Prevalence of Antibiotic Resistance in Older Adults and Alzheimer’s Disease Patients: A Systematic Review and Meta-Analysis
Abstract
Background:
Antibiotic resistance is a global health concern, and its prevalence among older adults and Alzheimer’s disease (AD) patients is gaining attention. Understanding the extent of antibiotic resistance in these populations is critical for designing targeted interventions.
Objective:
The objective of this systematic review and meta-analysis was to determine the prevalence of antibiotic resistance in older adults and AD patients with a focus on quantitative studies in order to provide comprehensive insights into the current landscape.
Methods:
To identify relevant studies, we conducted a thorough search of the PubMed, Scopus, CINAHL, and Web of Science databases. Only studies involving adults and AD patients, published in English, and reporting quantitative data on antibiotic resistance prevalence were considered. The Risk of Bias In Non-randomized Studies of Interventions (ROBINS-I) tool was used to assess quality. The data was summarized by using Revman 5.4.1.
Results:
A total of six studies met the final criteria for selection and results from the meta-analysis found a pooled prevalence odds ratio of OR = 1.27 (95% CI: [0.99, 1.63], Z = 1.87, p = 0.06). The studies showed significant heterogeneity (I2 = 100%, p < 0.00001), emphasizing the need for cautious interpretation.
Conclusions:
The findings indicate a potential trend of increased antibiotic resistance in older adults and AD patients, though statistical significance was not achieved for both. The significant heterogeneity highlights the complexity of resistance patterns in these populations, necessitating additional research for tailored interventions.
INTRODUCTION
Antibiotics have transformed modern medicine in the treatment of bacterial infections;1 however, the emergence and spread of antibiotic resistance (AR) has become a global health concern,2,3 highlighting the critical need to address it.4 The ability for bacteria to resist the effects of an antibiotic to which they were previously susceptible5–7 or evolve and develop the mechanisms to withstand exposure to drugs (antibiotics) designed to kill them, renders medication efforts ineffective.8,9 According to estimates, AR was responsible for nearly 5 million deaths in 2019 worldwide.10 If appropriate measures are not implemented, it is anticipated that this number will increase to between 10 and 44 million by the year 2050.1,6,11 Over 2.8 million cases of antibiotic-resistant infections happen each year in the United States, leading to more than 35,000 deaths.12 The annual cost of resistant infections is estimated to be $20 billion in excess health-care costs in the United States,7 accounting for over 30% of antimicrobials hospital pharmacy budgets. In the European Union, the cost is over 1.6 billion euros.10
Risk factors which contribute to the development and spread of AR, include but not limited to inappropriate use of antibiotics, inadequate infection prevention and control, suboptimal sanitary conditions,7,11,12 and conditions that necessitate frequent use of antibiotics, such as diabetes.13–15 It is therefore crucial to investigate the implications for vulnerable populations with coexisting conditions, particularly those with neurodegenerative disorders with dementia such as Alzheimer’s disease (AD).13–19
Millions of AD patients worldwide are vulnerable to a variety of health issues,20,21 and the combination of AR increases their vulnerability.22 Complications such as increased infection severity, longer hospitalizations, higher healthcare costs, and compromised treatment outcomes are significant challenges for AD patients with AR.23 The challenges extend beyond just medical issues, encompassing significant socioeconomic burdens.4 Medical complexities refer to the intricate interplay of managing both AR and AD, which can complicate treatment plans and patient care. Socioeconomic burdens include the increased healthcare costs, the need for specialized care, and the impact on the quality of life for both patients and caregivers. Addressing these factors is essential to improve patient outcomes and provide effective, comprehensive care.4
Existing studies report varying levels of AR in AD patients, with distinct incidence rates across regions: 15% in Africa, 19.38% in Europe.17,21,24–27 However, no comprehensive review exists on AR prevalence in AD patients, especially those with co-morbid diabetes, a known AR risk factor due to its impact on immune responses.22 Region-specific measures are crucial: Africa could focus on improving antibiotic stewardship and infection control, given its lower rate, while Europe might need enhanced screening and targeted antibiotic protocols to address higher prevalence. Such tailored approaches could effectively reduce14,18,19 AR in these vulnerable populations.28–30 Moreover there is not a comprehensive research on the impact of AR on treatment outcomes in AD patients14,18,19 creating a knowledge gap which necessitates a systematic review and meta-analysis.31,32 To this end, our current review aims to investigate the prevalence of AR in older adults and AD patients with the goal to inform and provide some comprehensive healthcare approaches and interventions that are tailored to the specific needs of these groups.
METHODS
A structured PRISMA reporting process was followed.33
Eligibility criteria
The PEO framework is a structured approach to developing research questions and defining eligibility criteria for systematic reviews and meta-analyses.34 PEO stands for Population, Exposure, and Outcome. Table 1 outlines the eligibility criteria for this systematic review based on the PEO framework and provides a clear rationale for each criterion to help guide the study selection process.
Table 1
Eligibility criteria
Criteria | Inclusion criteria | Exclusion criteria |
Population (P) | Older adults and AD patients | Patients without AD with other co-morbidities |
Exposure (E) | Antibiotic resistance | Studies examining other health conditions or interventions |
Outcome (O) | Prevalence of antibiotic resistance in the specified population | Studies not reporting prevalence of antibiotic resistance |
Design | Observational studies (cross-sectional, cohort), randomized control trials, quasi-experimental studies, controlled designs | Qualitative studies, systematic review designs, narrative reviews, and commentaries |
Publication Type | Published studies | Preprints |
Language | English studies | Non-English |
Search strategy
The search strategy for this systematic review was developed using the PEO framework (Table 2). Key components include population (AD patients), exposure (antibiotic resistance), and outcome (prevalence of antibiotic resistance).34 Medical Subject Headings was used to supplement the primary keyword,35,36 and account for spelling variations. The primary keywords included “Alzheimer’s disease”, “older adults”, “antibiotic resistance,” and “prevalence”. Boolean operators such as AND, OR, and NOT were used to combine or exclude specific terms, thereby improving the search strategy.37 Wildcards, brackets, and truncation were used as additional search strings to prevalence of antibiotic resistance in AD patients ensuring a thorough and exhaustive review of the available evidence.38
Table 2
Search strategy
S /N | Primary keywords | Joining type | MESH Terms and Free Text with Boolean Operators | Databases |
1 | Patients with Alzheimer’s disease | OR | ((“Patients with Alzheimer*”) OR (“Alzheimer Disease”) OR (“Alzheimer’s Disease”) OR (Alzheimer*) OR (Patient*) OR (“patient*, Alzheimer*”) OR (“Dementia, Alzheimer’s Type”) OR (“Early-Onset Alzheimer’s Disease”) OR (“Late-Onset Alzheimer’s Disease”) OR (“Senile Dementia”) OR (“Alzheimer’s, Presenile”)) OR (“older adults”) OR (“elderly patients”) OR (“elderly population”). | All Databases |
AND | ||||
2 | Antibiotic resistance | OR | ((“Antibiotic resistance”) OR (“Drug Resistance, Bacterial”) OR (“Antibiotics Resistance”) OR (“Drug Resistant Bacteria”) OR (“Antibiotics, bacteria, resistant”) OR (“Multidrug Resistance”) OR (“Antibiotic Resistant Infections”) OR (“Antimicrobial Resistance”) OR (“Resistance, Antibiotic”) OR (“Antimicrobial resistance”) OR (“Antimicrobial agent”) OR (“Antimicrobial drugs”) OR (“Antimicrobial therapy”) OR (“Antimicrobial treatment”) OR (“Antibacterial resistance”) OR (“Antibacterial agent”) OR (“Antibacterial drugs”) OR (“Antibacterial therapy”) OR (“Antibacterial treatment”) OR (“Tetracyclines”) OR (“Amphenicols”) OR (“Beta-lactam Antibacterials, Penicillins”) OR (“Other Beta-lactam Antibacterials”) OR (“Sulfonamides and Trimethoprim”) OR (“Macrolides, Lincosamides, and Streptogramins”) OR (“Aminoglycosides”) OR (“Quinolone Antibacterials”) OR (“Combinations of Antibacterials”) OR (“Other Antibacterials”)) | All Databases |
AND | ||||
3 | Prevalence | OR | ((Prevalence*) OR (Prevalence) OR (rate) OR (“prevalent* rate”) OR (Incidence) OR (“Epidemiology”) OR (“Occurrence”) OR (“Frequency”) OR (“Distribution”)) | All Databases |
The databases used were PubMed, Scopus, CINAHL, and Web of Science.
Study selection
The study selection process for this review began with the essential step of de-duplication, which was meticulously performed using the Zotero reference manager to ensure that each unique record was considered for inclusion.39 After de-duplication, a systematic screening process was employed.40 Initially, titles and abstracts were reviewed to identify potentially relevant studies based on predefined eligibility criteria, using the PEO framework. Revision was performed on the full text of the study articles and this was thoroughly done ensuring that they met the inclusion criteria and to extract relevant data.40–42
Data extraction
To organize the data for this systematic review, references were transferred from Zotero to an Excel spreadsheet.43–45 The full-text articles were then used to extract comprehensive characteristics about the included studies. These included author names, publication year, study design, participant demographics, interventions or exposures related to antibiotic resistance, outcome measures (prevalence of antibiotic resistance), and relevant findings. Furthermore, information about the study’s duration, geographic location, sample size, and any statistical methods used for data analysis were systematically documented. The extraction also included information about the publication type, ensuring that only published studies were considered in the analysis.46
Quality assessment
The quality assessment,47,48 also known as risk of bias evaluation,49 utilized the ROBINS-I tool (“Risk Of Bias In Non-randomized Studies - of Interventions”). The tool covers various important areas such as confounding variables, participant selection, intervention classification, deviations from intended interventions, missing data, outcome measurement, and selection of reported results. This was necessary as all the studies were non-randomized, and the ROBIN-I scale was considered the most suitable. This tool for quality assessment sought to provide a comprehensive evaluation of the internal validity of the studies, thereby contributing to the overall rigor of the systematic review.49
Data synthesis
Based on the method of data synthesis, a meta-analysis approach was adopted random effect meta-analysis approach for quantitative synthesis was carried out for this review using Review Manager [RevMan] Software 5.4.1.44,46,50–53 The odds ratio and prevalence data were extracted from the studies. This method accounted for both within-study and between-study variability, yielding a more conservative estimate of the overall effect size.54 The I2 statistic was used to assess study heterogeneity, with values greater than 50% indicating significant heterogeneity.55–57 Also, Forest plots were generated to illustrate the results.
RESULTS
A total of 1,203 studies were found following search from the databases, 272 studies were screened for full text assessment and at the end 6 studies met the full eligibility criteria for selection (Fig. 1).
Fig. 1
PRISMA flow diagram illustrating the study selection process.
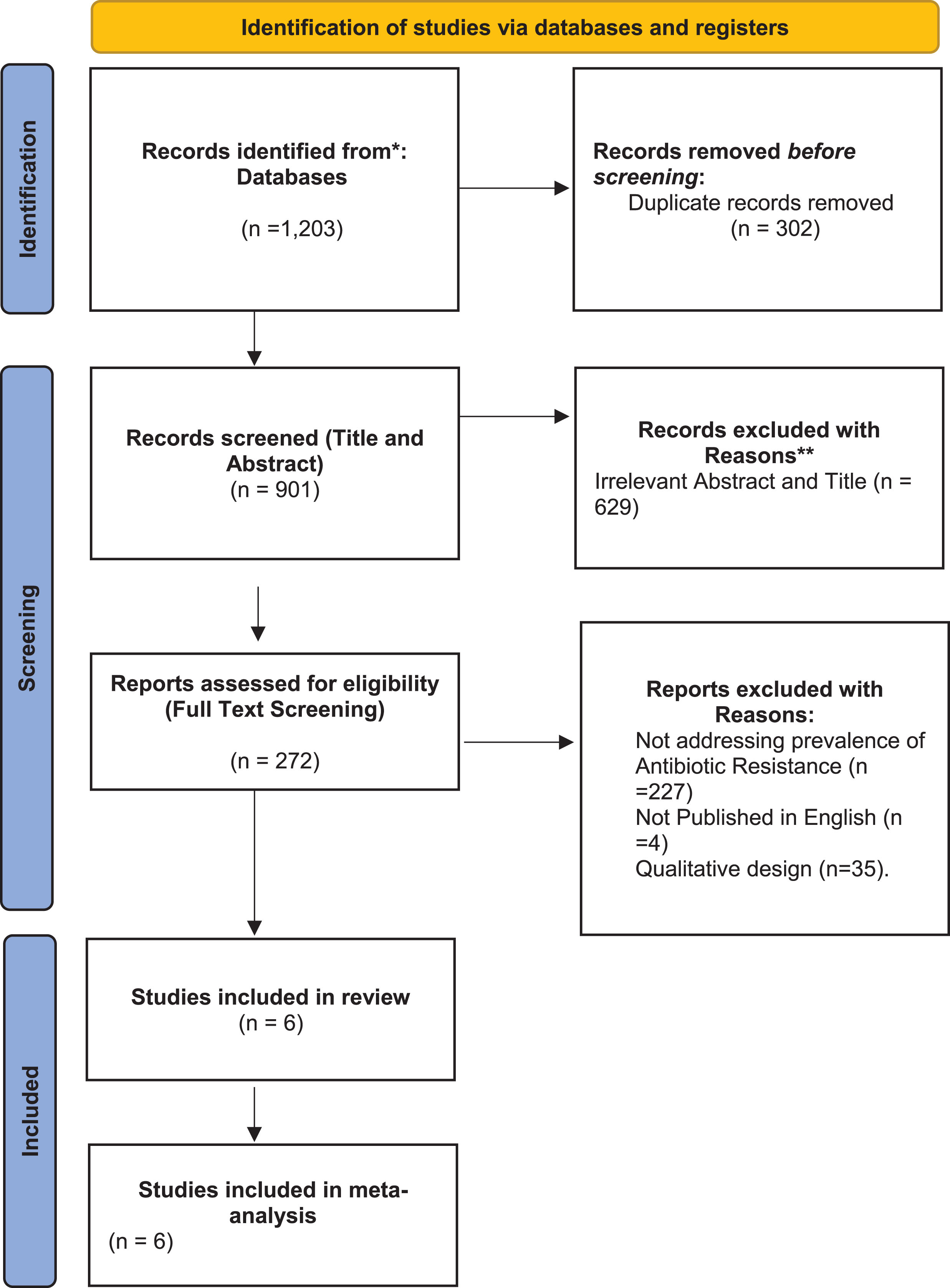
Characteristics of the included studies
A total of six studies were included in this review.58–63 Table 3 outlines the characteristics of the studies. The included studies, while diverse in their objectives and methods, share a focus on antibiotic resistance and its implications in various populations and settings. They encompass a range of study designs, from retrospective analyses (Weber et al., Washio et al.) to prospective evaluations (Eveillard et al., Fabiszewski et al.) and case-control studies (Wiener et al.). Sample sizes vary significantly, from small groups (Eveillard et al. with 35 patients) to large datasets (Weber et al. with 55,427 isolates). Key similarities include the identification of high antibiotic resistance prevalence, with MRSA and ESBL-producing bacteria being common focal points. Differences arise in participant characteristics, such as age groups (older versus younger adults, nursing home residents, AD patients), and in specific outcomes, like case fatality rates and treatment impacts. Limitations are consistently noted, including narrow population scopes and the challenge of establishing causation. Geographic and institutional contexts also vary, affecting the generalizability of findings. These studies collectively underscore the complexity of antibiotic resistance and the need for tailored interventions based on specific patient demographics and healthcare settings.
Table 3
Characteristics of the included studies
Study | Author publication date | Research objective | Research design &methods | Sample size or participant characteristics | Prevalence of antibiotic resistance | Outcome measures and findings | Location of study | Limitations |
1 | Weber et al. (2009)62 | Compare antimicrobial resistance in older and younger adults | Retrospective analysis; Two-centre study | 55,427 isolates between 1999–2005 | 11.82% | Heterogeneity observed; No uniform age-resistance association | Maryland and Chicago | Results vary by bacteria, age, and location; Temporal trends not specified |
2 | Eveillard et al. (2002)61 | Estimate MRSA prevalence at admission | Prospective screening; Logistic regression analysis | 35 patients; Demographics, clinical, and therapeutic data recorded | 14.6% MRSA carriage; Associated with recent hospitalization and wounds | High MRSA prevalence at admission; Selective screening recommended | French teaching hospital | Limited to acute geriatric wards; Association, not causation |
3 | Washio et al. (1997)59 | Evaluate factors influencing MRSA case fatality rate | Retrospective study; Univariate and multivariate analysis | 49 elderly patients with MRSA infection | 67.3% case fatality rate; Risk factors: male sex, hypoalbuminemia, excessive antibiotic usage | Case fatality rate may be high; Limited to a geriatric hospital | NR | NR |
4 | Wiener et al. (1999)60 | Report nursing home outbreak of ESBL-producing gram-negative bacilli | Case-control study; Point-prevalence survey | 55 patients; Clinical and molecular epidemiology | 9.02% | Nursing home patients important reservoir; Common antibiotic resistance plasmid | Tertiary care hospital and community nursing home | Broad-spectrum antibiotics and poor infection control practices may facilitate spread |
5 | Fabiszewski et al. (1990)58 | Impact of antibiotic treatment on fever outcomes in AD patients | Prospective evaluation; Survival analysis | 104 institutionalized AD patients | 11.02% | Antibiotic treatment does not alter fever outcome | NR | Limited to Alzheimer patients; Survival differences observed in less severely affected patients |
6 | Bello-Medina et al. (2022)63 | Effect of BGM dysbiosis on AD patients | Preclinical study on 3xTg mice; Antibiotics induced BGM dysbiosis | 102 patients | 29.39% | Delay in spatial memory impairment and Aβ deposits; Correlated with bacterial abundance and alpha diversity | NR | Specific BGM effects may not be universally applicable |
NR, not reported.
Quality assessment
The ROBINS-I tool assesses the risk of bias in non-randomized studies of interventions. Each study is evaluated across different domains to determine the overall risk of bias. The several domains used were: 1) Bias due to confounding, which evaluates whether the effect of interest is mixed with other factors, 2) Bias in selection of participants, which examines whether the participants included in the study are representative, 3) Bias in classification of interventions, addressing potential misclassification of intervention status, 4) Bias due to deviations from intended interventions, assessing whether deviations from the planned interventions have occurred, 5) Bias due to missing data and in measurement of outcomes, evaluating whether the outcomes are measured appropriately. These domains collectively help in evaluating the overall risk of bias in non-randomized studies. For studies with Low: it implied the study has a low risk of bias across all domains. For moderate: the study has some concerns regarding bias, but the overall risk is moderate. And for High: the study has a high risk of bias, indicating significant concerns across multiple domains. Figure 2 illustrates the Risk of bias across studies.
Fig. 2
Risk of bias across the studies.

Meta-analysis of prevalence of AR in older adults and AD
A total of six studies were included in the meta-analysis of pooled prevalence of antibiotic resistance in older adults and AD patients.58–63 Results from the study revealed as shown in Fig. 3 that the pooled prevalence was (OR = 1.27; 95% C.I: [0.99, 1.63] Z = 1.87, p = 0.06). Significant heterogeneity was observed among the studies (I2 = 100%, p < 0.00001).
Fig. 3
Forest plot demonstrating the pooled prevalence of antibiotic resistance in older adults and Alzheimer’s disease patients.
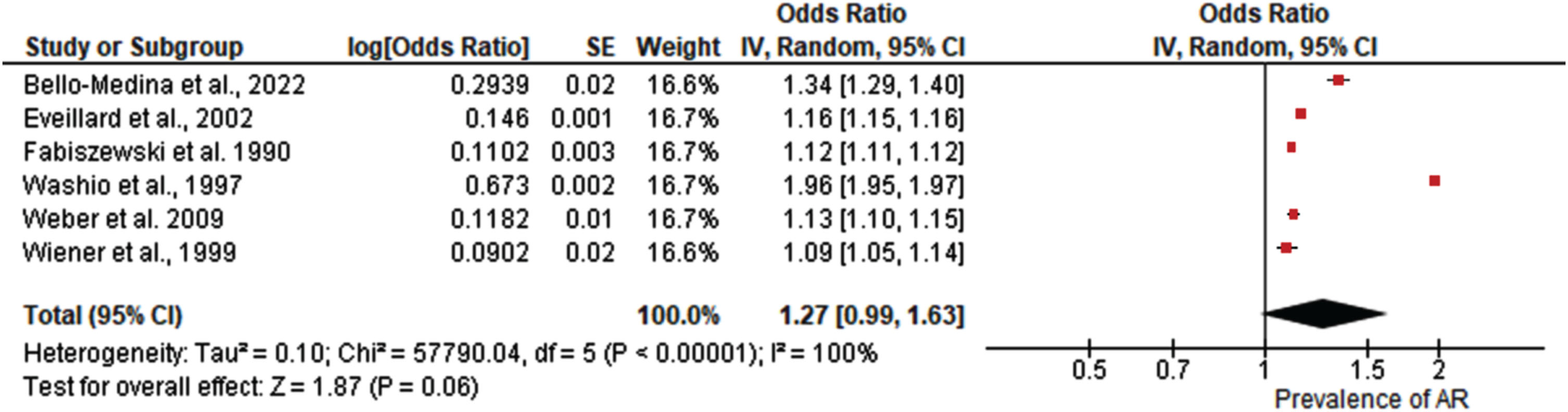
DISCUSSION
The objective of this systematic review and meta-analysis was to ascertain the prevalence of antibiotic resistance in older adults and AD patients. A meta-analysis of six selected studies found a pooled prevalence odds ratio (OR) of 1.27 (95% CI: [0.99, 1.63], Z = 1.87, P = 0.06), indicating a possible trend toward increased antibiotic resistance, though statistical significance was not reached. This analysis revealed significant heterogeneity among the studies (I2=100%, p < 0.00001), indicating substantial variation in methodologies and participant characteristics. This high level of heterogeneity necessitates careful interpretation of the results, as the differences between studies can influence the overall findings. Heterogeneity in a meta-analysis often arises from variations in study design, population demographics, intervention types, and outcome measurements.14 Such diversity can obscure true effects and complicate the synthesis of findings. For instance, studies may differ in their definitions of antibiotic resistance, the diagnostic criteria for AD, or the healthcaresettings in which data were collected.6,19,22
Discussing the effects of this heterogeneity is crucial, as it impacts the robustness and applicability of the meta-analysis results. Differences and similarities between studies were explored to understand the sources of variation and their implications and this variations in resistance trends across bacterial species, geographical locations, and healthcare settings highlight the need for targeted, context-specific interventions were seen. Comparing these findings with existing literature reveals key trends in antibiotic resistance, but the relevance to the current study’s results was noted.12 The variability underscores the necessity for nuanced strategies in combating antibiotic resistance, tailored to specific contexts. The authors however emphasize how their results align with or diverge from established literature, reinforcing the importance of customized interventions in addressing the global challenge of antibiotic resistance.33,39 This would provide a unifying theme and demonstrate the significance of their meta-analysis in the broader context of existing research. Paczosa and Mecsas discuss Klebsiella pneumoniae’s defensive mechanisms, emphasizing the changing nature of bacterial resistance strategies.64 Reyes et al. offer microbiological insights into carbapenem-resistant K. pneumoniae, emphasizing the clinical significance of antibiotic resistance.65 Zhang et al. emphasize the urgent need for antimicrobial stewardship in tertiary hospitals, which is consistent with the broader concerns raised in the systematic review.66 Li et al. describes a rapid increase in the prevalence of carbapenem-resistant Enterobacteriaceae (CRE) and the emergence of colistin resistance in a Chinese hospital, highlighting a global concern echoed in the meta-analysis.67 Park et al. present a downward trend in carbapenem-resistant K. pneumoniae infections in New York City hospitals, which contrasts with the potential trend suggested in the current review.68 Also, Morgenstern et al. investigate antibiotic resistance in commensal Staphylococcus aureus, providing a more comprehensive understanding of resistance patterns beyond Enterobacteriaceae.69 Kajumbula et al. in Lacor Hospital, Uganda, and Chen et al. in a regional burn center in China investigated antibiotic susceptibility in various healthcare settings.70,71 Also, Brooks and Mias investigate Streptococcus pneumoniae virulence and host immunity, addressing the larger context of bacterial infections and antibiotic resistance.72 However, while the systematic review provides useful insights into antibiotic resistance in older adults and AD patients, a critical comparison to existing literature highlights the complex and multifaceted nature of antibiotic resistance patterns. The variability in resistance trends across bacterial species, geographical locations, and healthcare settings emphasizes the need for targeted and context-specific interventions in the global fight against antibiotic resistance. Our current review, with its acknowledgement of significant heterogeneity, calls for additional research to identify the specific factors influencing resistance prevalence in these vulnerable populations.
This meta-analysis, which uncovered a potential trend of increased antibiotic resistance in older adults and AD patients, has important implications for clinical and public health practices. The observed heterogeneity among studies emphasizes the importance of tailoring interventions to the various contexts that influence resistance patterns. These findings highlight the importance of judicious antibiotic use in clinical settings, emphasizing the need for individualized treatment regimens and regular resistance pattern surveillance. Antibiotic resistance is a multifaceted issue that requires public health policies to integrate infection prevention, antimicrobial stewardship, and surveillance strategies. Because vulnerable populations, such as older adults with comorbidities, are especially vulnerable to the effects of antibiotic-resistant infections, these findings call for comprehensive public health measures to prevent the emergence and spread of resistant strains. Continuous monitoring, education, and collaboration among healthcare providers, researchers, and policymakers are critical in developing effective strategies to combat antibiotic resistance and protect the health of these communities.
Despite contributing valuable insights, our meta-analysis has certain limitations. Firstly, the availability of a limited number of studies on the topic restricted our ability to conduct a more extensive and robust analysis. The scarcity of data poses a challenge in establishing a comprehensive understanding of the antibiotic resistance landscape in older adults and AD patients. Furthermore, the substantial heterogeneity observed across the included studies raises concerns about the consistency of the findings. Variations in methodologies, participant demographics, and regional factors may have introduced confounding variables, affecting the reliability of the overall pooled estimate. Additionally, the lack of standardized reporting across studies may have impacted the accuracy and comparability of the results. As such, these limitations underscore the need for more well-designed and standardized research in this field to enhance the reliability and generalizability of future meta-analyses on antibiotic resistance in the context of older adults and AD patients.
Conclusions
In conclusion, our meta-analysis of the prevalence of antibiotic resistance in older adults and AD patients revealed a complex landscape with a suggestive but statistically insignificant trend toward increased resistance. The significant heterogeneity among the included studies highlights the importance of exercising caution when interpreting the overall pooled estimate, taking into account different methodologies and participant characteristics. The implications of these findings highlight the complexities of antibiotic resistance among vulnerable populations. Clinical practices should prioritize individualized treatment strategies and antimicrobial stewardship, while public health policies should implement comprehensive measures to address the multifaceted nature of resistance. Further research is needed to identify the specific factors influencing resistance patterns in these populations, allowing for the development of targeted interventions. This study adds to the ongoing discussion about antibiotic resistance by emphasizing the importance of collaborative efforts in clinical and public health settings to reduce the emergence and spread of antibiotic-resistant strains among older adults and ADpatients.
AUTHOR CONTRIBUTIONS
Namra Vinay Gohil (Conceptualization; Data curation; Writing – original draft; Writing – review & editing); Fabio Fuentes Gandara (Conceptualization; Data curation; Writing – original draft; Writing – review & editing); Harshal Gohil (Conceptualization; Data curation; Writing – original draft; Writing – review & editing); Swathi Gurajala (Conceptualization; Data curation; Writing – original draft; Writing – review & editing); David Chinaecherem Innocent (Conceptualization; Data curation; Writing – original draft; Writing – review & editing); Tadele Tesfaye (Conceptualization; Data curation; Writing – original draft; Writing – review & editing); Domenico Pratico (Conceptualization; Writing – review & editing).
ACKNOWLEDGMENTS
Domenico Praticò is the Scott Richards North Star Charitable Foundation Chair for Alzheimer’s disease research.
FUNDING
The authors have no funding to report.
CONFLICT OF INTEREST
The authors have no conflict of interest to report.
DATA AVAILABILITY
Data sharing is not applicable to this article as no datasets were generated or analyzed during this study.
REFERENCES
1. | Smith E and Buchan S. Skewed perception of personal behaviour as a contributor to antibiotic resistance and underestimation of the risks. Plos One (2023) ; 18: : e0293186. |
2. | World Health Organization. Global action plan on antimicrobial resistance, https://iris.who.int/bitstream/handle/10665/193736/9789241509763_eng.pdf. 2015 (accessed February 2024). |
3. | World Health Organization. Antibiotic resistance: multi-country public awareness survey. https://iris.who.int/bitstream/handle/10665/194460/9789241509817_eng.pdf, 2015 (accessed February 2024). |
4. | O’Neill J . Tackling drug-resistant infections globally: final report and recommendations. Review on Antimicrobial Resistance (2016) ; 1–84. |
5. | Kuehn BM . CDC establishes global networks to combat antimicrobial resistance. JAMA (2022) ; 327: : 315–315. |
6. | Church NA and McKillip JL. Antibiotic resistance crisis: challenges and imperatives. Biologia (Bratisl) (2021) ; 76: : 1535–1550. |
7. | Kadri SS . Key takeaways from the US CDC’s 2019 antibiotic resistance threats report for frontline providers. Crit Care Med (2020) ; 48: : 939–945. |
8. | Christaki E , Marcou M and Tofarides A. Antimicrobial resistance in bacteria: mechanisms, evolution, and persistence. J Mol Evol (2020) ; 88: : 26–40. |
9. | Fymat AL . Antibiotics and antibiotic resistance. Biomed J Sci Tech Res (2017) ; 1: : 1–16. |
10. | Fair RJ and Tor Y. Antibiotics and bacterial resistance in the 21st century. Perspect Med Chem (2014) ; 6: : 25–64. |
11. | Llor C and Bjerrum L. Antimicrobial resistance: risk associated with antibiotic overuse and initiatives to reduce the problem. Ther Adv Drug Saf (2014) ; 5: : 229–241. |
12. | Laxminarayan R , Duse A , Wattal C , et al. Antibiotic resistance—the need for global solutions. Lancet Infect Dis (2013) ; 13: : 1057–1098. |
13. | Scheiblich H , Trombly M , Ramirez A , et al. Neuroimmune connections in aging and neurodegenerative diseases. Trends Immunol (2020) ; 41: : 300–312. |
14. | Tahami Monfared AA , Byrnes MJ , White LA , et al. Alzheimer’s disease: epidemiology and clinical progression. Neurol Ther (2022) ; 11: : 553–569. |
15. | M Shaik M , Ahmad S , H Gan S , et al. How do periodontal infections affect the onset and progression of Alzheimer’s disease? CNS Neurol Disord-Drug Targets (2014) ; 13: : 460–466. |
16. | DeMaio A , Mehrotra S , Sambamurti K , et al. The role of the adaptive immune system and T cell dysfunction in neurodegenerative diseases. J Neuroinflammation (2022) ; 19: : 251. |
17. | Giridharan VV , Masud F , Petronilho F , et al. Infection-induced systemic inflammation is a potential driver of Alzheimer’s disease progression. Front Aging Neurosci (2019) ; 11: : 122. |
18. | Heneka MT , Carson MJ , El Khoury J , et al. Neuroinflammation in Alzheimer’s disease. Lancet Neurol (2015) ; 14: : 388–405. |
19. | Shinjyo N and Kita K. Infection and immunometabolism in the central nervous system: a possible mechanistic link between metabolic imbalance and dementia. Front Cell Neurosci (2021) ; 15: : 765217. |
20. | Bhushan I , Kour M , Kour G , et al. Alzheimer’s disease: causes & treatment–A review. Ann Biotechnol (2018) ; 1: : 1002. |
21. | Mitchell SL , Shaffer ML , Kiely DK , et al. The study of pathogen resistance and antimicrobial use in dementia: study design and methodology. Arch Gerontol Geriatr (2013) ; 56: : 16–22. |
22. | Kamer AR , Dasanayake AP , Craig RG , et al. Alzheimer’s disease and peripheral infections: the possible contribution from periodontal infections, model and hypothesis. J Alzheimers Dis (2008) ; 13: : 437–449. |
23. | Zhang Z , Yu P , Chang HC , et al. Developing an ontology for representing the domain knowledge specific to non-pharmacological treatment for agitation in dementia. Alzheimers Dement (N Y) (2020) ; 6: : e12061. |
24. | Mitchell SL , D’Agata EM , Hanson LC , et al. The trial to reduce antimicrobial use in nursing home residents with Alzheimer disease and other dementias (TRAIN-AD): a cluster randomized clinical trial. JAMA Intern Med (2021) ; 181: : 1174–1182. |
25. | Li F , Hearn M , Bennett LE . The role of microbial infection in the pathogenesis of Alzheimer’s disease and the opportunity for protection by anti-microbial peptides. Crit Rev Microbiol (2021) ; 47: : 240–253. |
26. | Itzhaki RF , Lathe R , Balin BJ , et al. Microbes and Alzheimer’s disease. J Alzheimers Dis (2016) ; 51: : 979–984. |
27. | Javaid SF , Giebel C , Khan MA , et al. Epidemiology of Alzheimer’s disease and other dementias: Rising global burden and forecasted trends. FRes (2021) ; 10: : 425. |
28. | Carrillo-Larco RM , Anza-Ramírez C , Saal-Zapata G , et al. Type 2 diabetes mellitus and antibiotic-resistant infections: a systematic review and meta-analysis. J Epidemiol Community Health (2022) ; 76: : 75–84. |
29. | Akash MSH , Rehman K , Fiayyaz F , et al. Diabetes-associated infections: development of antimicrobial resistance and possible treatment strategies. Arch Microbiol (2020) ; 202: : 953–965. |
30. | Nunez SA , Lacal V , Nunez J , et al. Antibiotic resistance in community-acquired intra-abdominal infections: diabetes mellitus as a risk factor. Surg Infect (2020) ; 21: : 62–68. |
31. | Panza F , Lozupone M , Solfrizzi V , et al. Time to test antibacterial therapy in Alzheimer’s disease. Brain (2019) ; 142: : 2905–2929. |
32. | Moir RD , Lathe R , Tanzi RE . The antimicrobial protection hypothesis of Alzheimer’s disease. Alzheimers Dement (2018) ; 14: : 1602–1614. |
33. | Shamseer L , Moher D , Clarke M , et al. Preferred reporting items for systematic review and meta-analysis protocols (PRISMA-P) 2015: elaboration and explanation. Br Med J (2015) ; 349: : g7647. |
34. | Martinez EC , Valdés JRF , Castillo JL , et al. Ten steps to conduct a systematic review. Cureus (2023) ; 15: : e51422. |
35. | Ho GJ , Liew SM , Ng CJ , et al. Development of a search strategy for an evidence-based retrieval service. PloS One (2016) ; 11: : e0167170. |
36. | Rethlefsen ML , Murad MH , Livingston EH . Engaging medical librarians to improve the quality of review articles. JAMA (2014) ; 312: : 999–1000. |
37. | Haig A and Dozier M. BEME guide no. 3: systematic searching for evidence in medical education–part 2: constructing searches. Med Teach (2003) ; 25: : 463–484. |
38. | Dickersin K , Scherer R , Lefebvre C . Systematic reviews: identifying relevant studies for systematic reviews. Br Med J (1994) ; 309: : 1286–1291. |
39. | Glassman NR . Using citation managers to provide library services. In: Glassman NR (ed) Citation Management Tools: A Practical Guide for Librarians. Lanham, MD: Rowman & Littlefield Eds, (2018) , pp. 53–133. |
40. | Karakan B . Tool support for systematic literature reviews: analyzing existing solutions and the potential for automation. University of Stuttgart, Institute of Software Technology. Thesis (2021) , pp. 1–34. |
41. | Isidoro-Cabañas E , Soto-Rodríguez FJ , Morales-Rodríguez FM , et al. Benefits of adaptive sport on physical and mental quality of life in people with physical disabilities: a meta-analysis. Healthcare (Basel) (2023) ; 11: : 2480. |
42. | Page MJ , Moher D , Bossuyt PM , et al. PRISMA 2020 explanation and elaboration: updated guidance and exemplars for reporting systematic reviews. Br Med J (2021) ; 372: : 60. |
43. | Guimarães NS , Ferreira AJ , Silva R de CR , et al. Deduplicating records in systematic reviews: There are free, accurate automated ways to do so. J Clin Epidemiol (2022) ; 152: : 110–115. |
44. | Han H , Xu H , Chen H . Social commerce: a systematic review and data synthesis. Electron Commer Res Appl (2018) ; 30: : 38–50. |
45. | Lorenzetti DL and Ghali WA. Reference management software for systematic reviews and meta-analyses: an exploration of usage and usability. BMC Med Res Methodol (2013) ; 13: : 141. |
46. | Munn Z , Tufanaru C and Aromataris E. JBI’s systematic reviews: data extraction and synthesis. Am J Nurs (2014) ; 114: : 49–54. |
47. | Viswanathan M , Patnode CD , Berkman ND , et al. Recommendations for assessing the risk of bias in systematic reviews of health-care interventions. J Clin Epidemiol (2018) ; 97: : 26–34. |
48. | Ma LL , Wang YY , Yang ZH , et al. Methodological quality (risk of bias) assessment tools for primary and secondary medical studies: what are they and which is better? Mil Med Res (2020) ; 7: : 7. |
49. | Higgins JP , Altman DG , Gøtzsche PC , et al. The Cochrane Collaboration’s tool for assessing risk of bias in randomised trials. Br Med J (2011) ; 343: : d5928. |
50. | Barnett-Page E and Thomas J. Methods for the synthesis of qualitative research: a critical review. BMC Med Res Methodol (2009) ; 9: : 59. |
51. | Coster JE , Turner JK , Bradbury D , et al. Why do people choose emergency and urgent care services? A rapid review utilizing a systematic literature search and narrative synthesis. Acad Emerg Med (2017) ; 24: : 1137–1149. |
52. | Haddaway NR and Rytwinski T. Meta-analysis is not an exact science: Call for guidance on quantitative synthesis decisions. Environ Int (2018) ; 114: : 357–359. |
53. | Lau J , Ioannidis JP and Schmid CH. Quantitative synthesis in systematic reviews. Ann Intern Med (1999) ; 127: : 820–826. |
54. | Gurevitch J , Koricheva J , Nakagawa S , et al. Meta-analysis and the science of research synthesis. Nature (2018) ; 555: : 175–182. |
55. | von Hippel PT . The heterogeneity statistic I2 can be biased in small meta-analyses. BMC Med Res Methodol (2015) ; 15: : 35. |
56. | Melsen W , Bootsma M , Rovers M , et al. The effects of clinical and statistical heterogeneity on the predictive values of results from meta-analyses. Clin Microbiol Infect (2014) ; 20: : 123–129. |
57. | Deeks JJ , Higgins JP , Altman DG . Analysing data and undertaking meta-analyses. In: Higgins JPT, Thomas J, Chandler J, et al. (eds) Cochrane Handbook for Systematic Reviews of Interventions. New York: Wiley Online Library, (2019) , pp. 241–284. |
58. | Fabiszewski KJ , Volicer B and Volicer L. Effect of antibiotic treatment on outcome of fevers in institutionalized Alzheimer patients. JAMA (1990) ; 263: : 3168–3172. |
59. | Washio M , Kiyohara C , Hamada T , et al. The case fatality rate of methicillin-resistant Staphylococcus aureus (MRSA) infection among the elderly in a geriatric hospital and their risk factors. Tohoku J Exp Med (1997) ; 183: : 75–82. |
60. | Wiener J , Quinn JP , Bradford PA , et al. Multiple antibiotic–resistant Klebsiella and Escherichia coli in nursing homes. JAMA (1999) ; 281: : 517–523. |
61. | Eveillard M , Ernst C , Cuviller S , et al. Prevalence of methicillin-resistant Staphylococcus aureus carriage at the time of admission in two acute geriatric wards. J Hosp Infect (2002) ; 50: : 122–126. |
62. | Weber SG , Miller RR , Perencevich EN , et al. Prevalence of antimicrobial-resistant bacteria isolated from older versus younger hospitalized adults: results of a two-centre study. J Antimicrob Chemother (2009) ; 64: : 1291–1298. |
63. | Bello-Medina PC , Corona-Cervantes K , Zavala Torres NG , et al. Chronic-antibiotics induced Gut microbiota dysbiosis rescues memory impairment and reduces β-amyloid aggregation in a preclinical Alzheimer’s disease model. Int J Mol Sci (2022) ; 23: : 8209. |
64. | Paczosa MK and Mecsas J. Klebsiella pneumoniae: going on the offense with a strong defense. Abstr Gen Meet Am Soc Microbiol (2016) ; 80: : 629–661. |
65. | Reyes J , Aguilar AC and Caicedo A. Carbapenem-resistant Klebsiella pneumoniae: microbiology key points for clinical practice. Int J Gen Med (2019) ; 12: : 437–446. |
66. | Zhang D , Cui K , Lu W , et al. Evaluation of carbapenem use in a tertiary hospital: antimicrobial stewardship urgently needed. Antimicrob Resist Infect Control (2019) ; 8: : 5. |
67. | Li Y , Sun Q-L , Shen Y , et al. Rapid increase in prevalence of carbapenem-resistant Enterobacteriaceae (CRE) and emergence of colistin resistance gene mcr-1 in CRE in a hospital in Henan, China. J Clin Microbiol (2018) ; 56: : e01932–17. |
68. | Park SO , Liu J , Furuya EY , et al. Carbapenem-resistant Klebsiella pneumoniae infection in three New York city hospitals trended downwards from 2006 to 2014 Open Forum Infect Dis (2016) ; 3: : ofw222. |
69. | Morgenstern M , Erichsen C , Hackl S , et al. Antibiotic resistance of commensal Staphylococcus aureus and coagulase-negative staphylococci in an international cohort of surgeons: a prospective point-prevalence study. PLoS One (2016) ; 11: : e0148437. |
70. | Ld K , Ad A , Acullu D , et al. Antibiotic susceptibility of Staphylococcus aureus in suppurative lesions in Lacor Hospital, Uganda. Afr Health Sci (2011) ; 11: : S34–S39. |
71. | Chen K , Lin S , Li P , et al. Characterization of Staphylococcus aureus isolated from patients with burns in a regional burn center, Southeastern China. BMC Infect Dis (2018) ; 18: : 51. |
72. | Brooks LRK and Mias GI. Streptococcus pneumoniae’s virulence and host immunity: aging, diagnostics, and prevention. Front Immunol (2018) ; 9: : 1366. |