Development of a Proxy-Free Objective Assessment Tool of Instrumental Activities of Daily Living in Mild Cognitive Impairment Using Smart Home Technologies
Abstract
Background: The assessment of activities of daily living (ADL) is essential for dementia diagnostics. Even in mild cognitive impairment (MCI), subtle deficits in instrumental ADL (IADL) may occur and signal a higher risk of conversion to dementia. Thus, sensitive and reliable ADL assessment tools are important. Smart homes equipped with sensor technology and video cameras may provide a proxy-free assessment tool for the detection of IADL deficits.
Objective:The aim of this paper is to investigate the potential of a smart home environment for the assessment of IADL in MCI.
Method: The smart home consisted of a two-room flat equipped with activity sensors and video cameras. Participants with either MCI or healthy controls (HC) had to solve a standardized set of six tasks, e.g., meal preparation, telephone use, and finding objects in the flat.
Results: MCI participants needed more time (1384 versus 938 seconds, p < 0.001) and scored less total points (48 versus 57 points, p < 0.001) while solving the tasks than HC. Analyzing the subtasks, intergroup differences were observed for making a phone call, operating the television, and retrieving objects. MCI participants showed more searching and task-irrelevant behavior than HC. Task performance was correlated with cognitive status and IADL questionnaires but not with participants’ age.
Conclusion: This pilot study showed that smart home technologies offer the chance for an objective and ecologically valid assessment of IADL. It can be analyzed not only whether a task is successfully completed but also how it is completed. Future studies should concentrate on the development of automated detection of IADL deficits.
INTRODUCTION
For the definition of dementia, impairment in activities of daily living (ADL) is one of the key clinical criteria [1]. The assessment of ADL functioning is also important for patients suffering from mild cognitive impairment (MCI), as MCI patients with deficits in complex ADL functioning have a higher risk of conversion to dementia than MCI patients without ADL deficits [2, 3]. In MCI, basic ADL functioning like eating or walking is preserved, but instrumental ADL (IADL) are often impaired. IADL requiring higher neuropsychological processes, like financial capacities, telephone use, and finding things at home, are most at risk of decline [4]. Investigating functional and cognitive abilities in MCI participants, Bangen et al. found an association between IADL deficits and global cognitive functioning [5]. A study by Farias et al. revealed impairment of IADL domains that depend heavily on memory function [6].
In clinical practice, ADL functioning is usually assessed by informant-report measures like interviews or standardized questionnaires; less common is the use of self-report or direct performance-based measures. All of these measures have specific advantages and disadvantages: Informant-report allows a quick evaluation of a broad range of everyday competences; however, a reliable proxy is not always available and evaluation can be prone to judgment biases [7, 8]. Self-report also allows a quick evaluation of ADL functioning, however, patients with dementia lack awareness of their problems. In MCI, there are inconsistent results regarding patients’ self-assessment: Some studies report preserved [9, 10], others reduced awareness of deficits [11, 12]. Using performance-based measures, a proxy is not necessary and judgment bias is a minor problem. Examples of existing performance-based measures are the “Revised Observed Tasks of Daily Living” [13], which assesses nine different IADL domains and has good psychometric properties or the “Financial Capacity Instrument” [14], which thoroughly analyzes financial abilities in seven domains. However, existing performance-based assessments are often time-consuming and mostly evaluate only a small range of ADL functioning, e.g., financial capacity [14]. Furthermore, the testing environment is quite artificial, which enhances internal validity but reduces ecological validity. A possible solution to this problem could be provided by smart home environments. A smart home is defined as ‘a physical world that is richly and invisibly interwoven with sensors, actuators, displays, and computational elements, embedded seamlessly in the everyday objects of our lives, and connected through a continuous network’ [15]. Smart homes have initially been developed to support people in their everyday life, monitor their health status, or detect falls. Research concentrated on activity recognition [16], detection of emergency situations, or automation of processes [17]. The diagnostic value of smart homes, however, has only been recognized by few researchers so far who focus on the automated assessment of cognitive health [18]. Another stream of research concentrates on the development of fully automated video analyses to detect deficits in everyday functioning [19–21], however, they do not apply any additional sensor technology.
As smart homes are equipped with advanced technological devices and video cameras, those settings could be used to evaluate a person’s IADL functioning. The advantage of a smart home is that it offers a controllable, yet ecologically valid testing environment. Furthermore, rater bias could be thoroughly eliminated, as the technology offers the opportunity for a fully automated assessment, given the right algorithms. The aim of this study is to investigate the potential of smart home technologies for IADL assessment in MCI. Video analyses and sensor-based data will be recorded to find differences in IADL performance between patients with mild cognitive impairment and healthy controls. Furthermore, relationships between task performance and traditional ADL questionnaires will be analyzed.
METHODS
Participants
Participants aged 65 to 80 years were recruited at the memory clinic of the Central Institute of Mental Health in Mannheim, Germany. MCI was determined using the Petersen clinical criteria [22]. Healthy controls were age- and gender-matched. All participants underwent a neuropsychological assessment to evaluate cognitive functioning. The assessment consisted of the CERAD-plus test battery [23], the Logical Memory task from the Wechsler-Memory Scale [24], the Clock Drawing Test [25], and the Mini-Mental State Examination (MMSE) [26]. For the MCI group, participants had to be impaired in at least one cognitive domain (i.e., 1.5 SD below age- and education adjusted norms) and the MMSE had to be higher than 25 points. For the HC group, no cognitive deficits (i.e., all tests within age- and education-adjusted norms) were allowed. Patients with aberrant motor activity, or psychotic or major depressive disorder according to the Diagnostic and Statistical Manual of Mental Disorders (DSM-IV-TR) [27] were not included. For MCI participants, brain MRI scans were obtained via a 3.0-T MR system (Magnetom Trio, Siemens Medical Systems, Erlangen, Germany). MRI data were analyzed by experienced neuroradiologists using qualitative rating scales. Mediotemporal atrophy was assessed via the Scheltens’ visual rating scale [28].
To control for depressive symptoms, the Geriatric Depression Scale (GDS) [29] was administered to all potential participants. GDS scores of 6 and higher were an exclusion criterion. For the assessment of ADL functioning, participants’ proxies completed the Barthel-ADL [30], the Bayer-ADL [31], and the ADCS-MCI-ADL-18 [32]. The Barthel-ADL assesses basic ADL functioning via 10 items, which include, among others, mobility, eating and continence. Maximum score is 100 points and indicates perfect ADL functioning. The Bayer-ADL consists of 25 items assessing basic and instrumental ADL functioning. Each item is rated on a 10-point scale, ranging from 1 (has never problems) to 10 (has always problems). All answered items are summed up and divided by the number of answered items so that total scores range from 1 to 10. The ADCS-MCI-ADL consists of 18 items that mainly assesses IADL functioning. The maximum score is 57 points and indicates completely intact ADL functioning.
The study was approved by the ethics committee of Heidelberg University, Germany. Informed consent was obtained from all participants and their proxies prior to enrolment.
IADL assessment in the smart home environment
The IADL assessment was conducted at the Fraunhofer Institute in Kaiserslautern, Germany. The smart home environment consisted of a 60 square meters two-room flat (see Fig. 1) equipped with (hidden) activity sensors and video cameras in every room.
The flat was fully furnished and equipped with everyday objects like a television, book shelves, a kettle, armchairs, and a telephone (see Fig. 2). Participants were told to imagine they moved into a holiday apartment and had to solve six tasks. Before the tasks started, participants had a 5-min exploration phase to get familiar with the environment. The six tasks (see Fig. 3) were standardized and participants were handed over an instruction sheet for each task.
Participants were instructed to do each task as quickly and accurately as they could. After task completion participants were instructed to exit the apartment via the hallway door to get instructions for the next task.
Assessed parameters in the smart home environment
Task performance, i.e., IADL functioning, was assessed via different parameters. First of all, time to solve the single tasks was recorded via the activity sensors (when did participant start/stop the specific activity). Furthermore, the video material was analyzed to assess qualitative task performance. Two independent raters evaluated the different steps of task completion, interrater agreement was 98%. For example, task 4 (preparing a sandwich) consisted of 9 steps: 1) go into the kitchen, 2) get the bread, 3) toast the bread, 4) get a plate, 5) get a knife, 6) get the jam, 7) get the butter, 8) spread the toasted bread with jam and butter, 9) put the plate with the bread on the table. Correctly performed steps were evaluated with 1 point each, i.e., in task 4, a maximum of 9 points could be achieved. The 9 steps outlined above were mandatory to solve task 4 successfully and get the full score. However, to be scored as correct, the single steps to complete a task did not have to follow a specific order. This scoring procedure was chosen to enhance ecological validity, as there is a lot of individual variability in solving the tasks correctly. For all tasks together, the maximum score was 60 points.
Furthermore, task irrelevant behavior (e.g., stirring prepared cup of coffee while solving the television task) and searching behavior (e.g., opening cupboards to find a plate) were documented.
Feasibility questionnaire
After completion of all tasks, a semi-structured interview was conducted with each participant to evaluate feasibility of the IADL assessment in the smart home environment. The interview consisted of 14 questions, 9 of them had answer categories with a 5-point Likert Scale. Participants were asked whether the flat was “natural”, the tasks resembled everyday tasks, the tasks were easy to understand, or whether they felt uncomfortable at any time. Furthermore, they had to indicate things that could be improved and tasks they experience as difficult in their everyday life.
Statistical analyses
As data did not follow a normal distribution, non-parametric tests were applied for data analysis. For group comparisons, the Kolmogorov-Smirnov-Z test was applied, since it is recommended for small sample sizes and independent variables with few categories. Furthermore, it provided more conservative results than the Mann-Whitney-U test. For correlation analyses, Kendall’s tau (τ) was used, as it is recommended for small sample sizes with tied ranks [33]. Chi-square tests were applied for comparisons of categorical variables. Significance level for all analyses was set to α= 0.05. All analyses were performed with SPSS 20.0.
RESULTS
Sample characteristics
The sample consisted of 11 MCI patients (mean age = 74.6 years, SD = 4.9) and 10 HC (mean age = 73.4 years, SD = 4.4). Clinical and demographic data of the sample are depicted in Table 1. The majority of participants was female (MCI 73%, HC 70%). As groups were matched for age and gender, no group differences were observed for these variables. Furthermore, no group differences emerged for years of education, GDS score, and the Barthel-ADL. Significant intergroup differences were found for the MMSE score (MCI: M = 27.5 points, SD = 1.0; HC: M = 29.6 points, SD = 0.5), the CERAD subtests, the Bayer-ADL score (MCI: M = 2.9, SD = 1.0; HC: M = 1.3, SD = 0.4) and the ADCS-MCI-ADL score (MCI: M = 45.4, SD = 4.4; HC: M = 54.1, SD = 2.8). For MCI participants, structural neuroimaging data, i.e., brain MRI scans, were available: 9 of 11 MCI participants (82%) showed clinically significant mediotemporal atrophy.
Performance in the smart home environment
Significant intergroup differences were observed for the performance of the IADL tasks in the smart home environment (see Table 2 for details). The MCI group needed more time to complete the six tasks than the HC group (1384 versus 938 seconds, p < 0.001). Looking at the single tasks, the MCI group needed more time than the HC group to complete task 1 ‘placing objects’, task 3 ‘making a phone call’, task 5 ‘operating the TV’, and task 6 ‘retrieving the objects’. No intergroup differences regarding time emerged for task 2 ‘making coffee’ and task 4 ‘preparing a sandwich’. In terms of total points, i.e., correctly performed steps to solve all tasks, the MCI group differed significantly from the HC group (48 points versus 57 points, p < 0.001). Looking at the single tasks, the MCI group obtained significantly fewer points than the HC group in task 3 ‘making a phone call’, task 5 ‘operating the TV’ and task 6 ‘retrieving the objects’. Furthermore, the MCI group showed more searching and task-irrelevant behavior than the HC group.
Correlation analyses
Correlation analyses revealed that neither IADL performance in the smart home nor ADL questionnaire scores were correlated with participants’ age (see Table 3 for details).
Total time to perform the six tasks was significantly negatively correlated with the MMSE scores, τ= –0.64, p < 0.01. Total points were significantly positively correlated with the MMSE scores, τ= 0.85, p < 0.01. Furthermore, total time was negatively correlated with total points, τ= –0.49, p < 0.01. Looking at the ADL questionnaires, significant correlations for both the Bayer-ADL and the ADCS-MCI-ADL with total time to solve the tasks and total points emerged. Regarding single items, the item ‘telephone use’ of the Bayer-ADL was significantly correlated with task 3 ‘making a phone call’, both for time (τ= 0.43, p < 0.01), total points (τ= –0.52, p < 0.01) and number of dial attempts (τ= 0.47, p < 0.01). Comparable results were observed for the corresponding item of the ADCS-MCI-ADL. The item ‘finding objects at home’ of the ADCS-MCI-ADL was correlated with the time to complete task 6 ‘retrieving the objects’ (τ= –0.35, p < 0.05), task 6 total points (τ= 0.63, p < 0.01), and the number of retrieved objects in task 6 (τ= 0.60, p < 0.01).
Table 4 depicts correlations between selected CERAD-subtests and completion time of the single tasks. Generally, all reported correlations are negative: The smaller the z-score in the CERAD-subtests (indicating worse performance), the more time was needed for task completion. Task 3 ‘making a phone call’ and task 6 ‘retrieving the objects’ were significantly correlated with all CERAD-subtests. The fewest correlations were found for task 2 ‘making coffee’ which showed moderate correlations with Trail Making Test A and B. Completion time of each of the 6 tasks was moderately correlated with Trail Making Test B.
Feasibility
The participants evaluated the smart home environment and the tasks as realistic (4.24 points, SD = 0.99, on a 5-point Likert scale, and 4.81 points, SD = 0.41, respectively). Moreover, they did not feel uncomfortable while solving the tasks (M = 1.2 points, SD = 0.5) or experienced the scenario as too long (M = 1.1 points, SD = 0.3). 52.4% evaluated task 6 ‘retrieving the objects’ as the most difficult task, 14.3% considered task 3 ‘making a phone call’ the most difficult.
DISCUSSION
The present study investigated the potential of smart home technologies to assess IADL in MCI. Results show that the new assessment tool detected differences between MCI participants and healthy controls. First of all, MCI participants needed more time to complete the tasks than healthy controls. This is in line with findings of Wadley et al. who observed reduced speed in MCI participants while solving IADL-related tasks [34]. Analyzing the subtasks in our sample, significant intergroup differences were observed for placing and retrieving objects, making a phone call, and operating the TV, whereas no differences regarding completion time emerged for making coffee and preparing a sandwich. The latter two tasks could be considered as not highly cognitive demanding: While they required the use of electronic devices (i.e., kettle and toaster, respectively) the participant only had to operate the on/off switch, not multiple steps as in the TV or telephone tasks. Research on IADL in MCI mainly comes to the conclusion that IADL requiring complex neuropsychological processes, e.g., financial capacity or operating technological devices, are affected early in the course of the disease [4, 35]. Reppermund et al. conducted a factor analysis by which they subdivided the Bayer-ADL items in items with high or low cognitive demand. Only the high cognitive demand items (e.g., observing important dates, doing two things at a time) reliably differentiated MCI patients from healthy controls [36].
In the present study, MCI participants not only needed more time to complete tasks, but also made more errors (i.e., scored fewer total points) than healthy controls. Looking at the subtasks, differences were observed for using the telephone, operating the TV, and retrieving the objects. This means that qualitative task performance only differed in three of six tasks, partially supporting the findings of Wadley et al. who evidenced reduced speed in MCI patients but qualitatively intact IADL functioning [34]. Investigating IADL performance in a naturalistic setting, Seelye et al. also observed that MCI participants made more errors than healthy controls while solving tasks but profited from indirect prompting [37].
Correlation analyses in the present study revealed moderate to strong relationships between “traditional” ADL questionnaires and the IADL assessment in the smart home environment. This underlines the usefulness of the newly developed method, as it reflects proxies’ evaluation. As the study aimed to develop a “proxy-free” assessment, the significant correlations are promising. Furthermore, the Trail Making Test B (a measure of executive functioning) was significantly correlated with completion time in each of the 6 tasks. This underlines the importance of intact executive functioning for task completion. Another interesting finding is that tasks 3 and 6, which were experienced as most difficult by participants, were significantly correlated with performance in all CERAD-subtests.
One major shortcoming of the present study is the small sample size. This is due to the fact that IADL evaluation in smart homes is an innovative field of research and cost-intensive. To some extent, logistic reasons were also responsible for the small sample size as participants had to be transported to the testing site which had the monitoring technology installed. However, the sample was very carefully chosen: MCI patients were matched to the healthy controls regarding age and gender. Only participants without any mobility constraints or major depression were included. The majority of MCI patients had clinically significant mediotemporal atrophy indicating underlying AD pathology. Moreover, MCI participants had an average MMSE of 27.5 points, which is quite high compared to other studies investigating intergroup differences between MCI and healthy controls (see [4] for an overview). Nevertheless, significant IADL deficits were observed for the MCI group. The biggest strength of the study can be seen in the ecologically valid IADL assessment. While performance-based assessments are most often conducted in quite artificial laboratory settings, our study provided participants with a fully furnished two-room flat. Thus, participants were able to profit from environmental cues which facilitate ADL performance. To make the setting even more naturalistic, future studies could incorporate distracters (e.g., a second person) and interruptions (e.g., a ringing phone which has to be answered) or tasks requiring multi-tasking abilities (e.g., listening to the radio and remembering the songs while making a sandwich).
By means of the sensor data and video recording, it was possible to unobtrusively observe and analyze how participants solved the tasks. Interestingly, in tasks 2 (making coffee) and 4 (preparing a sandwich), no significant intergroup differences emerged; however, MCI patients showed more searching and task-irrelevant behavior while solving the tasks. This could be a first indicator for cognitive decline and important for early detection of MCI. The chance to monitor how participants solve IADL-related tasks is an important advantage of smart home environments. However, in clinical routine settings, traditional IADL questionnaires are—at least for the moment—the method of choice, as they are inexpensive and do not involve logistic challenges.
Another shortcoming of the study is that it partially relied on an observer-rating of IADL performance. After successful pilot testing, the development of fully automated video and sensor data analyses should be intensified. Research groups already showed that automatic video monitoring systems can successfully detect differences in ADL performance (see [19]). Future research should concentrate on the validation of the tasks in a bigger sample and promote a fully-automated IADL assessment via sensors and video recordings. Besides, longitudinal studies exploring IADL performance of community-dwelling elders at baseline and their risk of conversion to dementia in the following years would be of interest. A first step into this direction has already been taken by Kaye et al. who unobtrusively collected in-home activity data in the homes of 265 elderly participants. The authors intend to use their assessment technology for the detection of incident cognitive and functional decline [38]. Similarly, it seems possible to implement the smart home technologies of our study at care facilities to identify IADL deficits and provide individual assistance.
CONCLUSIONS
In sum, this study demonstrates the big potential of smart home technologies for the assessment of IADL functioning. Smart homes offer an ecologically valid environment, in which–via sensor-based technology in combination with video recording–more information about a patient’s IADL can be gathered than via questionnaires. Future research should be conducted with a larger sample to validate tasks and concentrate on a fully-automated assessment of IADL functioning.
ACKNOWLEDGMENTS
KJ received a scholarship from the Robert-Bosch Stiftung, Germany.
Authors’ disclosures available online (http://j-alz.com/manuscript-disclosures/15-1054r2).
REFERENCES
[1] | McKhann GM , Knopman DS , Chertkow H , Hyman BT , Jack CR Jr , Kawas CH , Klunk WE , Koroshetz WJ , Manly JJ , Mayeux R , Mohs RC , Morris JC , Rossor MN , Scheltens P , Carrillo MC , Thies B , Weintraub S , Phelps CH ((2011) ) The diagnosis of dementia due to Alzheimer’s disease: Recommendations from the National Institute on Aging-Alzheimer’s Association workgroups on diagnostic guidelines for Alzheimer’s disease. Alzheimers Dement 7: , 263–269. |
[2] | Peres K , Chrysostome V , Fabrigoule C , Orgogozo JM , Dartigues JF , Barberger-Gateau P ((2006) ) Restriction in complex activities of daily living in MCI: Impact on outcome. Neurology 67: , 461–466. |
[3] | Triebel KL , Martin R , Griffith HR , Marceaux J , Okonkwo OC , Harrell L , Clark D , Brockington J , Bartolucci A , Marson DC ((2009) ) Declining financial capacity in mild cognitive impairment: A 1-year longitudinal study. Neurology 73: , 928–934. |
[4] | Jekel K , Damian M , Wattmo C , Hausner L , Bullock R , Connelly P , Dubois B , Eriksdotter M , Ewers M , Graessel E , Kramberger M , Law E , Mecocci P , Molinuevo J , Nygard L , Olde-Rikkert M , Orgogozo J-M , Pasquier F , Peres K , Salmon E , Sikkes S , Sobow T , Spiegel R , Tsolaki M , Winblad B , Frolich L ((2015) ) Mild cognitive impairment and deficits in instrumental activities of daily living: A systematic review. Alzheimers Res Ther 7: , 17. |
[5] | Bangen KJ , Jak AJ , Schiehser DM , Delano-Wood L , Tuminello E , Han SD , Delis DC , Bondi MW ((2010) ) Complex activities of daily living vary by mild cognitive impairment subtype. J Int Neuropsychol Soc 16: , 630–639. |
[6] | Farias ST , Mungas D , Reed BR , Harvey D , Cahn-Weiner D , DeCarli C ((2006) ) MCI is associated with deficits in everyday functioning. Alzheimer Dis Assoc Disord 20: , 217–223. |
[7] | DeBettignies BH , Mahurin RK , Pirozzolo FJ ((1990) ) Insight for impairment in independent living skills in Alzheimer’s disease and multi-infarct dementia. J Clin Exp Neuropsychol 12: , 355–363. |
[8] | Zanetti O , Geroldi C , Frisoni GB , Bianchetti A , Trabucchi M ((1999) ) Contrasting results between caregiver’s report and direct assessment of activities of daily living in patients affected by mild and very mild dementia: The contribution of the caregiver’s personal characteristics. J Am Geriatr Soc 47: , 196–202. |
[9] | Farias ST , Mungas D , Jagust W ((2005) ) Degree of discrepancy between self and other-reported everyday functioning by cognitive status: Dementia, mild cognitive impairment, and healthy elders. Int J Geriatr Psychiatry 20: , 827–834. |
[10] | Okonkwo OC ((2009) ) ProQuest Information & Learning, US. |
[11] | Albert SM , Michaels K , Padilla M , Pelton G , Bell K , Marder K , Stern Y , Devanand DP ((1999) ) Functional significance of mild cognitive impairment in elderly patients without a dementia diagnosis. Am J Geriatr Psychiatry 7: , 213–220. |
[12] | Tabert MH , Albert SM , Borukhova-Milov L , Camacho Y , Pelton G , Liu X , Stern Y , Devanand DP ((2002) ) Functional deficits in patients with mild cognitive impairment: Prediction of AD. Neurology 58: , 758–764. |
[13] | Diehl M , Marsiske M , Horgas AL , Rosenberg A , Saczynski JS , Willis SL ((2005) ) The revised observed tasks of daily living: A performance-based assessment of everyday problem solving in older adults. J Appl Gerontol 24: , 211–230. |
[14] | Marson D , Moye J , Wadley V , Griffith R , Griffith H , Belue K , Sicola A , Zamrini E , Harrell L ((2002) ) Financial capacity in patients with mild cognitive impairment. Gerontologist 42: , 327–328. |
[15] | Weiser M , Gold R , Brown JS ((1999) ) The origins of ubiquitous computing research at PARC in the late 1980s. IBM Syst J 38: , 693–696. |
[16] | Roy PC , Bouzouane A , Giroux S , Bouchard B ((2011) ) Possibilistic activity recognition in smart homes for cognitively impaired people. Appl Artif Intell 25: , 883–926. |
[17] | Kim E , Helal S , Cook D ((2010) ) Human activity recognition and pattern discovery. IEEE Pervasive Comput 9: , 48. |
[18] | Dawadi PN , Cook DJ , Schmitter-Edgecombe M , Parsey C ((2013) ) Automated assessment of cognitive health using smart home technologies. Technol Health Care 21: , 323–343. |
[19] | König A , Crispim Junior CF , Derreumaux A , Bensadoun G , Petit PD , Bremond F , David R , Verhey F , Aalten P , Robert P ((2015) ) Validation of an automatic video monitoring system for the detection of instrumental activities of daily living in dementia patients. J Alzheimers Dis 44: , 675–685. |
[20] | Romdhane R , Mulin E , Derreumeaux A , Zouba N , Piano J , Lee L , Leroi I , Mallea P , David R , Thonnat M , Bremond F , Robert PH ((2012) ) Automatic video monitoring system for assessment of Alzheimer’s disease symptoms. J Nutr Health Aging 16: , 213–218. |
[21] | Sacco G , Joumier V , Darmon N , Dechamps A , Derreumaux A , Lee JH , Piano J , Bordone N , Konig A , Teboul B , David R , Guerin O , Bremond F , Robert P ((2012) ) Detection of activities of daily living impairment in Alzheimer’s disease and mild cognitive impairment using information and communication technology. Clin Interv Aging 7: , 539–549. |
[22] | Petersen RC , Smith GE , Waring SC , Ivnik RJ , Tangalos EG , Kokmen E ((1999) ) Mild cognitive impairment: Clinical characterization and outcome. Arch Neurol 56: , 303–308. |
[23] | Welsh KA , Butters N , Mohs RC , Beekly D , Edland S , Fillenbaum G , Heyman A ((1994) ) The Consortium to Establish a Registry for Alzheimer’s Disease (CERAD). Part V. A normative study of the neuropsychological battery. Neurology 44: , 609–614. |
[24] | Härting C , Markowitsch HJ , Neufeld H , Calabrese P , Deisinger K , Kessler J ((2000) ) Wechsler Memory Scale - Revised Edition, German Edition. Manual, Huber, Bern. |
[25] | Shulman KI , Shedletsky R , Silver IL ((1986) ) The challenge of time: Clock-drawing and cognitive function in the elderly. Int J Geriatr Psychiatry 1: , 135–140. |
[26] | Folstein MF , Folstein SE , McHugh PR ((1975) ) “Mini-mental state”. A practical method for grading the cognitive state of patients for the clinician. J Psychiatr Res 12: , 189–198. |
[27] | American Psychiatric Association ((2000) ), Diagnostic and statistical manual of mental disorders (4th edition, text revision - DSM-IV-TR), Washington, DC. |
[28] | Scheltens P , Leys D , Barkhof F , Huglo D , Weinstein HC , Vermersch P , Kuiper M , Steinling M , Wolters EC , Valk J ((1992) ) Atrophy of medial temporal lobes on MRI in “probable” Alzheimer’s disease and normal ageing: Diagnostic value and neuropsychological correlates. J Neurol Neurosurg Psychiatry 55: , 967–972. |
[29] | Yesavage JA , Brink TL , Rose TL , Lum O , Huang V , Adey M , Leirer VO ((1982) ) Development and validation of a geriatric depression screening scale: A preliminary report. J Psychiatr Res 17: , 37–49. |
[30] | Mahoney FI , Barthel DW ((1965) ) Functional Evaluation: The Barthel Index. Md State Med J 14: , 61–65. |
[31] | Hindmarch I , Lehfeld H , de Jongh P , Erzigkeit H ((1998) ) The Bayer Activities of Daily Living Scale (B-ADL). Dement Geriatr Cogn Disord 9: (Suppl 2), 20–26. |
[32] | Galasko D , Bennett DA , Sano M , Marson D , Kaye J , Edland SD ((2006) ) ADCS Prevention Instrument Project: Assessment of instrumental activities of daily living for community-dwelling elderly individuals in dementia prevention clinical trials. Alzheimer Dis Assoc Disord 20: , S152–S169. |
[33] | Field A ((2009) ) Discovering statistics using SPSS, Sage, London. |
[34] | Wadley VG , Okonkwo O , Crowe M , Ross-Meadows LA ((2008) ) Mild cognitive impairment and everyday function: Evidence of reduced speed in performing instrumental activities of daily living. Am J Geriatr Psychiatry 16: , 416–424. |
[35] | Nygård L ((2003) ) Instrumental activities of daily living: A stepping-stone towards Alzheimer’s disease diagnosis in subjects with mild cognitive impairment?. Acta Neurol Scand 179: , 42–46. |
[36] | Reppermund S , Sachdev PS , Crawford J , Kochan NA , Slavin MJ , Kang K , Trollor JN , Draper B , Brodaty H ((2011) ) The relationship of neuropsychological function to instrumental activities of daily living in mild cognitive impairment. Int J Geriatr Psychiatry 26: , 843–852. |
[37] | Seelye AM , Schmitter-Edgecombe M , Cook DJ , Crandall A ((2013) ) Naturalistic assessment of everyday activities and prompting technologies in mild cognitive impairment. J Int Neuropsychol Soc 19: , 442–452. |
[38] | Kaye JA , Maxwell SA , Mattek N , Hayes TL , Dodge H , Pavel M , Jimison HB , Wild K , Boise L , Zitzelberger TA ((2011) ) Intelligent systems for assessing aging changes: Home-based, unobtrusive, and continuous assessment of aging. J Gerontol B Psychol Sci Soc Sci 66B: , i180–i190. |
Figures and Tables
Fig.1
Layout of the smart home.
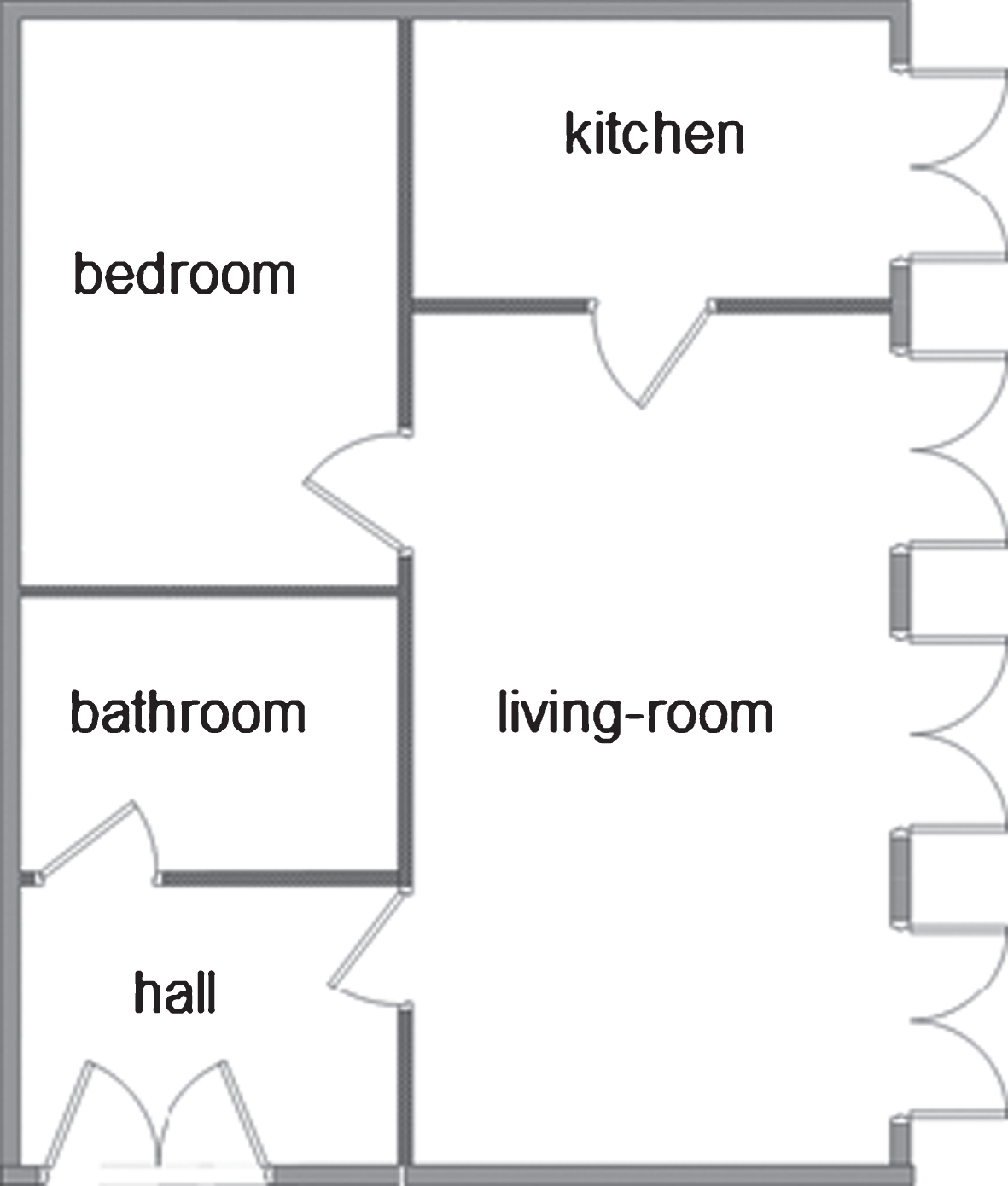
Fig.2
Living-room and kitchen of the smart home.
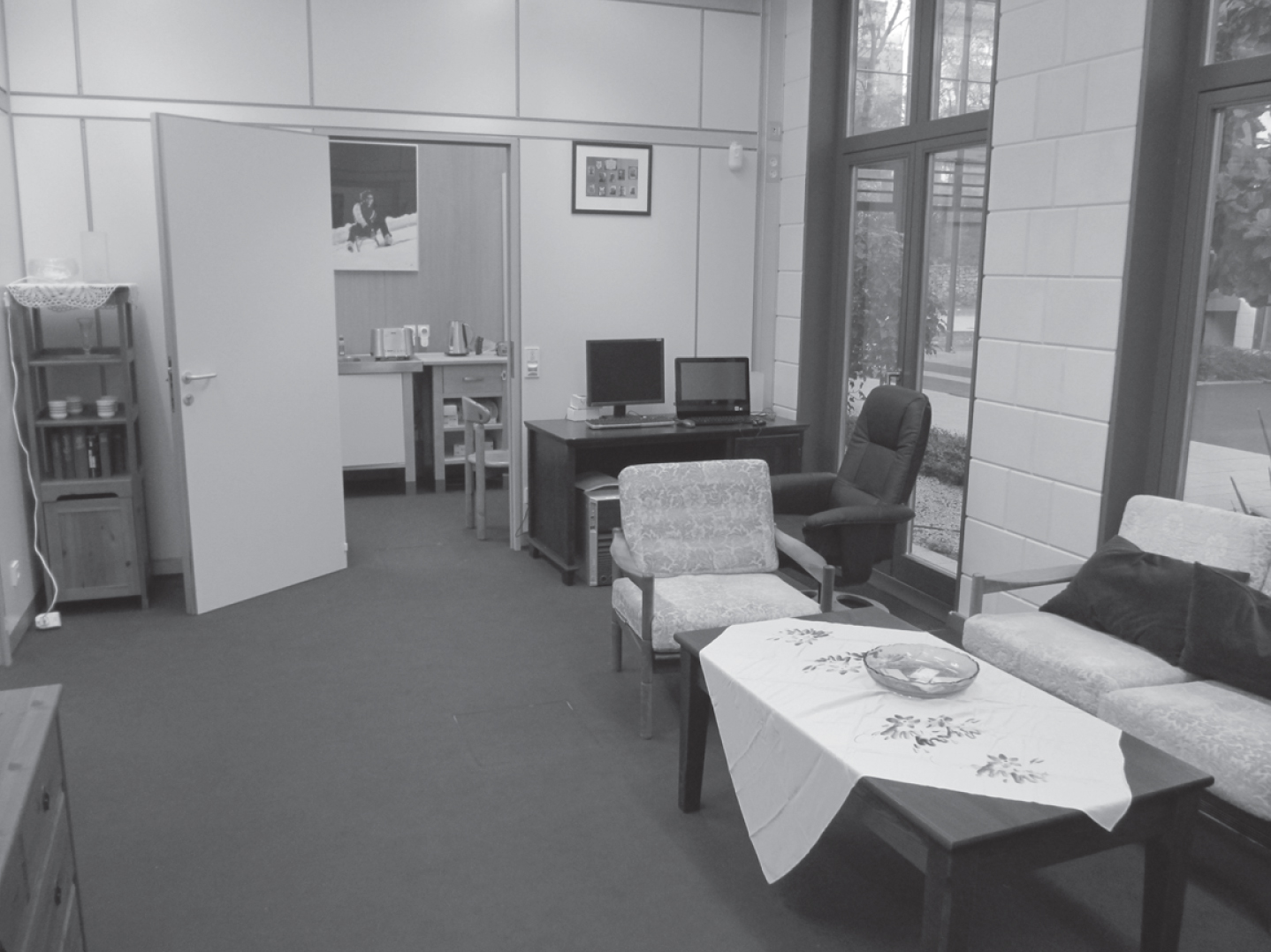
Fig.3
Tasks. Participants were instructed to.
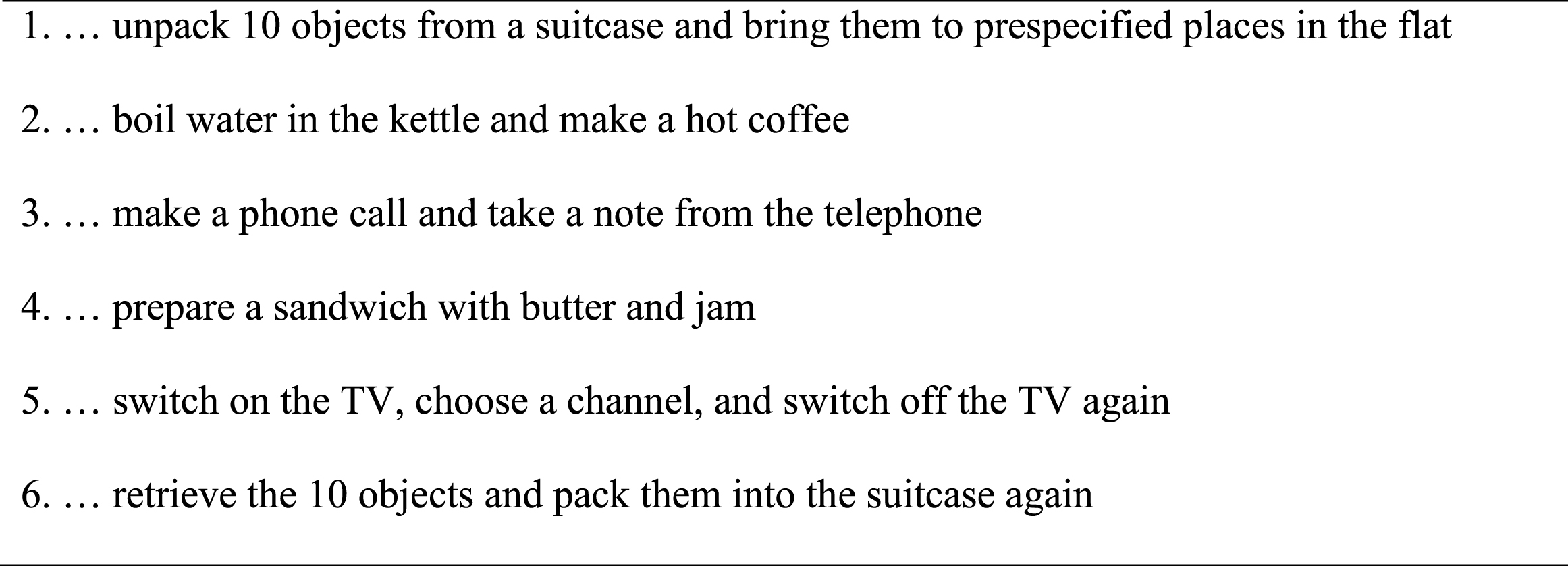
Table 1
Characteristics and group comparisons for MCI and HC
Characteristics | MCI group n = 11 | HC group n = 10 | p |
Age in years, mean (SD) | 74.6 (4.9) | 73.4 (4.4.) | 0.512 |
Female, n (%) | 8 (73%) | 7 (70%) | 0.890 |
Education, years, mean (SD) | 10.2 (3.0) | 11.5 (3.2) | 0.349 |
GDS, mean (SD) | 3.1 (1.9) | 2.7 (2.0) | 0.654 |
MMSE, mean (SD) | 27.5 (1.0) | 29.6 (0.5) | 0.001 |
Barthel-ADL, mean (SD) | 96.4 (4.5) | 97.0 (3.5) | 0.863 |
Bayer-ADL, mean (SD) | 2.9 (1.0) | 1.3 (0.4) | 0.001 |
ADCS-MCI-ADL, mean (SD) | 45.4 (4.4) | 54.1 (2.7) | 0.001 |
CERAD Word List learning, mean (SD) | –2.0 (0.6) | 0.9 (0.8) | 0.001 |
CERAD Constructional Praxis, mean (SD) | –1.0 (0.8) | 0.9 (0.8) | 0.002 |
CERAD Word List Recall, mean (SD) | –1.8 (0.3) | 0.6 (0.5) | 0.001 |
Trail Making Test A, mean (SD) | –0.8 (0.7) | 0.0 (0.4) | 0.037 |
Trail Making Test B, mean (SD) | –0.9 (0.6) | 0.1 (0.4) | 0.008 |
Mediotemporal atrophy, n (%) | 9 (82%) | – | – |
MCI, mild cognitive impairment; HC, healthy controls. Significant p-values < 0.05 are represented in bold characters. Geriatric Depression Scale (GDS) scores range from 0 to 15, with higher scores indicating depressive symptoms. Mini-Mental State Examination (MMSE) scores range from 0 to 30, with higher scores indicating better cognitive functioning. Barthel-ADL scores range from 0 to 100, with higher scores indicating better ADL functioning. Bayer-ADL scores range from 1 to 10, with higher scores indicating worse ADL functioning. ADCS-MCI-ADL scores range from 0 to 57, with higher scores indicating better ADL functioning. CERAD and Trail Making Test mean scores are age- and education adjusted z-scores.
Table 2
Comparison of IADL performance between groups
Parameters assessed | MCI group n = 11 M (SD) | HC group n = 10 M (SD) | p |
Time in seconds | |||
Total | 1384.3 (179.1) | 938.1 (88.2) | 0.000 |
Task 1 – placing objects | 309.7 (70.1) | 229.9 (58.1) | 0.029 |
Task 2 – making coffee | 194.0 (90.7) | 156.3 (38.7) | 0.493 |
Task 3 – making a phone call | 240.9 (60.3) | 126.7 (30.8) | 0.002 |
Task 4 – preparing a sandwich | 225.0 (55.7) | 175.5 (28.8) | 0.098 |
Task 5 – operating the TV | 167.0 (60.2) | 104.9 (29.1) | 0.032 |
Task 6 – retrieving objects | 247.6 (92.4) | 144.8 (46.4) | 0.041 |
Points | |||
Total | 48.0 (3.7) | 56.8 (1.7) | 0.000 |
Task 1 – placing objects | 10.8 (1.5) | 11.6 (1.0) | 0.559 |
Task 2 – making coffee | 6.9 (1.0) | 7.9 (0.3) | 0.980 |
Task 3 – making a phone call | 4.5 (1.2) | 7 (0.0) | 0.002 |
Task 4 – preparing a sandwich | 8.5 (0.8) | 8.7 (0.7) | 0.999 |
Task 5 – operating the TV | 7.7 (1.1) | 8.9 (0.3) | 0.032 |
Task 6 – retrieving objects | 9.5 (1.2) | 12.7 (1.3) | 0.000 |
Searching behavior | 7.6 (5.4) | 2.2 (2.0) | 0.029 |
Task-irrelevant behavior | 2.7 (1.5) | 0.3 (0.5) | 0.002 |
MCI, mild cognitive impairment; HC, healthy controls. Significant p-values <0.05 are represented in bold characters.
Table 3
Correlations between IADL performance in the smart home and MMSE, age, ADL questionnaires
Total Points | MMSE | Bayer-ADL | ADCS-MCI-ADL | Age | |
Total Time | –0.49** | –0.64** | 0.47** | –0.58** | 0.10 |
Total Points | 0.85** | –0.68** | 0.70** | –0.12 | |
MMSE | –0.67** | 0.66** | –0.12 | ||
Bayer-ADL | –0.80** | –0.03 | |||
ADCS-MCI-ADL | –0.07 |
**p < 0.01; N = 21.
Table 4
Correlations between completion time of the smart home tasks and CERAD-subtests
z-scores\time | Task 1 Placing objects | Task 2 Making coffee | Task 3 Making a phone call | Task 4 Preparing a sandwich | Task 5 Operating the TV | Task 6 Retrieving objects |
CERAD Word List Learning | –0.52** | –0.12 | –0.49** | –0.32* | –0.32* | –0.41* |
CERAD Constructional Praxis | –0.30* | –0.23 | –0.40** | –0.29* | –0.39** | –0.43** |
CERAD Word List Recall | –0.33* | –0.01 | –0.50** | –0.23 | –0.18 | –0.35* |
Trail Making Test A | –0.26 | –0.36* | –0.37** | –0.44** | –0.25 | –0.37** |
Trail Making Test B | –0.42** | –0.33* | –0.41** | –0.35* | –0.31* | –0.38** |
**p < 0.01; *p < 0.05; N = 21.