Tocotrienol-Rich Fraction of Palm Oil Improves Behavioral Impairments and Regulates Metabolic Pathways in AβPP/PS1 Mice
Abstract
We have recently shown that the tocotrienol-rich fraction (TRF) of palm oil, a mixture of vitamin E analogs, improves amyloid pathology in vitro and in vivo. However, precise mechanisms remain unknown. In this study, we examined the effects of long-term (10 months) TRF treatment on behavioral impairments and brain metabolites in (15 months old) AβPP/PS1 double transgenic (Tg) Alzheimer’s disease (AD) mice. The open field test, Morris water maze, and novel object recognition tasks revealed improved exploratory activity, spatial learning, and recognition memory, respectively, in TRF-treated Tg mice. Brain metabolite profiling of wild-type and Tg mice treated with and without TRF was performed using ultrahigh performance liquid chromatography (UHPLC) coupled to high-resolution accurate mass (HRAM)-orbitrap tandem mass spectrometry (MS/MS). Metabolic pathway analysis found perturbed metabolic pathways that linked to AD. TRF treatment partly ameliorated metabolic perturbations in Tg mouse hippocampus. The mechanism of this pre-emptive activity may occur via modulation of metabolic pathways dependent on Aβ interaction or independent of Aβ interaction.
INTRODUCTION
Alzheimer’s disease (AD) is an irreversible, progressive neurodegenerative disorder and the most prevalent form of dementia among the elderly. World-wide, 47 million people suffer from AD or related dementia, and this number is projected to reach 131.5 million by 2050 [1]. AD is characterized by a gradual impairment of memory and cognition, accompanied by pathological hallmarks such as accumulation of amyloid plaques and tau protein neurofibrillary tangles [2]. A combination of genetic, environmental, and lifestyle factors appear to increase AD risk [3].
Amyloid pathology is observed about 20 years before clinical onset [4, 5]. Therefore, treatment during very early stages of AD, including the preclinical stage, may halt or slow disease progression. However, treatments for preclinical AD patients should have minimal side effects. The development of therapeutic drugs from natural food products is thus of great interest. Natural-source antioxidants are one promising therapeutic strategy against AD. However, clinical studies conducted to date have reported inconsistent therapeutic efficacy [6–8].
Vitamin E, a lipid-soluble antioxidant, is classified into tocopherols and tocotrienols. In addition to free radical scavenging activity, vitamin E also modulates signal transduction [9, 10]. Both tocopherol and tocotrienol classes consist of alpha (α), beta (β), gamma (γ), and delta (δ) isomers. The tocotrienols, while less well characterized than tocopherols, have attracted attention due to more potent antioxidant activity and associations with human pathologies [11, 12]. Tocotrienols are usually minor vitamin E components in plants, but relatively high levels are found in palm oil extracted from Elaeis guineensis (African oil palm tree). Vitamin E in palm oil contains nearly 70% tocotrienols [13]. The unique structure of tocotrienols, a short tail with 3 double bonds, allows these molecules to penetrate easily into saturated fatty layers around the brain and liver [14]. Tocotrienols have demonstrated neuroprotective effects in vitro and in vivo [15, 16], and combining tocotrienols and tocopherols is believed to provide synergistic protection and reduce AD risk at an advanced age [17, 18]. We also reported that the treatment of tocotrienol-rich fraction (TRF) on AβPPswe/PS1dE9 (AβPP/PS1) double Tg mice for 10 months improved working memory and amyloid pathology in the brain [19]. Using the same treatment protocols as the paper to different individual wild-type (WT) and AβPP/PS1 double Tg mice, we compared behavioral indices of learning and brain metabolite profiles, in order to investigate the underlying mechanism. A number of metabolomic studies performed in the last several years have revealed the complexity of the neurochemical changes associated with AD [20–23]. Therefore, we also examined TRF effects in AD by untargeted metabolomics. To our knowledge, this is the first brain metabolomics study to investigate the neurochemical effects of TRF using ultrahigh-performance liquid chromatography (UHPLC) coupled to HRAM-orbitrap tandem mass spectrometry (MS/MS).
MATERIALS AND METHODS
Animals
All animal protocols were approved by the Animal Care and Use Committee of the Shiga University of Medical Science (Ethical committee approval number: 2013-6-12H). AβPP/PS1 double transgenic mice expressing a chimeric mouse/human amyloid protein precursor (Mo/HuAPP695swe) and a mutant human presenilin 1 with deletion at exon 9 (PS1-dE9) were obtained from Jackson Laboratory (Bar Harbor, ME, USA). Heterozygous females were bred with wild-type (WT) males. The offspring were genotyped by polymerase chain reaction (PCR) using the APP primers forward-GACTGACCACTCGACCAGGTTCTG/reverse-CTTGTAAGTTGGATTCTCATATCCG, and the following two presenilin 1 sequences forward-CTCTTTGTGACTATGTGGACTGATGTCGG/reverse-GTGGATAACCCCTCCCCCAGCCTAGACC and forward- ATTAGAGAACGGCAGGAGCA/reverse-GCCATGAGGGCACTAATCAT.
Heterozygous (n = 26) and WT (n = 12) male mice were used for experiments. All mice were housed in a controlled environment (23°C, 12/12 h light/dark cycle, light period from 8 am to 8 pm) with ad libitum access to food and water. One hundred grams of standard chow contains 24.88% crude protein, 5.03% crude fat, 49.78% of nitrogen-free extract (NFE), and 343.90 kcal energy (CLEA, Japan). This diet also contained 0.064 mg/g vitamin E, equivalent to a daily intake of 0.192 mg.
TRF administration
The TRF was dissolved at 12 mg/mL in palm oil stripped of vitamin E (PO) as previously described [19]. Briefly, five-month-old AβPP/PS1 mice were divided into three groups, Tg-ctrl, Tg-TRF, and Tg-PO, receiving daily water (Tg-ctrl, 5 mL/kg body weight), TRF (Tg-TRF, 60 mg/kg body weight), or PO (Tg-PO, 5 mL/kg body weight) by oral gavage from 5 to 15 months of age. The TRF in this study consisted of a mixture of α-tocopherol (23.40%) and α, β, γ, and δ-tocotrienol (27.30% α-tocotrienol; 3.34% β-tocotrienol, 35.51% γ-tocotrienol and 10.45% δ-tocotrienol). Composition and molecular structure of TRF were detailed in Fig. 1A.
Fig. 1.
Schedule of tocotrienol-rich fraction (TRF) administration to AβPP/PS1 mice and the experimental design from 5 to 15 months of age. A) The composition of α-tocopherol and α-, β-, γ-, and δ-tocotrienol isomers in TRF. α-Tocopherol has a long tail with no double bond, while all tocotrienols have a unique short tail structure with three double bonds. B) Mice were divided into four groups: wild-type mice that received water (WT-ctrl, n = 12) and three groups of AβPP/PS1 mice that received water (Tg-ctrl, n = 8), tocotrienol-rich fraction (Tg-TRF, n = 9), or palm oil stripped of vitamin E as a vehicle control (Tg-PO, n = 9). Daily administration started at 5 months and continued until 15 months of age. All mice were subjected to behavioral tasks for 13 days before sacrifice. Brains were collected for metabolites profiling.
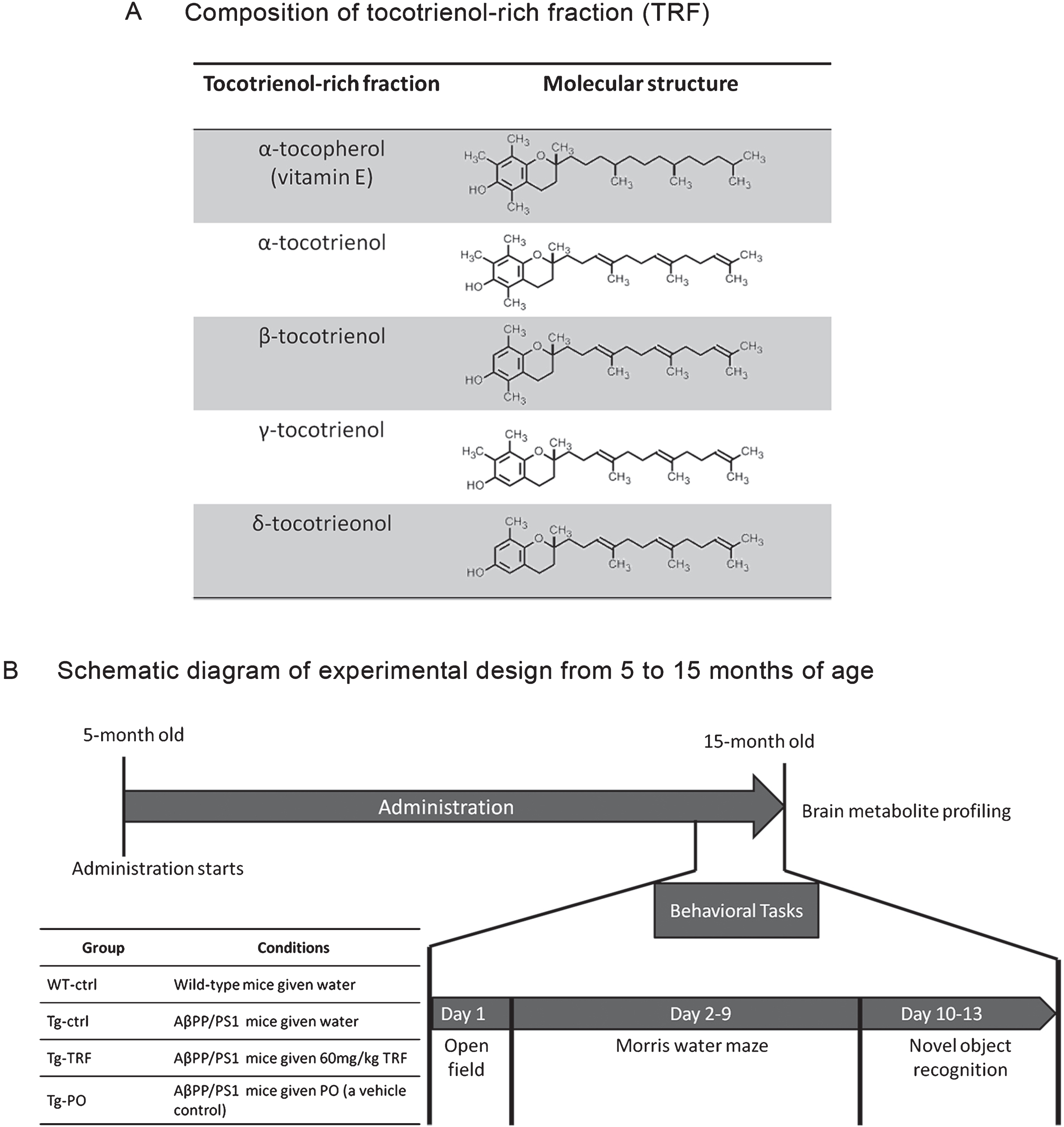
Behavioral tasks
All behavioral tasks were conducted at night. The timeline of the tasks is illustrated in Fig. 1B. Wild-type mice (WT-ctrl; n = 12) and AβPP/PS1 mice that received water (Tg-ctrl; n = 8), TRF (Tg-TRF; n = 9), or PO (Tg-PO; n = 9) were kept in the testing room at least 30 min before the test for acclimation. Extra-maze cues (laboratory furniture, lights, and several prominent visual features on the walls, as well as the location of the experimenter) were held constant throughout the experiment.
Open field test
An experimental chamber consisting of a 38-cm diameter home-built circular box with walls 22-cm high and elevated approximately 10 cm above the floor was used for analysis of locomotion, exploration, and anxiety. A camera was mounted centrally above the chamber and connected to a computerized tracking system (CompACT VAS Ver.3.0x, Muromachi, Tokyo, Japan). Each mouse was placed in the center of the chamber and allowed to explore freely for 5 min. The chamber was cleaned after every trial. Duration and frequency of rearing, time spent in the center, and frequency of central entries were analyzed.
Morris water maze test
The Morris water maze (MWM) task is frequently used to assess hippocampus-dependent spatial learning and memory in rodents [24]. This test was performed in a 120-cm diameter, 36-cm deep pool filled with water (20±1°C) made opaque with white non-toxic poster color. The pool was virtually divided into four quadrants with a clear platform of 10-cm diameter submerged approximately 1 cm beneath the surface in a designated target quadrant. The MWM protocol consisted of three different tests: place navigation, a probe test, and cued navigation. After completion of the MWM tasks, several performance variables were analyzed using CompACT VAS Ver.3.0x software.
Place navigation
The place navigation learning test was conducted over 6 consecutive days. Each mouse performed 5 trials per day at 30-min inter-trial intervals. During the test, a mouse was released facing the pool wall and allowed 90 s to locate and climb onto the hidden platform; any mouse that did not find the platform within 90 s was gently guided to the platform. After finding the platform, the mouse was allowed a 20-s rest period on the platform for orientation to the visual cues in the room. In the next trial, the mouse was placed at the wall in another quadrant. After completion of the test, escape latency and swim speed (cm/s) were analyzed off-line.
Probe test
A single probe test was conducted 24 h after the last place navigation trial. During this test, the platform was removed from the pool and the mouse was allowed to swim freely for 90 s. The fraction of time spent in the target quadrant and the number of platform position crossings were measured.
Cued navigation
During the cued navigation test, the platform was shifted to another (non-target) quadrant and raised 0.5 cm above the water surface. A red flag was mounted on the platform extending approximately 7 cm above the water surface. To ensure that the mouse was using this proximal cue to locate the platform, the prominent visual features on the walls were removed and the starting points were pseudorandomly selected. Each mouse received ten trials at 30-min inter-trial intervals. Again, the maximum searching time was 90 s and the mouse was allowed to remain on the platform for 20 s. Mice that did not find the platform within 90 s were gently guided to the platform. If the average latency of the last three trials was more than 20 s, the mouse was excluded from all analyses.
Novel object recognition
The novel object recognition test was conducted according to a previous study [19] using a 38-cm diameter home-built circular box with walls 22-cm high. Wild-type mice (n = 12) and AβPP/PS1 mice that received water (n = 8), TRF (n = 9), or PO (n = 9) were kept in the testing room at least 30 min before the test for acclimation. The test was conducted over four consecutive days and consisted of four phases: pre-habituation, habituation, training, and testing. Mice were habituated with no objects in the open chamber for 5 min on day 1 (pre-habituation) and for 20 min on both day 2 and 3 (habituation). On day 4, each mouse was given a training trial followed by a testing trial. During the training trial, the mouse was placed in the chamber with two identical objects and allowed to explore for 10 min before return to the cage. In the test trial conducted 1 h later, the mouse was placed back into the same chamber for 5 min with one previous object and one novel object. All objects and the chamber were cleaned with 70% ethanol after each trial. Exploration was defined as sniffing (within 2 cm), pawing, or biting the object, but not leaning against or standing on the object. Explorations times for each object were measured and a recognition index calculated as the fraction of total exploration time spent exploring the novel object. To exclude effects of location preference independent of object familiarity/novelty, the ratio of exploration times of the two identical objects was measured during the training trial.
Location preference = Time exploring one of the identical objects/Total time exploring both identical objects×100%
Recognition index = Time exploring novel object/(Time exploring novel object + Time exploring familiar object)×100%
Untargeted metabolomics
Brain tissue collection and extraction
Mice were sacrificed by cervical dislocation 24 h after the last behavioral test (n = 3 for each group). Hippocampus, medial prefrontal cortex (mPFC), and striatum were dissected using a brain matrix (Mouse Brain Slicer Matrix) and sectioned at 1.0-mm coronal slice intervals (Zivic Instruments Inc., Pittsburgh, PA, USA) on a cold plate and immediately frozen on dry ice. The three brain regions and the remaining brain sample were transferred to individual tubes, weighed, and stored at –80 °C until assay. For metabolite analyses, the tissue sample was submerged in 1.35 mL of a water-methanol-chloroform (2:1.5:1, v/v/v) solution and homogenized using a pestle with the addition of glass beads. The mixture was sonicated on ice and then centrifuged at 16,000×g for 10 min at 4 °C. The tubes were subsequently transferred to a –20°C freezer and kept there overnight to allow residual chloroform to separate from the aqueous methanol phase. The two liquid phases were transferred to separate 1.5 mL microcentrifuge tubes and lyophilized under vacuum (Thermo Scientific Savant SPD 1010 SpeedVac, USA). Only the methanol phase was used for the current analysis. The dry residue was reconstituted in 20μL cold methanol and diluted to a final volume of 200μL with the initial LC mobile phase (0.1% formic acid in water).
Metabolomic analysis by UHPLC-MS/MS
All solvents were purchased from Fisher Scientific (New Jersey, USA) and were of liquid chromatography mass spectrometry (LC-MS) grade. Chromatography was performed on an UltiMate 3000 Rapid Separation Liquid Chromatography (RSLC) system (GmbH, Germany) coupled to a Thermo Scientific Q Exactive HF Orbitrap mass spectrometer (Bremen, Germany). The Chromeleon Xpress system and Thermo Xcalibur™ mass spectrometry data system were used as system controllers. Two microliters of extracted sample were injected (n = 3 injections for each sample) onto a syncronis C18, 100×2.1 mm, 1.7μm column (Thermo Scientific, MA, USA) operating at 55°C and separated using a binary mobile phase system. The auto-sampler temperature was maintained at 0–10°C and the order in which the samples were injected was randomized throughout the experiment. Solvents were delivered at a flow rate 0.45 mL/min, using water (Solvent A) and acetonitrile (Solvent B), both containing 0.1% formic acid. The gradient elution program was 99.5% B at t = 0 min, 50% B at t = 5.5 min, 2% B at 6–13 min, and 99.5% B at 13.1–15 min. Positive and negative ionization modes were employed under the following conditions: sheath gas flow rate of 20 arbitrary units (AU), auxiliary gas flow rate of 6 AU, sweep gas flow rate of 0 AU, capillary temperature 320°C, and spray voltage 3.5 kV. Mass spectra were acquired using full MS/dd-MS2 mode, in which MS2 is triggered if an ion with high intensity is found in full MS. The orbitrap mass spectrometer was operated in Fourier transform mass spectrometry (FTMS) full MS scan mode with a high mass resolution of 60,000 and scan range of 100–1,000 m/z. Furthermore, the samples were subjected to mass fragmentation analysis (FTMS, high energy collision-trap dissociation (HCD), (20, 40, and 60 normalized collision energy (NCE)), MS2) with an isolation window of 1.5 m/z and 15,000 FTMS.
The MS was first calibrated using Pierce LTQ Electrospray Ionization (ESI) Positive Ion and Negative Ion Calibration Solution (Thermo Scientific). We used quality control (QC) samples prepared by mixing an equal volume of each sample to assess the reproducibility and reliability of the LC-MS/MS system. This pooled QC sample was injected five times prior to individual sample analysis to ensure system equilibrium and then every nine sample runs to further monitor the stability of the analysis.
Data pre-processing
Raw data were processed using Thermo ScientificTM Compound DiscovererTM 2.0 software with a single workflow ‘Untargeted Metabolomics workflow with statistics and ID using mzCloud ChemSpider, Kyoto Encyclopedia of Genes and Genomes (KEGG) Pathways’. This processing workflow uses the ‘Detect Unknown Compounds’ node to find chromatographic peaks of unknown compounds (MW×RT) and the ‘Predict Compositions’ node to determine the possible elemental compositions. The processing workflow also included library search against the mzCloudTM high-resolution accurate mass (HRAM) fragmentation library, mass list search against the built-in HRAM EFS library, and map to KEGG pathways. Mass tolerance on every node was set at 5 ppm. The pre-processed data were then exported to.xlsx files for further multivariate analysis.
Multivariate statistics
Metabolomic profiles were compared among groups using SIMCA-PTM software (version 14.1, Umetrics AB, Umea, Sweden). First, data were subjected to multivariate analysis by principal component analysis (PCA) and orthogonal partial least squares-discriminant analysis (OPLS-DA). Before statistical analyses to extract relevant biological information, data sets are usually scaled and transformed to minimize the technical variability between individual samples [25]. Data were Pareto scaled and logarithmic transformed to approximate a normal distribution. The quality of the OPLS-DA model was described by the total amount of the variation of the X variables explained by the model (R2X(cum)), total amount of the variation of the Y variables explained by the model (R2Y(cum)) and total amount of the predictability of the model (Q2(cum)) values. Good models are obtained when the cumulative values of these parameters are greater than or equal to 0.4. Permutation testing was used to confirm the model validity. Potential features were selected according to a ‘variable important in the projection’ (VIP) score above 1.0, indicative of significant differences among groups. In addition, MetaboAnalyst 3.0 software was used to conduct t-tests and fold-change analysis [26]. False discovery rates (FDR) below 0.05 were regarded as statistically significant.
Features identification
Discriminant features (VIP >1.0 and significant by FDR<0.05) detected by LC-MS profiling were identified using experimental accurate mass by searching the Metlin library [27] and Human Metabolome Database (HMDB) [28–30] with a mass accuracy of 5 ppm. These online databases are linked to KEGG [31], PubChem [32], LIPID MAPS [33], and ChEBI [34] for further investigation. Putative identifications were also confirmed by comparing MS/MS spectra against the online mzCloud mass spectral database linked to Compound Discoverer 2.0 software.
Metabolic pathway analysis
To identify and visualize the metabolic pathways altered in Tg-ctrl and Tg-TRF mice, the previously identified features were analyzed using Metabolomic Pathway Analysis (MetPA) software [26]. For this, we selected the Mus musculus library and used the ‘Fisher’s Exact Test’ and ‘Relative-Betweenness Centrality’ algorithms for pathway enrichment analysis and pathway topological analysis, respectively. Pathways with impact values above 0.1 are considered most relevant [35]. The information in this knowledgebase application was obtained primarily from the HMDB and KEGG [36].
Data analysis
Data are presented as mean±standard error of the mean (S.E.M.). Data sets that passed the Shapiro-Wilk normality test were compared by the analysis of variance (ANOVA), followed by post hoc correction for pair-wise comparisons. Data not normally distributed were compared by the Kruskal-Wallis test. One-way ANOVA was applied to all the behavioral tests data except for escape latency and swimming speed. The comparisons were performed between independent grouping variables including WT-ctrl, Tg-ctrl, Tg-TRF, and Tg-PO. Dependent variables were stated in each figure. While two-way ANOVA was applied for escape latency and swimming speed in the Morris water maze test. Days/trials and groups (WT-ctrl, Tg-ctrl, Tg-TRF, and Tg-PO) were considered as factors (independent variables). Interaction effect was not tested because we wanted to see the effects of independent variables factor only. The insignificance level was set at 0.05 for all comparisons. Statistical analysis was performed using GraphPad Prism 7 (GraphPad Software; La Jolla, CA, USA).
RESULTS
TRF increases exploratory activity of AβPP/PS1 mice
Mice were first examined in an open field for locomotion, exploration, and anxiety behaviors as measured by the total number of movements, total path length traveled, number of line crossings, number of rearings, total rearing duration, time spent in the center, the number of central entries. Tg-ctrl mice exhibited exploratory deficits compared to WT-ctrl mice as indicated by fewer rearings and shorter total rearing time (p < 0.05) (Fig. 2A, B). ANOVA with post hoc correction revealed a significant effect of TRF supplementation on duration of rearing (p < 0.05), indicating enhanced exploration, while PO was without effect. Tg-ctrl mice also spent less time in the field center than WT-ctrl mice (p < 0.05). Tg-TRF, however, did not reveal significant differences in time spent in center and number of central entries after Bonferroni correction (Fig. 2C, D). Administration of PO had no influence on open field parameters. Other parameters such as the total number of movements, total path length traveled, and number of line crossings showed no significant difference among groups (data not shown).
Fig. 2.
Administration with the tocotrienol-rich fraction (TRF) of palm oil improves exploration activity in AβPP/PS1 mice as measured by the open field test. A, B) Tg-ctrl mice displayed significantly lower total rearing time and number of rearings compared to WT-ctrl mice, while Tg-TRF mice showed a significant improvement in total rearing time and a slight increase in rearing frequency. C, D) The time spent in the field center and the frequencies of central entries were slightly higher in both WT-ctrl and Tg-TRF mice compared to Tg-ctrl mice, although the difference was not significant. Data represent mean±S.E.M. [Bonferroni post hoc test after analysis of variance (WT-ctrl, n = 12; Tg-ctrl, n = 8; Tg-TRF; n = 9; Tg-PO, n = 9)]. **p < 0.01, *p < 0.05.
![Administration with the tocotrienol-rich fraction (TRF) of palm oil improves exploration activity in AβPP/PS1 mice as measured by the open field test. A, B) Tg-ctrl mice displayed significantly lower total rearing time and number of rearings compared to WT-ctrl mice, while Tg-TRF mice showed a significant improvement in total rearing time and a slight increase in rearing frequency. C, D) The time spent in the field center and the frequencies of central entries were slightly higher in both WT-ctrl and Tg-TRF mice compared to Tg-ctrl mice, although the difference was not significant. Data represent mean±S.E.M. [Bonferroni post hoc test after analysis of variance (WT-ctrl, n = 12; Tg-ctrl, n = 8; Tg-TRF; n = 9; Tg-PO, n = 9)]. **p < 0.01, *p < 0.05.](https://content.iospress.com:443/media/jad/2018/64-1/jad-64-1-jad170880/jad-64-jad170880-g002.jpg)
TRF improves spatial learning and memory of AβPP/PS1 mice
The Morris water maze test was conducted to study the effects of TRF on the spatial learning and memory deficits of AβPP/PS1 mice. The test was carried out according to the schematic diagram shown in Fig. 3A. After six days of training in the place navigation test, WT-ctrl and Tg-TRF showed significantly shorter escape latencies on training day 4, 5, and 6 compared to day 1, indicating faster learning of the escape platform location (Fig. 3B). On day six, Tg-ctrl mice took significantly more time to find the hidden platform (longer escape latency) compared to WT-ctrl mice (65 s versus 34 s, p < 0.01), indicative of a spatial learning disability, while Tg-TRF exhibited escape latencies comparable to WT-ctrl mice (40 s, p > 0.05, Fig. 3C). These results were not attributable to differences in swimming speed (Fig. 3D). Further, administration of PO had no effect on spatial learning. Collectively, these results demonstrate a pre-emptive effect of TRF on the spatial learning deficit in AβPP/PS1 mice.
Fig. 3.
Administration with tocotrienol-rich fraction (TRF) enhances spatial learning in AβPP/PS1 mice as measured by the Morris water maze task. A) Schematic diagram of the Morris water maze (MWM) procedure. All mice were subjected to place navigation training, a probe test, and cued navigation test over 8 days. B) Escape latencies to reach the platform at a fixed location during place navigation training (acquisition) from day 1 to day 6. WT-ctrl and Tg-TRF showed significantly shorter escape latencies on training days 4, 5, and 6 compared to day 1, while Tg-ctrl mice showed no reduction compared to day 1. C) On day 6 of place navigation training, Tg-ctrl mice took significantly longer to reach the platform than WT-ctrl mice, while Tg-TRF were quicker than Tg-ctrl mice. D) No significant differences in swim speed were observed among groups. E, F) A single probe test was conducted one day after the last place navigation training trial. Tg-ctrl mice spent less significantly time in the target quadrant and made fewer annulus crossings than WT-ctrl mice. G) On day 8, the cued navigation test (visible platform test) was conducted. Mice failing to reach a criterion of 20-s latency for the last three trials were considered impaired and removed from the MWM task analysis. No significant differences were detected among groups. For B, D, and G, data represent mean±S.E.M. [Tukey post hoc test after 2-way analysis of variance (WT-ctrl, n = 12; Tg-ctrl, n = 8; Tg-TRF; n = 9; Tg-PO, n = 9)]. *p < 0.05, **p < 0.01, ***p < 0.001, ****p < 0.0001. For C, E, and F, data represent mean±S.E.M. [Bonferroni post hoc test after analysis of variance (WT-ctrl, n = 12; Tg-ctrl, n = 8; Tg-TRF; n = 9; Tg-PO, n = 9)]. *p < 0.05, **p < 0.01.
![Administration with tocotrienol-rich fraction (TRF) enhances spatial learning in AβPP/PS1 mice as measured by the Morris water maze task. A) Schematic diagram of the Morris water maze (MWM) procedure. All mice were subjected to place navigation training, a probe test, and cued navigation test over 8 days. B) Escape latencies to reach the platform at a fixed location during place navigation training (acquisition) from day 1 to day 6. WT-ctrl and Tg-TRF showed significantly shorter escape latencies on training days 4, 5, and 6 compared to day 1, while Tg-ctrl mice showed no reduction compared to day 1. C) On day 6 of place navigation training, Tg-ctrl mice took significantly longer to reach the platform than WT-ctrl mice, while Tg-TRF were quicker than Tg-ctrl mice. D) No significant differences in swim speed were observed among groups. E, F) A single probe test was conducted one day after the last place navigation training trial. Tg-ctrl mice spent less significantly time in the target quadrant and made fewer annulus crossings than WT-ctrl mice. G) On day 8, the cued navigation test (visible platform test) was conducted. Mice failing to reach a criterion of 20-s latency for the last three trials were considered impaired and removed from the MWM task analysis. No significant differences were detected among groups. For B, D, and G, data represent mean±S.E.M. [Tukey post hoc test after 2-way analysis of variance (WT-ctrl, n = 12; Tg-ctrl, n = 8; Tg-TRF; n = 9; Tg-PO, n = 9)]. *p < 0.05, **p < 0.01, ***p < 0.001, ****p < 0.0001. For C, E, and F, data represent mean±S.E.M. [Bonferroni post hoc test after analysis of variance (WT-ctrl, n = 12; Tg-ctrl, n = 8; Tg-TRF; n = 9; Tg-PO, n = 9)]. *p < 0.05, **p < 0.01.](https://content.iospress.com:443/media/jad/2018/64-1/jad-64-1-jad170880/jad-64-jad170880-g003.jpg)
On day 7, a probe trial was conducted in which the platform was removed from the pool to measure spatial memory retention. Tg-ctrl mice spent significantly less time in the escape platform (target) quadrant and made fewer annulus crossing of the former platform location than WT-ctrl mice (p < 0.05), consistent with a spatial memory deficit (Fig. 3E, F), while mice given TRF or PO showed increased time spent in the target quadrant and number of platform crossing.
The cued navigation test (visible platform test) was performed following the probe trial to determine if genetic manipulation or drug injection altered visual acuity, thereby influencing spatial learning. Escape latencies in all groups decreased progressively with trial number and all mice achieved an escape latency of less than 20 s on the last three trials (Fig. 3G), indicating comparable visual capabilities.
TRF improves recognition memory in AβPP/PS1 mice
Novel object recognition memory was tested according to the schematic diagram shown in Fig. 4A. All mice spent approximately equal time exploring the identical objects during the training trial, indicating no location bias (Fig. 4B). Following the training trial on day 4, one of the familiar objects was replaced by a novel object and the time spent exploring the novel object was measured as a recognition index (Fig. 4C). The Tg-ctrl mice spent markedly less time exploring the novel object than WT-ctrl and Tg-TRF mice, both of which exhibited a strong preference for the novel object (84% and 87% recognition indices, respectively). Moreover, the Tg-TRF mice exhibited greater exploratory activity to the novel object compared to PO-treated mice. This finding reveals that TRF ameliorates recognition memory deficit in AβPP/PS1 mice.
Fig. 4.
Administration with tocotrienol-rich fraction (TRF) prevents working memory deficit in AβPP/PS1 mice as measured by the novel object recognition task. A) Schematic representation of the novel object recognition test. Following pre-habituation and habituation in the empty arena, mice were allowed to explore an identical pair of objects placed in the arena for 10 min as the training session. After a 1 h stay in the home cage, the mice were returned to the arena where two objects, one familiar and one novel, were placed in the same locations as in the training session. The time spent exploring the two objects was recorded. B) All groups spent approximately equal time exploring the two identical objects during the training trial, indicating no inherent place preference. C) Tg-ctrl mice spent significantly less time exploring the novel object compared to WT-ctrl mice as indicated by the lower mean recognition index, while TRF treatment of Tg mice (Tg-TRF group) increased the recognition index to the WT-ctrl level. Data represent mean±S.E.M. [Bonferroni post hoc test after analysis of variance (WT-ctrl, n = 12; Tg-ctrl, n = 8; Tg-TRF; n = 9; Tg-PO, n = 9)]. *p < 0.05, **p < 0.01, ***p < 0.001.
![Administration with tocotrienol-rich fraction (TRF) prevents working memory deficit in AβPP/PS1 mice as measured by the novel object recognition task. A) Schematic representation of the novel object recognition test. Following pre-habituation and habituation in the empty arena, mice were allowed to explore an identical pair of objects placed in the arena for 10 min as the training session. After a 1 h stay in the home cage, the mice were returned to the arena where two objects, one familiar and one novel, were placed in the same locations as in the training session. The time spent exploring the two objects was recorded. B) All groups spent approximately equal time exploring the two identical objects during the training trial, indicating no inherent place preference. C) Tg-ctrl mice spent significantly less time exploring the novel object compared to WT-ctrl mice as indicated by the lower mean recognition index, while TRF treatment of Tg mice (Tg-TRF group) increased the recognition index to the WT-ctrl level. Data represent mean±S.E.M. [Bonferroni post hoc test after analysis of variance (WT-ctrl, n = 12; Tg-ctrl, n = 8; Tg-TRF; n = 9; Tg-PO, n = 9)]. *p < 0.05, **p < 0.01, ***p < 0.001.](https://content.iospress.com:443/media/jad/2018/64-1/jad-64-1-jad170880/jad-64-jad170880-g004.jpg)
Multivariate analysis of metabolomics data
In total, 783 accurate masses were detected in hippocampus using the positive electrospray ionization mode (ESI (+)) and 895 using the ESI (–) mode, while 110 and 81 accurate masses were detected by ESI (+) and ESI (–) in mPFC, respectively, and 331 and 324, respectively, in striatum. Pre-processed data were subjected to multivariate analysis for sample classification. Given the complexity of raw metabolomics data [22], multivariate data analysis based on projection methods was used for interpretation. Prior to supervised multivariate analysis, the separation trends in the datasets were evaluated by unsupervised PCA. This discriminated the groups and yielded good clustering of samples in score plots without significant outliers according to the Hotelling T2- range plot. Initially, separation trends between Tg-ctrl, Tg-TRF and QC samples were examined using PCA score plots (Supplementary Figure 1). The R2X(cum) and Q2(cum) of the score plots were 0.919 and 0.720 respectively, indicating that the models were reliable. Two groups of PCA model; WT-ctrl versus Tg-ctrl (hippocampus; ESI (–), striatum; ESI (+) and ESI (–)) and Tg-ctrl versus Tg-TRF (hippocampus; ESI (+) and ESI (–)), mPFC; ESI (+), striatum; ESI (+)) (Fig. 5) were performed to further visualized the separation trends. Both models required 4 PCs to achieved R2X(cum) and Q2(cum) above 0.4.
Fig. 5.
Principal component analysis (PCA) score plots from hippocampus, mPFC, and striatum obtained by ESI (+) and ESI (–) modes. A-C) PCA score plots from WT-ctrl and Tg-ctrl mice obtained by ESI (+). D-F) PCA score plots from WT-ctrl and Tg-ctrl mice obtained by ESI (–). G-I) PCA score plots from Tg-ctrl and Tg-TRF mice obtained by ESI (+). J-L) PCA score plots from Tg-ctrl and Tg-TRF mice obtained by ESI (–).
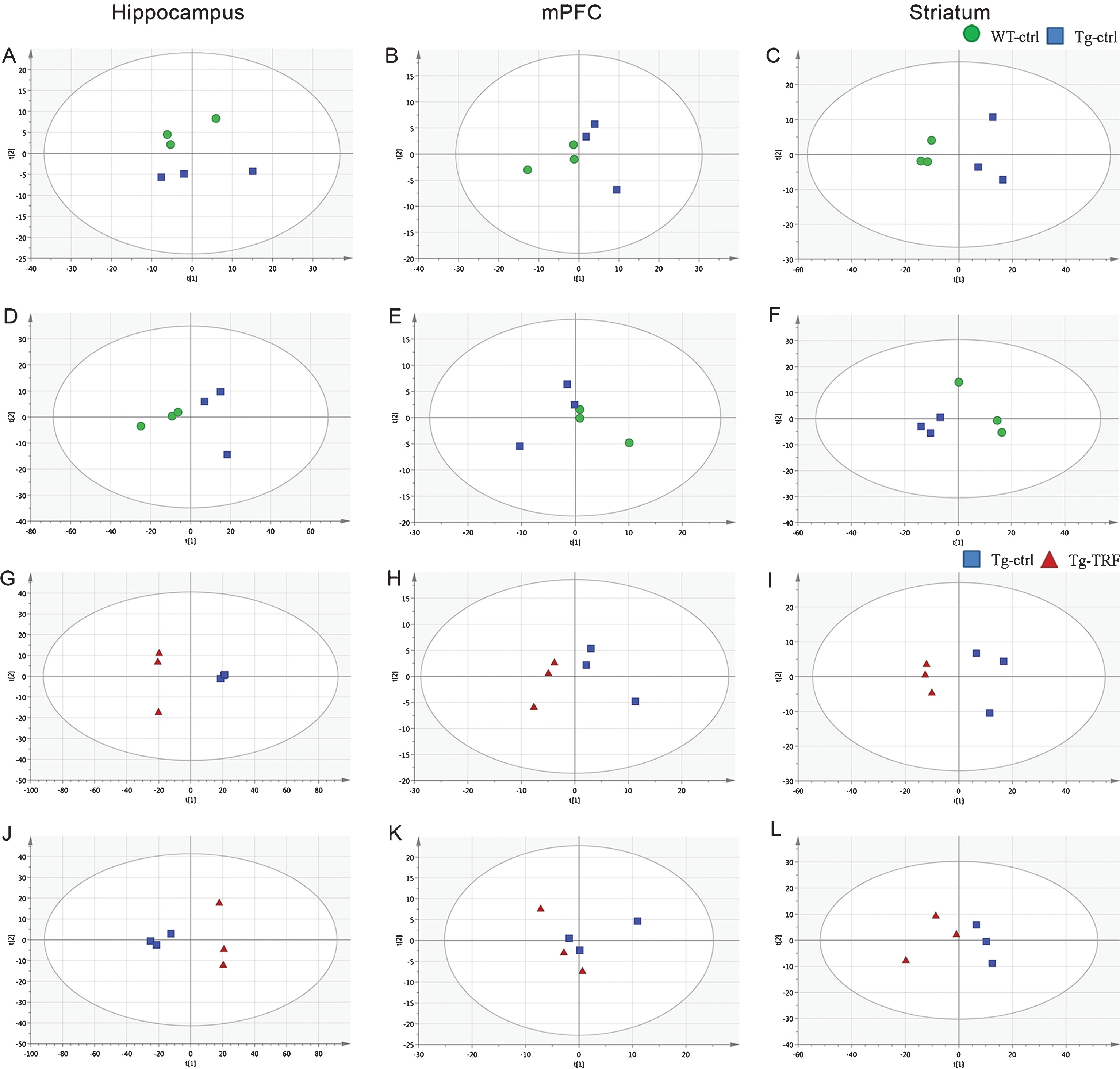
Subsequently, orthogonal partial least squares-discriminant analysis (OPLS-DA) was carried out to further enhance separation trends and isolate the features responsible for differences among the samples. OPLS-DA models representing WT-ctrl, Tg-ctrl, and Tg-TRF of hippocampus, mPFC and striatum datasets were performed as in Supplementary Figure 2. Table 1 shows the statistical parameters of R2Y(cum) and Q2(cum) to validate the OPLS-DA models. Despite the insignificance analysis of variance (ANOVA) of the cross validated residuals (CV-ANOVA) at a level of p < 0.05, the first principal component of OPLS-DA score plot revealed R2Y(cum) and Q2(cum) exceeding 0.4, indicating the robustness of model. Supplementary Figure 3 shows statistical validation of the OPLS-DA model by permutation analysis using 500 different model permutations. Model with p-CV-ANOVA value <0.05 indicates R2Y(cum) and Q2(cum) on the far right and remain higher than those of the 500 permuted models to the left. After OPLS-DA modeling, features with VIP values higher than 1.0 (VIP≥1) and significant analysis by t-test (FDR<0.05) were selected for identification. The potential features that matched these criteria are listed in Table 2. Namely Table 2 comprised putative identifications, ionization type, mass to charge ratio (m/z value), retention time (rt), fold change (Tg-ctrl versus WT-ctrl and Tg-TRF versus Tg-ctrl) and identifier. MS/MS fragmentation of the potential features can be found in Supplementary Table 1. In this study, we focus the analysis in the main three groups of mice which are WT-ctrl, Tg-ctrl, and Tg-TRF. Analysis of Tg-PO mice is shown in Supplementary Figure 4.
Table 1
Statistical parameters of PCA and OPLS-DA models for hippocampus, medial pre-frontal cortex (mPFC), and striatum
Region Model | Hippocampus | mPFC | Striatum | |||||
WT-ctrl: Tg-ctrl | Tg-ctrl: Tg-TRF | WT-ctrl: Tg-ctrl | Tg-ctrl: Tg-TRF | WT-ctrl: Tg-ctrl | Tg-ctrl: Tg-TRF | |||
PCA | ESI (+) | |||||||
A | 2 | 4 | 2 | 4 | 4 | 4 | ||
R2X(cum) | 0.724 | 0.989 | 0.706 | 0.976 | 0.970 | 0.962 | ||
Q2 (cum) | 0.235 | 0.876 | 0.091 | 0.811 | 0.708 | 0.719 | ||
ESI (–) | ||||||||
A | 4 | 4 | 3 | 3 | 4 | 3 | ||
R2X(cum) | 0.985 | 0.972 | 0.873 | 0.952 | 0.945 | 0.733 | ||
Q2 (cum) | 0.879 | 0.797 | 0.205 | 0.312 | 0.538 | 0.338 | ||
OPLS-DA | ESI (+) | |||||||
A | 1+3 | 1+1 | 1+3 | 1+3 | 1+3 | 1+2 | ||
R2X(cum) | 0.918 | 0.802 | 0.898 | 0.906 | 0.969 | 0.859 | ||
R2Y(cum) | 1.000 | 1.000 | 1.000 | 1.000 | 1.000 | 1.000 | ||
Q2 (cum) | 0.994 | 0.997 | 0.950 | 0.992 | 0.999 | 0.985 | ||
p (CV-ANOVA) | 0.05 | 0.004 | 0.05 | 0.05 | 0.05 | 0.185 | ||
ESI (–) | ||||||||
A | 1+3 | 1+1 | 1+3 | 1+3 | 1+3 | 1+3 | ||
R2X(cum) | 0.978 | 0.787 | 0.934 | 0.951 | 0.932 | 0.937 | ||
R2Y(cum) | 0.998 | 0.996 | 1.000 | 1.000 | 1.000 | 1.000 | ||
Q2 (cum) | 0.969 | 0.942 | 0.931 | 0.962 | 0.999 | 0.984 | ||
p (CV-ANOVA) | 0.05 | 0.086 | 0.05 | 0.05 | 0.05 | 0.05 |
[i] A: component (first component for PCA and first component plus orthogonal component for OPLS-DA); R2X(cum): total amount of the variation of the X variables explained by the model; R2Y(cum): total amount of the variation of the Y variables explained by the model; Q2 (cum): total amount of the predictability of the model.
Table 2
Putatively identified metabolites for discrimination between Tg-ctrl and WT-ctrl and between Tg-TRF and Tg-ctrl
Putative ID | ESI | Formula | m/z | rt | Fold change | Identifier | |
Tg-ctrl versus WT-ctrl | Tg-TRF versus Tg-ctrl | ||||||
Hippocampus | |||||||
N-Aspartic acid | + | C4 H7 N O4 | 134.0444 | 1.020 | ↓ | ↑ | 462 (mzCloud) |
N-Acetylaspartic acid | – | C6 H9 N O5 | 174.03961 | 0.964 | ns | ↑ | 1376 (mzCloud) |
L-Tyrosine | – | C9 H11 N O3 | 180.06551 | 1.440 | ↓ | ↑ | 2255 (mzCloud) |
L-Glutamic acid | – | C5 H9 N O4 | 146.0448 | 0.510 | ↓ | ↑ | 470 (mzCloud) |
DL-Glutamine | + | C5 H10 N2 O3 | 147.0759 | 0.497 | ↓ | ns | 2967 (mzCloud) |
L-Phenylalanine | + | C9 H11 N O2 | 166.08585 | 2.255 | ↓ | ns | 8 (mzCloud) |
L-Histidine | – | C6 H9 N3 O2 | 154.06114 | 0.466 | ns | ↑ | 473 (mzCloud) |
Pyroglutamate | + | C5 H7 N O3 | 130.0495 | 0.498 | ↓ | ns | 1831 (mzCloud) |
Creatinine | + | C4 H7 N3 O | 114.06602 | 0.516 | ns | ↑ | 375 (mzCloud) |
AMP | + | C10 H14 N5 O7 P | 348.0689 | 1.068 | ↓ | ↑ | 252 (mzCloud) |
IMP | – | C10 H13 N4 O8 P | 347.03943 | 1.318 | ns | ↑ | 452 (mzCloud) |
UMP | – | C9 H13 N2 O9 P | 323.02802 | 0.952 | ns | ↑ | 1405 (mzCloud) |
Adenosine | + | C10 H13 N5 O4 | 268.10309 | 1.952 | ↑ | ns | 297 (mzCloud) |
Adenine | + | C5 H5 N5 | 136.0614 | 0.781 | ↓ | ↑ | 296 (mzCloud) |
Inosine | + | C10 H12 N4 O5 | 269.08713 | 2.025 | ↑ | ns | 1234 (mzCloud) |
Guanine | + | C5 H5 N5 O | 152.05634 | 1.244 | ns | ↓ | 436 (mzCloud) |
Uridine | – | C9 H12 N2 O6 | 243.06168 | 1.641 | ns | ↑ | 1408 (mzCloud) |
Desmosterol | + | C27 H44 O | 385.34525 | 10.026 | ↓ | ns | HMDB02719 |
Glycerophosphocholine | + | C8 H20 N O6 P | 258.10947 | 0.566 | ↑ | ns | HMDB00049 |
Glycerylphosphorylethanolamine | + | C5 H14 N O6 P | 216.06259 | 0.517 | ↑ | ns | HMDB00114 |
Ceramide (d18:0/18:1(11Z)) | + | C36 H71 NO3 | 566.54926 | 9.672 | ns | ↓ | HMDB11762 |
Galabiosylceramide (d18:1/20:0) | + | C50 H95 N O13 | 918.68713 | 13.334 | ns | ↓ | HMDB04835 |
PS(22:1(11Z)/0:0) | + | C28 H54 N O9 P | 580.36005 | 6.799 | ↑ | ↓ | LMGP03050023 |
LysoPE(16:0) | + | C21 H44 N O7 P | 454.29132 | 6.938 | ns | ↓ | LMGP02050036 |
LysoPE(22:4) | + | C27 H48 N O7 P | 530.32361 | 7.131 | ns | ↓ | HMDB11493 |
GDP-glucose | – | C16 H25 N5 O16 P2 | 604.06952 | 1.011 | ↓ | ↑ | HMDB03351 |
Sedoheptulose 7-phosphate | – | C7H15O10P | 289.03262 | 0.562 | ↓ | ↑ | C05382 (KEGG) |
DL-Malic acid | – | C4 H6 O5 | 133.01306 | 0.711 | ↓ | ↑ | 1796 (mzCloud) |
Choline | + | C5 H13 N O | 104.10697 | 0.520 | ↑ | ns | 886 (mzCloud) |
Acetylcholine | + | C7 H15 N O2 | 146.11714 | 0.611 | ↓ | ↑ | 885 (mzCloud) |
Phosphocholine | + | C5 H14 N O4 P | 184.07281 | 0.541 | ↑ | ns | C00588 (KEGG) |
S-Adenosylmethionine | + | C15 H22 N6 O5 S | 399.14316 | 0.485 | ns | ↑ | 896 (mzCloud) |
Spermidine | + | C7 H19 N3 | 146.16478 | 0.365 | ↑ | ns | 9 (mzCloud) |
γ-Aminobutyric acid | + | C4 H9 N O2 | 104.07059 | 0.475 | ns | ↑ | 796 (mzCloud) |
Uric acid | – | C5 H4 N4 O3 | 167.02002 | 1.163 | ↓ | ↑ | 753 (mzCloud) |
Carnosine | – | C9 H14 N4 O3 | 225.09854 | 0.444 | ns | ↑ | C00386 (KEGG) |
Nicotinamide | + | C6 H6 N2 O | 123.05505 | 1.162 | ns | ↑ | 517 (mzCloud) |
mPFC | |||||||
Ceramide (d18:0/14:0) | + | C32 H65 N O3 | 512.50287 | 7.105 | ↑ | ns | 53968 (Metlin) |
Phosphatidylinositol | + | C45 H87 O12 P | 851.6037 | 8.417 | ↑ | ↓ | Several |
Phosphocholine | + | C11 H24 N O7 P | 315.14301 | 4.673 | ↑ | ↓ | Several |
Striatum | |||||||
γ-Glutamylcysteine | + | C8 H14 N2 O5 S | 251.06882 | 0.949 | ↑ | ns | 427 (mzCloud) |
γ-L-Glutamyl-L-glutamic acid | + | C10 H16 N2 O7 | 277.1022 | 0.682 | ↓ | ns | 1223 (mzCloud) |
PS(18:1(9Z)/0:0) | + | C24 H46 N O9 P | 524.29797 | 6.619 | ↓ | ns | 40829 (Metlin) |
LysoPC(18:1(11Z)) | + | C26 H52 N O7 P | 522.35492 | 6.755 | ↑ | ns | HMDB10385 |
LysoPE(20:1(11Z)/0:0) | + | C25 H50 N O7 P | 508.3389 | 7.162 | ns | ↑ | HMDB11513 |
LysoPE(0:0/20:4(5Z,8Z,11Z,14Z)) | – | C25 H44 N O7 P | 500.27817 | 13.450 | ns | ↓ | HMDB11487 |
Sphingosine | + | C18 H37 N O2 | 300.28873 | 6.084 | ↑ | ↓ | HMDB00252 |
ADP ribose | – | C15 H23 N5 O14 P2 | 558.0636 | 0.847 | ↑ | ↓ | 1708 (mzCloud) |
β-D-Fructose 1,6-bisphosphate | – | C6 H14 O12 P2 | 338.98853 | 0.524 | ↑ | ns | C05378 (KEGG) |
Isocitrate | + | C6 H8 O7 | 193.03407 | 1.126 | ↑ | ↓ | HMDB00193 |
cis-Aconitate | – | C6 H6 O6 | 173.0083 | 1.577 | ↑ | ↓ | C00417 (KEGG) |
Adenylosuccinate | + | C14 H18 N5 O11 P | 464.08066 | 2.166 | ↑ | ↓ | HMDB00536 |
Acetyl-L-carnitine | + | C9 H17 N O4 | 204.12244 | 0.799 | ↓ | ns | 879 (mzCloud) |
6β-Prostaglandin I1 | – | C20 H34 O5 | 353.2333 | 6.225 | ↑ | ↓ | 4594 (mzCloud) |
D-4’-Phosphopantothenate | – | C9 H18 N O8 P | 298.06964 | 0.873 | ns | ↓ | C03492 (KEGG) |
[i] ↑, upregulated; ↓, downregulated; AMP, adenosine monophosphate; GDP, guanosine diphosphate; PS, phosphatidylserine; ADP, adenosine diphosphate; PS, phosphatidylserine; LysoPC, lysophosphatidylcholine; UMP, uridine monophosphate; IMP, inosine-5’-monophosphate; LysoPE, lysophosphatidylethanolamine.
Features changes with TRF treatment
Of the total discriminated features, 39 and 38 were selected as potential markers to differentiate between Tg-ctrl and WT-ctrl mice and between Tg-ctrl and Tg-TRF mice, respectively, regardless of the brain regions. Among those features, 19 were detected as altered between both comparisons. Of these, 10 were upregulated and nine were downregulated by the TRF treatment. The majority of metabolomic alterations were found in the hippocampus region, indicating that this is the most affected region in AβPP/PS1 mice. The largest changes were observed in nucleotides, amino acids, and lipids. Nucleotides (adenine, AMP, ADP ribose and adenylosuccinate), features involve in bioenergetics (GDP-glucose, sedoheptulose, DL-malic acid, isocitrate and cis-aconitate), neurotransmission (L-aspartic acid, L-tyrosine, L-glutamic acid and acetylcholine), membrane lipid metabolism (phosphatidylinositol, phosphatidylserine, phosphocholine, and sphingosine) and oxidative stress defense (uric acid and prostaglandin) were found to be altered in Tg-ctrl mice. The alterations were then ameliorated by the TRF treatment (Table 2). There were also 18 putatively identified metabolite (11 upregulated and 7 downregulated) that significantly changed in Tg-ctrl (versus WT-ctrl) but not in Tg-TRF, while 19 putatively identified metabolite (12 upregulated and 7 downregulated) significantly changed in Tg-TRF mice (versus Tg-ctrl) only (Table 2). Almost all altered pathways in Tg-ctrl and Tg-TRF mice were present in the hippocampus, while only two metabolic pathways in the striatal region showed a significant impact-value more than 0.1 and none of the metabolic pathways in mPFC region were above 0.1 (data not shown). The three most altered pathways in Tg-ctrl were phenylalanine, tyrosine and tryptophan biosynthesis, D-glutamine and D-glutamate metabolism, and alanine, aspartate and glutamate metabolism (Fig. 6A). TRF was shown to modulate these pathways with the greatest impact on D-glutamine and D-glutamate metabolism (Fig. 6B).
Fig. 6.
Metabolomics pathway analysis. A) Pathway analysis in the hippocampus region from Tg-ctrl mice. B) Pathway analysis in the hippocampus region from Tg-TRF mice. C) Altered metabolic pathways in the brain of Tg-ctrl and Tg-TRF mice. (a) Phenylalanine, tyrosine and tryptophan biosynthesis; (b) D-Glutamine and D-glutamate metabolism; (c) Alanine, aspartate and glutamate metabolism; (d) Phenylalanine metabolism; (e) Glycerophospholipid metabolism; (f) Glyoxylate and dicarboxylate metabolism; (g) Histidine metabolism; (h) Purine metabolism; (i) Arginine and proline metabolism; (j) Tyrosine metabolism; (k) Glutathione metabolism; (l) Citrate cycle (TCA cycle); (m) Pyrimidine metabolism; (n) Pentose phosphate pathway; (o) Cysteine and methionine metabolism; (p) Vitamin B6 metabolism; (q) Nicotinate and nicotinamide metabolism. Each node represents an altered metabolic pathway in the hippocampus of both groups. The color and size of each node is based on p-value and pathway impact-value, respectively. () increased in Tg-ctrl mice; (
) decreased in Tg-ctrl mice; (
) increased in Tg-TRF mice; (
) decreased in Tg-TRF mice. Simplified biochemical pathways were schematized according to the KEGG database. Solid arrows represent direct metabolic reactions, and dashed arrows represent multiple reactions and indirect connections between two features.
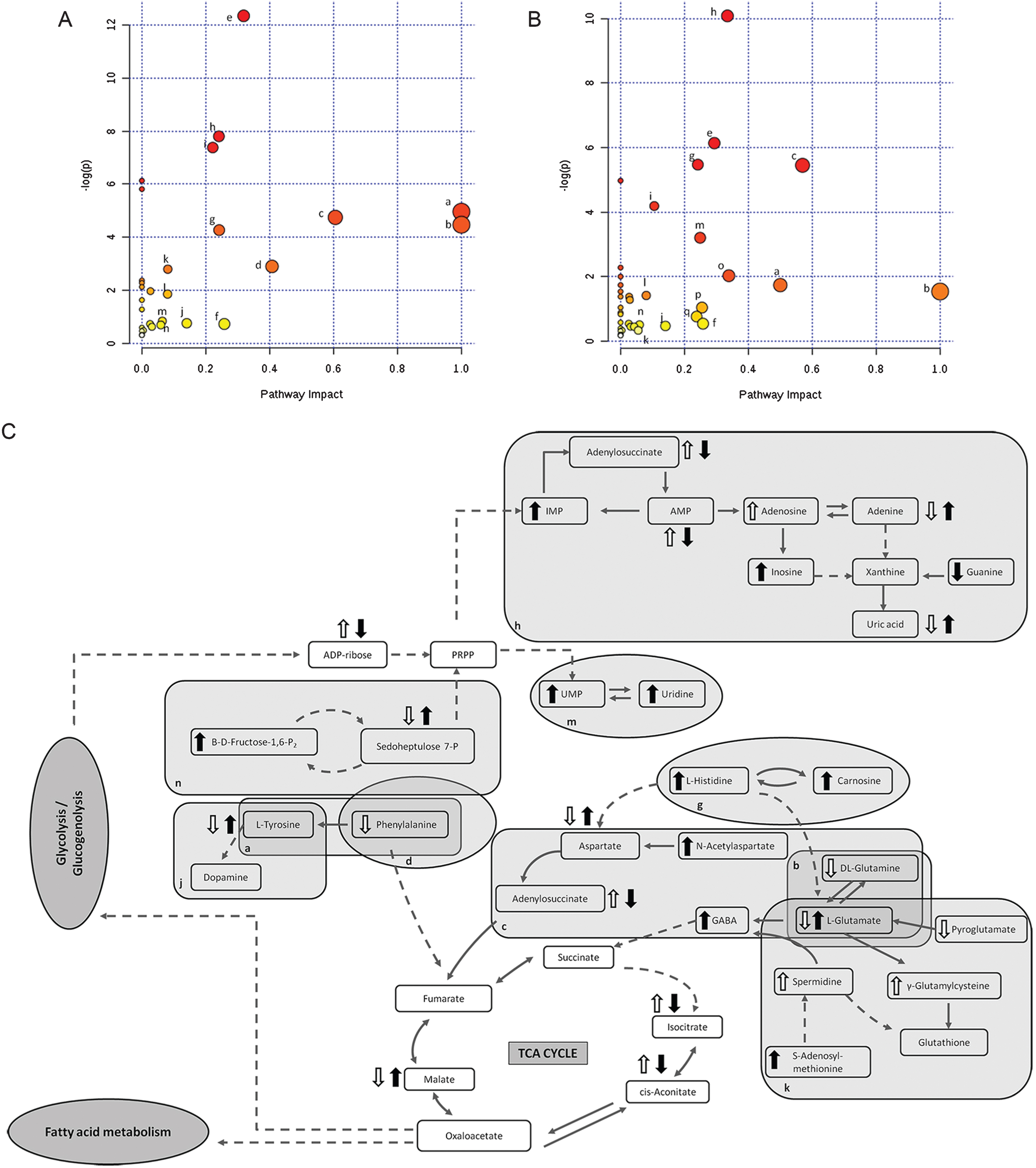
DISCUSSION
Tocotrienol has garnered wide attention as a potential therapeutic agent against neurodegenerative disease due to its potent antioxidant and neuroprotective properties. In this present study, for the first time, we evaluated the effect of TRF on behavior and cognitive functions in (15 months old) AβPP/PS1 mice and investigated the associated metabolomic changes in different subregions of the brain. We found that certain behavioral and cognitive impairments of AβPP/PS1 mice were alleviated by long-term TRF treatment. We also conducted a pilot untargeted metabolomic study examining the metabolic changes in three brain regions of untreated and TRF-treated AβPP/PS1 mice. The hippocampus was the most affected region in these mice according to metabolomics, and TRF treatment was shown to modulate several metabolic pathways linked to AD.
Behavioral examinations
Long-term administration of TRF for 10 months improved the exploratory behavior of AβPP/PS1 mice as evidenced by longer total rearing time, while comparable total distances travel and proportional times in the open field center indicated no locomotor impairments or elevated anxiety in AβPP/PS1 mice, consistent with a previous comprehensive behavioral study [37]. The present study also assessed spatial learning and memory in the Morris water maze task by training mice to find a hidden platform in a fixed location (place navigation). Tg-ctrl mice exhibited impaired place navigation learning and spatial memory as revealed by the slower acquisition of platform location during training and less time spent in the target quadrant during the probe trial. This performance impairment was not due to motor or sensory deficits as there were no significant differences in swimming speed and latency to reach the visible platform among groups. We previously reported that AβPP/PS1 mice at 15 months of age exhibit impairments in relatively long-term spatial memory [38]. Treatment with TRF improved spatial learning and memory, as evidenced by shorter escape latency. However, TRF did not improve 24-h memory retention as measured by a probe trial. Our results are in partial agreement with prior studies demonstrating positive effects of dietary TRF supplementation on spatial learning [39–41].
Working memory is a system for temporarily storing, processing and manipulating the information required to perform complex cognitive tasks [42]. Impaired working memory has been demonstrated even at the earliest stage of AD [43] due to focal neuronal damage that gradually spreads throughout the brain. Thus, we examined the short-term recognition memory of AβPP/PS1 using a spontaneous and non-forced test of novelty [44, 45]. Tg-ctrl mice showed a significant reduction in novel object exploration time compared to age-matched WT-ctrl mice, suggesting impaired working memory, in accord with earlier reports of age-related memory impairment in AβPP/PS1 mice [46–48]. TRF treatment for 10 months ameliorated this memory deficit in AβPP/PS1 mice, while PO vehicle had no effect.
Metabolomics analysis
Nineteen putatively identified metabolites involved in several metabolic pathways related to AD were altered by TRF, suggesting that TRF exerts protective effects against AD-associated metabolic disruption. Numerous studies have provided evidence for mitochondrial dysfunction in AD, including abnormalities in the TCA cycle and energy production [20, 49, 50]. Marked abnormalities in brain energy metabolism were observed in Tg-ctrl hippocampus (Table 2). The decrease in glucose may reduce amino sugar and nucleotide sugar metabolism, while alterations in some component of TCA cycle could impair energy production, in accord with previous studies [22, 51]. Also, altered TCA enzyme activities have been reported in the AD brain [50], including lower activities of isocitrate dehydrogenase and malate dehydrogenase, consistent with our findings of changes in isocitrate and malic acid. In addition, we found a significant reduction in the levels of high-energy biomolecules in Tg-ctrl mice relative to WT-ctrl mice. Reduced AMP may contribute to cellular energy dyshomeostasis and decreased AMP-kinase activity, a critical regulator of cellular energy charge as well as glucose and lipid homeostasis [52]. AMP-kinase activity decreases in AD brain, indicating reduced mitochondrial integrity, which can subsequently lead to enhanced Aβ generation through dysregulation of AβPP processing [53]. These brain metabolic changes were rescued partially by TRF treatment. A recent study suggested that dietary tocotrienol protects neurons in the brains of aged mice by increasing protein expression of the mitochondrial transcription factor A and by maintaining mitochondrial membrane potential and ATP synthesis [16]. An in vitro study demonstrated that tocotrienol was able to maintain oxidative phosphorylation and ATP levels too [54]. Apart from modulating these features, TRF increased the levels of UMP, IMP, GMP, and ADP-ribose, all of which have been reported to be lower in the AD brain [35] and in the serum of cognitive decline mice [55]. Therefore, our findings suggest that TRF alleviates the cognitive dysfunction in AD at least in part by rescuing brain energy metabolism.
Adenosine, a purine ribonucleoside, also serves as a neuromodulator with neuroprotective effects in the central nervous system [56]. It regulates neurotransmitter release such as glutamate and acetylcholine [57–59]. Prior studies detected altered purine metabolism in the cerebrospinal fluid and brain of AD patients [60–62]. Here, we found that purine metabolism was disturbed in Tg-ctrl, while TRF treatment modulated the changes by increasing some of the features involved in this pathway. These effects may be related to the antioxidant activity of TRF, specifically to a reduction in superoxide generated by the non-mitochondrial ROS-generating enzyme xanthine oxidase [63].
Metabolomic analysis also indicated disruption of neurotransmitter synthesis and dopaminergic transmission pathways in Tg-ctrl mice as observed by deficits in acetylcholine and amino acids involved in neurotransmitter production as well as by increased spermidine (polyamine). Cholinergic abnormalities [64] and deficits in excitatory neurotransmitter [35, 65] are believed to underlie the progressive loss of cognitive function in AD. Meanwhile, increased polyamine synthesis could be one of the downstream effects of Aβ [66]. Overall, TRF improved neurotransmitter metabolism in the AβPP/PS1 mice as evidenced by increasing levels of acetylcholine, L-tyrosine, L-glutamic acid, and L-aspartic acid (Table 2). To our knowledge, few studies have examined the effect of vitamin E (tocopherol and tocotrienol) on neurotransmitter levels. Dietary vitamin E supplementation has been shown to protect the brain against oxidative stress, thus sustaining cholinergic function after injection of scopolamine, an inducer of dementia [67]. More recently, a study of patients with mild to moderate AD found that α-tocopherol was more effective in delaying cognitive dysfunction than the glutamatergic system drug memantine [68]. Taking all these facts into account, several amino acids and nucleotides are perturbed in AD, and TRF seems partly to reduce the abnormalities, as schematized in Fig. 6C.
Normal brain function is particularly susceptible to the deleterious effects of oxidative stress due to the high contents (and functional relevance) of polyunsaturated fatty acids, the high metabolic rate, and relatively low levels of antioxidants [69]. Decreased pyroglutamate and uric acid in Tg-ctrl mice may reflect glutathione insufficiency [70] and a failure in scavenging of peroxynitrite in neurons [71]. Moreover, increased prostaglandin which is a product of the arachidonic acid cascade, possibly reflecting damaging pro-oxidant cascades [72, 73]. Thus, oxidative stress is strongly implicated in AD, as has been reported previously [74–76], in contrast, treatment with the antioxidant TRF reduces the stress. Several studies have also asserted that markers of oxidative stress in AD, including products of lipid peroxidation, and decreased antioxidant enzyme activities are correlated with low levels of vitamin E [77], further underscoring the potential of TRF as a promising treatment to improve oxidative stress responses.
Neuronal membrane breakdown caused by over-activation of phospholipase A2, with ensuing membrane phospholipid degradation and release of free fatty acids and lysophospholipids, is also a significant pathogenic process in AD [78]. In fact, phospholipase A2 is most active toward phospholipids after peroxidation [79]. Perturbation of membrane phospholipids destabilizes ion homeostasis by changing membrane fluidity and permeability and leads to synaptic loss [80]. The rises in phosphocholine and choline in Tg-ctrl mice in this study may have resulted from the accelerated breakdown of phosphatidylcholine, a major membrane phospholipid. We also observed accumulation of sphingolipid intermediates such as ceramide and sphingosine in Tg-ctrl mice, indicating perturbed sphingomyelin metabolism. Oxidative stress and Aβ are thought to induce sphingomyelin degradation and to trigger ceramide accumulation [81, 82]. This ceramide can then promote Aβ deposition via an interaction with β-secretase [83].
Few studies have examined a direct connection between tocotrienol and cell membrane lipid metabolism in AD. Generally, the antioxidant actions of tocotrienols are attributed to scavenging chain-propagating peroxyl radicals, thereby disrupting the lipid peroxidation cycle. The antioxidant function of vitamin E is decentralized in the chromanol ring, whereas the phenolic hydroxy group donates a hydrogen atom to quench lipid radicals [84]. After administration of TRF in AβPP/PS1 mice, we observed a reduction in lysophospholipid levels which support the previous study that inhibition of the formation of lysophospholipids by tocopherol protects cell membranes [79]. Tocopherol may inhibit the oxidation of phospholipid substrates, thereby reducing their availability for phospholipase A2. The biological structure and hydrophobic character of vitamin E also play significant roles in membrane stability. In order to better understand the function of vitamin E at the molecular level, a few studies have attempted to examine its interaction with membrane components. The unsaturated structure of tocotrienols imparts greater flexibility to the side chain and it is proposed that this enhances curvature stress on phospholipid membranes [79] and allows for more efficient penetration and distribution into saturated fatty layers of brain tissue [14]. Reduction in sphingosine and ceramides in Tg-TRF mice indicates that TRF may also help in maintaining sphingolipid metabolism, as has been shown by tocopherol and combination of vitamin E in the published reports [85–87].
Taken together, as illustrated in Supplementary Figure 5 we proposed that TRF may improve neuronal function and behavioral performance by modulating-Aβ deposition and/or via modulation of metabolic pathways (dependent or independent of Aβ interaction). Although the mechanism is speculative at this moment, we found that pre-emptive activity of TRF is related to the modulation of metabolic pathways involved in bioenergetics, neurotransmission, membrane lipid metabolism and oxidative stress defense.
To our knowledge, this is the first study reporting that TRF can partially ameliorate behavioral deficits and alter brain metabolic abnormalities in AβPP/PS1 transgenic mice. Nevertheless, this study has several limitations. This study used a small sample size for the metabolomics analysis due to sample constraints. Thus, we agree that additional studies are necessary to validate these findings in a larger cohort. However, in order to compensate the small size number issue, we have used appropriate statistical analyses with an emphasis to avoid over-fitting the data and have interpreted the results with caution. As shown in the study, we have performed both parametric and non-parametric to address significance and non-significance, addressed the R2X, Q2 values for PCA and OPLS-DA, validation of OPLS-DA using permutation and CV-ANOVA, and MS/MS analysis for putative identification of potential metabolites. Another limitation is that we treated the mice from the age of 5 months which the amyloid deposits not yet occurred. Further studies will be needed to clarify whether TRF treatment in the later aged mice shows therapeutic effects or not.
In summary, Aβ accumulation and disturbances in metabolic pathways cause neuronal dysfunction and lead to behavioral impairment. Long-term TRF treatment was able to improve exploratory activity, memory and learning abilities of AβPP/PS1 AD mice, while metabolomics results suggest that TRF treatment partly ameliorates the abnormalities in the brain. Mechanism of this pre-emptive activity may occur via modulation of metabolic pathways dependent on Aβ interaction or independent of Aβ interaction. These results provide a foundation for future studies on novel etiological pathways and treatment strategies for AD. We hope that these findings will stimulate further preclinical and clinical study of TRF.
ACKNOWLEDGMENTS
The authors thank Dr. Tan Jen Kit for excellent technical assistance. This work was supported by the Japan Society for Promotion of Science (grant numbers JSPS KAKENHI 17H03560 to I.T. and 17K01355 to D.Y.) and the Ministry of Education Malaysia (grant number LRGS/BU/2012/UKM-UKM/K/04).
Authors’ disclosures available online (https://www.j-alz.com/manuscript-disclosures/17-0880r3).
SUPPLEMENTARY MATERIAL
[3] The supplementary material is available in the electronic version of this article: http://dx.doi.org/10.3233/JAD-170880.
REFERENCES
[1] | World Alzheimer Report 2016, World Alzheimer Report 2016. Improving healthcare for people living with dementia. Coverage, quality and costs now and in the future, https://www.alz.co.uk/research/WorldAlzheimerReport2016.pdf, Accessed on March 21, 2017. |
[2] | Selkoe DJ ((2003) ) Folding proteins in fatal ways. Nature 426: , 900–904. |
[3] | Povova J, Ambroz P, Bar M, Pavukova V, Sery O, Tomaskova H, Janout V ((2012) ) Epidemiological of and risk factors for Alzheimer’s disease: A review. Biomed Pap Med Fac Univ Palacky Olomouc Czech Repub 156: , 108–114. |
[4] | Bateman RJ, Xiong C, Benzinger TLS, Fagan AM, Goate A, Fox NC, Marcus DS, Cairns NJ, Xie X, Blazey TM, Holtzman DM, Santacruz A, Buckles V, Oliver A, Moulder K, Aisen PS, Ghetti B, Klunk WE, McDade E, Martins RN, Masters CL, Mayeux R, Ringman JM, Rossor MN, Schofield PR, Sperling RA, Salloway S, Morris JC ((2012) ) Clinical and biomarker changes in dominantly inherited Alzheimer’s disease. N Engl J Med 367: , 795–804. |
[5] | JackJr CR, Knopman DS, Jagust WJ, Petersen RC, Weiner MW, Aisen PS, Shaw LM, Vemuri P, Wiste HJ, Weigand SD, Lesnick TG, Pankratz VS, Donohue MC, Trojanowski JQ ((2013) ) Tracking pathophysiological processes in Alzheimer’s disease: An updated hypothetical model of dynamic biomarkers. Lancet Neurol 12: , 207–216. |
[6] | Lloret A, Giraldo E, Viña J ((2011) ) Is antioxidant therapy effective to treat Alzheimer’s disease? Free Radic Antioxid 1: , 8–14. |
[7] | Galasko DR, Peskind E, Clark CM, Quinn JF, Ringman JM, Jicha GA, Cotman C, Cottrell B, Montine TJ, Thomas RG, Aisen P ((2012) ) Antioxidants for Alzheimer disease: A randomized clinical trial with cerebrospinal fluid biomarker measures. Arch Neurol 69: , 836–841. |
[8] | Feng Y, Wang X ((2012) ) Antioxidant therapies for Alzheimer’s disease. Oxid Med Cell Longev 2012: , 472932. |
[9] | Ahmed HH ((2012) ) Modulatory effects of vitamin E, acetyl-L-carnitine and alpha-lipoic acid on new potential biomarkers for Alzheimer’s disease in rat model. Exp Toxicol Pathol 64: , 549–556. |
[10] | Zingg JM ((2007) ) Modulation of signal transduction by vitamin E. Mol Aspects Med 28: , 481–506. |
[11] | Aggarwal BB, Sundaram C, Prasad S, Kannappan R ((2010) ) Tocotrienols, the vitamin E of the 21st century: Its potential against cancer and other chronic diseases. Biochem Pharmacol 80: , 1613–1631. |
[12] | Sen CK, Khanna S, Roy S ((2006) ) Tocotrienols: Vitamin E beyond tocopherols. Life Sci 78: , 2088–2098. |
[13] | Maarasyid C, Muhamad I, Supriyantob E ((2014) ) Potential source and extraction of vitamin E from palm-based oils: A review. J Teknologi 69: , 43–50. |
[14] | Suzuki YJ, Tsuchiya M, Wassall SR, Choo YM, Govil G, Kagan VE, Packer L ((1993) ) Structural and dynamic membrane properties of alpha-tocopherol and alpha-tocotrienol: Implication to the molecular mechanism of their antioxidant potency. Biochemistry 32: , 10692–10699. |
[15] | Aan GJ, Adi MM ((2017) ) Tocotrienol rich fraction modulates the expression of synaptogenic RNAs in neurons of Caenorhabditis elegans. Res Updates Med Sci 5: , 4–11. |
[16] | Schloesser A, Esatbeyoglu T, Piegholdt S, Dose J, Ikuta N, Okamoto H, Ishida Y, Terao K, Matsugo S, Rimbach G ((2015) ) Dietary tocotrienol/γ-cyclodextrin complex increases mitochondrial membrane potential and ATP concentrations in the brains of aged mice. Oxid Med Cell Longev 2015: , 789710. |
[17] | Budin SB, Othman F, Louis SR, Bakar MA, Das S, Mohamed J ((2009) ) The effects of palm oil tocotrienol-rich fraction supplementation on biochemical parameters, oxidative stress and the vascular wall of streptozotocin-induced diabetic rats. Clinics (Sao Paulo) 64: , 235–244. |
[18] | Mangialasche F, Kivipelto M, Mecocci P, Rizzuto D, Palmer K, Winblad B, Fratiglioni L ((2010) ) High plasma levels of vitamin E forms and reduced Alzheimer’s disease risk in advanced age. J Alzheimers Dis 20: , 1029–1037. |
[19] | Ibrahim NF, Yanagisawa D, Durani LW, Hamezah HS, Damanhuri HA, Wan Ngah WZ, Tsuji M, Kiuchi Y, Ono K, Tooyama I ((2017) ) Tocotrienol-rich fraction modulates amyloid pathology and improves cognitive function in AbetaPP/PS1 mice. J Alzheimers Dis 55: , 597–612. |
[20] | Trushina E, Dutta T, Persson X-MT, Mielke MM, Petersen RC ((2013) ) Identification of altered metabolic pathways in plasma and CSF in mild cognitive impairment and Alzheimer’s disease using metabolomics. PLoS One 8: , e63644. |
[21] | Graham SF, Chevallier OP, Roberts D, Holscher C, Elliott CT, Green BD ((2013) ) Investigation of the human brain metabolome to identify potential markers for early diagnosis and therapeutic targets of Alzheimer’s disease. Anal Chem 85: , 1803–1811. |
[22] | Gonzalez-Dominguez R, García-Barrera T, Vitorica J, Gómez-Ariza JL ((2015) ) Deciphering metabolic abnormalities associated with Alzheimer’s disease in the APP/PS1 mouse model using integrated metabolomic approaches. Biochimie 110: , 119–128. |
[23] | Pan X, Nasaruddin MB, Elliott CT, McGuinness B, Passmore AP, Kehoe PG, Holscher C, McClean PL, Graham SF, Green BD ((2016) ) Alzheimer’s disease-like pathology has transient effects on the brain and blood metabolome. Neurobiol Aging 38: , 151–163. |
[24] | Morris RG, Garrud P, Rawlins JN, O’Keefe J ((1982) ) Place navigation impaired in rats with hippocampal lesions. Nature 297: , 681–683. |
[25] | van den Berg RA, Hoefsloot HC, Westerhuis JA, Smilde AK, van der Werf MJ ((2006) ) Centering, scaling, and transformations: Improving the biological information content of metabolomics data. BMC Genomics 7: , 142. |
[26] | Xia J, Wishart DS ((2016) ) Using MetaboAnalyst 3.0 for comprehensive metabolomics data analysis. Curr Protoc Bioinformatics 55: , 14.10.11-14.10.91. |
[27] | Smith CA, O’Maille G, Want EJ, Qin C, Trauger SA, Brandon TR, Custodio DE, Abagyan R, Siuzdak G ((2005) ) METLIN: A metabolite mass spectral database. Ther Drug Monit 27: , 747–751. |
[28] | Wishart DS, Jewison T, Guo AC, Wilson M, Knox C, Liu Y, Djoumbou Y, Mandal R, Aziat F, Dong E, Bouatra S, Sinelnikov I, Arndt D, Xia J, Liu P, Yallou F, Bjorndahl T, Perez-Pineiro R, Eisner R, Allen F, Neveu V, Greiner R, Scalbert A ((2013) ) HMDB 3.0–The Human Metabolome Database in 2013. Nucleic Acids Res 41: , D801–807. |
[29] | Wishart DS, Knox C, Guo AC, Eisner R, Young N, Gautam B, Hau DD, Psychogios N, Dong E, Bouatra S, Mandal R, Sinelnikov I, Xia J, Jia L, Cruz JA, Lim E, Sobsey CA, Shrivastava S, Huang P, Liu P, Fang L, Peng J, Fradette R, Cheng D, Tzur D, Clements M, Lewis A, De Souza A, Zuniga A, Dawe M, Xiong Y, Clive D, Greiner R, Nazyrova A, Shaykhutdinov R, Li L, Vogel HJ, Forsythe I ((2009) ) HMDB: A knowledgebase for the human metabolome. Nucleic Acids Res 37: , D603–610. |
[30] | Wishart DS, Tzur D, Knox C, Eisner R, Guo AC, Young N, Cheng D, Jewell K, Arndt D, Sawhney S, Fung C, Nikolai L, Lewis M, Coutouly M-A, Forsythe I, Tang P, Shrivastava S, Jeroncic K, Stothard P, Amegbey G, Block D, Hau DD, Wagner J, Miniaci J, Clements M, Gebremedhin M, Guo N, Zhang Y, Duggan GE, MacInnis GD, Weljie AM, Dowlatabadi R, Bamforth F, Clive D, Greiner R, Li L, Marrie T, Sykes BD, Vogel HJ, Querengesser L ((2007) ) HMDB: The Human Metabolome Database. Nucleic Acids Res 35: , D521–D526. |
[31] | Kanehisa M, Goto S ((2000) ) KEGG: Kyoto encyclopedia of genes and genomes. Nucleic Acids Res 28: , 27–30. |
[32] | Kim S, Thiessen PA, Bolton EE, Chen J, Fu G, Gindulyte A, Han L, He J, He S, Shoemaker BA, Wang J, Yu B, Zhang J, Bryant SH ((2016) ) PubChem substance and compound databases. Nucleic Acids Res 44: , D1202–1213. |
[33] | Sud M, Fahy E, Cotter D, Brown A, Dennis EA, Glass CK, MerrillJr AH, Murphy RC, Raetz CR, Russell DW, Subramaniam S ((2007) ) LMSD: LIPID MAPS structure database. Nucleic Acids Res 35: , D527–532. |
[34] | Hastings J, de Matos P, Dekker A, Ennis M, Harsha B, Kale N, Muthukrishnan V, Owen G, Turner S, Williams M, Steinbeck C ((2013) ) The ChEBI reference database and ontology for biologically relevant chemistry: Enhancements for 2013. Nucleic Acids Res 41: , D456–D463. |
[35] | Gonzalez-Dominguez R, Garcia-Barrera T, Vitorica J, Gomez-Ariza JL ((2015) ) Metabolomic screening of regional brain alterations in the APP/PS1 transgenic model of Alzheimer’s disease by direct infusion mass spectrometry. J Pharm Biomed Anal 102: , 425–435. |
[36] | Xia J, Wishart DS ((2011) ) Metabolomic data processing, analysis, and interpretation using MetaboAnalyst. Curr Protoc Bioinformatics Chapter 14, Unit 14.10. |
[37] | Webster SJ, Bachstetter AD, Van Eldik LJ ((2013) ) Comprehensive behavioral characterization of an APP/PS-1 double knock-in mouse model of Alzheimer’s disease. Alzheimers Res Ther 5: , 28. |
[38] | Yanagisawa D, Ibrahim NF, Taguchi H, Morikawa S, Hirao K, Shirai N, Sogabe T, Tooyama I ((2015) ) Curcumin derivative with the substitution at C-4 position, but not curcumin, is effective against amyloid pathology in APP/PS1 mice. Neurobiol Aging 36: , 201–210. |
[39] | Taridi NM, Yahaya MF, Teoh SL, Latiff AA, Ngah WZ, Das S, Mazlan M ((2011) ) Tocotrienol rich fraction (TRF) supplementation protects against oxidative DNA damage and improves cognitive functions in Wistar rats. Clin Ter 162: , 93–98. |
[40] | Taridi NM, Abd Rani N, Abd Latiff A, Ngah WZ, Mazlan M ((2014) ) Tocotrienol rich fraction reverses age-related deficits in spatial learning and memory in aged rats. Lipids 49: , 855–869. |
[41] | Nagapan G, Meng Goh Y, Shameha Abdul Razak I, Nesaretnam K, Ebrahimi M ((2013) ) The effects of prenatal and early postnatal tocotrienol-rich fraction supplementation on cognitive function development in male offspring rats. BMC Neuroscience 14: , 77. |
[42] | Baddeley AD, Hitch G ((1974) ) Working memory. Psychol Learn Motiv 8: , 47–89. |
[43] | Belleville S, Chertkow H, Gauthier S ((2007) ) Working memory and control of attention in persons with Alzheimer’s disease and mild cognitive impairment. Neuropsychology 21: , 458–469. |
[44] | Moscardo E, Salvetti B, Becchi S, Bertini G, Fabene P ((2012) ) The novel object recognition test in rodents: Which are the essential methodological aspects? Proceedings of Measuring Behavior 2012, Spink AJ, Grieco F, Krips OE, Loijens LWS, Noldus LPJJ, Zimmerman PH, eds. Utrecht, The Netherlands August 28-31, 2012, pp. 476–478. |
[45] | Zhang R, Xue G, Wang S, Zhang L, Shi C, Xie X ((2012) ) Novel object recognition as a facile behavior test for evaluating drug effects in AβPP/PS1 Alzheimer’s disease mouse model. J Alzheimers Dis 31: , 801–812. |
[46] | Porquet D, Griñán-Ferré C, Ferrer I, Camins A, Sanfeliu C, del Valle J, Pallás M ((2014) ) Neuroprotective role of trans-resveratrol in a murine model of familial Alzheimer’s disease. J Alzheimers Dis 42: , 1209–1220. |
[47] | Zhou Y, Xie N, Li L, Zou Y, Zhang X, Dong M ((2014) ) Puerarin alleviates cognitive impairment and oxidative stress in APP/PS1 transgenic mice. Int J Neuropsychopharmacol 17: , 635–644. |
[48] | Armand-Ugón M, Aso E, Moreno J, Riera-Codina M, Sánchez A, Vegas E, Ferrer I ((2015) ) Memory improvement in the AβPP/PS1 mouse model of familial Alzheimer’s disease induced by carbamylated-erythropoietin is accompanied by modulation of synaptic genes. J Alzheimers Dis 45: , 407–421. |
[49] | Atamna H, Frey 2nd WH ((2007) ) Mechanisms of mitochondrial dysfunction and energy deficiency in Alzheimer’s disease. Mitochondrion 7: , 297–310. |
[50] | Bubber P, Haroutunian V, Fisch G, Blass JP, Gibson GE ((2005) ) Mitochondrial abnormalities in Alzheimer brain: Mechanistic implications. Ann Neurol 57: , 695–703. |
[51] | Gonzalez-Dominguez R, Garcia-Barrera T, Vitorica J, Gomez-Ariza JL ((2014) ) Region-specific metabolic alterations in the brain of the APP/PS1 transgenic mice of Alzheimer’s disease. Biochim Biophys Acta 1842: , 2395–2402. |
[52] | Vingtdeux V, Chandakkar P, Zhao H, Davies P, Marambaud P ((2011) ) Small-molecule activators of AMP-activated protein kinase (AMPK), RSVA314 and RSVA405, inhibit adipogenesis. Mol Med 17: , 1022–1030. |
[53] | Cai Z, Yan LJ, Li K, Quazi SH, Zhao B ((2012) ) Roles of AMP-activated protein kinase in Alzheimer’s disease. Neuromolecular Med 14: , 1–14. |
[54] | Nowak G, Bakajsova D, Hayes C, Hauer-Jensen M, Compadre CM ((2012) ) γ-Tocotrienol protects against mitochondrial dysfunction and renal cell death. J Pharmacol Exp Ther 340: , 330–338. |
[55] | Zheng H, Zheng Y, Zhao L, Chen M, Bai G, Hu Y, Hu W, Yan Z, Gao H ((2017) ) Cognitive decline in type 2 diabetic db/db mice may be associated with brain region-specific metabolic disorders. Biochim Biophys Acta 1863: , 266–273. |
[56] | Cunha RA ((2001) ) Adenosine as a neuromodulator and as a homeostatic regulator in the nervous system: Different roles, different sources and different receptors. Neurochem Int 38: , 107–125. |
[57] | Gomes CV, Kaster MP, Tomé AR, Agostinho PM, Cunha RA ((2011) ) Adenosine receptors and brain diseases: Neuroprotection and neurodegeneration. Biochim Biophys Acta 1808: , 1380–1399. |
[58] | Rahman A ((2009) ) The role of adenosine in Alzheimer’s disease. Curr Neuropharmacol 7: , 207–216. |
[59] | Ribeiro J, Sebastiao A, De Mendonça A ((2002) ) Adenosine receptors in the nervous system: Pathophysiological implications. Prog Neurobiol 68: , 377–392. |
[60] | Ansoleaga B, Jove M, Schluter A, Garcia-Esparcia P, Moreno J, Pujol A, Pamplona R, Portero-Otin M, Ferrer I ((2015) ) Deregulation of purine metabolism in Alzheimer’s disease. Neurobiol Aging 36: , 68–80. |
[61] | Jové M, Portero-Otín M, Naudí A, Ferrer I, Pamplona R ((2014) ) Metabolomics of human brain aging and age-related neurodegenerative diseases. Neuropathol Exp Neuro 73: , 640–657. |
[62] | Kaddurah-Daouk R, Zhu H, Sharma S, Bogdanov M, Rozen SG, Matson W, Oki NO, Motsinger-Reif AA, Churchill E, Lei Z, Appleby D, Kling MA, Trojanowski JQ, Doraiswamy PM, Arnold SE ((2013) ) Alterations in metabolic pathways and networks in Alzheimer/’s disease. Transl Psychiatry 3: , e244. |
[63] | Ali SF, Woodman OL ((2015) ) Tocotrienol rich palm oil extract is more effective than pure tocotrienols at improving endothelium-dependent relaxation in the presence of oxidative stress. Oxid Med Cell Longev 2015: , 150829. |
[64] | Blokland A ((1995) ) Acetylcholine: A neurotransmitter for learning and memory? Brain Res Rev 21: , 285–300. |
[65] | Wang H, Lian K, Han B, Wang Y, Kuo SH, Geng Y, Qiang J, Sun M, Wang M ((2014) ) Age-related alterations in the metabolic profile in the hippocampus of the senescence-accelerated mouse prone 8: A spontaneous Alzheimer’s disease mouse model. J Alzheimers Dis 39: , 841–848. |
[66] | Yatin SM, Yatin M, Aulick T, Ain KB, Butterfield DA ((1999) ) Alzheimer’s amyloid beta-peptide associated free radicals increase rat embryonic neuronal polyamine uptake and ornithine decarboxylase activity: Protective effect of vitamin E. Neurosci Lett 263: , 17–20. |
[67] | Lee L, Kang SA, Lee HO, Lee B-H, Jung IK, Lee JE, Heo Y-S ((2001) ) Effect of Supplementation of vitamin E and vitamin C on brain acetylcholinesterase activity and neurotransmitter levels in rats treated with scopolamine, an inducer of dementia. J Nutr Sci Vitaminol 47: , 323–328. |
[68] | Dysken MW, Sano M, Asthana S, Vertrees JE, Pallaki M, Llorente M, Love S, Schellenberg GD, McCarten JR, Malphurs J ((2014) ) Effect of vitamin E and memantine on functional decline in Alzheimer disease: The TEAM-AD VA cooperative randomized trial. JAMA 311: , 33–44. |
[69] | Sultana R, Piroddi M, Galli F, Butterfield DA ((2008) ) Protein levels and activity of some antioxidant enzymes in hippocampus of subjects with amnestic mild cognitive impairment. Neurochem Res 33: , 2540–2546. |
[70] | Aoyama K, Nakaki T ((2015) ) Glutathione in cellular redox homeostasis: Association with the excitatory amino acid carrier 1 (EAAC1). Molecules 20: , 8742–8758. |
[71] | Sautin YY, Johnson RJ ((2008) ) Uric acid: The oxidant–antioxidant paradox. Nucleosides Nucleotides Nucleic Acids 27: , 608–619. |
[72] | Cudaback E, Jorstad NL, Yang Y, Montine TJ, Keene CD ((2014) ) Therapeutic implications of the prostaglandin pathway in Alzheimer’s disease. Biochem Pharmacol 88: , 565–572. |
[73] | Lin S, Kanawati B, Liu L, Witting M, Li M, Huang J, Schmitt-Kopplin P, Cai Z ((2014) ) Ultrahigh resolution mass spectrometry-based metabolic characterization reveals cerebellum as a disturbed region in two animal models. Talanta 118: , 45–53. |
[74] | Chen X, Guo X, Huang R, Chen Y, Zheng Z, Shang H ((2014) ) Serum uric acid levels in patients with Alzheimer’s disease: A meta-analysis. PLoS One 9: , e94084. |
[75] | Gonzalez-Dominguez R, García-Barrera T, Vitorica J, Gómez-Ariza JL ((2015) ) Metabolomic investigation of systemic manifestations associated with Alzheimer’s disease in the APP/PS1 transgenic mouse model. Mol Biosyst 11: , 2429–2440. |
[76] | Paglia G, Stocchero M, Cacciatore S, Lai S, Angel P, Alam MT, Keller M, Ralser M, Astarita G ((2016) ) Unbiased metabolomic investigation of Alzheimer’s disease brain points to dysregulation of mitochondrial aspartate metabolism. J Proteome Res 15: , 608–618. |
[77] | Skoumalová A, Hort J ((2012) ) Blood markers of oxidative stress in Alzheimer’s disease. Cell Mol Med 16: , 2291–2300. |
[78] | Farooqui AA, Ong WY, Horrocks LA ((2004) ) Biochemical aspects of neurodegeneration in human brain: Involvement of neural membrane phospholipids and phospholipases A2. Neurochem Res 29: , 1961–1977. |
[79] | Atkinson J, Epand RF, Epand RM ((2008) ) Tocopherols and tocotrienols in membranes: A critical review. Free Radic Biol Med 44: , 739–764. |
[80] | Farooqui AA, Wells K, Horrocks LA ((1995) ) Breakdown of membrane phospholipids in Alzheimer disease. Mol Chem Neuropathol 25: , 155–173. |
[81] | Cutler RG, Kelly J, Storie K, Pedersen WA, Tammara A, Hatanpaa K, Troncoso JC, Mattson MP ((2004) ) Involvement of oxidative stress-induced abnormalities in ceramide and cholesterol metabolism in brain aging and Alzheimer’s disease. Proc Nat Acad Sci U S A 101: , 2070–2075. |
[82] | Lee JT, Xu J, Lee JM, Ku G, Han X, Yang DI, Chen S, Hsu CY ((2004) ) Amyloid-beta peptide induces oligodendrocyte death by activating the neutral sphingomyelinase-ceramide pathway. J Mol Cell Biol 164: , 123–131. |
[83] | Puglielli L, Ellis BC, Saunders AJ, Kovacs DM ((2003) ) Ceramide stabilizes beta-site amyloid precursor protein-cleaving enzyme 1 and promotes amyloid beta-peptide biogenesis. J Biol Chem 278: , 19777–19783. |
[84] | Serbinova E, Tsuchiya M, Goth S, Kagan V, Packer L ((1993) ) Vitamin E in Health and Disease. Eds L Inc., New York, pp. 235–243. |
[85] | Ayasolla K, Khan M, Singh AK, Singh I ((2004) ) Inflammatory mediator and beta-amyloid (25-35)-induced ceramide generation and iNOS expression are inhibited by vitamin E. Free Radic Biol Med 37: , 325–338. |
[86] | Babenko NA, Hassouneh L, Kharchenko VS, Garkavenko VV ((2012) ) Vitamin E prevents the age-dependent and palmitate-induced disturbances of sphingolipid turnover in liver cells. Age (Dordr) 34: , 905–915. |
[87] | Jiang Q, Wong J, Fyrst H, Saba JD, Ames BN ((2004) ) γ-Tocopherol or combinations of vitamin E forms induce cell death in human prostate cancer cells by interrupting sphingolipid synthesis. Proc Natl Acad Sci U S A 101: , 17825–17830. |