Human Brain Single Nucleotide Polymorphism: Validation of DNA Sequencing
Abstract
Genetic factors may be involved in the onset of neurodegenerative diseases like Alzheimer’s disease. In the case of the familial type, the disease is due to an inherited mutation at specific sites in three genes. Also, there are some genetic risk factors that facilitate the development of sporadic Alzheimer’s disease. All of these genetic analyses were performed using blood samples as a source of DNA. However, the presence of somatic mutations in the brain can be identified only using brain samples. In this review, we comment on a method that correctly identifies single nucleotide variations in the human brain and that can be used to validate high-through sequencing techniques. This method involves selective enrichment of the DNA population bearing the nucleotide variations, thereby facilitating posterior validation of the data by Sanger’s sequencing.
INTRODUCTION
The primary cause of some cases of Alzheimer’s disease (AD) (familial AD) is an inherited mutation (s) at a specific site (s) in the genes APP, PSEN1, or PSEN2. These mutations can be detected in blood cells since they are already present in the germinal cells [1]. However, in most cases of AD (sporadic AD), the primary cause is not well determined, although several non-modifiable (aging and genetic) and modifiable (non-genetic) risk factors may facilitate the onset of the condition [2].
However, it has recently been proposed that brain somatic mutations [3] are involved in the development of the sporadic AD [4, 5] and that the presence of these mutations causes mosaic genomic heterogeneity [6].
Somatic mutations may be due to a change in a single nucleotide or to the insertion or deletion (indels) of a small number of linked (oligo) nucleotides that can sometimes “jump” from one part of the genome to another one [7]. These oligonucleotides “jump” via a mechanism involving Piwi-RNAs [8]. This mechanism is impaired in aging—a physiological process known to be a main risk for neurodegenerative disorders.
When a somatic single mutation is present in a small number of brain cells, methods like Sanger’s sequencing cannot be used for detection purposes. Sanger’s sequencing requires an allelic frequency of 20% (or higher) for the detection of a somatic mutation [9]. However, other techniques, like massive parallel sequencing (e.g., Illumina), allow the detection of mutations present in a very small number of cells [10, 11]. However, these techniques can introduce a low proportion of errors when reading sequence alignments. Here we comment on the validation of brain somatic mutations detected by high-throughput sequencing techniques.
HIGH-THROUGHPUT SEQUENCING TECHNIQUES: POSSIBLE ERRORS
When only a small number of cells bear the somatic mutation compared to the total cell number, detection techniques like Sanger’s method are unsuitable and others are required. These somatic mutations sometimes occur at CpG nucleotides, at cytosines in modified (methylated) or unmodified form [12]. In this regard, as glycine (Gly) or arginine (Arg) amino acids begin with a CG dinucleotide, a larger proportion of somatic mutations have been reported to involve the replacement of Gly or Arg by other residues [13]. Thus, reported mutations at CpG sites can often be real mutations. Nevertheless, errors can also be introduced by new sequencing technologies.
Among sequencing technologies, the Illumina platform enjoys widespread use in the field because of its low costs for a high number of fragments and samples [14, 15]. However, despite its popularity and advantages, the platform has some drawbacks [16]. Its performance depends greatly on the starting material and its uniformity. For example, starting material with a high uniformity, such as 16S DNA, leads to a higher number of false read nucleotides [17, 18]. Two kinds of errors can be distinguished: those involving incorrect sorting of the sequence and those that are inside the sequence itself.
Index errors
A second issue is the correct identification of indices [19]. Under normal circumstances, the index of the read is analyzed in a separate step to identify those that belong to a sample in a multiplex setting. Sinha reported that up to 10% of sequencing reads (or signals) are incorrectly assigned in a multiplexed pool of samples. In contrast to substitution errors, which can be tackled by bioinformatics tools and analysis, this kind of error is often undetectable. It is not possible to discern whether a human genome read in one sample truly originates in this sample or whether it is from another that has been included in the sequencing process with the intention to reduce costs. Of course, in the case of distinct genomes, such incorrect sequence alignments would be resolved, but with the same genome this is not possible. Therefore, it is also important to analyze non-matched reads. This can be done with the high-throughput tools Kraken [20] or Centrifuge [21] when an analysis of all reads is needed, or, for example, with TruePure, a tool that focuses on only a small part of the reads and provides a first impression [22].
Substitution errors
The most important error, especially in a clinical analysis, is an undiscovered SNP an artificially introduced SNP that is not present in the original DNA. These SNPs are often mutations with a frequency below 50% and are thus low level mutations (LLMs) [23]. It is widely known that the Illumina platform gives such substitution errors. The occurrence of these types of error contrasts with those given by Roche/454 sequencers, which give more indel errors [24, 25]. The behavior of the Illumina platform is explained not only by the sequencing step itself, but also by amplification steps, which are needed to add adapters or during cluster generation on the flow cell [26]. Substitution errors are not evenly distributed, and some errors, like A to C and C to G, are more common, as are inverted repeats [27]. The technology itself, which uses similar emission spectra of the fluorophores for A and C and G and T, is responsible for the errors [24]. Errors gather at the end of the sequence due to technical accumulation of phasing and pre-phasing [24, 28]. Thus, longer reads, which then cover more of the genome and can be more easily aligned, do not provide a simple solution for this issue. Also, quality scores are not always useful to detect such errors, as they are sometimes associated with high quality [17]. Schirmer et al. analyzed these substitution errors and found the bias to be associated with ddGTPs [15].
Occurrence and distribution
Substitutions errors are not evenly distributed across the genome as was always used in general analysis for sequencing data to show how few errors are across a read [29–32]. The above-mentioned motifs occur in certain regions of the genome and thus such regions are prone to errors and are therefore difficult to scan for LLMs. Another issue is that repeated sequencing of the same region may result in detection of the same error, which leads to the assumption that a certain mutation is present at a high percentage [23]. The best way to tackle this issue is to increase the number of molecules with the mutation of interest without a simple error-prone PCR.
VALIDATION OF HIGH-THROUGHPUT SEQUENCING TECHNIQUES
It is difficult to confirm the somatic SNVs detected by high-throughput sequencing techniques like the Illumina platform. However, only a few of the total mutations that are identified may be caused by an error in the sequencing. Thus, it is advisable to achieve total validation of all the SNVs detected, thus moving from suspected SNVs to true SNVs.
Sanger’s sequencing remains one of the most reliable techniques with respect to errors. However, it cannot be used when there is a very low proportion of a specific SNV.
In this regard, it is convenient to have a procedure by which to remove the population lacking the specific SNV and thus enrich the population with it. This SNV could be then validated by Sanger’s sequencing. In addition, prior to SNV removal, a first validation of the SNV based on a data filter using software such as Virmid is recommended [33].
After filtering the data, the DNA molecules bearing the specific SNV can be amplified by treating the whole DNA population with specific restriction enzymes that recognize a motif present in those molecules in which the specific SNV is absent. In this regard, those DNA molecules lacking the specific SNV can be removed, thus achieving the enrichment of the population with the SNV of interest.
RESTRICTION NUCLEASES
Restriction (endo) nucleases are enzymes that cut DNA at a specific sequence (motif). These nucleases were discovered in the last century during research into bacterial DNases [34].
Several types of restriction nucleases have been described, including the most recent, Cos-9-gRNA complex (CRISPRs), which use RNA to target specific DNA sequences [35] (Table 1). However, for the purpose of validating Illumina sequencing, we will focus on type II restriction nucleases, which were discovered by H.O. Smith [36]. These nucleases recognize a specific sequence of nucleotides and then cut it at specific DNA site. The motif can be 4 to 8 base pairs long. Thousands of restrictions enzymes have been analyzed and many are commercially available. The structure and function of type II restriction endonucleases have been deeply reviewed, and type II have been classified into eight subtypes: orthodox, IIS, IIE, IIF, IIT, IIG, IIIB, and IIM. Each of them is characterized by a specific example of restriction enzyme, indicating the characteristic features of the subtype [37, 38]. Among those features, the presence of metal ions may play a role in the properties of protein-DNA interaction [39].
Table 1
Restriction nucleases (type). Different types (I-IV) of restriction nucleases, reviewed in reference [34], together with others discovered later [35], are shown
Type | Cut |
I | DNA at a random distance from the recognition sequence |
II | DNA at the site of the recognition sequence |
III | DNA 20–30 nucleotides away from the recognition sequence |
IV | Modified (usually methylated) DNA |
Others | CRISPRs, zinc finger nucleases, talens nucleases |
On the other hand, the use of restriction endonucleases has recently decreased, and for genome engineering techniques the use of artificial DNases, like zinc finger nucleases, to modify specific target sequences has increased [40]. However, these artificial nucleases have not yet been used for validation of DNA sequencing.
As previously indicated, for our sequencing validation analysis, we used type II restriction nucleases, which were selected after identifying the motif in which the somatic mutation is found (see Fig. 1 and reference [41]). The specific restriction nuclease is used to remove the normal sequence, thus allowing the detection of the rare variant.
Fig.1
Removal of fragments with a specific length by means of nuclease digestion. The rational of the process is shown. Thus, when a mixture of DNA fragments of the same length is digested with an enzyme that cuts only those fragments bearing a specific nucleotide, the uncleaved fragments can be isolated by gel electrophoresis, since they maintain their length. These fragments can then be amplified and sequenced in further steps.
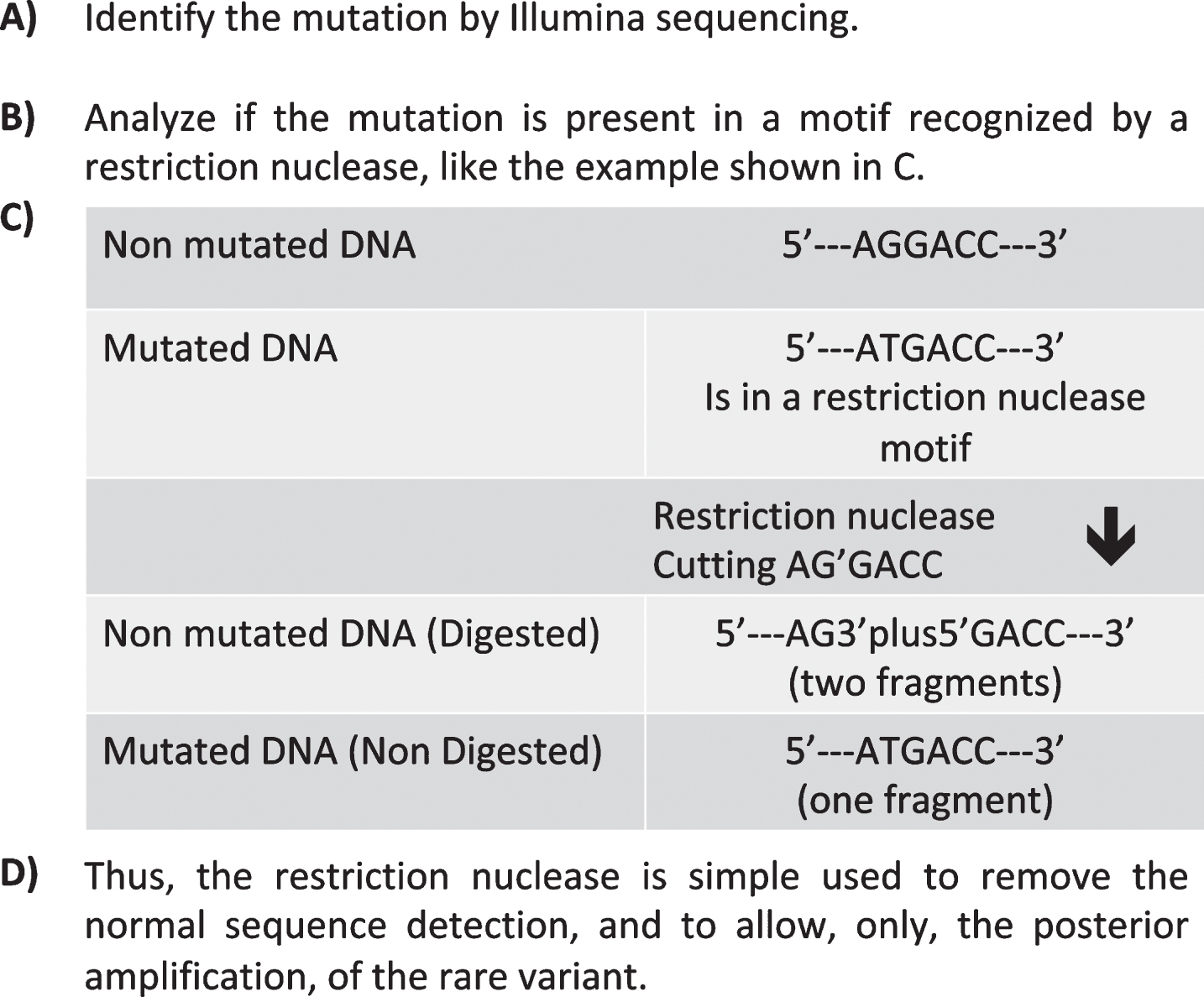
Thus, the use of type II restriction nucleases to remove DNA fragments of a specific length and lacking the SNV of interest was tested in blood and brain, with the aim to identify specific brain somatic mutations. Curiously, DNA cleavage by these nucleases provided clear data and better resolutions when blood cell DNA was used than when brain DNA was tested [41, 42].
After isolating the uncleaved brain DNA fragments containing the mutation by means of gel electrophoresis, they were amplified and sequenced in further steps.
AMPLIFICATION
Whole genome amplification (WGA) is a non-sequence-specific technique that allows amplification of the entire DNA sample. Given the insufficient amount of DNA present in certain samples (e.g., a single cell), WGA is a useful tool for several research fields (e.g., genetic diseases), as well as new for technologies such as next-generation sequencing (NGS) and comparative genomic hybridization (CGH) array. Unfortunately, DNA amplification is prone to the introduction of bias, error and co-amplification of minute levels of contaminating DNA.
In recent years, various techniques have been developed for WGA. These can be broadly categorized into PCR-related protocols and methods based on multiple displacement amplification (MDA). The former, in turn, can be classified into degenerate oligonucleotide-primed polymerase chain reaction (DOP-PCR; iDOP-PCR) [43, 44], linker-adapter PCR (LA-PCR) [45], primer extension pre-amplification PCR (PEP-PCR/ I-PEP-PCR) [46, 47], and variations thereof. MDA methods are based on using the highly processive Phi29 DNA polymerase [48] either in combination with random hexamers [49–52] or with a DNA primase (TthPrimPol) responsible for synthesizing the primers for the polymerase during the reaction [53]. Another variant of the MDA method, called pWGA, is based on the reconstituted T7 replication system [54]. A hybrid PCR / MDA method called multiple annealing and looping-based amplification cycles (MALBAC), which relies on the Bst polymerase for the MDA, has also been reported [55]. Finally, a method called Linear Amplification via Transposon Insertion (LIANTI), which combines Tn5 transposition and T7 in vitro transcription, has recently been described [56]. Each of these methods has its own merits and limitations.
The quality of the amplification result is determined by the following key parameters: the absence of contamination and artefacts in the reaction products; coverage breadth and uniformity; nucleotide error rates; and the ability to recover single-nucleotide variants (SNVs), copy number variants (CNVs) and structural variants. In general, PCR-based methods are thought to be appropriate for CNV detection [57], whereas MDA-based methods have the advantage that they give extremely low nucleotide error rates due to the high fidelity of Phi29 DNA polymerase and they produce very long amplification products, thus providing more complete coverage of the genome. Other issues affecting all amplification methods to some extent are chimera formation and preferential amplification of one allele (allelic dropout, ADO).
These WGA techniques can be adapted to the needs of studies that require specific amplification of particular DNA molecules, such as those containing somatic mutations or any other structural variants.
In our validation method [35], the genomic region containing the SNV of interest in the COL3A1 gene was amplified by PCR and digested with a restriction enzyme (Eco0109I) that cleaves only the molecules lacking the SNV. The low recovery yield of the uncleaved DNA after the digestion and gel-based purification steps were the main bottleneck for posterior Sanger sequencing. Therefore, an amplification step after DNA digestion and before sequencing was introduced. For this purpose, we first heat-denatured and circularized the DNA molecules remaining after the enzymatic digestion and purification, in order to generate a substrate suitable for rolling circle amplification (RCA). To enrich the amplification products with molecules bearing the SNV of interest and reduce the effect of non-digested or contaminating DNA molecules lacking the SNV, we then performed TruePrime™ RCA in the presence of specific forward and reverse oligonucleotides complementary only to the DNA molecules of interest. Two distinct amplification protocols were followed in the presence of increasing concentrations of the SNV-specific oligonucleotides. In the first case, all the components of the amplification mixture were added simultaneously. In the alternative protocol, to prioritize the use of the specific primers as starting sites of RCA and therefore increase the specificity of the procedure, TthPrimPol DNA primase was added after incubating the rest of the amplification mixture for 1 h. The subsequent addition of TthPrimPol allowed an increase in the number of starting points for the amplification and therefore in the efficiency of the process, thereby enhancing the final amplification yield.
Sanger sequencing of the amplified DNA samples demonstrated the effectiveness of the method to validate low frequency allele variations present in at least 10% of the original DNA molecules. Thus, this method could be suitable to validate SNPs identified by high-throughput sequencing techniques.
CONCLUSIONS
Somatic mutations in the brain may be involved in the onset of various neurodegenerative disorders. However, if there is a low proportion of brain cells bearing the mutation, the proper characterization of these mutations is not straightforward as brain tissue, in contrast to blood, is not suitable material for genetic studies. Furthermore, the use of high-throughput sequencing techniques can introduce errors. Thus, to identify true brain-specific mutations, a novel procedure has been proposed [41, 42]. This procedure involves the use of suitable software for data processing [33], the removal of DNA fragments lacking the mutation by specific restriction nucleases, amplification of the uncleaved DNA fragments bearing the mutation, and characterization of the mutation by Sanger sequencing (see Fig. 2).
Fig.2
Schematic diagram of the method for validating somatic mutations in the brain characterized by Illumina sequencing.
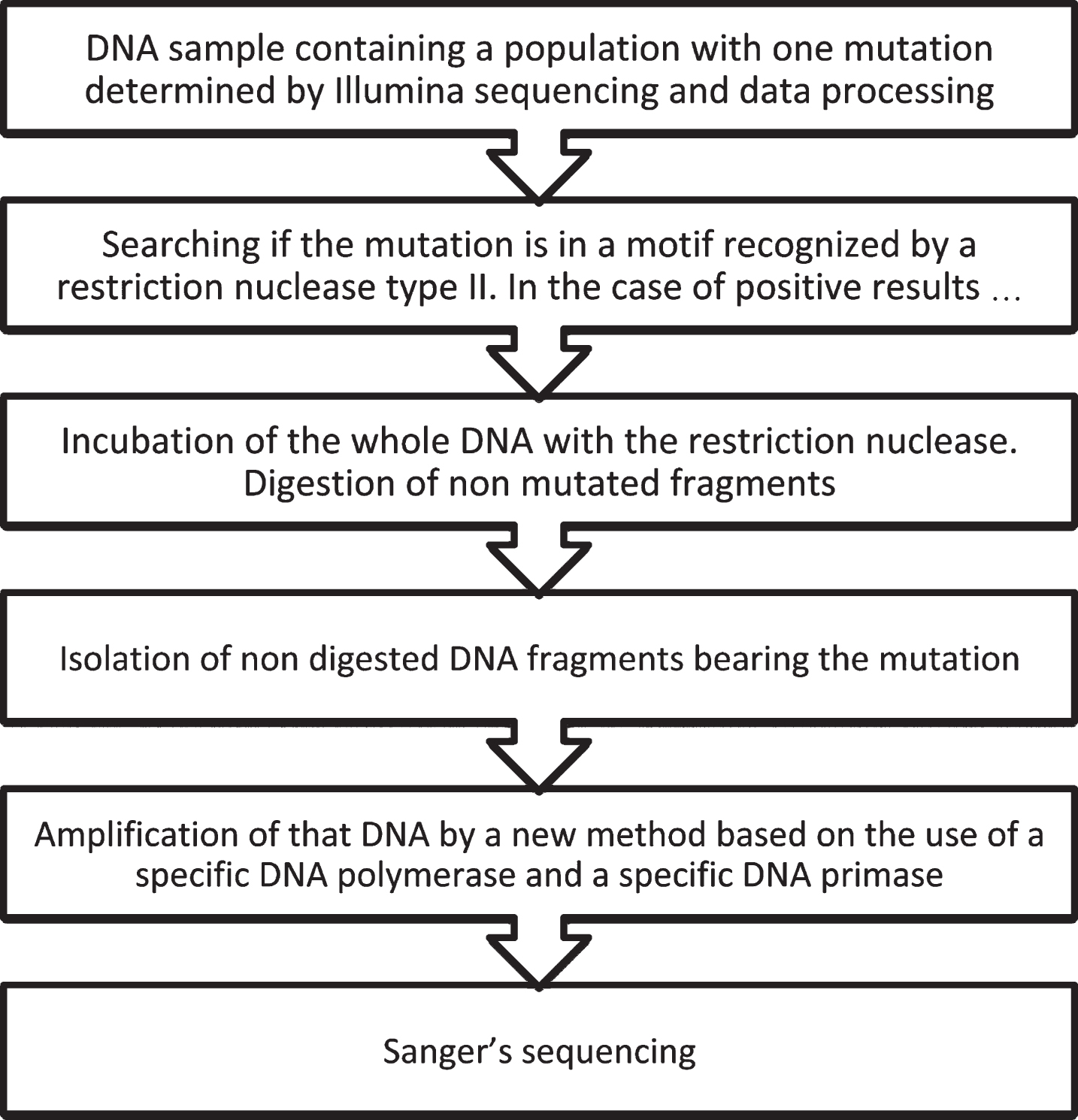
REFERENCES
[1] | Price DL , Tanzi RE , Borchelt DR , Sisodia SS ((1998) ) Alzheimer’s disease: Genetic studies and transgenic models. Annu Rev Genet 32: , 461–493. |
[2] | Mayeux R ((2003) ) Epidemiology of neurodegeneration. Annu Rev Neurosci 26: , 81–104. |
[3] | Lodato MA , Woodworth MB , Lee S , Evrony GD , Mehta BK , Karger A , Lee S , Chittenden TW , D’Gama AM , Cai X , Luquette LJ , Lee E , Park PJ , Walsh CA ((2015) ) Somatic mutation in single human neurons tracks developmental and transcriptional history. Science 350: , 94–98. |
[4] | Parcerisas A , Rubio SE , Muhaisen A , Gomez-Ramos A , Pujadas L , Puiggros M , Rossi D , Urena J , Burgaya F , Pascual M , Torrents D , Rabano A , Avila J , Soriano E ((2014) ) Somatic signature of brain-specific single nucleotide variations in sporadic Alzheimer’s disease. J Alzheimers Dis 42: , 1357–1382. |
[5] | Sala Frigerio C , Lau P , Troakes C , Deramecourt V , Gele P , Van Loo P , Voet T , De Strooper B ((2015) ) On the identification of low allele frequency mosaic mutations in the brains of Alzheimer’s disease patients. Alzheimers Dement 11: , 1265–1276. |
[6] | Evrony GD ((2016) ) One brain, many genomes. Science 354: , 557–558. |
[7] | Erwin JA , Marchetto MC , Gage FH ((2014) ) Mobile DNA elements in the generation of diversity and complexity in the brain. Nat Rev Neurosci 15: , 497–506. |
[8] | Sturm A , Perczel A , Ivics Z , Vellai T ((2017) ) The Piwi-piRNA pathway: Road to immortality. Aging Cell 16: , 906–911. |
[9] | Tsiatis AC , Norris-Kirby A , Rich RG , Hafez MJ , Gocke CD , Eshleman JR , Murphy KM ((2010) ) Comparison of Sanger sequencing, pyrosequencing, and melting curve analysis for the detection of KRAS mutations: Diagnostic and clinical implications. J Mol Diagn 12: , 425–432. |
[10] | Shendure J , Ji H ((2008) ) Next-generation DNA sequencing. Nat Biotechnol 26: , 1135–1145. |
[11] | Goodwin S , McPherson JD , McCombie WR ((2016) ) Coming of age: Ten years of next-generation sequencing technologies. Nat Rev Genet 17: , 333–351. |
[12] | Shendure J , Akey JM ((2015) ) The origins, determinants, and consequences of human mutations. Science 349: , 1478–1483. |
[13] | Cooper DN , Krawczak M ((1990) ) The mutational spectrum of single base-pair substitutions causing human genetic disease: Patterns and predictions. Hum Genet 85: , 55–74. |
[14] | White SJ , Laros JFJ , Bakker E , Cambon-Thomsen A , Eden M , Leonard S , Lochmuller H , Matthijs G , Mattocks C , Patton S , Payne K , Scheffer H , Souche E , Thomassen E , Thompson R , Traeger-Synodinos J , Van Vooren S , Janssen B , den Dunnen JT ((2017) ) Critical points for an accurate human genome analysis. Hum Mutat 38: , 912–921. |
[15] | Schirmer M , D’Amore R , Ijaz UZ , Hall N , Quince C ((2016) ) Illumina error profiles: Resolving fine-scale variation in metagenomic sequencing data. BMC Bioinformatics 17: , 125. |
[16] | Kircher M , Heyn P , Kelso J ((2011) ) Addressing challenges in the production and analysis of illumina sequencing data. BMC Genomics 12: , 382. |
[17] | Schirmer M , Ijaz UZ , D’Amore R , Hall N , Sloan WT , Quince C ((2015) ) Insight into biases and sequencing errors for amplicon sequencing with the Illumina MiSeq platform. Nucleic Acids Res 43: , e37. |
[18] | Quail MA , Smith M , Coupland P , Otto TD , Harris SR , Connor TR , Bertoni A , Swerdlow HP , Gu Y ((2012) ) A tale of three next generation sequencing platforms: Comparison of Ion Torrent, Pacific Biosciences and Illumina MiSeq sequencers. BMC Genomics 13: , 341. |
[19] | Sinha R , Stanley G , Gulati GS , Ezran C , Travaglini KJ , Wei E , Chan CKF , Nabhan AN , Su T , Morganti RM , Conley SD , Chaib H , Red-Horse K , Longaker MT , Snyder MP , Krasnow MA , Weissman IL ((2017) ) Index switching causes “spreading-of-signal” among multiplexed samples in Illumina HiSeq 4000 DNA sequencing. bioRxiv, doi: https://doi.org/10.1101-125724. |
[20] | Wood DE , Salzberg SL ((2014) ) Kraken: Ultrafast metagenomic sequence classification using exact alignments. Genome Biol 15: , R46. |
[21] | Kim D , Song L , Breitwieser FP , Salzberg SL ((2016) ) Centrifuge: Rapid and sensitive classification of metagenomic sequences. Genome Res 26: , 1721–1729. |
[22] | Budeus B ((2017) ) Expedeon TruePure™ tool: Contamination Analysis for Sequencing. https://www.expedeon.com/services/truehelix/truepure. |
[23] | Li M , Stoneking M ((2012) ) A new approach for detecting low-level mutations in next-generation sequence data. Genome Biol 13: , R34. |
[24] | Kircher M , Stenzel U , Kelso J ((2009) ) Improved base calling for the Illumina Genome Analyzer using machine learning strategies. Genome Biol 10: , R83. |
[25] | Hoffmann S , Otto C , Kurtz S , Sharma CM , Khaitovich P , Vogel J , Stadler PF , Hackermuller J ((2009) ) Fast mapping of short sequences with mismatches, insertions and deletions using index structures. PLoS Comput Biol 5: , e1000502. |
[26] | Kozarewa I , Ning Z , Quail MA , Sanders MJ , Berriman M , Turner DJ ((2009) ) Amplification-free Illumina sequencing-library preparation facilitates improved mapping and assembly of (G+C)-biased genomes. Nat Methods 6: , 291–295. |
[27] | Nakamura K , Oshima T , Morimoto T , Ikeda S , Yoshikawa H , Shiwa Y , Ishikawa S , Linak MC , Hirai A , Takahashi H , Altaf-Ul-Amin M , Ogasawara N , Kanaya S ((2011) ) Sequence-specific error profile of Illumina sequencers.e. Nucleic Acids Res 39: , 90. |
[28] | Taub MA , Corrada Bravo H , Irizarry RA ((2010) ) Overcoming bias and systematic errors in next generation sequencing data. Genome Med 2: , 87. |
[29] | Li M , Schonberg A , Schaefer M , Schroeder R , Nasidze I , Stoneking M ((2010) ) Detecting heteroplasmy from high-throughput sequencing of complete human mitochondrial DNA genomes. Am J Hum Genet 87: , 237–249. |
[30] | Dohm JC , Lottaz C , Borodina T , Himmelbauer H ((2008) ) Substantial biases in ultra-short read data sets from high-throughput DNA sequencing. Nucleic Acids Res 36: , e105. |
[31] | Meacham F , Boffelli D , Dhahbi J , Martin DI , Singer M , Pachter L ((2011) ) Identification and correction of systematic error in high-throughput sequence data. BMC Bioinformatics 12: , 451. |
[32] | Minoche AE , Dohm JC , Himmelbauer H ((2011) ) Evaluation of genomic high-throughput sequencing data generated on Illumina HiSeq and genome analyzer systems. Genome Biol 12: , R112. |
[33] | Kim S , Jeong K , Bhutani K , Lee J , Patel A , Scott E , Nam H , Lee H , Gleeson JG , Bafna V ((2013) ) Virmid: Accurate detection of somatic mutations with sample impurity inference. Genome Biol 14: , R90. |
[34] | Roberts RJ ((1976) ) Restriction endonucleases. CRC Crit Rev Biochem 4: , 123–164. |
[35] | Makarova KS , Wolf YI , Alkhnbashi OS , Costa F , Shah SA , Saunders SJ , Barrangou R , Brouns SJ , Charpentier E , Haft DH , Horvath P , Moineau S , Mojica FJ , Terns RM , Terns MP , White MF , Yakunin AF , Garrett RA , van der Oost J , Backofen R , Koonin EV ((2015) ) An updated evolutionary classification of CRISPR-Cas systems. Nat Rev Microbiol 13: , 722–736. |
[36] | Smith HO , Wilcox KW ((1970) ) A restriction enzyme from Hemophilus influenzae. I. Purification and general properties. J Mol Biol 51: , 379–391. |
[37] | Pingoud A , Jeltsch A ((2001) ) Structure and function of type II restriction endonucleases. Nucleic Acids Res 29: , 3705–3727. |
[38] | Marshall JJ , Halford SE ((2010) ) The type IIB restriction endonucleases. Biochem Soc Trans 38: , 410–416. |
[39] | Advani S , Mishra P , Dubey S , Thakur S ((2010) ) Categoric prediction of metal ion mechanisms in the active sites of 17 select type II restriction endonucleases. Biochem Biophys Res Commun 402: , 177–179. |
[40] | Chandrasegaran S , Carroll D ((2016) ) Origins of programmable nucleases for genome engineering. J Mol Biol 428: , 963–989. |
[41] | Gomez-Ramos A , Picher AJ , Garcia E , Garrido P , Hernandez F , Soriano E , Avila J ((2017) ) Validation of suspected somatic single nucleotide variations in the brain of Alzheimer’s disease patients. J Alzheimers Dis 56: , 977–990. |
[42] | Gomez-Ramos A , Podlesniy P , Soriano E , Avila J ((2015) ) Distinct X-chromosome SNVs from some sporadic AD samples. Sci Rep 5: , 18012. |
[43] | Telenius H , Carter NP , Bebb CE , Nordenskjold M , Ponder BA , Tunnacliffe A ((1992) ) Degenerate oligonucleotide-primed PCR: General amplification of target DNA by a single degenerate primer. Genomics 13: , 718–725. |
[44] | Blagodatskikh KA , Kramarov VM , Barsova EV , Garkovenko AV , Shcherbo DS , Shelenkov AA , Ustinova VV , Tokarenko MR , Baker SC , Kramarova TV , Ignatov KB ((2017) ) Improved DOP-PCR (iDOP-PCR): A robust and simple WGA method for efficient amplification of low copy number genomic DNA. PLoS One 12: , e0184507. |
[45] | Klein CA , Schmidt-Kittler O , Schardt JA , Pantel K , Speicher MR , Riethmuller G ((1999) ) Comparative genomic hybridization, loss of heterozygosity, and DNA sequence analysis of single cells. Proc Natl Acad Sci U S A 96: , 4494–4499. |
[46] | Zhang L , Cui X , Schmitt K , Hubert R , Navidi W , Arnheim N ((1992) ) Whole genome amplification from a single cell: Implications for genetic analysis. Proc Natl Acad Sci U S A 89: , 5847–5851. |
[47] | Arneson N , Hughes S , Houlston R , Done S ((2008) ) Whole-genome amplification by improved primer extension preamplification PCR (I-PEP-PCR). CSH Protoc 2008: , pdb prot4921. |
[48] | Blanco L , Bernad A , Lazaro JM , Martin G , Garmendia C , Salas M ((1989) ) Highly efficient DNA synthesis by the phage phi 29 DNA polymerase. Symmetrical mode of DNA replication. J Biol Chem 264: , 8935–8940. |
[49] | Dean FB , Hosono S , Fang L , Wu X , Faruqi AF , Bray-Ward P , Sun Z , Zong Q , Du Y , Du J , Driscoll M , Song W , Kingsmore SF , Egholm M , Lasken RS ((2002) ) Comprehensive human genome amplification using multiple displacement amplification. Proc Natl Acad Sci U S A 99: , 5261–5266. |
[50] | Spits C , Le Caignec C , De Rycke M , Van Haute L , Van Steirteghem A , Liebaers I , Sermon K ((2006) ) Whole-genome multiple displacement amplification from single cells. Nat Protoc 1: , 1965–1970. |
[51] | Paez JG , Lin M , Beroukhim R , Lee JC , Zhao X , Richter DJ , Gabriel S , Herman P , Sasaki H , Altshuler D , Li C , Meyerson M , Sellers WR ((2004) ) Genome coverage and sequence fidelity of phi29 polymerase-based multiple strand displacement whole genome amplification. Nucleic Acids Res 32: , e71. |
[52] | Spits C , Le Caignec C , De Rycke M , Van Haute L , Van Steirteghem A , Liebaers I , Sermon K ((2006) ) Optimization and evaluation of single-cell whole-genome multiple displacement amplification. Hum Mutat 27: , 496–503. |
[53] | Picher AJ , Budeus B , Wafzig O , Kruger C , Garcia-Gomez S , Martinez-Jimenez MI , Diaz-Talavera A , Weber D , Blanco L , Schneider A ((2016) ) TruePrime is a novel method for whole-genome amplification from single cells based on TthPrimPol. Nat Commun 7: , 13296. |
[54] | Li Y , Kim HJ , Zheng C , Chow WH , Lim J , Keenan B , Pan X , Lemieux B , Kong H ((2008) ) Primase-based whole genome amplification.e. Nucleic Acids Res 36: , 79. |
[55] | Zong C , Lu S , Chapman AR , Xie XS ((2012) ) Genome-wide detection of single-nucleotide and copy-number variations of a single human cell. Science 338: , 1622–1626. |
[56] | Chen C , Xing D , Tan L , Li H , Zhou G , Huang L , Xie XS ((2017) ) Single-cell whole-genome analyses by Linear Amplification via Transposon Insertion (LIANTI). Science 356: , 189–194. |
[57] | Deleye L , De Coninck D , Christodoulou C , Sante T , Dheedene A , Heindryckx B , Van den Abbeel E , De Sutter P , Menten B , Deforce D , Van Nieuwerburgh F ((2015) ) Whole genome amplification with SurePlex results in better copy number alteration detection using sequencing data compared to the MALBAC method. Sci Rep 5: , 11711. |