54,162 / 17,008) | Inverse-variance weighted combined summary statistics | AD IV estimated OR per SD decrease of telomere length = 1.36 (1.12, 1.67), p = 0.002 |
AD, Alzheimer’s disease; CI, confidence interval; GRS, genetic risk score; MR, Mendelian randomization; OR, odds ratio; SD, standard deviation; SNP, single nucleotide polymorphism; T2D, type 2 diabetes; 2SLS, two-stage least squares; 25(OH)D, 25-hydroxyvitamin D. aReported for binary outcomes only. bStudy applied a Bonferroni corrected significance threshold of p < 3.8×10−3. cIV estimated OR per fasting glucose SD = 1.19; 95% CI: 1.03–1.37, p = 0.02 after exclusion of 1 SNP associated with AD. dAdjusted for age, sex and 6 population eigenvectors in analyses of dementia probability. eBonferroni corrected p = 0.08. fAdjusted for age, sex, education, apolipoprotein E ɛ4 status, depressive symptoms, body mass index, and season of 25(OH)D collection. gIV estimated OR per SD decrease in natural log 25(OH)D = 1.19 (0.96, 1.45), p = 0.11 and 1.26 (1.00, 1.60), p = 0.05 after exclusion of rs2282679 due to potential pleiotropic effects and rs12785878 as an ancestry marker, respectively.
One study used a combination of genetic variants (rs2282679 in GC, rs12785878 near DHCR7, rs10741657 near CYP2R1 and rs6013897 in CYP24A1), to examine the association between genetically instrumented 25-hydroxyvitamin D [25(OH)D] and AD risk, reporting some evidence for an inverse association (odds ratio [OR] = 1.25, 95% confidence interval [CI] = 1.03–1.51, p = 0.02) [23]. However, when rs2282679 in GC was excluded due to potential pleiotropic effects [29, 30], there was no evidence for the association with AD (OR = 1.19, 95% CI = 0.96, 1.45, p = 0.11). Further analyses separating the genetic instrument into SNPs involved in vitamin D metabolism (rs2282679 and rs10741657) and synthesis (rs12785878 and rs6013897), again suggested the association may be driven by rs2282679 in GC (Table 3). Another study used two SNPs in the GC as a GRS, and found no support for a causal association with global cognition [20]. Two studies in Chinese men and women, respectively reported no significant association between testosterone [22] or 17β-estradiol [24] and global cognition (Table 3).
Telomere length
Shorter telomeres were statistically significantly associated with increased odds of AD in a study using the IGAP data [25] (Table 3). Additional results for individual SNPs are provided in Supplementary Table 4 with two out of seven SNPs (rs2736100 and rs9420907) providing evidence for a causal association with AD when used as single SNP IVs [25].
DISCUSSION
MR is increasingly used to provide support for causal effects where RCTs are impractical or unethical. In this systematic review, we included 18 studies using this methodology to examine causal relationships between risk factors and global cognitive function or dementia. Evidence for a causal association was seen for shorter telomeres and higher odds of AD demonstrating that MR can provide novel causal insight for exposures that an RCT cannot be designed for. There was also some suggestion of a causal relationship between higher SBP and higher smoking quantity with lower odds of AD, and higher fasting glucose, HDL, insulin sensitivity and homocysteine with higher odds of AD. Evidence for the role of vitamin D in AD was inconclusive, with some evidence for a causal association obtained from a study using a variant coding the vitamin D binding protein. While there was no evidence to support causal relationships with other risk factors, we cannot rule them out because for most exposures, data was only available from one study, with relatively modest sample sizes (n < 10,000) in half of the studies.
Power in MR studies is determined largely by the strength of the instrument, typically evaluated as the proportion of variation in the exposure explained by the IV; the weaker the instrument (indicated by smaller R2), the larger the sample size required to achieve the same power. In Fig. 2, we present power calculations for diverse but realistic scenarios for instruments with R2 ranging from 0.1% to 5.0%, with an odds ratio (OR) of 1.5 (binary outcome) or 0.15 standard deviations (standardized continuous outcome). For example, for a binary outcome with a weak IV predicting only 0.1% of the variance in the exposure, a sample size of over 200,000 is required to achieve 80% power. Even studies using the IGAP data (n = 54,162) are likely to be underpowered to detect small effects if they use weak instruments. When using a weak genetic IV for education (R2 = 0.11%) Nguyen and colleagues [11] did not find a significant causal relationship with dementia probability. However, when they used a stronger non-genetic IV (compulsory schooling laws, R2 = 0.29%) with a larger sample size, each year of education reduced the dementia probability by 0.095 (95% confidence interval (CI): – 0.148, – 0.042; p < 0.001), demonstrating their MR analysis was possibly underpowered.
One study using summary statistics from genome-wide association studies to maximize power, found a significant causal effect for shorter telomeres and higher odds of AD in line with previous non-genetic studies [31]. The causal nature of the association is an important finding given that telomere length has been implicated in AD pathology (tau hyperphosphorylation and Aβ accumulation) and pathogenesis (oxidative stress and inflammation) [32].
One [12] of the two MR studies [12, 15] suggested that the association between higher smoking quantity and lower AD risk may be due to altered nicotinic receptor function. However, there is insufficient evidence for neuroprotective effects of nicotine in humans and findings indicating smoking might decrease AD risk are likely an artefact of survival bias [33] present also in MR studies. This significant finding may also reflect a diagnostic bias as smokers are more likely to suffer a stroke [34] and therefore to be diagnosed with vascular or mixed dementia. Non-genetic observational studies suggested light to moderate alcohol consumption may decrease dementia risk [35] but MR studies included in our systematic review did not support a causal association with dementia-related outcomes [13, 14]. Another MR study investigating the link between alcohol consumption and specific cognitive domains in participants of European ancestry (n = 34,452) also did not provide evidence for causal associations with immediate and delayed word recall, verbal fluency and processing speed [36].
While higher genetically determined SBP was statistically significantly associated with lower AD risk, it was also related to higher probability of taking antihypertensive medication which may influence this relationship [12]. A recent systematic review of non-genetic observational studies suggested antihypertensive medication might be protective against AD [37]. However, another systematic review and meta-analysis did not find a significant association of antihypertensive medication with incident all-cause dementia when restricted to RCTs (OR = 0.89, 95% CI = 0.74, 1.07) [38]. As the link between SBP and all-cause dementia or vascular dementia was not investigated by our included studies, we cannot exclude a potential diagnostic bias as those with vascular risk factors may be more likely to receive a diagnosis of vascular or mixed dementia rather than AD.
Fig. 2
Power curves for genetic instruments with R2 of 0.1%, 0.2%, 0.4%, 0.8%, 1.6%, and 5.0% when outcomes are binary (A) and continuous (B). The power functions were taken from Burgess [46]. For binary outcomes, two-sided type 1 error, effect size (in odds ratio for 1 standard deviation (SD) increase in the exposure), and case to control ratio were set to be 0.05, 1.5, and 1 : 3, respectively. For continuous outcomes, two-sided type 1 error and effect size (in SD for 1 SD increase in the exposure) were set to be 0.05 and 0.15, respectively.
![Power curves for genetic instruments with R2 of 0.1%, 0.2%, 0.4%, 0.8%, 1.6%, and 5.0% when outcomes are binary (A) and continuous (B). The power functions were taken from Burgess [46]. For binary outcomes, two-sided type 1 error, effect size (in odds ratio for 1 standard deviation (SD) increase in the exposure), and case to control ratio were set to be 0.05, 1.5, and 1 : 3, respectively. For continuous outcomes, two-sided type 1 error and effect size (in SD for 1 SD increase in the exposure) were set to be 0.05 and 0.15, respectively.](https://content.iospress.com:443/media/jad/2018/64-1/jad-64-1-jad180013/jad-64-jad180013-g002.jpg)
One study [19] suggested a causal association between increased homocysteine levels and increased odds of AD. However, the study population was of mixed ancestry and stratified analyses revealed statistically significant associations in a population of Asian and other ancestry but not in Caucasians [19]. Two sample MR used in this study assumes that the genetic effects that are being compared are derived from the same population [39], questioning the appropriateness of basing the primary result on a mixed population when the IGAP data used was limited to European ancestry.
For vitamin D, in line with previous non-genetic observational findings [40], there was some evidence for a causal link with AD [23]. However, this association appeared to be driven by a SNP in the GC, coding the vitamin D binding protein. As GC polymorphisms are the primary determinants of circulating concentrations of vitamin D binding protein rather than 25(OH)D [41], and as they have been shown to affect 25(OH)D bioavailability to the target cells [29], it is difficult to directly translate related findings to reflect possible causal associations with 25(OH)D concentrations [42]. Interestingly, while another study which used two SNPs in GC as IV [20] did not find any association with global cognition, there was evidence for a causal association with specific cognitive domains such as executive function, psychomotor speed and confrontational naming. Other studies have suggested significant links with word recall for SNPs in GC [43] and VDR [44] genes, and with psychomotor speed for a SNP in the VDR gene [44], highlighting the interest in further studies using variants involved in vitamin D metabolism.
We have conducted the first systematic review of studies that applied an MR framework to test for causal associations between risk factors and global cognitive function or dementia. Our review considered a broad range of exposures due to a comprehensive search strategy including forward and backward citation searches and no restrictions regarding publication date or language. As most of the included studies did not perform power calculations, it is unclear whether there are few causal relationships, or the data are insufficient to detect the magnitude of the effect. Moreover, results of MR and observational studies may represent different underlying processes as exposures in MR studies reflect lifelong differences determined by differences in genetic variants [45] whereas exposures in observational studies are determined by genetic and non-genetic influences at a given point in time. A number of studies used a two sample approach, taking advantage of GWAS with the largest sample sizes to date for each risk factor and the summary statistics for AD published by the IGAP consortium. Many of the included risk factors have a polygenic architecture where each associated SNP has very low predictive power meaning the authors were dependent upon adequately powered GWAS to select their genetic instruments. Weak instrument bias can be overcome by combining multiple associated SNPs to increase the variance explained and ultimately the strength of the instrument. However, as more SNPs are included the risk of pleiotropic effects increases, violating one of the main assumptions of MR. This was potentially the case for total, HDL and LDL cholesterol, where excluding one variant also associated with AD meant the associations were no longer significant [12]. There was considerable variation in mean age between the included studies (47–74 years), and the etiology and risk factor profiles of different dementia subtypes are known to vary by age. Differences in the samples incorporated in the included studies may therefore have contributed to the heterogeneity observed. Potential survival and diagnostic bias also remain key limitations of the original included studies.
Conclusions
There is convincing evidence for a causal association of shorter telomeres with higher AD risk. Uncertainties remain regarding other modifiable risk factors as existing MR studies do not in themselves provide convincing evidence for a causal link. Given the methodological shortcomings identified and the relatively limited number of MR studies it is important to consider these findings cautiously and in combination with other evidence from observational studies and trials. That said observational studies do not in themselves demonstrate causality and may be consistently confounded. Furthermore, RCTs can be expensive, impractical or unethical to conduct. Larger well-designed MR studies are therefore likely to be helpful in establishing the causal status of these dementia risk factors.
ACKNOWLEDGMENTS
This work was supported by the Mary Kinross Charitable Trust (DJL and EK), Halpin Trust (DJL, EK and IL), the James Tudor Foundation (DJL and EK), National Institute for Health Research (NIHR) Collaboration for Leadership in Applied Health Research and Care (CLAHRC) for the South West Peninsula (IL, JTC, AB and DJL) and the National Institute on Aging of the National Institutes of Health under Award Number RF1AG055654 (DJL).. None of the funding sources had any role in the design of the study, in the analysis and interpretation of the data or in the preparation of the manuscript. The views expressed in this publication are those of the authors and not necessarily those of the NHS, the NIHR, the Department of Health in England or the National Institutes of Health.
An early version of this study was presented in part at the Alzheimer’s Association International Conference (AAIC), July 16–20, 2017, in London, UK.
Authors’ disclosures available online (https://www.j-alz.com/manuscript-disclosures/18-0013r1).
SUPPLEMENTARY MATERIAL
[1] The supplementary material is available in the electronic version of this article: http://dx.doi.org/10.3233/JAD-180013.
REFERENCES
[1] | Prince M , Bryce R , Albanese E , Wimo A , Ribeiro W , Ferri CP . ((2013) ) The global prevalence of dementia: A systematic review and metaanalysis. Alzheimers Dement 9: , 63–75. |
[2] | Norton S , Matthews FE , Barnes DE , Yaffe K , Brayne C . ((2014) ) Potential for primary prevention of Alzheimer’s disease: An analysis of population-based data. Lancet Neurol 13: , 788–794. |
[3] | Smith GD , Ebrahim S . ((2003) ) ‘Mendelian randomization’: Can genetic epidemiology contribute to understanding environmental determinants of disease?. Int J Epidemiol 32: , 1–22. |
[4] | Burgess S , Butterworth A , Malarstig A , Thompson SG . ((2012) ) Use of Mendelian randomisation to assess potential benefit of clinical intervention. BMJ 345: , 7325. |
[5] | Centre for Reviews and Dissemination ((2009) ) Systematic reviews: CRD’s guidance for undertaking reviews in health care. Centre for Reviews and Disination, University of York. |
[6] | Lawlor DA , Harbord RM , Sterne JA , Timpson N , Davey Smith G . ((2008) ) Mendelian randomization: Using genes as instruments for making causal inferences in epidemiology. Stat Med 27: , 1133–1163. |
[7] | Cruchaga C , Kauwe JS , Nowotny P , Bales K , Pickering EH , Mayo K , Bertelsen S , Hinrichs A , Fagan AM , Holtzman DM , Morris JC , Goate AM . ((2012) ) Cerebrospinal fluid APOE levels: An endophenotype for genetic studies for Alzheimer’s disease. Hum Mol Genet 21: , 4558–4571. |
[8] | Marioni RE , Deary IJ , Murray GD , Lowe GD , Strachan MW , Luciano M , Houlihan LM , Gow AJ , Harris SE , Rumley A , Stewart MC , Fowkes FG , Price JF . ((2011) ) Genetic associations between fibrinogen and cognitive performance in three ish cohorts. Behav Genet 41: , 691–699. |
[9] | Quinn TJ , Alghamdi J , Padmanabhan S , Porteous DJ , Smith BH , Hocking L , Deary IJ , Gallacher J , Messow M , Stott DJ . ((2015) ) Association between cognition and gene polymorphisms involved in thrombosis and haemostasis. Age 37: , 9820. |
[10] | Sohani ZN , Meyre D , de Souza RJ , Joseph PG , Gandhi M , Dennis BB , Norman G , Anand SS . ((2015) ) Assessing the quality of published genetic association studies in meta-analyses: The quality of genetic studies (Q-Genie) tool. BMC Genet 16: , 1–8. |
[11] | Nguyen TT , Tchetgen Tchetgen EJ , Kawachi I , Gilman SE , Walter S , Liu SY , Manly JJ , Glymour MM . ((2016) ) Instrumental variable approaches to identifying the causal effect of educational attainment on dementia risk. Ann Epidemiol 26: , e71–73. |
[12] | Østergaard SD , Mukherjee S , Sharp SJ , Proitsi P , Lotta LA , Day F , Perry JR , Boehme KL , Walter S , Kauwe JS , Gibbons LE , Larson EB , Powell JF , Langenberg C , Crane PK , Wareham NJ , Scott RA . ((2015) ) Associations between potentially modifiable risk factors and Alzheimer disease: A Mendelian randomization study. PLoS Med 12: , 1001841. |
[13] | Almeida OP , Hankey GJ , Yeap BB , Golledge J , Flicker L . ((2014) ) Alcohol consumption and cognitive impairment in older men: A mendelian randomization study. Neurology 82: , 1038–1044. |
[14] | Au Yeung SL , Jiang CQ , Cheng KK , Liu B , Zhang WS , Lam TH , Leung GM , Schooling CM . ((2012) ) Evaluation of moderate alcohol use and cognitive function among men using a Mendelian randomization design in the Guangzhou biobank cohort study. Am J Epidemiol 175: , 1021–1028. |
[15] | North TL , Palmer TM , Lewis SJ , Cooper R , Power C , Pattie A , Starr JM , Deary IJ , Martin RM , Aihie Sayer A , Kumari M , Cooper C , Kivimaki M , Kuh D , Ben-Shlomo Y , Day IN . ((2015) ) Effect of smoking on physical and cognitive capability in later life: A multicohort study using observational and genetic approaches. BMJ Open 5: , 008393. |
[16] | Kwok MK , Leung GM , Schooling CM . ((2016) ) Habitual coffee consumption and risk of type 2 diabetes, ischemic heart disease, depression and Alzheimer’s disease: A Mendelian randomization study. Sci Rep 6: , 36500. |
[17] | Mukherjee S , Walter S , Kauwe JS , Saykin AJ , Bennett DA , Larson EB , Crane PK , Glymour MM . ((2015) ) Genetically predicted body mass index and Alzheimer’s disease-related phenotypes in three large samples: Mendelian randomization analyses. Alzheimers Dement 11: , 1439–1451. |
[18] | Proitsi P , Lupton MK , Velayudhan L , Newhouse S , Fogh I , Tsolaki M , Daniilidou M , Pritchard M , Kloszewska I , Soininen H , Mecocci P , Vellas B , Williams J , Stewart R , Sham P , Lovestone S , Powell JF . ((2014) ) Genetic predisposition to increased blood cholesterol and triglyceride lipid levels and risk of Alzheimer disease: A Mendelian randomization analysis. PLoS Med 11: , 1001713. |
[19] | Hu Q , Teng W , Li J , Hao F , Wang N . ((2016) ) Homocysteine and Alzheimer’s disease: Evidence for a causal link from Mendelian randomization. J Alzheimers Dis 52: , 747–756. |
[20] | Kueider AM , Tanaka T , An Y , Kitner-Triolo MH , Palchamy E , Ferrucci L , Thambisetty M . ((2016) ) State- and trait-dependent associations of vitamin-D with brain function during aging. Neurobiol Aging 39: , 38–45. |
[21] | Walter S , Marden JR , Kubzansky LD , Mayeda ER , Crane PK , Chang SC , Cornelis M , Rehkopf DH , Mukherjee S , Glymour MM . ((2016) ) Diabetic phenotypes and late-life dementia risk: A mechanism-specific Mendelian randomization study. Alzheimer Dis Assoc Disord 30: , 15–20. |
[22] | Zhao JV , Lam TH , Jiang C , Cherny SS , Liu B , Cheng KK , Zhang W , Leung GM , Schooling CM . ((2016) ) A Mendelian randomization study of testosterone and cognition in men. Sci Rep 6: , 21306. |
[23] | Mokry LE , Ross S , Morris JA , Manousaki D , Forgetta V , Richards JB . ((2016) ) Genetically decreased vitamin D and risk of Alzheimer disease. Neurology 87: , 2567–2574. |
[24] | Au Yeung SL , Jiang C , Cheng KK , Zhang W , Lam TH , Leung GM , Schooling CM . ((2016) ) Genetically predicted 17beta-estradiol, cognitive function and depressive symptoms in women: A Mendelian randomization in the Guangzhou Biobank Cohort Study. Prev Med 88: , 80–85. |
[25] | Zhan Y , Song C , Karlsson R , Tillander A , Reynolds CA , Pedersen NL , Hagg S . ((2015) ) Telomere length shortening and Alzheimer disease–a Mendelian randomization study. JAMA Neurol 72: , 1202–1203. |
[26] | Macgregor S , Lind PA , Bucholz KK , Hansell NK , Madden PA , Richter MM , Montgomery GW , Martin NG , Heath AC , Whitfield JB . ((2009) ) Associations of ADH and ALDH2 gene variation with self report alcohol reactions, consumption and dependence: An integrated analysis. Hum Mol Genet 18: , 580–593. |
[27] | Lambert JC , Ibrahim-Verbaas CA , Harold D , Naj AC , Sims R , Bellenguez C , DeStafano AL , Bis JC , Beecham GW , Grenier-Boley B , Russo G , Thorton-Wells TA , Jones N , Smith AV , Chouraki V , Thomas C , Ikram MA , Zelenika D , Vardarajan BN , Kamatani Y , Lin CF , Gerrish A , Schmidt H , Kunkle B , Dunstan ML , Ruiz A , Bihoreau MT , Choi SH , Reitz C , Pasquier F , Cruchaga C , Craig D , Amin N , Berr C , Lopez OL , De Jager PL , Deramecourt V , Johnston JA , Evans D , Lovestone S , Letenneur L , Morón FJ , Rubinsztein DC , Eiriksdottir G , Sleegers K , Goate AM , Fiévet N , Huentelman MW , Gill M , Brown K , Kamboh MI , Keller L , Barberger-Gateau P , McGuiness B , Larson EB , Green R , Myers AJ , Dufouil C , Todd S , Wallon D , Love S , Rogaeva E , Gallacher J , St George-Hyslop P , Clarimon J , Lleo A , Bayer A , Tsuang DW , Yu L , Tsolaki M , Bossù P , Spalletta G , Proitsi P , Collinge J , Sorbi S , Sanchez-Garcia F , Fox NC , Hardy J , Deniz Naranjo MC , Bosco P , Clarke R , Brayne C , Galimberti D , Mancuso M , Matthews F ; European Alzheimer’s Disease Initiative (EADI); Genetic and Environmental Risk in Alzheimer’s Disease; Alzheimer’s Disease Genetic Consortium; Cohorts for Heart and Aging Research in Genomic Epidemiology, Moebus S , Mecocci P , Del Zompo M , Maier W , Hampel H , Pilotto A , Bullido M , Panza F , Caffarra P , Nacmias B , Gilbert JR , Mayhaus M , Lannefelt L , Hakonarson H , Pichler S , Carrasquillo MM , Ingelsson M , Beekly D , Alvarez V , Zou F , Valladares O , Younkin SG , Coto E , Hamilton-Nelson KL , Gu W , Razquin C , Pastor P , Mateo I , Owen MJ , Faber KM , Jonsson PV , Combarros O , O’Donovan MC , Cantwell LB , Soininen H , Blacker D , Mead S , Mosley TH Jr , Bennett DA , Harris TB , Fratiglioni L , Holmes C , de Bruijn RF , Passmore P , Montine TJ , Bettens K , Rotter JI , Brice A , Morgan K , Foroud TM , Kukull WA , Hannequin D , Powell JF , Nalls MA , Ritchie K , Lunetta KL , Kauwe JS , Boerwinkle E , Riemenschneider M , Boada M , Hiltuenen M , Martin ER , Schmidt R , Rujescu D , Wang LS , Dartigues JF , Mayeux R , Tzourio C , Hofman A , Nöthen MM , Graff C , Psaty BM , Jones L , Haines JL , Holmans PA , Lathrop M , Pericak-Vance MA , Launer LJ , Farrer LA , van Duijn CM , Van Broeckhoven C , Moskvina V , Seshadri S , Williams J , Schellenberg GD , Amouyel P . ((2013) ) Meta-analysis of 74,046 individuals identifies 11 new susceptibility loci for Alzheimer’s disease. Nat Genet 45: , 1452–1458. |
[28] | Verghese PB , Castellano JM , Holtzman DM . ((2011) ) Apolipoprotein E in Alzheimer’s disease and other neurological disorders. Lancet Neurol 10: , 241–252. |
[29] | Chun RF , Lauridsen AL , Suon L , Zella LA , Pike JW , Modlin RL , Martineau AR , Wilkinson RJ , Adams J , Hewison M . ((2010) ) Vitamin D-binding protein directs monocyte responses to 25-hydroxy- and 1,25-dihydroxyvitamin D. J Clin Endocrinol Metab 95: , 3368–3376. |
[30] | Vimaleswaran KS , Cavadino A , Berry DJ , Jorde R , Dieffenbach AK , Lu C , Alves AC , Heerspink HJ , Tikkanen E , Eriksson J , Wong A , Mangino M , Jablonski KA , Nolte IM , Houston DK , Ahluwalia TS , van der Most PJ , Pasko D , Zgaga L , Thiering E , Vitart V , Fraser RM , Huffman JE , de Boer RA , Schottker B , Saum KU , McCarthy MI , Dupuis J , Herzig KH , Sebert S , Pouta A , Laitinen J , Kleber ME , Navis G , Lorentzon M , Jameson K , Arden N , Cooper JA , Acharya J , Hardy R , Raitakari O , Ripatti S , Billings LK , Lahti J , Osmond C , Penninx BW , Rejnmark L , Lohman KK , Paternoster L , Stolk RP , Hernandez DG , Byberg L , Hagstrom E , Melhus H , Ingelsson E , Mellstrom D , Ljunggren O , Tzoulaki I , McLachlan S , Theodoratou E , Tiesler CM , Jula A , Navarro P , Wright AF , Polasek O , Wilson JF , Rudan I , Salomaa V , Heinrich J , Campbell H , Price JF , Karlsson M , Lind L , Michaelsson K , Bandinelli S , Frayling TM , Hartman CA , Sorensen TI , Kritchevsky SB , Langdahl BL , Eriksson JG , Florez JC , Spector TD , Lehtimaki T , Kuh D , Humphries SE , Cooper C , Ohlsson C , Marz W , de Borst MH , Kumari M , Kivimaki M , Wang TJ , Power C , Brenner H , Grimnes G , van der Harst P , Snieder H , Hingorani AD , Pilz S , Whittaker JC , Jarvelin MR , Hypponen E . ((2014) ) Association of vitamin D status with arterial blood pressure and hypertension risk: A mendelian randomisation study. Lancet Diabetes Endocrinol 2: , 719–729. |
[31] | Forero DA , Gonzalez-Giraldo Y , Lopez-Quintero C , Castro-Vega LJ , Barreto GE , Perry G . ((2016) ) Meta-analysis of telomere length in Alzheimer’s disease. J Gerontol A Biol Sci Med Sci 71: , 1069–1073. |
[32] | Cai Z , Yan LJ , Ratka A . ((2013) ) Telomere shortening and Alzheimer’s disease. Neuromolecular Med 15: , 25–48. |
[33] | Durazzo TC , Mattsson N , Weiner MW . ((2014) ) Smoking and increased Alzheimer’s disease risk: A review of potential mechanisms.S122-S. Alzheimers Dement 10: , 145. |
[34] | Shah RS , Cole JW . ((2010) ) Smoking and stroke: The more you smoke the more you stroke. Expert Rev Cardiovasc Ther 8: , 917–932. |
[35] | Ilomaki J , Jokanovic N , Tan EC , Lonnroos E . ((2015) ) Alcohol consumption, dementia and cognitive decline: An overview of systematic reviews. Curr Clin Pharmacol 10: , 204–212. |
[36] | Kumari M , Holmes MV , Dale CE , Hubacek JA , Palmer TM , Pikhart H , Peasey A , Britton A , Horvat P , Kubinova R , Malyutina S , Pajak A , Tamosiunas A , Shankar A , Singh-Manoux A , Voevoda M , Kivimaki M , Hingorani AD , Marmot MG , Casas JP , Bobak M . ((2014) ) Alcohol consumption and cognitive performance: A Mendelian randomization study. Addiction 109: , 1462–1471. |
[37] | Xu W , Tan L , Wang H-F , Jiang T , Tan M-S , Tan L , Zhao Q-F , Li J-Q , Wang J , Yu J-T . ((2015) ) Meta-analysis of modifiable risk factors for Alzheimer’s disease. J Neurol Neurosurg Psychiatry 86: , 1299–306. |
[38] | Levi Marpillat N , Macquin-Mavier I , Tropeano AI , Bachoud-Levi AC , Maison P . ((2013) ) Antihypertensive classes, cognitive decline and incidence of dementia: A network meta-analysis. J Hypertens 31: , 1073–1082. |
[39] | Burgess S , Scott RA , Timpson NJ , Davey Smith G , Thompson SG , Consortium E-I . ((2015) ) Using published data in Mendelian randomization: A blueprint for efficient identification of causal risk factors. Eur J Epidemiol 30: , 543–552. |
[40] | Sommer I , Griebler U , Kien C , Auer S , Klerings I , Hammer R , Holzer P , Gartlehner G . ((2017) ) Vitamin D deficiency as a risk factor for dementia: A systematic review and meta-analysis. BMC Geriatr 17: , 16. |
[41] | Moy KA , Mondul AM , Zhang H , Weinstein SJ , Wheeler W , Chung CC , Mannisto S , Yu K , Chanock SJ , Albanes D . ((2014) ) Genome-wide association study of circulating vitamin D-binding protein. Am J Clin Nutr 99: , 1424–1431. |
[42] | Berry D , Hypponen E . ((2011) ) Determinants of vitamin D status: Focus on genetic variations. Curr Opin Nephrol Hypertens 20: , 331–336. |
[43] | Alfred T , Ben-Shlomo Y , Cooper R , Hardy R , Deary IJ , Elliott J , Harris SE , Hypponen E , Kivimaki M , Kumari M , Maddock J , Power C , Starr JM , Kuh D , Day IN . ((2013) ) Genetic variants influencing biomarkers of nutrition are not associated with cognitive capability in middle-aged and older adults. J Nutr 143: , 606–612. |
[44] | Jorde R , Mathiesen EB , Rogne S , Wilsgaard T , Kjaergaard M , Grimnes G , Schirmer H . ((2015) ) Vitamin D and cognitive function: The Tromso Study. J Neurol Sci 355: , 155–161. |
[45] | Burgess S , Thompson SG . ((2015) ) Chapman & Hall/CRC. Mendelian randomization: Methods for using genetic variants in causal estimation. |
[46] | Burgess S . ((2014) ) Sample size and power calculations in Mendelian randomization with a single instrumental variable and a binary outcome. Int J Epidemiol 43: , 922–929. |