A Human-Based Integrated Framework forAlzheimer’s Disease Research
Abstract
Animal models of Alzheimer’s disease (AD) have been extensively utilized for decades in an effort to elucidate the pathophysiological mechanisms of this disease and to test novel therapeutic approaches. However, research success has not effectively translated into therapeutic success for human patients. This translational failure is partially due to the overuse of animal models that cannot accurately recapitulate human AD etiopathogenesis or drug responses and the inadequate use of human-relevant research methods. Here, we propose how to mitigate this translational barrier by employing human-based methods to elucidate disease processes occurring at multiple levels of complexity, accounting for gene and protein expression and the impact of disease at the cellular, tissue/organ, individual, and population levels. In particular, novel human-based cellular and computational models, together with epidemiological and clinical studies, represent the ideal tools to facilitate human-relevant data acquisition, in the effort to better elucidate AD pathogenesis in a human-based setting and design more effective treatments and preventive strategies. Our analysis indicates that a paradigm shift toward human-based, rather than animal-based research is required in the face of the ever-increasing prevalence of AD in the 21st century.
INTRODUCTION
Alzheimer’s disease (AD) represents the most common cause of dementia, accounting for 50–75% of all dementia cases [1]. This is a devastating disease affecting every aspect of life, as AD patients progress from very mild to severe cognitive impairment, losing their memory, their relationships with their families, and their ability to perform daily functions, such as talking, eating, and walking. The number of people living with AD in the United States alone is expected to increase from 5.2 million in 2013 to 13.8 million in 2050 [2], with an estimated cost of care expected to reach more than $1 trillion per year [1, 3]. It is clear that effective preventive and therapeutic strategies are urgently needed. In this regard, the AD research community has been very active with extensive research efforts designed to elucidate disease mechanisms and to develop novel therapeutics. However, these efforts have not successfully translated into effective drugs for AD patients. To date, only three drugs have been approved for AD (donepezil, galantamine, and rivastigmine) and they can only treat the symptoms of AD. It is well known that they are effective in only a small subset of patients, and only for a limited period of time [4]. Since 1998, 101 potential AD drugs have advanced to human trials but failed [5], and with recent costly failures such as Dimebon [6], drug companies are downgrading their efforts to develop novel pharmacotherapeutics for AD [7]. Understanding and resolving the reasons for these translational failures in AD is therefore imperative. Additionally, several lines of evidence indicate that AD should be reinterpreted as part of a constellation of diseases, in particular, as a complex systemic/metabolic dysfunction, given the significant correlations found between AD and the metabolic syndrome [8], as well as hypometabolism, oxidative stress, and glucose-fatty acid cycle modifications [9]. Consequently, current and past research failures might also be attributed to the fact that traditional research efforts and strategies have not been taking this complexity into account. Indeed, over the last decade, AD molecular mechanisms and drug efficacies have been studied extensively in animal models— primarily in murine models, but also in nonhuman primates, rats, dogs, and other species— all in an effort to assess in animals the contributions of specific genes and proteins to the onset of AD, commonly without accounting for the multifactorial nature of the disease. These animal models consistently fail to accurately recapitulate human AD causes, complex molecular and cellular dynamics, clinical manifestations, and drug responses. This is primarily due to anatomical, biochemical, and physiological as well as genetic and epigenetic interspecies differences between animals and humans [10]. In this review, we propose a novel human-based integrated framework, accounting for multiple levels of biological complexity, which can be used to improve characterization of human AD and develop effective treatments and preventive strategies for human patients.
AD AND INADEQUACY OF TRADITIONAL AD MODELS
The two types of AD are familial AD (∼5% of all AD cases), often occurring before age 60, and late-onset AD ( 95% of all AD cases), occurring in people over the age of 65. Familial AD is generally characterized by the presence of mutations found in genes encoding the γ-secretase complex components, such as presenilin-1 and −2, or in the amyloid-β (A4) protein precursor (AβPP) [11]. Duplication of the AβPP gene has also been linked to autosomal dominant familial AD [12]. Late-onset AD is generally defined as ‘sporadic’, given the lack of specific genetic factors directly associated with the disease. Genome-wide association studies (GWAS) have shown that AD is also associated with variations of several gene loci, such as the apolipoprotein E ɛ4 allele (APOE ɛ4) [13–17]. Accumulation of amyloid-β (Aβ) plaques and neurofibrillary tangles, composed of accumulated microtubule-associated total, and phosphorylated tau (t-tau and p-tau) protein, are the two physiological hallmarks of human AD, though it is unclear what role, if any, they play in the disease [18] (Supplementary Table 1).
Over the last decade, AD research has focused on the development of animal models to mimic at least some features of human AD described above. However, while certain murine models have been able to exhibit some of the genetic traits found in early-onset familial AD [19–21], their suitability to study late-onset AD is questionable [22], as confirmed by the dramatically high number of failed clinical trials [10, 23]. Without an understanding of the genetics of AD as it occurs in humans, the genetic contribution to disease pathogenesis is virtually impossible to model in mice [24]. In addition to the use of animals, overly simplistic cellular models of AD, often utilizing cancer cell lines or nonhuman cells cultured under nonhomeostatic and nonphysiologic in vitro culture conditions [25] have been commonly applied, further hampering research and drug discovery in AD [10]. Altogether, this highlights the need to rethink current research strategies and improve both sensitivity and specificity of research methods that will mitigate this wide translationalgap.
RETHINKING AD RESEARCH FOCUSING ON HUMAN-RELEVANT DATA ACQUISITION
Human-based cellular and tissue models, combined with epidemiological data and high-throughput readouts, can serve as the basis for a paradigm shift in AD research, facilitating human-relevant data acquisition. These tools and novel assays will allow researchers to better elucidate AD pathogenesis in a human-based setting and to design more effective treatments and preventive strategies. To integrate the vast amount of data deriving from comprehensive ‘omics’ studies, systems biology methods are of fundamental importance. This approach will allow researchers to describe molecular mechanisms underlying AD pathogenesis. In particular, several environmental and lifestyle risk factors, such as specific food nutrients and environmental toxicants, are known to play a pivotal role in the onset of pathologic changes underlying AD, which often appear many years before the symptomatic stages [26]. By using human-based models and novel computational methods, it is now possible to unravel the epigenetic and molecular mechanisms underlying AD pathogenesis (Fig. 1, Table 1).
The population and individual levels: Epidemiological studies and novel ‘omics’ readouts
Epidemiological studies have been important in identifying AD-related risk factors. The primary risk factors for AD include advancing age [27–29], nutritional patterns characterized by low intake of plant-derived foods [30], together with metabolic syndrome-related dysfunctions (e.g., cardiovascular disease and diabetes) [8, 31], low socioeconomic status and a low level of educational attainment [32–34], low level of daily physical activity [35, 36], and low cognitive training [37]. Additionally, sleep disorders [38–40], known to positively correlate to early Aβ deposition [41, 42], exposure to air pollution [43], smoking [44], and the intake of metals (e.g. aluminum [45], dietary copper [46], and manganese [47]) have been described as possible risk factors. Knowledge of these risk factors may enable the discovery of early biomarkers of AD and the development of intervention strategies to ameliorate or prevent early symptoms of AD.
In particular, analysis of both AD patient-derived plasma and cerebrospinal fluid (CSF) has been proven essential to identify possible early biomarkers of AD (Table 2). The presence of chronic neuroinflammation, caused by the progressive senescence of the immune system and by a sustained secretion of adipose tissue cytokines, seems to play a major role in cardiovascular disease and neurodegeneration [48], possibly contributing to AD [49, 50]. The presence of high levels of both plasma and brain homocysteine (HCys) is correlated to AD and neurodegenerative disorders. Elevated HCys in brain microvessels may be implicated in the disruption of the blood-brain barrier [51] and induction of excitotoxicity in cells expressing glutamate receptors of the N-methyl-D-aspartate class [52]. Other biomarkers considered relevant to AD are: low levels of plasma uric acid [53, 54], high levels of serum 3,4-dihydroxybutanoic acid (C4H8O4), docosapentaenoic acid (C22:5) [55], and hexacosanoic acid (C26:0) [56], the presence of insulin resistance and high insulin-like growth factor expression [57–59], impaired glycemic levels [60], mitochondrial damage and increased mitochondrial O-linked N-acetylglucosamine transferase activity [61], the presence of a dyslipidemic profile [31, 62–66], deregulation of plasma orexin [67–70], and high levels of the secreted heparin-binding glycoprotein YKL-40 [71, 72] (Table 2). Additionally, the CSF milieu is altered in subjects presenting AD. Analysis of the CSF revealed the deregulation of several biomarkers currently hypothesized to play a key role for AD early diagnosis, such as the Aβ 1 - 42, t-tau, and p-tau181 [73], and in particular the levels of p-tau181/t-tau and p-tau181/Aβ 1 - 42 ratios, considered good indicators of dementia severity and contributing to discrimination between mild or moderate-to-severe AD cases [74](Table 2).
The novel concept of the ‘exposome’, accounting for the totality of environmental exposures from gestation onward, is currently considered complementary to the genome in the study of disease etiology [75, 76]. In particular, among the possible triggers of the neurodegenerative process and in particular of AD, nutrients have the potential to be either prevention- or risk-related factors. The study of their effects at the gene expression level, by means of nutrigenomic analyses, is a field of broad scientific interest [77, 78]. Despite some conflicting evidences, plant-based, plant-rich diets and plant-derived bioactive nutrients appear to inhibit neuroinflammation and neurodegeneration molecular and cellular processes [79] and seem to be particularly beneficial in the management of early stages of cognitive impairment [80–84]. Some nutrigenetic studies have highlighted correlations between specific nutrient intakes and single-nucleotide polymorphisms. In this regard, it has been reported that subjects presenting the TT homozygous methylene tetrahydrofolate reductase allelic variant showed the lowest serum folate level, the highest serum HCys level and the lowest Mini-Mental State Examination (MMSE) score, as compared to all other genotypes [85]. Further studies aiming to identify the genetic susceptibility to AD and the interaction between specific genetic variations and nutrient intake might be invaluable in designing patient-tailored therapies and preventive approaches based on patient genetic makeups.
Novel and more powerful in vivo imaging readouts, such as ultra-high-field magnetic resonance imaging (MRI) and positron emission tomography, are currently available to diagnose AD [86, 87] and assess the effects of specific nutritional interventions [88–90]. Additionally, novel human connectomics (the production and study of connectomes) provides a three-dimensional reconstruction of neuronal networks within the human brain. In particular, diffusion magnetic resonance tractography, by combining MRI and computer-based imaging analysis, allows studying human brain anatomy through 2D and 3D images [91], and registering variations of brain cortical surfaces in AD patients [92, 93]. Additionally, electron microscopy images have been suitable to generate 3D neuron reconstruction in publicly available electron microscopy datasets [94]. Neuropsychological/cognitive tests (e.g., MMSE and the Hopkins Verbal Learning Test [95]) can be also used to assess multiple correlations among Aβ plaque distribution, specific AD-related biomarkers in CSF and plasma, and cognitive performance (Fig. 1, Table 1).
The organ/tissue and cellular levels: Novel human stem cell models and cell function readouts
In recent years, novel cellular models cultured under conditions more closely reflecting the human physiological cellular microenvironment and accounting for patient heterogeneity have been developed. AD patient-derived primary cells, such as neurons, might represent an ideal cellular source to study AD pathogenic features. However, these cells are generally available only postmortem and are difficult to keep in culture for extended periods of time [96]. To overcome these limitations, human induced pluripotent stem cells (hiPSCs) have been recently applied [97, 98]. hiPSCs can be generated by reprogramming adult somatic cells (e.g., skin fibroblasts) into pluripotent stem cells and further differentiating them in vitro to obtain AD-relevant tissues [10]. hiPSCs have been generated from patients affected with either familial or late-onset AD and have been differentiated into electrophysiologically active neurons, recapitulating common features of AD, such as high level of Aβ 1–40, active glycogen synthase kinase 3β, and p-tau [99], Aβ accumulation in neuronal cells and astrocytes, and endoplasmic reticulum oxidative stress [100]. Additionally, these differentiated neurons might be useful to identify the specific roles of cellular subtypes in the pathogenesis of AD, to obtain insights into patient-specific drug responses, for prospective diagnostics [101], and to assess the susceptibility of specific AD-related genes [102]. More recently, reprogramming of AD patient-derived non-neuronal somatic cells into neuronal mature cells (iNCs) [98, 103], AD patient-derived olfactory mucosa stem cells [96], genetically modified human neural stem cells (NSCs) [19], and three-dimensional culture systems [104] have been applied. Additionally, microglial cells, known to get activated in AD [105], have been derived from elderly patients to define the role of active microglia in the inflammatory process characterizing AD [106], and can be applied in co-culture systems to complement hiPSC, iNC, and NSC cultures. Moreover, novel brain-on-a-chip systems are currently under development, ideally leading to the design of novel drugs in human-based systems [107, 108]. Despite their current limitations, such as the lack of standardized criteria to build such systems [109] and their poor suitability for long term treatments [110], in general, these models might better represent the heterogeneity of the patient population, might reduce experimental timeframes and costs, might more accurately identify human AD characteristics, might be better tools for drug development, and might be applicable for use on high-throughput platforms [111](Fig. 1, Table 1).
A wide range of high-throughput readouts are currently available to assess etiopathological aspects of AD at tissue and cellular levels. Microelectrode array (MEA) devices have been developed in recent years, as substitutes of the more challenging and time-consuming patch clamp analyses [112]. Optogenetic techniques, used to monitor the activities of individual neurons in living tissue, together with MEA assays, antibody immunofluorescence (IF) and high content screening (HCS), could enable rapid and reproducible screening of drugs in vitro (Fig. 1, Table 1).
The signaling pathway/protein and epigenetic/genetic levels: High-throughput readouts
Several high-throughput technologies are currently available to unravel the molecular mechanisms underlying AD pathogenesis. Protein-related readouts (e.g., proteomics, antibody IF-HCS, metabolomics) will provide knowledge on the signaling pathways and protein interactions that are perturbed in AD at the translational and/or post-translational level (Fig. 1, Table 1). In particular, proteomics and phosphoproteomics have been useful to uncover signaling pathways involved in APOE ɛ4-related late-onset AD [114]. The use of capillary electrophoresis–mass spectrometry can help identify abnormal phosphorylation patterns of proteins implicated in cell metabolism, signal transduction, cytoskeleton integration, and synaptic function [115]. Also, analysis of in vivo metabolites of neuronal and glial cells, representative of energy metabolism, level of inflammation and neurotransmitter release, can be performed by using proton magnetic resonance spectroscopy [116]. Finally, genomics, epigenetics and gene expression analyses (e.g., GWAS, gene expression profiling, nutrigenomics, and nutrigenetics), will serve to identify perturbed gene expression particularly in late-onset AD [117, 118]. Late-onset AD patients often present abnormal patterns of histone acetylation and methylation and deregulated noncoding microRNA, which may play a role in AD pathophysiology[118].
Computational models, data extrapolation, and functional studies to validate epidemiological observations
Novel computational models that are currently applied in toxicology, such as in vitro-in vivo extrapolation, physiologically based pharmacokinetic and pharmacodynamics modeling, suitable to define kinetics and dynamics of compound exposure and to predict chemical long term effects [119], might be applied to predict the absorption, distribution, metabolism, and excretion of chemicals, such as chlorpyrifos [120] and manganese [121], both implicated in Aβ deposition [47, 122], and also to assess the efficacy of compounds for AD treatment, such as β-secretase inhibitors [123], the bioactive phytocompound curcumin [124], and APOE ɛ4 inhibitors [125].
Extrapolation of data obtained from the above described high-throughput readouts and computational simulations might be useful to define early biomarkers of AD, confirm meta-analysis of epidemiological observations, and predict therapeutic efficacy (Fig. 1). In particular, functional studies might be useful to confirm specific roles of identified perturbed pathways/genes at the onset of AD [126, 127]. In this regard, reporter human cell cultures of an identified perturbed signaling pathway might be generated, in an effort to investigate at high-throughput scale possible perturbation of the identified pathway when exposed to known and unknown compounds, in relation to AD pathogenesis. The ultimate goals would be the definition of novel therapeutic compounds and the implementation of intervention strategies aimed at preventing and/or reducing the early symptoms of AD. Additional in vitro functional studies aimed at defining the role of specific genes in the onset of AD would include gain- or loss-of-function approaches and also RNA interference-induced gene silencing using novel human cellular models of AD [128, 129].
DISCUSSION
Following decades of extensive research, it is now clear that traditional animal and cell culture models of AD are not reliable for studying complex pathophysiological aspects of the disease or for designing effective drugs. This deep gap in translational research highlights the need for a paradigm shift in AD research, from animal in vivo models and suboptimal in vitro cell lines, toward a more reliable and reproducible human conceptual framework. Here we described an integrated human-based framework suitable for investigating cellular and molecular mechanisms underlying AD pathology, pharmacotherapeutics, and preventive strategies for human patients. In recent years, the shift toward a new human-based paradigm has been advocated extensively in toxicology and regulatory testing [130], but also in other research fields, including AD [10, 131–134]. The envisioned human-based framework will not only increase human relevance and translatability, but also contribute to the reduction and/or replacement of animals traditionally used in AD research. In this day and age where there is growing concern for the ethical justification of the use of animals in research [135], it is important to consider not only the scientific dimensions, but also the ethical cost of the use of sentient beings in AD research.
Several human stem cell models of AD have been developed, spanning from patient-derived neurons and reprogrammed cells to three-dimensional genetically modified NSCs and the more complex brain-on-a-chip. Human stem cell-based tools and high-throughput readouts, supported by epidemiology studies, represent the basis of a paradigm shift in AD research that will increase knowledge of the molecular mechanisms that are perturbed at the onset of the disease, helping define novel biomarkers for early detection, and establishing preventive and treatment strategies.
It should be noted that in the envisioned strategic framework, the use of patient-derived cellular models such as iPSCs, and the application of omics readouts— while addressing human relevance— would still constitute the lower level/scale of ‘wet lab’ research. Therefore, large computational approaches together with large-scale epidemiological data sets represent the essential tools required to account for higher level/scale and to establish systemic correlations among signaling pathways, epigenomic and genomic perturbations, patients heterogeneity, and lifestyle components. In this regard, a map of signaling pathways and networks that are deregulated in AD is provided by ‘AlzPathway’, which may help in identifying candidate genes as predictors of AD risk, in combination with other ‘omics’ data [136].
Importantly, the implementation of epidemiological, prevention, and intervention clinical studies required to unravel the role of risk factors currently associated with AD, will require increasing the level of current research funding (currently only 7% – 9% of the total $30 billion NIH discretionary budget [137]). Redefining the strategy by which current and future NIH research budget is allocated is of particular relevance, especially considering the major role played by lifestyle factors, such as nutrition, physical activity, and level of cognitive training in the determination of AD risk [27–30, 35–37] as well as the proven efficacy of intervention strategies aimed at preventing dementia-related symptoms [88–90].
In conclusion, combining data derived from a wide range of studies, also accounting for neuropsychological/cognitivity tests, neuroimaging, the analysis of patient-derived CSF- and plasma-related biomarkers, together with computational models and high-throughput readouts applied to patient-derived cell-based models to assess signaling pathways, post-translational, translational, and transcriptional events, represent an invaluable and more reliable strategy to better understand AD pathology, predict long-term sequelae, and develop successful treatments [10, 138]. The envisioned framework will help redefine human AD pathology and etiology according to a more holistic perspective, taking into account the numerous human-related risk factors implicated in the onset and consolidation of AD [8, 9]. Modern research must take these multifactorial aspects into account. Indeed, current and past research failures may in fact be due to (i) a failure to recognize that AD lies on the spectrum of dementia and may be more accurately considered as part of a constellation of diseases, and/or (ii) more radically, AD may be inexorably linked to aging, making it a more intractable problem with more profound implications than generally acknowledged.
The feasibility of the envisioned human-basedstrategy necessarily requires the combined application of several methods and readouts and, consequentially, of multiple areas of expertise and laboratory facilities. The establishment of a collaborative scenario is clearly mandatory to determine what occurs throughout the course of AD.
CONCLUSION
Human stem-cell in vitro models, high-throughput (‘omics’) readouts, computational models, together with data obtained from meta-analysis of epidemiological and interventional studies, are among the ideal tools to elucidate etiopathological aspects of AD in a human-based setting and to predict environment-elicited biological perturbations occurring in AD, accounting for multiple levels of complexity, frompopulation/individual level down to gene level.
ACKNOWLEDGMENTS
We thank Drs. John J. Pippin and Neal D. Barnard for their constructive comments on the manuscript.
Authors’ disclosures available online (http://j-alz.com/manuscript-disclosures/15-0281r1).
Appendices
The supplementary material is available in the electronic version of this article: http://dx.doi.org/10.3233/JAD-150281.
REFERENCES
1 | Alzheimer’s Association (2013) 2013 Alzheimer’s Disease Facts and Figures, http://www.alz.org/downloads/facts_figures_2013.pdf, Accessed March 19, 2015 |
2 | Hebert LE, Weuve J, Scherr PA, Evans DA(2013) Alzheimer disease in the United States (2010-2050) estimated using the 2010 censusNeurology80: 17781783 |
3 | Alzheimer’s Disease International (2009) World Alzheimer Report 2009http://www.alz.co.uk/research/files/WorldAlzheimerReport.pdf,Accessed March 19, 2015 |
4 | Mayo Clinic, Alzheimer’s: Drugs help manage symptoms, http://www.mayoclinic.org/diseases-conditions/alzheimers-disease/in-depth/alzheimers/art-20048103, Accessed March 19, 2015 |
5 | PhRMA (2012) Researching Alzheimer’s Medicines: Setbacks and Stepping Stones,http://www.phrma.org/sites/default/files/pdf/alzheimersetbacksreportfinal912.pdf, Accessed March 19, 2015 |
6 | Bezprozvanny I(2010) The rise and fall of DimebonDrug News Perspect23: 518523 |
7 | Laurance J (2012) Drug giants give up on Alzheimer’s cure. The Independent,http://www.independent.co.uk/life-style/health-and-families/health-news/drug-giants-give-up-on-alzheimers-cure-8153606.html ,Accessed March, 19 2015 |
8 | Rios JA, Cisternas P, Arrese M, Barja S, Inestrosa NC(2014) Is Alzheimer’s disease related to metabolic syndrome? A Wnt signaling conundrumProg Neurobiol121: 125146 |
9 | Heininger K(2000) A unifying hypothesis of Alzheimer’s disease. IV. Causation and sequence of eventsRev Neurosci11 Spec No: 213328 |
10 | Langley GR(2014) Considering a new paradigm for Alzheimer’s disease researchDrug Discov Today19: 11141124 |
11 | Bertram L, Tanzi RE(2008) Thirty years of Alzheimer’s disease genetics: The implications of systematic meta-analysesNat Rev Neurosci9: 768778 |
12 | Rovelet-Lecrux A, Hannequin D, Raux G, Le Meur N, Laquerriere A, Vital A, Dumanchin C, Feuillette S, Brice A, Vercelletto M, Dubas F, Frebourg T, Campion D(2006) APP locus duplication causes autosomal dominant early-onsetAlzheimer disease with cerebral amyloid angiopathyNat Genet38: 2426 |
13 | Chouraki V, Seshadri S(2014) Genetics of Alzheimer’s diseaseAdv Genet87: 245294 |
14 | Hollingworth P, Harold D, Jones L, Owen MJ, Williams J(2011) Alzheimer’s disease genetics: Current knowledge andfuture challengesInt J Geriatr Psychiatry26: 793802 |
15 | Shibata N, Nagata T, Tagai K, Shinagawa S, Ohnuma T, Kawai E, Kasanuki K, Shimazaki H, Toda A, Tagata Y, Nakada T, Nakayama K, Yamada H, Arai H(2014) Association between the catechol-O-methyltransferase polymorphism Val158Met and Alzheimer’s disease in a Japanese populationInt J Geriatr Psychiatry10.1002/gps.4237 |
16 | Peterson D, Munger C, Crowley J, Corcoran C, Cruchaga C, Goate AM, Norton MC, Green RC, Munger RG, Breitner JC, Welsh-Bohmer KA, Lyketsos C, Tschanz J, Kauwe JSAlzheimer’s Disease Neuroimaging I(2014) Variants in PPP3R1and MAPT are associated with more rapid functional decline in Alzheimer’s disease: The Cache County Dementia Progression StudyAlzheimers Dement10: 366371 |
17 | Barberger-Gateau P, Lambert JC, Feart C, Peres K, Ritchie K, Dartigues JF, Alperovitch A(2013) From genetics todietetics: The contribution of epidemiology to understanding Alzheimer’s diseaseJ Alzheimers Dis33: Suppl 1S457S463 |
18 | Ballatore C, Lee VM, Trojanowski JQ(2007) Tau-mediated neurodegeneration in Alzheimer’s disease and related disordersNat Rev Neurosci8: 663672 |
19 | Choi SH, Kim YH, Hebisch M, Sliwinski C, Lee S, D’Avanzo C, Chen H, Hooli B, Asselin C, Muffat J, Klee JB, Zhang C, Wainger BJ, Peitz M, Kovacs DM, Woolf CJ, Wagner SL, Tanzi RE, Kim DY(2014) A three-dimensional human neuralcell culture model of Alzheimer’s diseaseNature515: 274278 |
20 | Tanzi RE(2012) The genetics of Alzheimer diseaseCold Spring Harb Perspect Med2: pii: a006296 |
21 | Oddo S, Caccamo A, Shepherd JD, Murphy MP, Golde TE, Kayed R, Metherate R, Mattson MP, Akbari Y, LaFerla FM(2003) Triple-transgenic model of Alzheimer’s disease with plaques and tangles: Intracellular Abeta and synapticdysfunctionNeuron39: 409421 |
22 | Duyckaerts C, Potier MC, Delatour B(2008) Alzheimer disease models and human neuropathology: Similarities and differencesActa Neuropathol115: 538 |
23 | Cavanaugh SE, Pippin JJ, Barnard ND(2014) Animal models of Alzheimer disease: Historical pitfalls and a path forwardALTEX31: 279302 |
24 | Livesey FJ(2012) Stem cell models of Alzheimer’s disease and related neurological disordersAlzheimers ResTher4: 44 |
25 | Hartung T(2013) Look back in anger - what clinical studies tell us about preclinical workALTEX30: 275291 |
26 | Drzezga A(2009) Diagnosis of Alzheimer’s disease with [18F]PET in mild and asymptomatic stagesBehav Neurol21: 101115 |
27 | Chai CK(2007) The genetics of Alzheimer’s diseaseAm J Alzheimers Dis Other Demen22: 3741 |
28 | Gatz M, Reynolds CA, Fratiglioni L, Johansson B, Mortimer JA, Berg S, Fiske A, Pedersen NL(2006) Role of genesand environments for explaining Alzheimer diseaseArch Gen Psychiatry63: 168174 |
29 | Rossor MN, Fox NC, Freeborough PA, Harvey RJ(1996) Clinical features of sporadic and familial Alzheimer’s diseaseNeurodegeneration5: 393397 |
30 | Pistollato F, Battino M(2014) Role of plant-based diets in the prevention and regression of metabolic syndrome and neurodegenerative diseasesTrends Food Sci Technol40: 6281 |
31 | Rosendorff C, Beeri MS, Silverman JM(2007) Cardiovascular risk factors for Alzheimer’s diseaseAm J Geriatr Cardiol16: 143149 |
32 | Russ TC, Stamatakis E, Hamer M, Starr JM, Kivimaki M, Batty GD(2013) Socioeconomic status as a risk factor for dementia death: Individual participant meta-analysis of 86 508 men and women from the UKBr J Psychiatry203: 1017 |
33 | Sattler C, Toro P, Schonknecht P, Schroder J(2012) Cognitive activity, education and socioeconomic status as preventive factors for mild cognitive impairment and Alzheimer’s diseasePsychiatry Res196: 9095 |
34 | Paradise M, Cooper C, Livingston G(2009) Systematic review of the effect of education on survival in Alzheimer’s diseaseInt Psychogeriatr21: 2532 |
35 | Hernandez SS, Sandreschi PF, Silva FC, Arancibia BA, da Silva R, Gutierres PJ, Andrade A(2014) What are thebenefits of exercise for Alzheimer’s disease? A systematic review of past 10 yearsJ Aging Phys Act10.1123/japa.2014-0180 |
36 | Okonkwo OC, Schultz SA, Oh JM, Larson J, Edwards D, Cook D, Koscik R, Gallagher CL, Dowling NM, Carlsson CM, Bendlin BB, LaRue A, Rowley HA, Christian BT, Asthana S, Hermann BP, Johnson SC, Sager MA(2014) Physicalactivity attenuates age-related biomarker alterations in preclinical ADNeurology83: 17531760 |
37 | Gates NJ, Sachdev P(2014) Is cognitive training an effective treatment for preclinical and early Alzheimer’sdisease?J Alzheimers Dis42: S551S559 |
38 | Lim MM, Gerstner JR, Holtzman DM(2014) The sleep-wake cycle and Alzheimer’s disease: What do we know?Neurodegener Dis Manag4: 351362 |
39 | Videnovic A, Lazar AS, Barker RA, Overeem S(2014) ’The clocks that time us’-circadian rhythms in neurodegenerative disordersNat Rev Neurol10: 683693 |
40 | Peter-Derex L, Yammine P, Bastuji H, Croisile B(2015) Sleep and Alzheimer’s diseaseSleep Med Rev19: 2938 |
41 | Lucey BP, Bateman RJ(2014) Amyloid-beta diurnal pattern: Possible role of sleep in Alzheimer’s disease pathogenesisNeurobiol Aging35: Suppl 2S29S34 |
42 | Ju YE, Lucey BP, Holtzman DM(2014) Sleep and Alzheimer disease pathology–a bidirectional relationshipNat Rev Neurol10: 115119 |
43 | Moulton PV, Yang W(2012) Air pollution, oxidative stress, and Alzheimer’s diseaseJ Environ Public Health2012: 472751 |
44 | Cataldo JK, Prochaska JJ, Glantz SA(2010) Cigarette smoking is a risk factor for Alzheimer’s Disease: An analysis controlling for tobacco industry affiliationJ Alzheimers Dis19: 465480 |
45 | Shcherbatykh I, Carpenter DO(2007) The role of metals in the etiology of Alzheimer’s diseaseJ Alzheimers Dis11: 191205 |
46 | Brewer GJ(2012) Copper excess, zinc deficiency, and cognition loss in Alzheimer’s diseaseBiofactors38: 107113 |
47 | Tong Y, Yang H, Tian X, Wang H, Zhou T, Zhang S, Yu J, Zhang T, Fan D, Guo X, Tabira T, Kong F, Chen Z, Xiao W, Chui D(2014) High manganese, a risk for Alzheimer’s disease: High manganese induces amyloid-beta relatedcognitive impairmentJ Alzheimers Dis42: 865878 |
48 | Michaud M, Balardy L, Moulis G, Gaudin C, Peyrot C, Vellas B, Cesari M, Nourhashemi F(2013) Proinflammatory cytokines, aging, and age-related diseasesJ Am Med Dir Assoc14: 877882 |
49 | Obasi CN, Cruickshanks KJ, Nondahl DM, Klein BE, Klein R, Nieto FJ, Shankar A, Fischer ME, Tsai MY, Chappell R(2012) Association of biomarkers for inflammation, endothelial dysfunction and oxidative stress with cognitiveimpairment. The Epidemiology of Hearing Loss Study (EHLS)Oxid Antioxid Med Sci1: 169173 |
50 | Tripathy D, Sanchez A, Yin X, Luo J, Martinez J, Grammas P(2013) Thrombin, a mediator of cerebrovascularinflammation in AD and hypoxiaFront Aging Neurosci5: 19 |
51 | Kamath AF, Chauhan AK, Kisucka J, Dole VS, Loscalzo J, Handy DE, Wagner DD(2006) Elevated levels of homocysteinecompromise blood-brain barrier integrity in miceBlood107: 591593 |
52 | Boldyrev A, Bryushkova E, Mashkina A, Vladychenskaya E(2013) Why is homocysteine toxic for the nervous and immune systems?Curr Aging Sci6: 2936 |
53 | Al-khateeb E, Althaher A, Al-khateeb M, Al-Musawi H, Azzouqah O, Al-Shweiki S, Shafagoj Y(2015) Relation between uric acid and Alzheimer’s disease in elderly JordaniansJ Alzheimers Dis44: 859865 |
54 | Chen X, Guo X, Huang R, Chen Y, Zheng Z, Shang H(2014) Serum uric acid levels in patients with Alzheimer’s disease: A meta-analysisPLoS One9: e94084 |
55 | Mousavi M, Jonsson P, Antti H, Adolfsson R, Nordin A, Bergdahl J, Eriksson K, Moritz T, Nilsson LG, Nyberg L(2014) Serum metabolomic biomarkers of dementiaDement Geriatr Cogn Dis Extra4: 252262 |
56 | Zarrouk A, Riedinger JM, Ahmed SH, Hammami S, Chaabane W, Debbabi M, Ben Ammou S, Rouaud O, Frih M, Lizard G, Hammami M(2015) Fatty acid profiles in demented patients: Identification of hexacosanoic acid (C26:0) as a bloodlipid biomarker of dementiaJ Alzheimers Dis44: 13491359 |
57 | Ferreira ST, Clarke JR, Bomfim TR, De Felice FG(2014) Inflammation, defective insulin signaling, and neuronal dysfunction in Alzheimer’s diseaseAlzheimers Dement10: S76S83 |
58 | Willette AA, Johnson SC, Birdsill AC, Sager MA, Christian B, Baker LD, Craft S, Oh J, Statz E, Hermann BP, Jonaitis EM, Koscik RL, La Rue A, Asthana S, Bendlin BB(2015) Insulin resistance predicts brain amyloiddeposition in late middle-aged adultsAlzheimers Dement11: 504 e501510 e501 |
59 | de la Monte SM, Tong M, Lester-Coll N, Plater MJr, Wands JR(2006) Therapeutic rescue of neurodegeneration inexperimental type 3 diabetes: Relevance to Alzheimer’s diseaseJ Alzheimers Dis10: 89109 |
60 | Morris JK, Vidoni ED, Honea RA, Burns JMAlzheimer’s Disease Neuroimaging I(2014) Impaired glycemia increases disease progression in mild cognitive impairmentNeurobiol Aging35: 585589 |
61 | Lozano L, Lara-Lemus R, Zenteno E, Alvarado-Vasquez N(2014) The mitochondrial O-linked N-acetylglucosamine transferase (mOGT) in the diabetic patient could be the initial trigger to develop Alzheimer diseaseExp Gerontol58: 198202 |
62 | Vitali C, Wellington CL, Calabresi L(2014) HDL and cholesterol handling in the brainCardiovasc Res103: 405413 |
63 | Blom K, Emmelot-Vonk MH, Koek HL(2013) The influence of vascular risk factors on cognitive decline in patientswith dementia: A systematic reviewMaturitas76: 113117 |
64 | Vikarunnessa S, Weiner MF, Vega GL(2013) LDL phenotype in subjects with mild cognitive impairment and Alzheimer’s diseaseJ Alzheimers Dis36: 571575 |
65 | Reed B, Villeneuve S, Mack W, DeCarli C, Chui HC, Jagust W(2014) Associations between serum cholesterol levels and cerebral amyloidosisJAMA Neurol71: 195200 |
66 | Warren MW, Hynan LS, Weiner MF(2012) Lipids and adipokines as risk factors for Alzheimer’s diseaseJ Alzheimers Dis29: 151157 |
67 | Ferini-Strambi L(2014) Possible role of orexin in the pathogenesis of Alzheimer diseaseJAMA Neurol71: 14781480 |
68 | Nixon JP, Mavanji V, Butterick TA, Billington CJ, Kotz CM, Teske JA(2015) Sleep disorders, obesity, and aging: The role of orexinAgeing Res Rev20: 6373 |
69 | Adam JA, Menheere PP, van Dielen FM, Soeters PB, Buurman WA, Greve JW(2002) Decreased plasma orexin-A levels inobese individualsInt J Obes Relat Metab Disord26: 274276 |
70 | Fronczek R, van Geest S, Frolich M, Overeem S, Roelandse FW, Lammers GJ, Swaab DF(2012) Hypocretin (orexin) lossin Alzheimer’s diseaseNeurobiol Aging33: 16421650 |
71 | Antonell A, Mansilla A, Rami L, Llado A, Iranzo A, Olives J, Balasa M, Sanchez-Valle R, Molinuevo JL(2014) Cerebrospinal fluid level of YKL-40 protein in preclinical and prodromal Alzheimer’s diseaseJ AlzheimersDis42: 901908 |
72 | Craig-Schapiro R, Perrin RJ, Roe CM, Xiong C, Carter D, Cairns NJ, Mintun MA, Peskind ER, Li G, Galasko DR, Clark CM, Quinn JF, D’Angelo G, Malone JP, Townsend RR, Morris JC, Fagan AM, Holtzman DM(2010) YKL-40: A novelprognostic fluid biomarker for preclinical Alzheimer’s diseaseBiol Psychiatry68: 903912 |
73 | Engelborghs S(2013) Clinical indications for analysis of Alzheimer’s disease CSF biomarkersRev Neurol (Paris)169: 709714 |
74 | Huded CB, Bharath S, Chandra SR, Sivakumar PT, Varghese M, Subramanian S(2015) Supportive CSF biomarker evidenceto enhance the National Institute on Aging-Alzheimer’s Association criteria for diagnosis of Alzheimer’s typedementia - A study from Southern IndiaAsian J Psychiatr13: 4447 |
75 | Rappaport SM(2012) Discovering environmental causes of diseaseJ Epidemiol Community Health66: 99102 |
76 | Yegambaram M, Manivannan B, Beach TG, Halden RU(2015) Role of environmental contaminants in the etiology of Alzheimer’s disease: A reviewCurr Alzheimer Res12: 116146 |
77 | Oboudiyat C, Glazer H, Seifan A, Greer C, Isaacson RS(2013) Alzheimer’s diseaseSemin Neurol33: 313329 |
78 | Nicolia V, Lucarelli M, Fuso A(2015) Environment, epigenetics andneurodegeneration: Focus on nutrition in Alzheimer’s diseaseExp Gerontol68: 812 |
79 | Virmani A, Pinto L, Binienda Z, Ali S(2013) Food, nutrigenomics, and neurodegeneration–neuroprotection by what you eat!Mol Neurobiol48: 353362 |
80 | Jicha GA, Markesbery WR(2010) Omega-3 fatty acids: Potential role in the management of early Alzheimer’s diseaseClin Interv Aging5: 4561 |
81 | Bourre JM(2006) Effects of nutrients (in food) on the structure and function of the nervous system: Update ondietary requirements for brain. Part 1: MicronutrientsJ Nutr Health Aging10: 377385 |
82 | Feart C, Samieri C, Alles B, Barberger-Gateau P(2013) Potential benefits of adherence to the Mediterranean diet on cognitive healthProc Nutr Soc72: 140152 |
83 | Schaffer S, Asseburg H, Kuntz S, Muller WE, Eckert GP(2012) Effects of polyphenols on brain ageing and Alzheimer’s disease: Focus on mitochondriaMol Neurobiol46: 161178 |
84 | Joseph J, Cole G, Head E, Ingram D(2009) Nutrition, brain aging, and neurodegenerationJ Neurosci29: 1279512801 |
85 | Kageyama M, Hiraoka M, Kagawa Y(2008) Relationship between genetic polymorphism, serum folate and homocysteine in Alzheimer’s diseaseAsia Pac J Public Health20 Suppl: 111117 |
86 | Kerchner GA(2011) Ultra-high field 7T MRI: A new tool for studying Alzheimer’s diseaseJ Alzheimers Dis26: Suppl 39195 |
87 | Maruyama M, Shimada H, Suhara T, Shinotoh H, Ji B, Maeda J, Zhang MR, Trojanowski JQ, Lee VM, Ono M, Masamoto K, Takano H, Sahara N, Iwata N, Okamura N, Furumoto S, Kudo Y, Chang Q, Saido TC, Takashima A, Lewis J, Jang MK, Aoki I, Ito H, Higuchi M(2013) Imaging of tau pathology in a tauopathy mouse model and in Alzheimer patients compared to normal controlsNeuron79: 10941108 |
88 | Blasko I, Hinterberger M, Kemmler G, Jungwirth S, Krampla W, Leitha T, Heinz Tragl K, Fischer P(2012) Conversionfrom mild cognitive impairment to dementia: Influence of folic acid and vitamin B12 use in the VITA cohortJ Nutr Health Aging16: 687694 |
89 | Mosconi L, Murray J, Tsui WH, Li Y, Davies M, Williams S, Pirraglia E, Spector N, Osorio RS, Glodzik L, McHugh P, de Leon MJ(2014) Mediterranean diet and magnetic resonance imaging-assessed brain atrophy in cognitively normal individuals at risk for Alzheimer’s diseaseJ Prev Alzheimers Dis1: 2332 |
90 | Titova OE, Ax E, Brooks SJ, Sjogren P, Cederholm T, Kilander L, Kullberg J, Larsson EM, Johansson L, Ahlstrom H, Lind L, Schioth HB, Benedict C(2013) Mediterranean diet habits in older individuals: Associations with cognitivefunctioning and brain volumesExp Gerontol48: 14431448 |
91 | Jbabdi S, Johansen-Berg H(2011) Tractography: Where do we go from here?Brain Connect1: 169183 |
92 | Gutman B, Leonardo C, Jahanshad N, Hibar D, Eschenburg K, Nir T, Villalon J, Thompson P(2014) Registeringcortical surfaces based on whole-brain structural connectivity and continuous connectivity analysisMedImage Comput Comput Assist Interv17: 161168 |
93 | Prescott JW, Guidon A, Doraiswamy PM, Roy Choudhury K, Liu C, Petrella JRAlzheimer’s Disease Neuroimaging I(2014) The Alzheimer structural connectome: Changes in cortical network topology with increased amyloid plaque burdenRadiology273: 175184 |
94 | Ensafi S, Shijian L, Kassim AA, Chew Lim T(2014) 3D reconstruction of neurons in electron microscopy imagesConf Proc IEEE Eng Med Biol Soc2014: 67326735 |
95 | Folstein M(2007) Improving dementia assessment by reducing sample heterogeneityInt Psychogeriatr19: 383389 |
96 | Mackay-Sim A(2013) Patient-derived stem cells: Pathways to drug discovery for brain diseasesFront CellNeurosci7: 29 |
97 | Saporta MA, Grskovic M, Dimos JT(2011) Induced pluripotent stem cells in the study of neurological diseasesStem Cell Res Ther2: 37 |
98 | Qiang L, Inoue K, Abeliovich A(2014) Instant neurons: Directed somatic cell reprogramming models of central nervous system disordersBiol Psychiatry75: 945951 |
99 | Israel MA, Yuan SH, Bardy C, Reyna SM, Mu Y, Herrera C, Hefferan MP, Van Gorp S, Nazor KL, Boscolo FS, Carson CT, Laurent LC, Marsala M, Gage FH, Remes AM, Koo EH, Goldstein LS(2012) Probing sporadic and familial Alzheimer’sdisease using induced pluripotent stem cellsNature482: 216220 |
100 | Kondo T, Asai M, Tsukita K, Kutoku Y, Ohsawa Y, Sunada Y, Imamura K, Egawa N, Yahata N, Okita K, Takahashi K, Asaka I, Aoi T, Watanabe A, Watanabe K, Kadoya C, Nakano R, Watanabe D, Maruyama K, Hori O, Hibino S, Choshi T, Nakahata T, Hioki H, Kaneko T, Naitoh M, Yoshikawa K, Yamawaki S, Suzuki S, Hata R, Ueno S, Seki T, Kobayashi K, Toda T, Murakami K, Irie K, Klein WL, Mori H, Asada T, Takahashi R, Iwata N, Yamanaka S, Inoue H(2013) ModelingAlzheimer’s disease with iPSCs reveals stress phenotypes associated with intracellular Abeta and differentialdrug responsivenessCell Stem Cell12: 487496 |
101 | Ooi L, Sidhu K, Poljak A, Sutherland G, O’Connor MD, Sachdev P, Munch G(2013) Induced pluripotent stem cells as tools for disease modelling and drug discovery in Alzheimer’s diseaseJ Neural Transm120: 103111 |
102 | Vaccarino FM, Stevens HE, Kocabas A, Palejev D, Szekely A, Grigorenko EL, Weissman S(2011) Induced pluripotentstem cells: A new tool to confront the challenge of neuropsychiatric disordersNeuropharmacology60: 13551363 |
103 | Qiang L, Fujita R, Abeliovich A(2013) Remodeling neurodegeneration: Somatic cell reprogramming-based models of adult neurological disordersNeuron78: 957969 |
104 | de Vries RB, Leenaars M, Tra J, Huijbregtse R, Bongers E, Jansen JA, Gordijn B, Ritskes-Hoitinga M(2013) Thepotential of tissue engineering for developing alternatives to animal experiments: A systematic reviewJTissue Eng Regen Med10.1002/term.1703 |
105 | Schlachetzki JC, Hull M(2009) Microglial activation in Alzheimer’s diseaseCurr Alzheimer Res6: 554563 |
106 | Walker DG, Lue LF(2005) Investigations with cultured human microglia on pathogenic mechanisms of Alzheimer’s disease and other neurodegenerative diseasesJ Neurosci Res81: 412425 |
107 | Huh D, Torisawa YS, Hamilton GA, Kim HJ, Ingber DE(2012) Microengineered physiological biomimicry: Organs-on-chipsLab Chip12: 21562164 |
108 | Sutherland ML, Fabre KM, Tagle DA(2013) The National Institutes of Health Microphysiological Systems Program focuses on a critical challenge in the drug discovery pipelineStem Cell Res Ther4 Suppl 1: I1 |
109 | Abaci HE, Shuler ML(2015) Human-on-a-chip design strategies and principles for physiologically based pharmacokinetics/pharmacodynamics modelingIntegr Biol (Camb)7: 383391 |
110 | Marx U, Walles H, Hoffmann S, Lindner G, Horland R, Sonntag F, Klotzbach U, Sakharov D, Tonevitsky A, Lauster R(2012) ’Human-on-a-chip’ developments: A translational cutting-edge alternative to systemic safety assessment andefficiency evaluation of substances in laboratory animals and man?Altern Lab Anim40: 235257 |
111 | Grskovic M, Javaherian A, Strulovici B, Daley GQ(2011) Induced pluripotent stem cells–opportunities for disease modelling and drug discoveryNat Rev Drug Discov10: 915929 |
112 | Pizzi R, Cino G, Gelain F, Rossetti D, Vescovi A(2007) Learning in human neural networks on microelectrode arraysBiosystems88: 115 |
113 | Dranias MR, Ju H, Rajaram E, VanDongen AM(2013) Short-term memory in networks of dissociated cortical neuronsJ Neurosci33: 19401953 |
114 | Rhinn H, Fujita R, Qiang L, Cheng R, Lee JH, Abeliovich A(2013) Integrative genomics identifies APOE epsilon4 effectors in Alzheimer’s diseaseNature500: 4550 |
115 | Zahid S, Oellerich M, Asif AR, Ahmed N(2012) Phosphoproteome profiling of substantia nigra and cortex regions ofAlzheimer’s disease patientsJ Neurochem121: 954963 |
116 | Graff-Radford J, Kantarci K(2013) Magnetic resonance spectroscopy in Alzheimer’s diseaseNeuropsychiatr Dis Treat9: 687696 |
117 | Mastroeni D, Grover A, Delvaux E, Whiteside C, Coleman PD, Rogers J(2011) Epigenetic mechanisms in Alzheimer’s diseaseNeurobiol Aging32: 11611180 |
118 | Millan MJ(2014) The epigenetic dimension of Alzheimer’s disease: Causal, consequence, or curiosity?Dialogues Clin Neurosci16: 373393 |
119 | Chetty M, Rose RH, Abduljalil K, Patel N, Lu G, Cain T, Jamei M, Rostami-Hodjegan A(2014) Applications oflinking PBPK and PD models to predict the impact of genotypic variability, formulation differences, differencesin target binding capacity and target site drug concentrations on drug responses and variabilityFrontPharmacol5: 258 |
120 | Poet TS, Timchalk C, Hotchkiss JA, Bartels MJ(2014) Chlorpyrifos PBPK/PD model for multiple routes of exposureXenobiotica44: 868881 |
121 | Santamaria AB(2008) Manganese exposure, essentiality & toxicityIndian J Med Res128: 484500 |
122 | Salazar JG, Ribes D, Cabre M, Domingo JL, Sanchez-Santed F, Colomina MT(2011) Amyloid beta peptide levelsincrease in brain of AbetaPP Swedish mice after exposure to chlorpyrifosCurr Alzheimer Res8: 732740 |
123 | Janson J, Eketjall S, Tunblad K, Jeppsson F, Von Berg S, Niva C, Radesater AC, Falting J, Visser SA(2014) Population PKPD modeling of BACE1 inhibitor-induced reduction in Abeta levels in vivo and correlation to in vitro potency in primary cortical neurons from mouse and guinea pigPharm Res31: 670683 |
124 | Rao PP, Mohamed T, Teckwani K, Tin G(2015) Curcumin binding tobeta amyloid: A computational studyChem Biol Drug Des10.1111/cbdd.12552 |
125 | Huang HJ, Chen HY, Lee CC, Chen CY(2014) Computational design of apolipoprotein E4 inhibitors for Alzheimer’sdisease therapy from traditional Chinese medicineBiomed Res Int2014: 452625 |
126 | Jin N, Qian W, Yin X, Zhang L, Iqbal K, Grundke-Iqbal I, Gong CX, Liu F(2013) CREB regulates the expression ofneuronal glucose transporter 3: A possible mechanism related to impaired brain glucose uptake in Alzheimer’sdiseaseNucleic Acids Res41: 32403256 |
127 | Vukic V, Callaghan D, Walker D, Lue LF, Liu QY, Couraud PO, Romero IA, Weksler B, Stanimirovic DB, Zhang W(2009) Expression of inflammatory genes induced by beta-amyloid peptides in human brain endothelial cells and in Alzheimer’s brain is mediated by the JNK-AP1 signaling pathwayNeurobiol Dis34: 95106 |
128 | Gan X, Huang S, Wu L, Wang Y, Hu G, Li G, Zhang H, Yu H, Swerdlow RH, Chen JX, Yan SS(2014) Inhibition of ERK-DLP1 signaling and mitochondrial division alleviates mitochondrial dysfunction in Alzheimer’s disease cybrid cellBiochim Biophys Acta1842: 220231 |
129 | Esposito G, Scuderi C, Lu J, Savani C, De Filippis D, Iuvone T, Steardo LJr, Sheen V, Steardo L(2008) S100Binduces tau protein hyperphosphorylation via Dickopff-1 up-regulation and disrupts the Wnt pathway in humanneural stem cellsJ Cell Mol Med12: 914927 |
130 | Gibb S(2008) Toxicity testing in the 21st century: A vision and a strategyReprod Toxicol25: 136138 |
131 | van der Worp HB, Howells DW, Sena ES, Porritt MJ, Rewell S, O’Collins V, Macleod MR(2010) Can animal models ofdisease reliably inform human studies?PLoS Med7: e1000245 |
132 | Begley CG, Ellis LM(2012) Drug development: Raise standards for preclinical cancer researchNature483: 531533 |
133 | Geerts H(2009) Of mice and men: Bridging the translational disconnect in CNS drug discoveryCNS Drugs23: 915926 |
134 | Mak IW, Evaniew N, Ghert M(2014) Lost in translation: Animal models and clinical trials in cancer treatmentAm J Transl Res6: 114118 |
135 | National Institutes of Health (2013) NIH to reduce significantly the use of chimpanzees in research,http://www.nih.gov/news/health/jun2013/od-26.htm, Accessed May 19, 2015 |
136 | Ogishima S, Mizuno S, Kikuchi M, Miyashita A, Kuwano R, Tanaka H, Nakaya J(2013) A map of Alzheimer’s disease-signaling pathways: A hope for drug target discoveryClin Pharmacol Ther93: 399401 |
137 | Calitz C, Pollack KM, Millard C, Yach D(2015) National institutes of health funding for behavioral interventions to prevent chronic diseasesAm J Prev Med48: 462471 |
138 | Reid AT, Evans AC(2013) Structural networks in Alzheimer’sdiseaseEur Neuropsychopharmacol23: 6377 |
Figures and Tables
Fig.1
Overview of the novel available tools and readouts applicable to design human-oriented AD research, accounting for multiple levels of complexity. CSF, cerebrospinal fluid; MRI, magnetic resonance imaging; PET, positron emission tomography; MMSE, Mini-Mental State Examination; HVLT, Hopkins verbal learning test; iPSCs, induced pluripotent stem cells; iNCs, induced neuronal cells; NSCs, neural stem cells; MEA, microelectrode array; IF HCS, immunofluorescence-high content screening; MS, mass spectrometry; MRS, magnetic resonance spectroscopy; GWAS, genome-wide association studies; GEP, gene expression profiling.
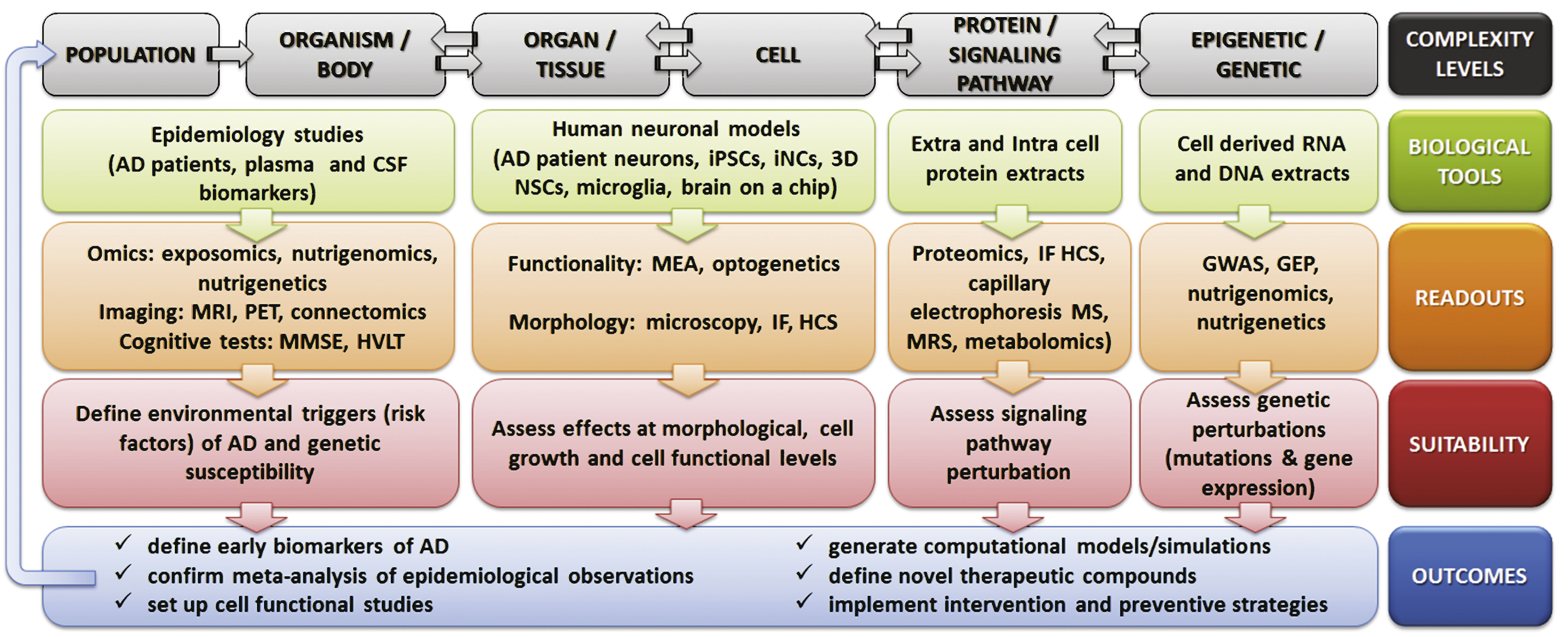
Table 1
Human-based models and methods for AD research
Complexity levels | Models/Readouts | Characteristics/Possible applications | References |
Population/individual level: data from epidemiological, clinical studies; tests on AD patients; test on AD patient samples (e.g., CSF, serum) | Ultra-high-field MRI | To assess cortical plaque disposition and early hippocampal tissue loss | [86] |
PET | To monitor Aβ plaque distribution in patients | [87] | |
Connectomics (e.g., diffusion magnetic resonance tractography) | 3D reconstruction of neuronal network within the human brain; allows registering variations of brain cortical surfaces in AD patients | [91–93] | |
Electron microscopy | Electron microscopy images suitable to generate 3D neuron reconstruction | [94] | |
Exposomics | To assess environmental exposure effects on gene expression | [75] | |
Nutrigenomics | To assess nutrient effects on gene expression | [77, 78] | |
Nutrigenetics | To assess genetic variants’ susceptibility to specific nutrients | [85] | |
Neuropsychological/cognitive tests (e.g., MMSE, HVLT) | To assess cognitive level | [95] | |
Tissue/cell levels: AD patient-derived tissues and cells | AD patient-derived primary cells (e.g., neurons) | Accounting for patient heterogeneity, available only postmortem, short periods of time in culture | [96] |
AD patient-derived hiPSCs and further differentiation into neurons | Accounting for patient heterogeneity, recapitulating common features of AD, applicable for drug evaluations, suitable for high-throughput assays | [97–102] | |
AD patient-derived iNCs | To study late-onset AD | [98, 103] | |
AD patient-derived olfactory mucosa | Easily biopsied or collected postmortem | [96] | |
3D human NSCs expressing AD-related mutations | Recapitulate extracellular Aβ deposition, plaque formation, high levels of p-tau and filamentous tau in both neuronal soma and neurites. Suitable to study familial AD | [19] | |
Human microglial cells | Derived from elderly patients; useful to define the role of active microglia in the inflammatory process at the onset of AD | [106] | |
3D in vitro human tissue models, with microfluidics | To better mimic in vivo physiological conditions | [104] | |
Brain-on-a-chip | To test drug efficacy and toxicity | [107, 108] | |
Readout: MEA | To directly record neuronal culture activity on chip, for drug testing | [112] | |
Readout: Optogenetics | To monitor the activities of individual neurons in living tissue | [113] | |
Readout: IF-HCS | High-throughput, to assess the effects of compounds at cellular level | [96] | |
Signaling pathway/protein level: human AD cell-derived protein samples | Proteomics and phosphoproteomics | High-throughput, to define molecules and signaling pathways involved in APOE ɛ4-related late-onset AD | [114] |
Capillary electrophoresis-mass spectrometry | To assess phosphorylation of metabolic proteins, signal transduction, cytoskeleton integration and synaptic functions | [115] | |
Proton MRS and metabolomics | To analyze in vivo metabolites of neuronal and glial cells representative of energy metabolism, level of inflammation and neurotransmitter release | [116] | |
Further functional studies: reporter human AD cell cultures of identified perturbed signaling pathways | To assess perturbation of identified pathways upon exposure to compounds | [126, 127] | |
Epigenetic/genetic level: human AD cell-derived RNA and DNA samples | Laser-capture microdissection and GEP | High-throughput, to analyze subtypes of cortical neurons obtained from postmortem brain | [117, 118] |
GWAS | To identify associations between specific gene loci and AD | [13–17] | |
Further functional studies: gain- or loss-of-function, RNA interference | To define the role of specific genes onset of AD | [128, 129] |
MRI, magnetic resonance imaging; PET, positron emission tomography; MMSE, mini-mental state examination; HVLT, Hopkins verbal learning test; hiPSCs, human induced pluripotent stem cells; iNCs, induced neuronal cells; NSCs, neural stem cells; MEA, microelectrode array; IF-HCS, immunofluorescence-high content screening; MRS, magnetic resonance spectroscopy; GEP, gene expression profiling; GWAS, genome-wide association studies.
Table 2
Summary of biomarkers possibly useful for AD detection
Biomarkers | Localization | References |
IL-6, IL-1, TNF-α, CRP | Plasma | [48] |
High HCys | Plasma, brain | [51, 52] |
Low uric acid | Plasma | [53, 54] |
High levels of: 3,4-dihydroxybutanoic acid (C4H8O4), docosapentaenoic acid (C22:5), hexacosanoic acid (C26:0) | Serum, plasma | [55, 56] |
Insulin resistance | Plasma, brain | [57, 58] |
IGF resistance | Plasma, brain | [59] |
Impaired glycemia | Plasma, brain | [60] |
Mitochondrial damage and high mOGT activity | Plasma, brain | [61] |
Low HDL-cholesterol | Plasma, brain | [62] |
High LDL-cholesterol, total cholesterol and triglycerides | Plasma, brain | [31, 63–66] |
Low orexin level | Plasma, CSF, hypothalamus | [67–70] |
High YKL-40 (a secreted heparin-binding glycoprotein) | Plasma, brain | [71, 72] |
Aβ1 - 42, t-tau, and p-tau181, p-tau181/t-tau and p-tau181/Aβ 1 - 42 ratios | CSF | [73, 74] |
IL-6, interleukin-6; TNF-α, tumor necrosis factor α; CRP, C-reactive protein; HCys, homocysteine; IGF, insulin growth factor; mOGT, high mitochondrial O-linked N-acetylglucosamine transferase; HDL, high-density lipoprotein; LDL, low-density lipoprotein; YKL-40, Chitinase-3-like protein 1 (or CHI3L1).