Pain Assessment in Dementia: Evaluation of a Point-of-Care Technological Solution
Abstract
Pain is common among people with moderate to severe dementia, but inability of patients to self-report means it often goes undetected and untreated. We developed the electronic Pain Assessment Tool (ePAT) to address this issue. A point-of-care App, it utilizes facial recognition technology to detect facial micro-expressions indicative of pain. ePAT also records the presence of pain-related behaviors under five additional domains (Voice, Movement, Behavior, Activity, and Body). In this observational study, we assessed the psychometric properties of ePAT compared to the Abbey Pain Scale (APS). Forty aged care residents (70% females) over the age of 60 years, with moderate to severe dementia and a history of pain-related condition(s) were recruited into the study. Three hundred and fifty-three paired pain assessments (either at rest or post-movement) were recorded and analyzed. The ePAT demonstrated excellent concurrent validity (r = 0.882, 95% CI: 0.857–0.903) and good discriminant validity. Inter-rater reliability score was good overall (weighted κ= 0.74, 95% CI: 0.68–0.80) while internal consistency was excellent. ePAT has psychometric properties which make it suitable for use in non-communicative patients with dementia. ePAT also has the advantage of automated facial expression assessment which provides objective and reproducible evidence of the presence of pain.
INTRODUCTION
Pain is a frequent symptom in residential aged care with up to 80% of residents experiencing pain at some point of time, whilst at least 50% of those with dementia have pain on a regular basis [1, 2]. Pain often is under-detected and undertreated particularly in those with moderate to severe dementia who can no longer self-report pain due to the neurodegenerative changes associated with the condition [3]. In these individuals, pain may manifest as a behavioral disturbance(s) [4], which could be inappropriately treated with a range of psychotropic medications such as benzodiazepines and antipsychotics [5].
An alternative communication channel to report pain for these residents is through non-verbal signals. Non-verbal communications were recognized by the American Geriatric Society (AGS) in 2002 as indicators of pain, and since have been recommended for inclusion in behavioral (also known as observational) pain assessment tools [1]. Of these tools, none use objective facial measures, and consequently are all dependent on the subjective knowledge, skills and training level of raters. This is problematic given the fact that there is a high staff turnover in the aged care industry and inconsistencies exist among health-care professionals in detecting pain [6, 7].
Facial expressions are key non-verbal pain related behaviors that have been included in many observational pain scales [8]. Existing scales often contain abstract and abstruse descriptors such as grimacing, which are difficult to identify as pain related expressions by assessors. The Facial Action Coding System (FACS) offers a more objective way of describing and measuring these facial expressions [9]. FACS is an anatomical catalogue and taxonomy of facial expressions, which contains 52 Action Unit (AU) codes [9]. FACS is regarded the gold standard of evaluating facial expressions including those related to pain [10]. Despite its efficiency in categorizing expressions, manual facial decoding is laborious, time consuming, and inconvenient, because it uses video recordings and requires lengthy training and experts’ input; hence making it unrealistic for clinical applications [10, 11]. A recent systematic review supported the notion of using FACS in pain assessment, including automated pain assessment FACS-related systems [12].
Patients with Alzheimer’s disease (the most common form of dementia) have been shown to display an increase in the frequency and intensity of facial activity as measured by Action Units (AUs) of the FACS [13]. Kunz et al. reported that pain related AU codes (AU4,6/7,9/10) are three times more frequent in people with dementia compared to healthy controls [13], perhaps due to their impaired operant learning and reinforcement ability to mask negative expressions such as pain [14–16].
With the advancement of technologies including computer vision and smart devices (e.g., Android and iOS smartphones), automation may reduce the reliance on human raters, making pain assessment less prone to error and less subjective. The purpose of this paper is to briefly describe a new pain assessment tool, namely the electronic Pain Assessment Tool (ePAT) [17] that integrates these technologies to benefit patients with cognitive impairment and to evaluate its psychometric properties compared to the Abbey Pain Scale (APS).
METHODS
Pain assessment tools
Abbey Pain Scale (standard care)
The APS was used as a comparator in this study because it is frequently used in clinical practice and is also endorsed by the Australian Pain Society in their recommended management strategies [18]. The instrument has good psychometric properties in older adults with dementia as reported in a number of systematic reviews in the literature [11, 19–23]. The instrument consists of six subscales, namely: vocalization, facial expressions, change in body language, behavioral change, physiological change, and physical change [24]. Each subscale is scored on an ordinal rating range of 0–3 to indicate intensity. A score of 0 indicates absence of pain while mild = 1, moderate = 2, and severe = 3. A total pain score (sum of subscales) of 0–2 indicates no pain, 3–7 mild pain, 8–13 moderate pain, and 14 and above indicates severe pain [24]. Figure 1 shows the Abbey Pain Scale.
Fig.1
The Abbey Pain Scale. Source: Abbey J, De Bellis A, Piller N, Esterman A, Giles L, Parker D, Lowcay B. Funded by the JH & JD Gunn Medical Research Foundation 1998–2002.
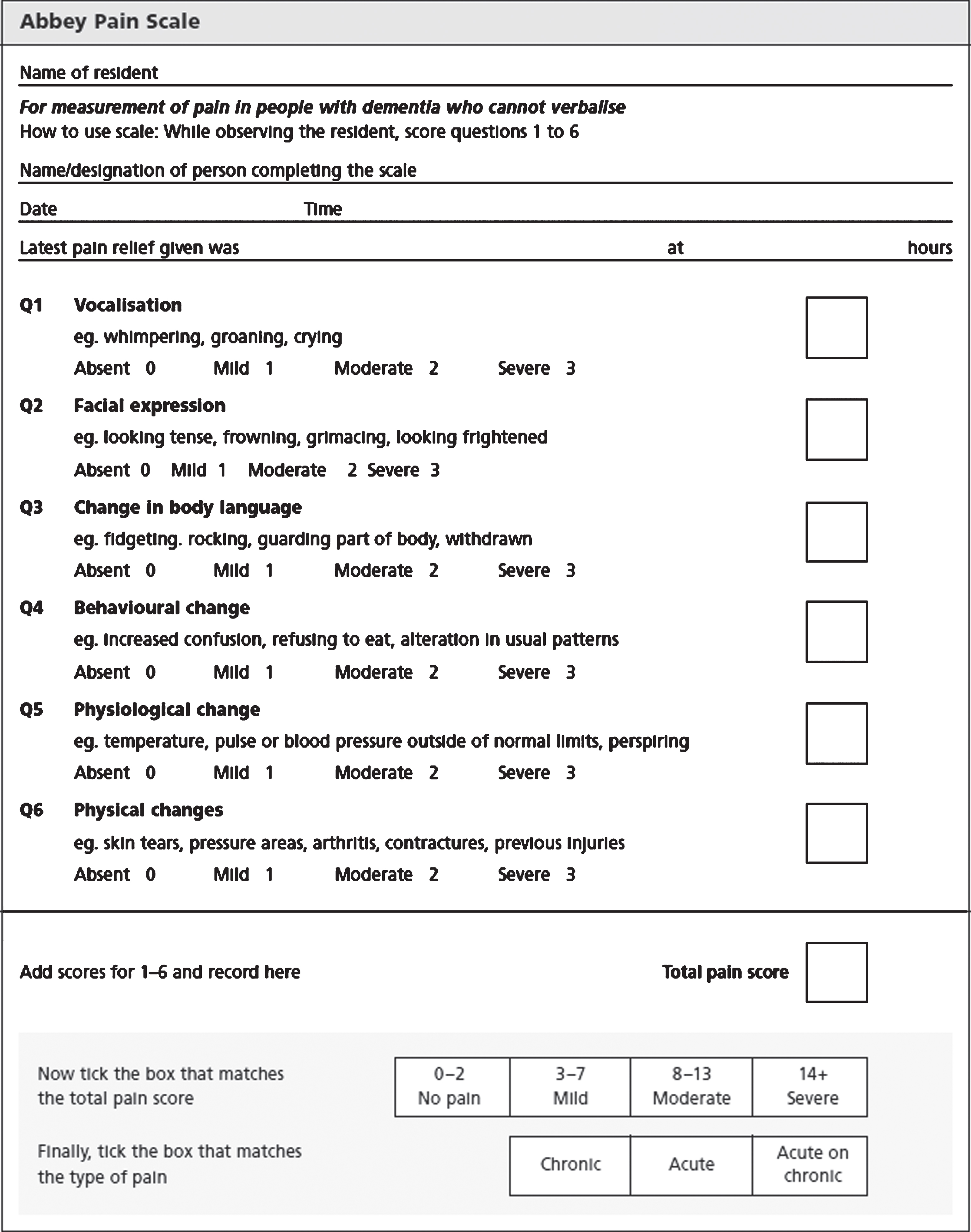
The electronic Pain Assessment Tool (ePAT) (new tool)
Purpose: The ePAT is a multimodal pain scale designed to assist clinicians and health care workers assess pain in people with moderate to severe dementia at the point of care.
Developers: The ePAT has been developed by a research team at Curtin University, Western Australia, in collaboration with the Swiss company, nViso SA which is located at the Swiss Federal Institute of Technology in Lausanne (EPFL).
Content (Images 1–6): The ePAT utilizes automated facial recognition technology to detect the presence of facial micro-expressions indicative of the presence of pain, which, when combined with a range of behavioral and physical features based on the other five domains of the AGS, can be used to assess pain severity. Using a 10 second video of the subject’s face, the ePAT maps the face and automatically identifies in real-time the presence of pain related facial micro-expressions (otherwise known as Action Units).
Image 1
Face detection and tracking in the ePAT App during a clinical encounter.
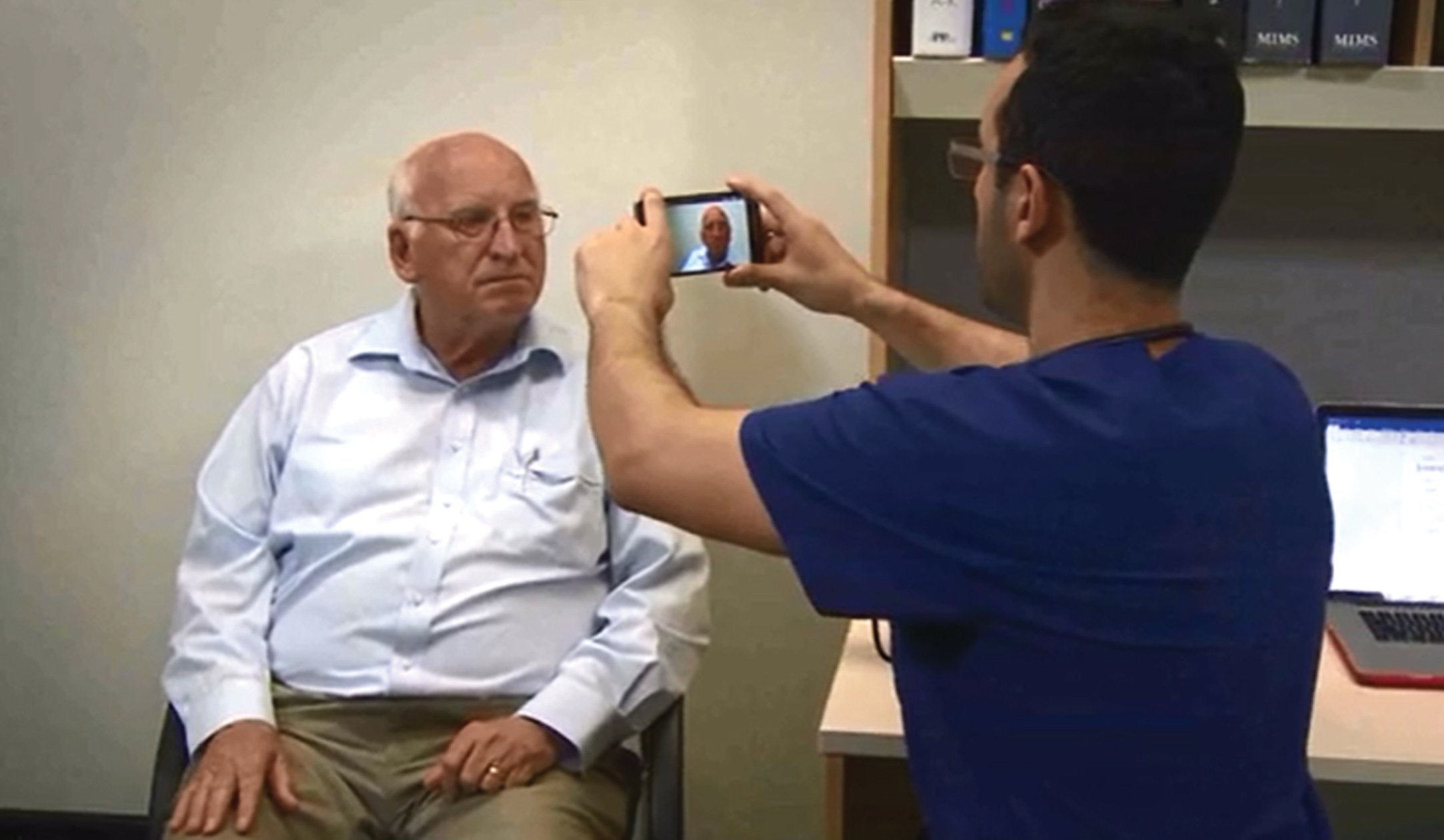
Image 2
Facial features extraction of the ePAT App.
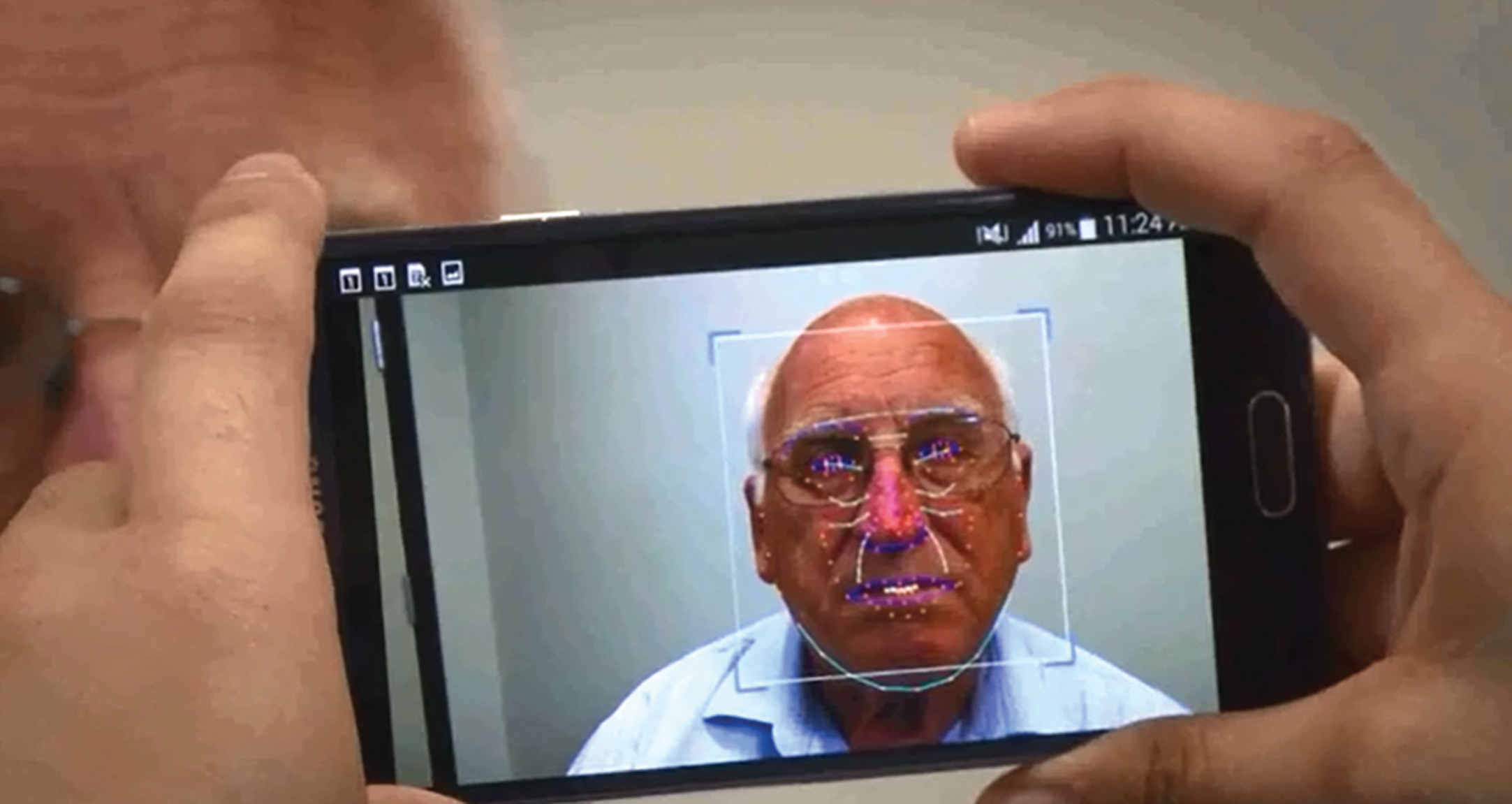
Image 3
Detection of facial Action Units (AUs) codes in the ePAT App.
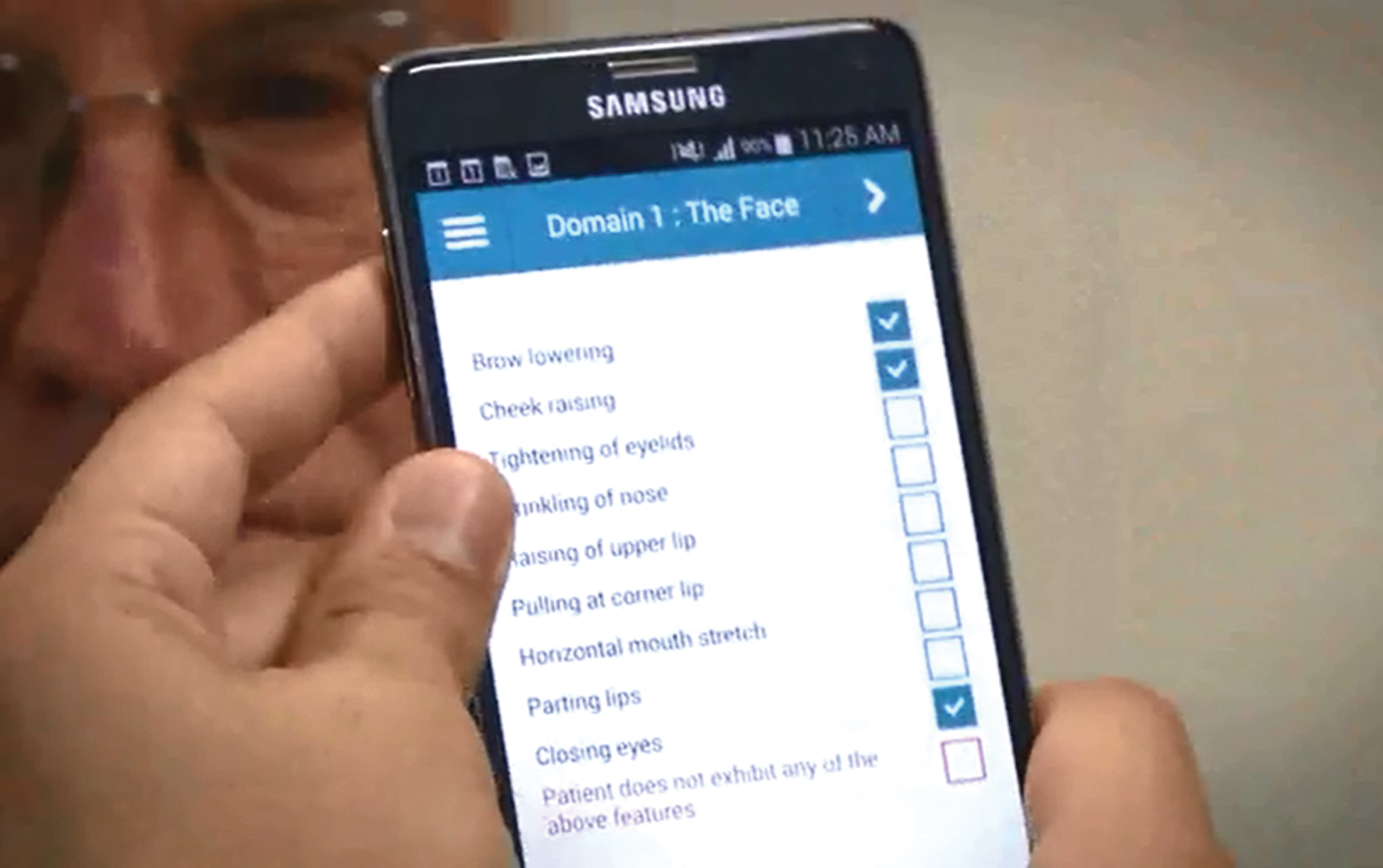
Image 4
Domain 5 of the ePAT; The Activity.
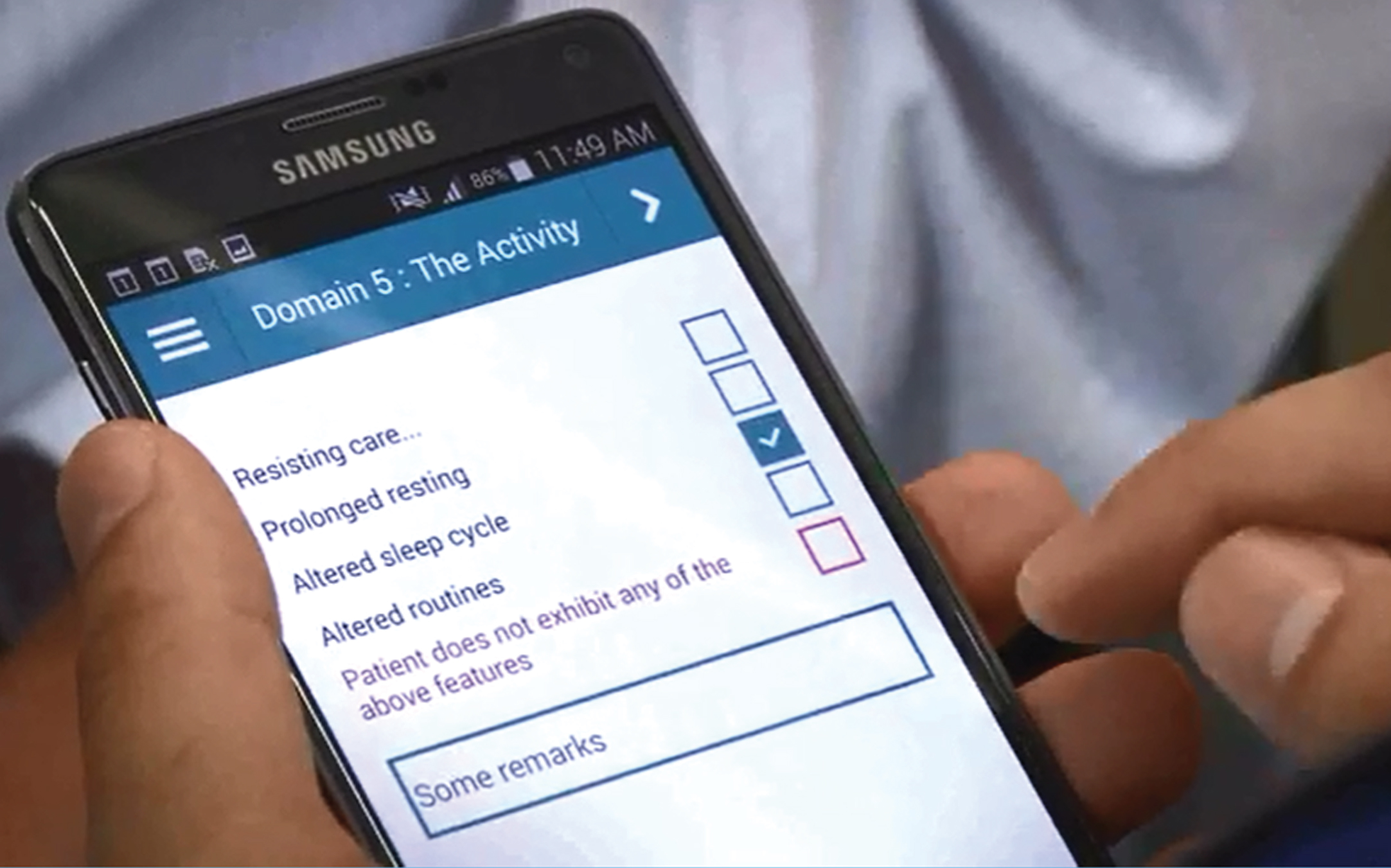
Image 5
Domain 6 of the ePAT; The Body.
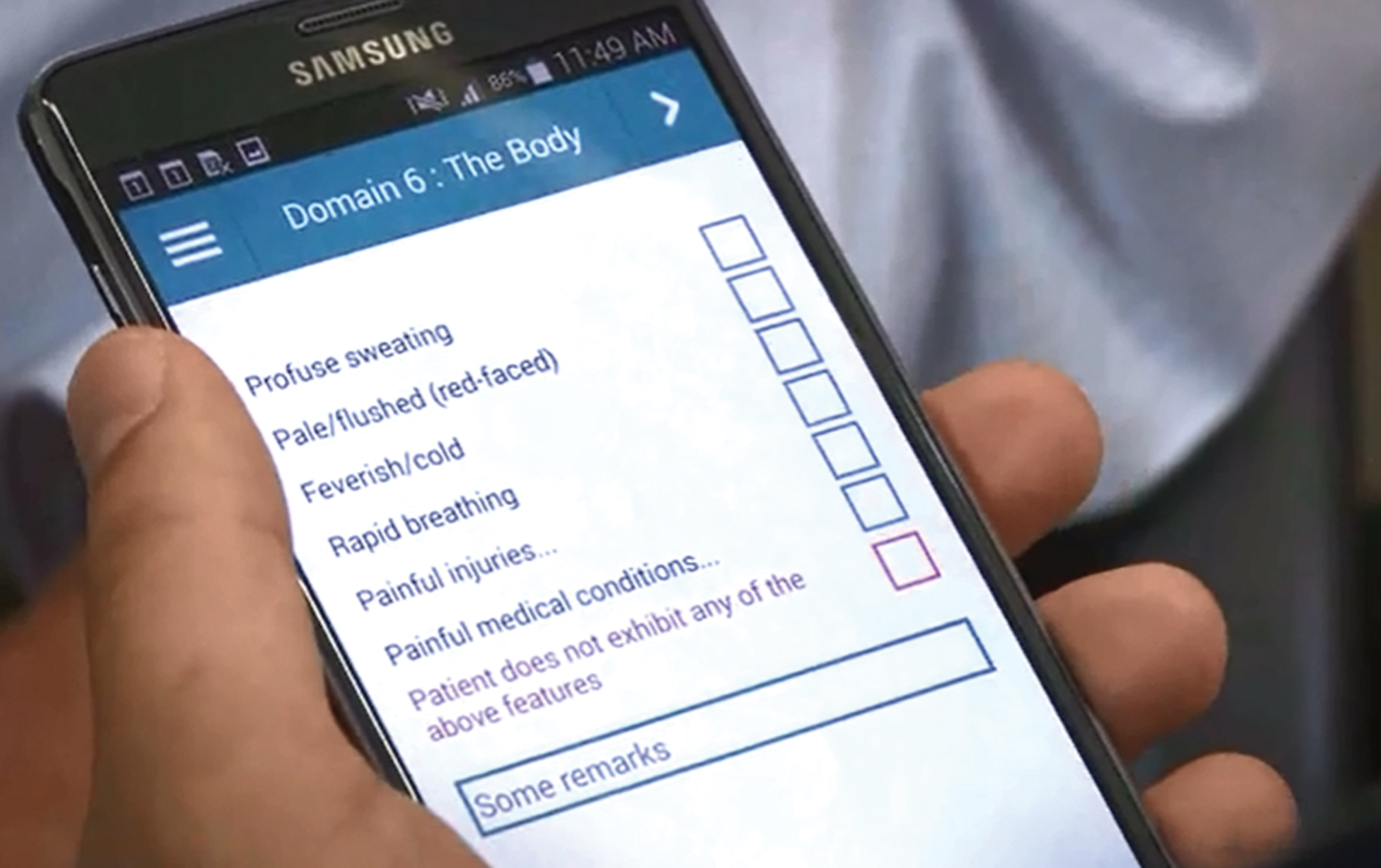
Image 6
Total score screen of the ePAT App depicting to pain intensity score.
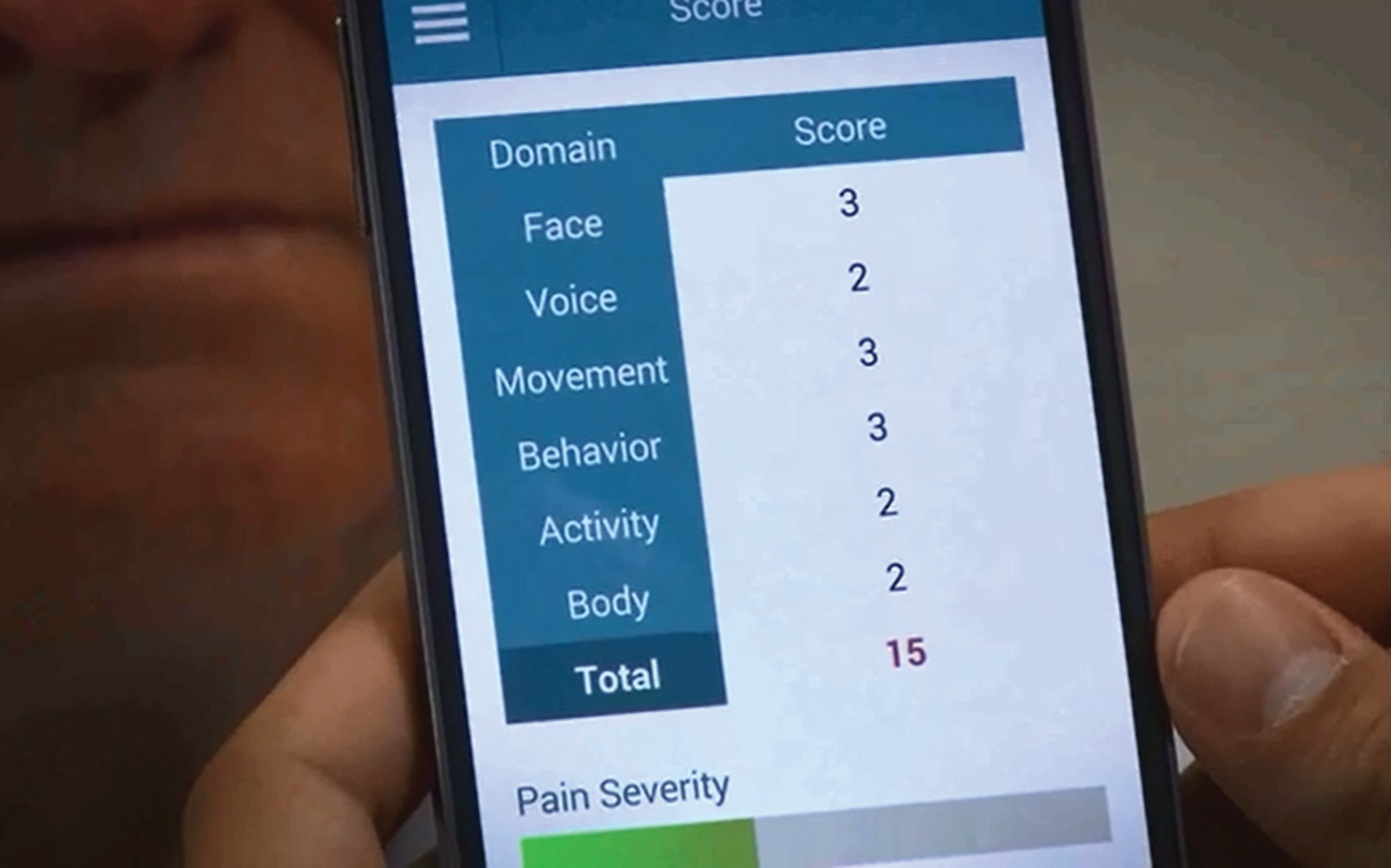
The ePAT consists of 42 descriptor items distributed across six domains in the following order [Domain 1: The Face (9 items), Domain 2: The Voice (9 items), Domain 3: The Movement (7 items), Domain 4: The Behavior (7 items), Domain 5: The Activity (4 items) and Domain 6: The Body (6 items)]. Each domain represents a subclass that evaluates a certain dimension of pain (refer to Table 1 for more details).
Table 1
Comparison between the observational pain tools APS and ePAT
APS | ePAT | |
Number of domains | 6 | 6 |
Tool item domains &number of descriptors per domain | Vocalization (1 item) | The Face (9 items) |
(e.g., whimpering, groaning, crying) | [see facial expressions below] | |
Facial expressions (1 item) | The Voice (9 items) | |
[e.g., looking tense, frowning grimacing, looking frightened] | [noisy Pain Sounds, e.g., ouch, ah, mm, requesting help repeatedly, groaning, moaning, crying, screaming, loud talk, howling, sighing] | |
in body language (1 item) | The Movement (7 items) | |
fidgeting, rocking, guarding part of body, withdrawn] | [altered or random leg/arm movement, restlessness, freezing, guarding/ | |
Behavioral change# (1 item) | touching body part, moving away, abnormal (altered) sitting/standing/walking, pacing/wandering] | |
[e.g., increased confusion, refusing to eat, alteration in usual patterns] | The Behavior (7 items) | |
Physiological change# (1 item) | [introvert (unsocial) or altered behavior, verbally offensive, aggressive, fear or extreme dislike of touch, people, inappropriate behavior, confused, distressed] | |
[e.g., temperature, pulse or blood pressure outside normal | The Activity (4 items) | |
limits, perspiring, flushing or pallor] | [resisting care, prolonged resting, altered sleep cycle, altered routines] | |
Physical change# (1 item) | The Body (6 items) | |
[e.g., skin tears, pressure areas, arthritis, contractures, | ||
previous injuries] | sweating, pale/flushed (red-faced), feverish/cold, rapid breathing, painful injuries, painful medical conditions] | |
Facial expressions | Abstract description | Specific annotation |
Emotion-like expressions | Action Unit (AU) codes of Facial Action Coding System (FACS) | |
(frowning, grimacing, looking frightened, | AU4: Brow Lowering | |
looking tense) | AU6: Cheek Raising | |
AU7: Tightening of Eyelids | ||
AU9: Wrinkling of Nose | ||
AU10: Raising of Upper Lip | ||
AU12: Pulling at Corner Lip | ||
AU20: Horizontal Mouth Stretch | ||
AU25: Parting Lips | ||
AU43: Closing Eyes | ||
Scoring format | Ordinal | Binary |
[4 point scale (absent = 0, mild = 1, moderate = 2, severe = 3) for each of the 6 domains] | [2 point scale (no = 0, yes = 1) for each item in each domain] | |
Scoring procedure | Pen and paper recording | Automated facial recognition for the Face domain technology (with an option of manual recording by the user) and touch screen electronic completion of other 5 domains using a smart device |
Total pain score range | 0–18 | 0–42 |
#Change refers to observer assessed change compared to previous assessment (relies on having familiarity with the person being assessed).
Platform used and method of administration: The technology is packaged in a software app that can be used across a range of mobile smart devices. The current Android version of the app tested during this study was installed on a Samsung Note 3 (SM-N9005) device. The ePAT is also an observational (informant-based) tool, which can be administered by a care worker or clinician (user) using a smart device. The user must be trained on the use of the tool and be familiar with the patient undergoing assessment. The user needs to navigate from one domain to another to complete the assessment.
Scoring: The ePAT uses a hybrid model in which the Face domain is fully automated while other domains (Domains 2–6) are questionnaire-based checklists manually completed by the assessor, using the mobile device. Similar to the Pain Assessment Checklist for Seniors with Limited Ability to Communicate (PACSLAC), a binary (2-point) format is adopted to evaluate the presence (score = 1) or absence (score = 0) of pain related behaviors on each of the 42 items. Magnitude of pain is measured by obtaining a cumulative score across all items. Total pain score, cumulated over all domains, can range from 0–42, with the corresponding band categories of pain intensity (no pain, mild, moderate, severe) to be explored in this study.
Conceptual foundation: The tool was developed on the basis of the definition of pain as “an unpleasant sensory and emotional experience associated with actual or potential tissue damage, or described in terms of such damage” [25]. There is also a great need for developing novel and innovative pain assessment instruments for non-verbal people with dementia as evident in the current literature. A meta-review by Lichtner et al. suggested that new pain assessment tools need to be developed on an innovative conceptual basis [26]. In addition, a review by Hadjistavropoulos et al. has strongly recommended that including a FACS-based pain expression should be considered by researchers for future development and refinement of pain instruments for older adults with dementia [27].
Therefore, we considered these main principles in designing the ePAT:
1) Objectivity
(a) Through integration of FACS into the tool
(b) Via automation: this is achieved by using a deep learning algorithm, with the purpose of reducing proxy rating error associated with human judgement
(c) Use of a binary (yes/no) approach to the identification of the presence of non-facial pain cues
2) Comprehensiveness
(a) Inclusion of AGS items in the tool to identify subtle behavioral changes based on pain items specifically geared towards older persons with dementia
3) Portability and smart device interoperability
(a) Smart device capabilities (such as high computational efficiency, e.g., processing power, digitization, and in-built cameras) and their popular use (due to reasonable costs, use with various platforms, e.g., Android, iOS) make them suitable to facilitate pain assessment at the point-of-care.
A comparative account between the ePAT and APS is summarized in Table 1.
Ethics
All clinical assessments were performed in accordance with principles outlined in the Declaration of Helsinki, Alzheimer’s Australia Guidelines, and clauses for undertaking research in cognitively impaired individuals by the Australian National Statement for Ethical Conduct in Human Research.
Ethical approval (HREC: HR10/2014) was granted by Human Research Ethics Committee, Curtin University, Western Australia and by ethics review boards of participating facilities. Informed consent could not be elicited from residents with dementia due to their impaired cognitive capacity. Thus, proxy consents were provided by relatives or an authorized representative of the cognitively impaired residents prior to participation. Proxies were notified that they could revoke their consent at any time without affecting the quality of care or the relationship of participants with those working in the aged care facility. Verbal explanations using very simple language (e.g., “we are checking whether you have any pain today by taking a short video of you”) were also used to explain the study to the residents.
Design and setting
The study was a prospective observational study which involved residents from three metropolitan aged care homes (ACHs) in Perth, Western Australia.
Participants
Residents were eligible to enroll if they met the following criteria: (1) age greater than 60 y, (2) living in a designated dementia unit of the ACH, (3) had a diagnosis of dementia, (4) their cognitive score based on the Mini-Mental State Examination (MMSE): <19 or Psychogeriatric Assessment Scale–Cognitive Impairment Scale (PAS-CIS): >10, and (5) possessed a documented history of a chronic pain condition such as osteoarthritis or currently suffer from acute (e.g., urinary tract infection), recurrent (e.g., gout) or incidental pain (e.g., pressure sores).
Residents were excluded from the study if they could not partially or completely exhibit any facial expression (for example as a result of a facial palsy), were clinically too unwell, or where it was inappropriate for them to be assessed for pain, as determined by the treating doctor.
Protocol plan
The study was conducted over a 13-week period in each of the three participating ACHs. The study was initiated at Aged Care Home 1 (ACH 1) from March-July 2015, then Aged Care Home 2 (ACH 2) from October 2015-January 2016, and Aged Care Home 3 (ACH 3) from January-April 2016. The choice of 13 weeks was made to allow adequate time for testing to occur under various conditions and while residents were doing their routine activities (i.e., at rest and upon movements, e.g., walking, repositioning, bathing, etc.).
Each resident was independently evaluated using the two assessment tools during routine care. The APS (i.e., standard care) was administered by a staff member (nurse or carer) employed by the facility as part of normal care, while the ePAT (the new tool) was administered, in most instances, by the primary researcher (MA), although health care professionals (e.g., registered nurse), personal care workers, or nursing and occupational therapy students also conducted some assessments. All raters were blinded to each other’s assessments. With the exception of the health science students, those involved in performing the assessments were already experienced in using APS or ePAT. Practical training on the use of the ePAT and the APS was delivered by the primary investigator to health science students. Paired pain assessments were undertaken during various levels of activities such as walking, after toileting or showering to induce nociceptive painful experiences, and during resting to mimic non-nociceptive periods.
Pain ratings were conducted mainly during daytime between 8 am and 6 pm. Ratings were undertaken indoors in multiple locations (e.g., activity room, resident’s room, dining room) inside the ACHs. In cases where the ePAT assessor was unfamiliar with the resident, care staff not involved with the study were consulted to answer various questions about residents’ behaviors (e.g., sleeping/eating pattern). Both ePAT and APS assessments were brief in nature and they were administered either concurrently or within 2-3 min of each other. The order in which the assessments were delivered was random to minimize the possibility of any learning effect.
Statistical analysis
Standard descriptive statistics were used to summarize the study participants and number of assessments conducted (frequencies and percentages for categorical variables, means, standard deviations, and ranges for continuous variables).
Concurrent validity was assessed using the Pearson’s correlation coefficient between the overall pain scores assigned by the APS and ePAT instruments, and separately for observations made at rest and following movement. The correlation is not a measure of exact agreement, as the instruments are based on different scoring mechanisms, but a strong correlation would indicate that the ePAT is equivalent to the APS up to a scaling factor. A refinement of the Pearson’s correlation coefficient was also calculated, following the method of Lam et al. [28], using a SAS macro described by Hamlett [29]. This refinement took into account the repeated measurements made on each participant in case agreement between APS and ePAT differed between participants.
Discriminant validity investigated whether the agreement between APS and ePAT depended on the conditions (at rest or with movement). This was explored by using the difference in pain scores (APS minus ePAT) as the dependent variable in a random effects regression model, with the timing (rest or with movement) as the independent variable and the subject number as the random effect. Naming the subject as a random effect in this model took into account any correlations between the repeated measures made on each study participant. The p-value associated with timing indicated its influence on the agreement between measures.
Inter-rater reliability was assessed by classifying the pain scores for APS and ePAT into four categories from no pain to mild, moderate, and severe pain. Agreement between the measures according to these categories was then assessed using the Cohen’s kappa statistic. The standard (unweighted) kappa is a measure of exact agreement within categories, while the weighted kappa gives some weight to small disagreements.
Internal consistency between the two measures was calculated using Cronbach’s alpha. This assesses the extent to which two or more measures are essentially measuring the same construct [30]. It was used in this study to compare the overall APS and ePAT scores. Values of Cronbach’s alpha above 0.7 are indicative of a good agreement between measures [30].
Statistical analyses were performed using the SAS version 9.2 software (SAS Institute Inc, Cary, NC, USA, 2008).
RESULTS
Demographic characteristics
A total of 40 residents were recruited into the study from the three aged care homes. The average age of the participants was 79.7 y (SD: 9.1; range: 60 to 98 y). The majority of residents were females (70%) and Caucasians (n = 39), with the remaining participant being Asian. The residents had a range of chronic pain conditions as a result of arthritis (e.g., osteoarthritis, rheumatoid arthritis and gout), previous injuries and/or surgeries, skin tears and sores, dental disorders (e.g., sore gums associated with gingivitis), and neuropathic pain (e.g., post-herpetic neuralgia). Seventy percent of the cohort had one or more documented chronic pain diagnoses. A number of participating residents were bed-ridden, immobile or had limited mobility. All residents were identified as having moderate to severe cognitive impairment based on a PAS-CIS score in the range of 10–15 and 16–21, respectively. Eighty-seven percent of residents had severe impairment. MMSE scores were unable to be completed for most residents due to severe impairments and were only recorded for eight residents with a mean of 14.0±3.9. More than half (57.5%) of the sample had a diagnosis of Alzheimer’s dementia while 25% reported to have an unspecified type of dementia. Other documented dementias were frontotemporal dementia (7.5%), Lewy body dementia (2.5%), Parkinsonian’s dementia (5%), and mixed dementia (2.5%). Refer to Table 2 for further details.
Table 2
Resident demographics and pain characteristics
Number (%) | Mean (SD) | |
Age (y) | 79.7 (9.1) | |
(Median: 79.0, range: 60–98) | ||
Gender | ||
Female | 29 (70) | |
Male | 11 (30) | |
Country of birth | ||
Australia | 16 (40) | |
Czech Republic | 1 (2.5) | |
England | 13 (32.5) | |
Ireland | 1 (2.5) | |
Lithuania | 1 (2.5) | |
Mauritius | 1 (2.5) | |
Scotland | 3 (7.5) | |
Unknown | 4 (10) | |
Ethnicity | ||
Caucasians | 39 (97.5) | |
Asian | 1 (2.5) | |
Primary language | ||
English | 38 (95) | |
French | 1 (2.5) | |
Lithuanian | 1 (2.5) | |
Mobility | ||
Limited | 18 (45) | |
Immobile | 4 (10) | |
Bed-ridden | 2 (5) | |
Cognitive performance | ||
MMSE (range: 8–17) | 8 (20) | 14.0 (3.9) |
PAS-CIS (range: 10–15) | 5 (12.5) | |
PAS-CIS (range: 16–21) | 35 (87) | |
Diagnosis of dementia | ||
Alzheimer’s disease | 23 (57.5) | |
Frontotemporal dementia | 3 (7.5) | |
Lewy Body dementia | 1 (2.5) | |
Parkinson’s dementia | 2 (5) | |
Mixed (Alzheimer’s/Vascular | 1 (2.5) | |
dementia) | ||
Unspecified | 10 (25) | |
Number of documented chronic | ||
painful diagnoses | ||
0 | 12 (30) | |
1 | 10 (25) | |
2 | 9 (22.5) | |
3 | 4 (10) | |
4 | 1 (2.5) | |
5 | 4 (10) |
Pain assessment data
Pain assessments for residents were undertaken during routine care while at rest or with movement. The number of paired pain assessments per resident varied and ranged from 2 to 15. Overall, the total number of paired pain assessments was 353 (Table 3). Those performing the pain assessments (Table 4) included seven nurses (clinical nurse (CN); n = 2, registered nurse (RN); n = 3, and enrolled nurse (EN); n = 2), two care workers (CW), four health sciences students (HSS), and the primary investigator (MA).
Table 3
Pain assessment data for the three participating aged care homes
Aged Care Homes | ||||
ACH 1 | ACH 2 | ACH 3 | Combined | |
Study period | Mar 2015 –Jul 2015 | Oct 2015 –Jan 2016 | Jan 2016 –Apr 2016 | Mar 2015 –Apr 2016 |
Sample size | 8 | 15 | 17 | 40 |
% males | 50% | 40% | 12% | 30% |
Total No. of ePAT assessments | 40 | 127 | 186 | 353 |
No. of ePAT assessments during rest | 22 | 70 | 118 | 209 |
No. of ePAT assessments upon movement | 18 | 57 | 69 | 144 |
Table 4
Number of assessments completed by each assessor
Number of assessments | ACH 1 | ACH2 | ACH 3 | Total |
per staff classification | ||||
CN# | 1 | 116 | 0 | 117 |
RN# | 11 | 0 | 156 | 167 |
EN# | 23 | 11 | 0 | 34 |
CW# | 1 | 0 | 30 | 31 |
HSS# ,* | 8@ | 0 | 0 | 8 |
MA* | 36 | 127 | 186 | 349 |
Total | 80 | 254 | 372 | 706 |
CN, clinical nurse; CW, care worker; RN, registered nurse; EN, enrolled nurse; HSS, health science student; MA, primary investigator. #completed APS assessments, *completed ePAT assessments, @students did a total of four APS and four ePAT assessments.
Concurrent validity
Pearson’s correlation coefficient to assess overall agreement between APS and ePAT was 0.882 (95% CI: 0.857–0.903). This was based on the 353 paired assessments made on the 40 study participants. This correlation indicates a very strong and positive relationship between the two scores. Figure 2 below represents the ePAT pain scores graphed against the APS scores, with black dots indicating pain score at rest and red dots pain score with movement. Note that some dots represent more than one observation.
Fig.2
Scatter plot of individual APS scores and ePAT scores. Black dots indicating pain score at rest and red dots pain score with movement. Note that some dots represent more than one observation.
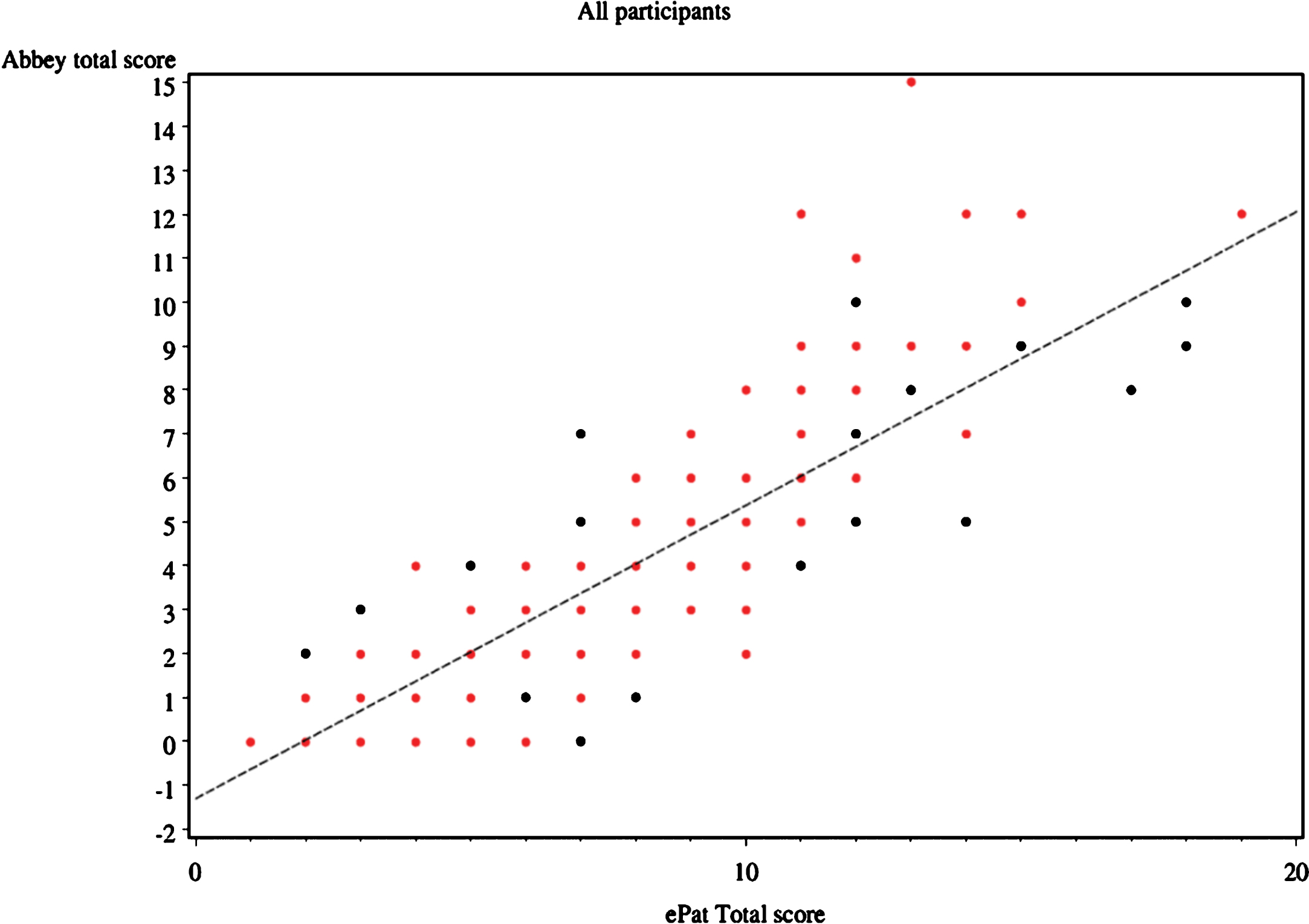
In a similar fashion the ePAT pain scores and the APS scores demonstrate significant correlation both at rest (r = 0.880; 95% CI: 0.845–0.907) and with movement (r = 0.894; 95% CI: 0.855–0.922). The refinement to the standard correlation coefficient, which took into account the repeated measures on each participant, led to adjusted correlation coefficients at rest: r = 0.881; and with movement: r = 0.894. As these figures differed only in the third decimal place from the unadjusted figures, this suggests that the repeated measurements on participants had little impact on the correlation between the measures.
Discriminant validity
Discriminant validity was assessed by comparing ePAT scores to APS for the same resident at rest and then after movement, e.g., movement in walking, repositioning, and toileting. As was the case with APS scores, the ePAT pain scores increased after residents were subjected to movement which elicited pain. The random effects regression model showed that the difference between ePAT and APS scores was not significantly influenced by the timing of the assessment (at rest versus with movement; p = 0.795).
Inter-rater reliability
Association between pain groups of APS and ePAT was evaluated using a contingency table. A preliminary analysis of the results (n = 229) led to the following categorization of ePAT scores into pain groups as follows: 1–6 = No pain; 7–11 = Mild pain, 12–15 = Moderate pain, and 16–42 = Severe pain. These cut-off scores were selected as they provided good agreement with the APS with respect to these categories of pain. They were obtained by cross-tabulating the raw ePAT scores against the APS categories, and optimum cut-off scores were obtained in a manner similar to a discriminant analysis. These categories continued to give good agreement with the APS categories for the full dataset (n = 353). Table 5 below showed the overall agreement.
Table 5
Numbers shown in the cells are the number of assessments (percentage of the APS category)
APS category | ePAT category | Total | |||
No pain | Mild | Moderate | Severe | ||
No pain | 183 (95.3) | 9 (4.7) | 0 | 0 | 192 |
Mild | 32 (23.4) | 97 (70.8) | 8 (5.8) | 0 | 137 |
Moderate | 0 | 5 (21.7) | 14 (60.9) | 4 (17.4) | 23 |
Severe | 0 | 0 | 1 (100) | 0 | 1 |
The weighted kappa scores (Table 6) demonstrated that there was moderate to good reliability based on the following guide: Kappa ≤ 0.20 is considered poor; 0.21–0.40, fair; 0.41–0.60, moderate; 0.61–0.80, good, and 0.81–1.00, very good [31].
Internal consistency
Table 6
Inter-rater reliability data for ePAT versus Abbey Pain Scale
Activity | Weighted Kappa | 95% CI |
All (Rest + Movement) n = 353 | 0.74 | 0.69–0.80 |
At rest n = 209 | 0.71 | 0.63–0.80 |
With movement n = 144 | 0.78 | 0.70–0.86 |
The Cronbach’s alpha (α) statistic was used to compare the overall APS and ePAT scores. Cronbach’s α was 0.925 and Pearson’s correlation coefficient (r) was r = 0.882 (95% CI: 0.857 –0.903). Internal consistency was excellent overall for ePAT versus APS.
DISCUSSION
The results of this study demonstrated that ePAT offers a valid and reliable new method to assess pain in people with moderate to severe dementia who can no longer self-report their pain. We believe it offers significant advantages over currently available behavioral pain assessment tools. It utilizes automated facial recognition technology to identify the presence of specific AUs which are associated with pain. In addition, it utilizes binary answers to each parameter rather than subjective 0–3 scoring of intensity as with APS, therefore removing the subjectivity associated with the assessment of the features of pain and providing an objective and reproducible assessment of pain facial expression for each individual. Further, the ePAT app automatically calculates a pain severity score once the user enters other non-facial pain cues observed in the person.
The strong correlation demonstrated between ePAT and APS in this study is very encouraging. According to Herr et al. [19], an acceptable correlation coefficient for a new pain assessment tool is 0.4–0.6, whereas ePAT achieved a correlation coefficient was ≥ 0.88 when compared with the APS. By way of comparison, Lichtner et al., in their systematic review, reported on the outcomes of concurrent validity assessments in which the scores of one tool were compared with those of another, or with healthcare professional ratings of pain or with self-reports (using VAS scales) [26]. The results obtained from this study are generally better than those reported from other head to head comparisons of behavioral (observational) pain scales, or when such tools’ scores are compared with observer pain ratings or self-reports [26].
In regards to the discriminant validity, it should be noted that pain scores associated with movement were higher than those at rest in the same individual, when assessed using either the APS or ePAT. Statistical analysis of the effect of timing of assessment (i.e., whether the assessment was undertaken at rest or on movement) demonstrated no difference between the two pain assessment tools. This means that whether the measurements were taken at rest or with movement had no influence on the relationship between the ePAT and APS scores. Given that the APS is one of a number of behavioral pain scales which have been shown to demonstrate significant differences in scores pre- and post-interventions/events (e.g., movement) [26], this suggests that ePAT is also able to discriminate between pain at rest and pain on movement as non-facial items of both tools share same conceptual foundation (i.e., AGS). Other tools that have proven discriminant validity include the Certified Nursing Assistant Pain Assessment Tool (CPAT), Checklist of Nonverbal Pain Indicator (CNPI), Discomfort Scale-Dementia of Alzheimer Type (DS-DAT), Pain Assessment Checklist for Seniors with Limited Ability to Communicate (PACSLAC), Mobilization – Observation – Behavior – Intensity – Dementia Pain Scale (MOBID), Assessment of Discomfort in Dementia (ADD), and the Behavior Checklist [26]. In the future, it will be important to assess whether ePAT can detect changes in individuals’ pain scores post-intervention, both pharmacological and non-pharmacological.
A test cannot be valid if it is not reliable, i.e., the assessment tool must produce stable and consistent results. In this study, ePAT demonstrated good inter-rater reliability in comparison to APS when results for each instrument were categorized as representing mild, moderate, or severe pain with weighted kappa scores >0.6. The inter-rater reliability of the APS was found to be moderately good (0.335–0.475) when tested by 26 nurses in 126 residents in a study by Neville and Ostini in 2014 [32]. By comparison the Pain Assessment in Advanced Dementia Scale (PAINAD), for example, has also shown similar inter-rater reliability when compared head to head with CNPI in the range of 0.31 at rest and 0.54 during movement [33]. Lints-Martindale et al. examined the inter-rater agreement (Cohen’s kappa) for six observation pain tools; namely ADD, Nursing Assistant-Administered Instrument to Assess Pain in Demented Individuals (NOPAIN), Pain Assessment for the Dementing Elderly (PADE), PACSLAC, PAINAD, and CNPI. Employing an influenza vaccination as the painful stimulus, they found that agreement between the tools ranged from substantial (i.e., κ= 0.61 to 0.80) to high levels of agreement (i.e., κ= 0.81 to 1.0) [34]. The highest level of agreement was obtained between the PACSLAC and the CNPI. Interestingly, like the PACSLAC, the scoring for the CNPI is binary. Assessors assign 1 if a behavior is present and 0 if it is absent. As there are six categories (namely: non-verbal vocalizations [e.g., moans, groans], facial grimaces/winces, bracing [e.g., clutching or holding onto side rails], restlessness, rubbing, and verbal vocal complaints [e.g., “ouch”, “that hurts”], pain is scored on a scale of 0 to 6 [35]. PACSLAC utilizes a series of 60 questions across four categories (namely: facial expressions, activity/body movements, social/personality/mood indicators) with a range of 0 to 60 [36]. Other tools such as PAINAD and APS require the assessor both to identify presence of a particular behavior (items) and rate its intensity [24, 37].
The overall internal consistency of ePAT when compared with APS was found to be excellent (α> 0.9), and in keeping with those of other observational pain tools. For example, PACSLAC (total scale) α range 0.82 to 0.87 [19, 38] and subscales α range 0.55 to 0.73; CNPI (both at rest and with movement) α= 0.54 [35], APS (total scale) α range 0.71 to 0.81 [19], PADE Part 1, Physical (e.g., facial expressions) α range 0.76 to 0.88 [39]; DOLOPLUS 2 α= 0.82 [19]; and L’ échelle Comportementale pour Personnes Agées (ECPA) α= 0.7 [19].
One major strength of this study was that it is the first study of its kind (as far as we know) to evaluate a pain assessment tool linked to automated facial recognition technology and built into a smart device for people with moderate to severe dementia. Compared to other existing tools, this offers the advantage of minimizing rater subjectivity in one of the key AGS pain assessment domains, i.e., facial expressions. Another strength is that pain scores were obtained while participants were receiving their standard care. The latter was provided during the study at all times and with minimal or no interruption. Standard care is believed to elicit nociceptive pain and also offers a real world context as encountered in the residential aged care setting, and with less potential for recall bias from raters. Residents had various types of dementias and pain diagnoses covered a wide spectrum of medical conditions. Also, pain measurements were performed on a weekly basis for a period of 13 consecutive weeks to portray a clearer clinical picture about the frequency and status of pain symptoms in these subjects.
Limitations of this psychometric evaluation include the following: (1) small sample size and non-random selection of participants; however, a point of saturation was reached with regard to correlation findings; (2) homogenous nature of the sample in terms of gender and ethnicity because of the overrepresentation of Caucasian (n = 39) females (n = 28) so that findings may be only applicable to this group; (3) unequal number of assessments per resident over the study period; (4) some participants might have exhibited little or no pain-related behaviors even in the presence of severe pain; (5) proxy reporting and recall bias are possible because a care worker could have a fallible memory and may not remember events accurately, which might affect the quality and amount of information provided (although this limitation also exists for APS); (6) these findings were observed based on clinical pain, so agreement between ePAT and APS may differ in experimentally-induced pain modalities; (7) potential for judgement subjectivity and interpretation bias when scoring non-facial domains on ePAT (minimized by employing a binary assessment) and all domains of the APS which uses an ordinal scale; (8) despite using the APS in the current study, there is currently no globally accepted gold standard observational pain scale; and (9) some pain behavioral cues are difficult to interpret and they could be identified by raters as pain where they actually related to other signs of mental disorders such as depression. Our study design is observational in nature where no intervention (e.g., analgesics) is given to subjects. Hence unless an adequately powered, tightly controlled clinical trial is employed with an intervention targeted towards these behavioral problems, the confounding effect is inevitable to occur. Rater-related limitations also include the fact that only a small number of raters completed the assessments, and there were a number of novice raters. The impact of the latter was evaluated by comparing the results with and without the inclusion of their assessments, this had a negligible impact on the results. Although it is also desirable to conduct an additional multivariate analysis, we consider the current analysis of variables provides sufficient information to meet the objectives of the study.
This study demonstrates that ePAT has psychometric properties which make it suitable for use in people with moderate to severe dementia. It has proven validity and reliability compared to APS, which is the current gold standard for pain assessment in people with dementia who cannot self-report pain in Australia. We believe it offers a significant advantage in that the facial expression assessment is automated, providing an objective and reproducible evidence of the presence of pain, in conjunction with non-facial features. Further, the non-facial domain items, which have been specifically geared towards older people with dementia, only require Yes/No responses, rather than judgements about their presence and intensity, providing objective assessment and a point for future reference. The fact that the tool is integrated into a mobile application, which can store repeat pain assessments for individuals, is highly advantageous as it facilitates ongoing monitoring of patients’ pain and the effectiveness of their management. Lastly, it has been designed for use by healthcare professionals and lay carers alike.
ACKNOWLEDGMENTS
The project described was supported by grant funding and stipend scholarship from Alzheimer’s Australia. The content is solely the responsibility of the authors and does not necessarily represent the official views of Alzheimer’s Australia Dementia Research Foundation. The authors want to thank aged care staff, residents, and their families for their involvement in the project.
Authors’ disclosures available online (http://j-alz.com/manuscript-disclosures/17-0375r1).
REFERENCES
[1] | AGS Panel on Persistent Pain in Older Persons ((2002) ) The management of persistent pain in older persons. J Am Geriatr Soc 50: , S205–S224. |
[2] | Achterberg WP , Pieper MJC , van Dalen-Kok AH , de Waal MWM , Husebo BS , Lautenbacher S , Kunz M , Scherder EJA , Corbett A ((2013) ) Pain management in patients with dementia. Clin Interv Aging 8: , 1471–1482. |
[3] | Maxwell CJ , Dalby DM , Slater M , Patten SB , Hogan DB , Eliasziw M , Hirdes JP ((2008) ) The prevalence and management of current daily pain among older home care clients. Pain 138: , 208–216. |
[4] | Cipher DJ , Clifford PA , Roper KD ((2006) ) Behavioral manifestations of pain in the demented elderly. J Am Med Dir Assoc 7: , 355–365. |
[5] | Cohen-Mansfield J , Thein K , Marx MS , Dakheel-Ali M ((2012) ) What are the barriers to performing nonpharmacological interventions for behavioral symptoms in the nursing home? J Am Med Dir Assoc 13: , 400–405. |
[6] | Castle NG , Engberg J ((2005) ) Staff turnover and quality of care in nursing homes. Medical Care 43: , 616–626. |
[7] | Zwakhalen SMG , Hamers JPH , Peijnenburg RHA , Berger MPF ((2007) ) Nursing staff knowledge and beliefs about pain in elderly nursing home residents with dementia. Pain Res Manag 12: , 177–184. |
[8] | Sheu E , Versloot J , Nader R , Kerr D , Craig KD ((2011) ) Pain in the elderly: Validity of facial expression components of observational measures. Clin J Pain 27: , 593–601. |
[9] | Ekman P , Friesen WV ((1978) ) Facial Action Coding System, Consulting Psychologists Press, Palo Alto, CA. |
[10] | Ekman P , Friesen WV , Hager J ((1978) ) The Facial Action Coding System (FACS): A technique for the measurement of facial action, Consulting Psychologists Press, Palo Alto, CA. |
[11] | van Herk R , van Dijk M , Baar FP , Tibboel D , de Wit R ((2007) ) Observation scales for pain assessment in older adults with cognitive impairments or communication difficulties. Nurs Res 56: , 34–43. |
[12] | Rojo R , Prados-Frutos JC , Lopez-Valverde A ((2015) ) Pain assessment using the Facial Action Coding System. A systematic review. Med Clin (Barc) 145: , 350–355. |
[13] | Kunz M , Scharmann S , Hemmeter U , Schepelmann K , Lautenbacher S ((2007) ) The facial expression of pain in patients with dementia. Pain 133: , 221–228. |
[14] | Hadjistavropoulos T , LaChapelle DL , MacLeod FK , Snider B , Craig KD ((2000) ) Measuring movement-exacerbated pain in cognitively impaired frail elders. Clin J Pain 16: , 54–63. |
[15] | Kunz M , Mylius V , Schepelmann K , Lautenbacher S ((2009) ) Effects of age and mild cognitive impairment on the pain response system. Gerontology 55: , 674–682. |
[16] | Kunz M , Rainville P , Lautenbacher S ((2011) ) Operant conditioning of facial displays of pain. Psychosom Med 73: , 422–431. |
[17] | Hughes J , Hoti K , Atee MAW , Inventors ((2016) ) Electronic Pain Assessment Technologies (ePAT) Pty Ltd, assignee. A pain assessment method and system, World Intellectual Property Organization (WIPO), Patent 025989, 2016-02-25. |
[18] | Australian Pain Society ((2005) ) Pain in Residential Aged Care Facilities: Management Strategies, Australian Pain Society, North Sydney. |
[19] | Herr K , Bjoro K , Decker S ((2006) ) Tools for assessment of pain in nonverbal older adults with dementia: A state-of-the-science review. J Pain Symptom Manage 31: , 170–192. |
[20] | Zwakhalen SMG , Hamers JPH , Abu-Saad HH , Berger MPF ((2006) ) Pain in elderly people with severe dementia: A systematic review of behavioural pain assessment tools. BMC Geriatr 6: , 3. |
[21] | Park J , Castellanos-Brown K , Belcher J ((2010) ) A review of observational pain scales in nonverbal elderly with cognitive impairments. Res Social Work Prac 20: , 651–664. |
[22] | Qi S , Diane J , Kay D ((2012) ) The psychometric properties, feasibility and utility of behavioural-observation methods in pain assessment of cognitively impaired elderly people in acute and long-term care: A systematic review. JBI Libr Syst Rev 10: , 977–1085. |
[23] | Corbett A , Husebo B , Malcangio M , Staniland A , Cohen-Mansfield J , Aarsland D , Ballard C ((2012) ) Assessment and treatment of pain in people with dementia. Nat Rev Neurol 8: , 264–274. |
[24] | Abbey J , Piller N , De Bellis A , Esterman A , Parker D , Giles L , Lowcay B ((2004) ) The Abbey pain scale: A 1-minute numerical indicator for people with end-stage dementia. Int J Palliat Nurs 10: , 6–13. |
[25] | Merskey H , Bogduk N ((1994) ) IASP Task Force on Taxonomy: Classification of chronic pain: Description of chronic pain syndromes and definition of pain terms, IASP Press, Seattle. |
[26] | Lichtner V , Dowding D , Esterhuizen P , Closs SJ , Long AF , Corbett A , Briggs M ((2014) ) Pain assessment for people with dementia: A systematic review of systematic reviews of pain assessment tools. BMC Geriatr 14: , 138. |
[27] | Hadjistavropoulos T , Herr K , Prkachin KM , Craig KD , Gibson SJ , Lukas A , Smith JH ((2014) ) Pain assessment in elderly adults with dementia. Lancet Neurol 13: , 1216–1227. |
[28] | Lam M , Webb KA , O’Donnell DE ((1999) ) Correlation between two variables in repeated measures. In American Statistical Association, Proceedings of the Biometric Section; 1999 213-18; Alexandria, Virginia, pp. 213–218. |
[29] | Hamlett A , Ryan L , Wolfinger R ((2004) ) On the use of PROC MIXED to estimate correlation in the presence of repeated measures. SAS Users Group International, Proceedings of the Statistics and Data Analysis Section SAS Institute Inc., pp. 1–7. |
[30] | Portney L , Mary P ((2009) ) Foundations of clinical research: Applications to practice, Pearson/Prentice Hall, Upper Saddle River, NJ. |
[31] | Viera AJ , Garrett JM ((2005) ) Understanding interobserver agreement: The kappa statistic. Fam Med 37: , 360–363. |
[32] | Neville C , Ostini R ((2014) ) A psychometric evaluation of three pain rating scales for people with moderate to severe dementia. Pain Manag Nurs 15: , 798–806. |
[33] | Ersek M , Herr K , Neradilek MB , Buck HG , Black B ((2010) ) Comparing the psychometric properties of the Checklist of Nonverbal Pain Behaviors (CNPI) and the Pain Assessment in Advanced Dementia (PAIN-AD) instruments. Pain Med 11: , 395–404. |
[34] | Lints-Martindale AC , Hadjistavropoulos T , Lix LM , Thorpe L ((2012) ) A comparative investigation of observational pain assessment tools for older adults with dementia. Clin J Pain 28: , 226–237. |
[35] | Feldt KS ((2000) ) The checklist of nonverbal pain indicators (CNPI). Pain Manag Nurs 1: , 13–21. |
[36] | Fuchs-Lacelle S , Hadjistavropoulos T ((2004) ) Development and preliminary validation of the pain assessment checklist for seniors with limited ability to communicate (PACSLAC). Pain Manag Nurs 5: , 37–49. |
[37] | Warden V , Hurley AC , Volicer L ((2003) ) Development and psychometric evaluation of the Pain Assessment in Advanced Dementia (PAINAD) scale. J Am Med Dir Assoc 4: , 9–15. |
[38] | Fuchs-Lacelle S , Hadjistavropoulos T ((2005) ) Inter-rater reliability and additional psychometric information on the pain assessment checklist for seniors with limited ability to communicate (PACSLAC). In IASP Poster presented at the 2005 World Congress on Pain, Sydney, Australia. |
[39] | Villanueva MR , Smith TL , Erickson JS , Lee AC , Singer CM ((2003) ) Pain Assessment for the Dementing Elderly (PADE): Reliability and validity of a new measure. J Am Med Dir Assoc 4: , 1–8. |