Machine learning for psychological disorder prediction in Indians during COVID-19 nationwide lockdown
Abstract
As the world combats with the outrageous and perilous novel coronavirus, national lockdown has been enforced in most of the countries. It is necessary for public health but on the flip side it is detrimental for people’s mental health. While the psychological repercussions are predictable during the period of COVID-19 lockdown but this enforcement can lead to long-term behavioral changes post lockdown too. Moreover, the detection of psychological effects may take months or years. This mental health crisis situation requires timely, pro-active intervention to cope and persevere the Coro-anxiety (Corona-related). To address this gap, this research firstly studies the psychological burden among Indians using a COVID-19 Mental Health Questionnaire and then does a predictive analytics using machine learning to identify the likelihood of mental health outcomes using learned features of 395 Indian participants. The proposed Psychological Disorder Prediction (PDP) tool uses a multinomial Naïve Bayes classifier to train the model to detect the onset of specific psychological disorder and classify the participants into two pre-defined categories, namely, anxiety disorder and mood disorder. Experimental evaluation reports a classification accuracy of 92.15%. This automation plays a pivotal role in clinical support as it aims to suggest individuals who may need psychological help.
1.Introduction
According to World Health Organization(WHO) situation
report- 88,11
the novel coronavirus disease, COVID-19 has spread to at least 213 countries and regions around the globe, while a total of
139,378 people have died, and confirmed cases hit 20,74,529
(17
Similar to many countries, India also implemented a stringent nationwide lockdown to contain the spread of COVID-19 and to reduce fatalities. Initial preventive measures such as closing borders and cancelling passenger air transport to & from India and inter-state public transport were executed as national action plan. However, along with the lockdown, a feeling of uncertainty and anxiety has also taken over the country. Undeniably there is a sense of threat as people are prone to overestimating the likelihood or severity of disease. Lockdowns have triggered elements of panic in community where people are bulk buying amid corona fears. This psychosocial disturbance associated with restrictions to everyday practices can further have rippling effects. Separation from loved ones, boredom, loss of freedom, uncertainty over disease status, stigmatization, job and financial stress are some emotional challenges that have aroused during outbreak.
People are experiencing mental health crisis with this new sudden arrangement. WHO has acknowledged
that the crisis is generating stress and has advised people to avoid watching, reading or listening to news that
causes feelings of anxiety or
distress.44
Though it is essential to stay adequately informed but information overload can aggravate the reaction to stress, anxiety and depression [1]. It is critical to stop multiplying of fear, assess the stress levels and act on strategies to maintain mental wellness. Everyone differs in their need for control, their tolerance for uncertainty, and their ability to be resilient. In some cases reaching out to friends and family may help whereas in some formal professional help may be required. All of this is taking its toll on people’s mental health and causing psychological distress. Reliable artificial intelligence based preventative intervention tools can aid mental health professionals [2, 3, 4]. Each psychological disorder has its own characteristic symptoms and some general warning signs to alert the need of professional help. An intelligent mental illness diagnostic can support clinicians with early detection. One of the most proverbial application of artificial intelligence, machine learning provides systems the ability to infer from the past experience and make decisions accordingly. Indeed data-driven decision making using machine learning techniques can been used as a valuable technique to help predict which patients with mental health crisis at an early stage. Thus, it is imperative to build viable prediction tool which detects and characterizes the type of depression with minimal human intervention. Motivated by this, in this paper, a machine learning tool to predict psychological disorders during the COVID-19 lockdown is proposed. The psychological disorder prediction (PDP) tool classifies the mental illness into two types, namely, anxiety disorder and mood disorder. The classifier is characteristically a Navie Bayes classifier trained to predict the onset of mental illness, a health condition that changes thinking, feelings, or behavior (or all three) in individuals over a sample data set of 395 Indians. A mental health questionnaire based response dataset is created and 18 attributes are identified from the data set to train the classifier. The response is collected for a period from 26
• A COVID-18 Mental Health Questionnaire (MHQ COVID-19) is designed to comprehend the psychological burden experienced by the participants and its outcomes are reported.
• Supervised Machine learning is used to predict the categories of psychological disorder type.
• A comparative analysis of five classifiers, namely, decision tree, k-nearest neighbor, support vector machine, logistic regression and naïve Bayes classifier is done.
• The psychological disorder prediction (PDP) tool is conceptually positioned to refine clinical management by early diagnosis and disorder classification for psychological first aid.
The organization of the paper is as follows: Section 2 briefly describes the COVID-19 outbreak and its impact on mental health. The psychological disorders considered in this work are also concisely presented in this section. The review of related literature work is given in Section 3 followed by Section 4 which presents the architecture and working details of proposed PDP tool followed by its empirical analysis in Section 5. The conclusion, limitations and future scope of the research is presented in Section 6.
2.Background details: COVID-19 and psychological distress
This section briefly discusses the background details on the COVID-19 pandemic and the reason to address mental health and psychological needs during the outbreak. The anatomy of the psychological disorders considered for this study is also explained.
2.1COVID-19
COVID-19, is an acronym for coronavirus disease 2019, where CO stands for corona, VI for virus and D for disease, while 19 is for the year that the outbreak was first identified (31 December 2019) [5]. The International Committee on Taxonomy of Viruses55 refers the virus as “severe acute respiratory syndrome coronavirus 2”, or SARS-CoV-2. The disease was first identified in 2019 in Wuhan, the capital of China’s Hubei province, and on 11 March, 2020, the World Health Organization (WHO) announced that the outbreak should be considered a pandemic as it spread globally across multiple countries. It is a contagious respiratory illness with fever, dry cough and tiredness as commonly reported symptoms. The virus can be asymptomatic or in mild cases people may get just a runny nose or a sore throat. In the most severe cases, people with the virus can develop difficulty breathing, and may ultimately experience a multiple-organ failure causing fatalities. The COVID-19 virus spreads primarily through droplets of saliva or discharge from the nose when an infected person coughs or sneezes [6]. Figure 2 shows the microscopic image of SARS-CoV-2. The spikes on the outer edge of the virus particles resemble a crown, giving the virus its characteristic name.
Figure 2.
Microscopic image of SARS-CoV-2.
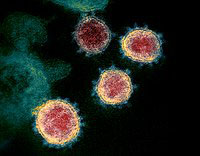
There is no available vaccine for COVID-19. Social distancing (stay at home, no handshakes), practicing good hygiene (washing hands) and respiratory etiquette (for example, by coughing or sneezing with a flexed elbow) are some key preventive methodologies adopted to prevent community transmission. The Indian Council of Medical Research (ICMR)66 categories the spread of disease into four stages, namely stage-1 where transmission is limited to cases with travel history to other countries, stage-2 is local transmission from known infected persons, stage-3 is community transmission and stage-4 is when it turns into an epidemic. An epidemic that has spread over several countries or continents, usually affecting a large number of people is referred to as a pandemic. Though, India is at stage-2 now, the government has begun preparing for stage-3 and taken stern precautions to curb social gatherings. A 21-day nationwide lockdown starting 24th March 2020 has been announced for all 1.3 Billion Indians.
2.2COVID-19 and mental health
Though stress and sadness are normal and natural responses to COVID-19, self-isolation, social distancing, quarantine and a national lockdown can all be emotionally challenging. People who are working in different cities and live away from their families, all alone, are now stuck at home for this given time period. Their daily routine of going to office, hanging out with their friends or colleagues and meeting people in public spaces; things that give them joy and keep them entertained has come to a halt. This sudden arrangement has traumatized many people and triggered corona-phobia. The outbreak is continually affecting the global economy and consequently the people are facing job risks or financial insecurity. COVID-19 can add to one’s “typical” levels of stress and worry and can lead to increase in distress, symptoms and relapse into mental health. Further, epidemics lead to stigmatization of affected individuals, authority figures, and health care professionals. Reports regarding threatening by landlords and ostracizing healthcare professionals (doctors, nurses and paramedics) treating COVID-19 patients and aviation professionals (flight crew) have been reported in various news media in India.77 Undoubtedly, this behavior reflects the levels of psychological distress that people are suffering amid the global Coronavirus outbreak. Further, the fear of health contamination may lead someone to become obsessed with hand hygiene and trigger compulsion to wash or clean spiking the obsessive compulsive disorder. The psychological effects can lead to a range of reactions from fear to anxiety, from boredom to depression, from frustration to anger, from stigmatization to repulsion. While it is vital to remain mentally healthy it is equally essential to seek professional support & intervention if symptoms of this Corona-related “Coro-anxiety” impair daily functioning or personal/professional relationships.
2.3Psychological disorders
Feeling of uncertainty and panic are natural when a traumatic or grueling event occurs. But for some people the struggle becomes overwhelming when worries and fears start interfering with relationships and daily life. Formally, mental illnesses are health conditions involving changes in emotion, thinking or behavior (or a combination of these) [7]. The general cognitive function is hindered to an extent that it can trigger inappropriate responses because those responses are based upon inaccurate thoughts. That is, the person finds difficult to stay focused, process information, store it in memory, and accurately respond. Mental illness is conceptualized as a clinically significant behavioral dysfunction or psychological syndrome. There many different categories of mental/psychological disorders defined in the ICD-10, 10th revision of the International Statistical Classification of Diseases and Related Health Problems (ICD), a medical classification list by the World Health Organization (WHO) known as Mental and behavioral disorders, ICD codes F00 to F99. Figure 3 show the broad categories of psychological disorders [8].
Figure 3.
Categories of psychological disorder.
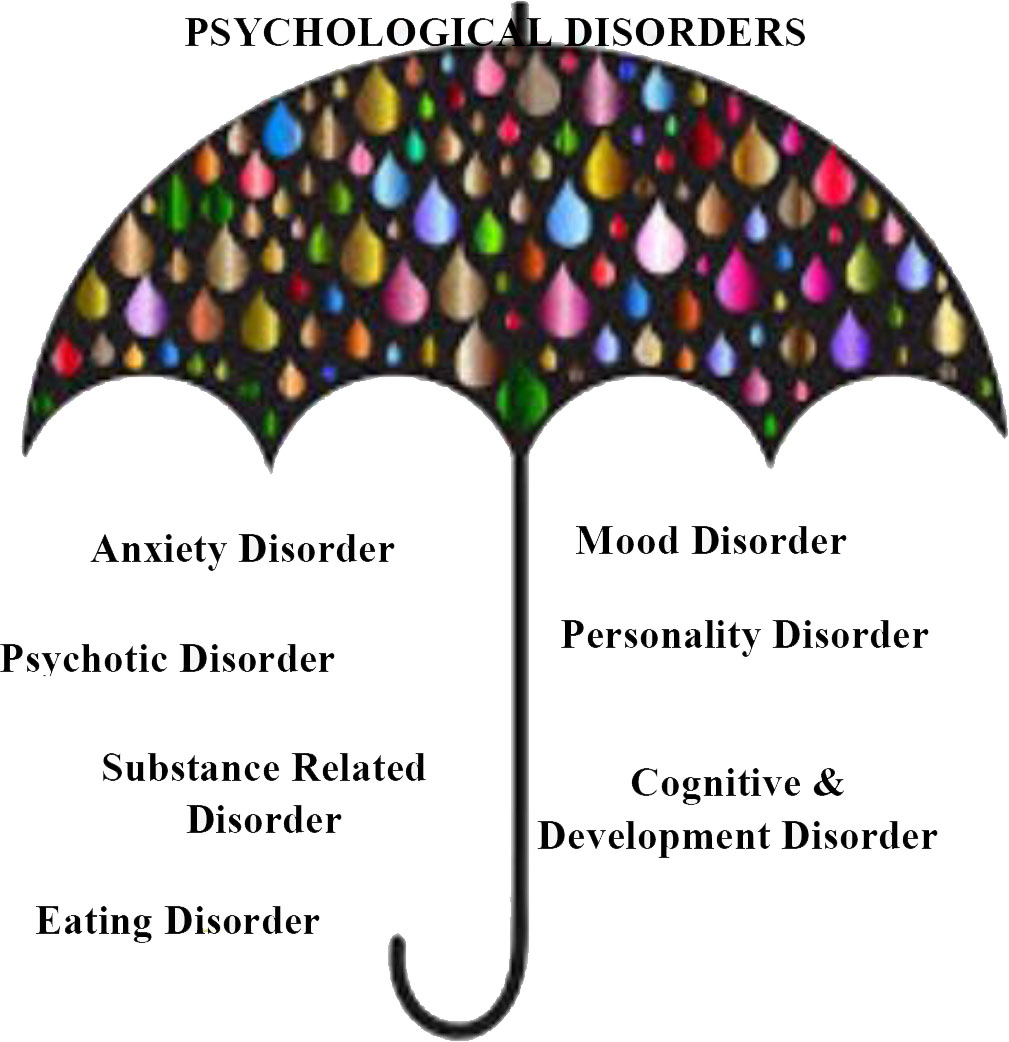
In this work, we considered binary classification into two types of common psychological disorder, namely, anxiety disorder (AD) and mood disorder (MD). The symptoms of AD and MD significantly overlap but mental markers such as fear and worry are commonly associated with AD whereas hopelessness and worthlessness are associated with MD.
2.3.1Anxiety disorder
Anxiety disorder [9] is an umbrella term to pool a collection of psychological disorders characterized by significant feelings of panic and fear (Fig. 4). It is an intrusive apprehension about future and frightfulness to the present situation, making it difficult to concentrate and accomplish daily tasks. It is an excessive, irrational dread of everyday situations which can interfere with daily activities. These feelings may cause physical symptoms, such as headaches, chest pain, palpitations, stomach ache and shakiness.
Figure 4.
Types of anxiety disorders.
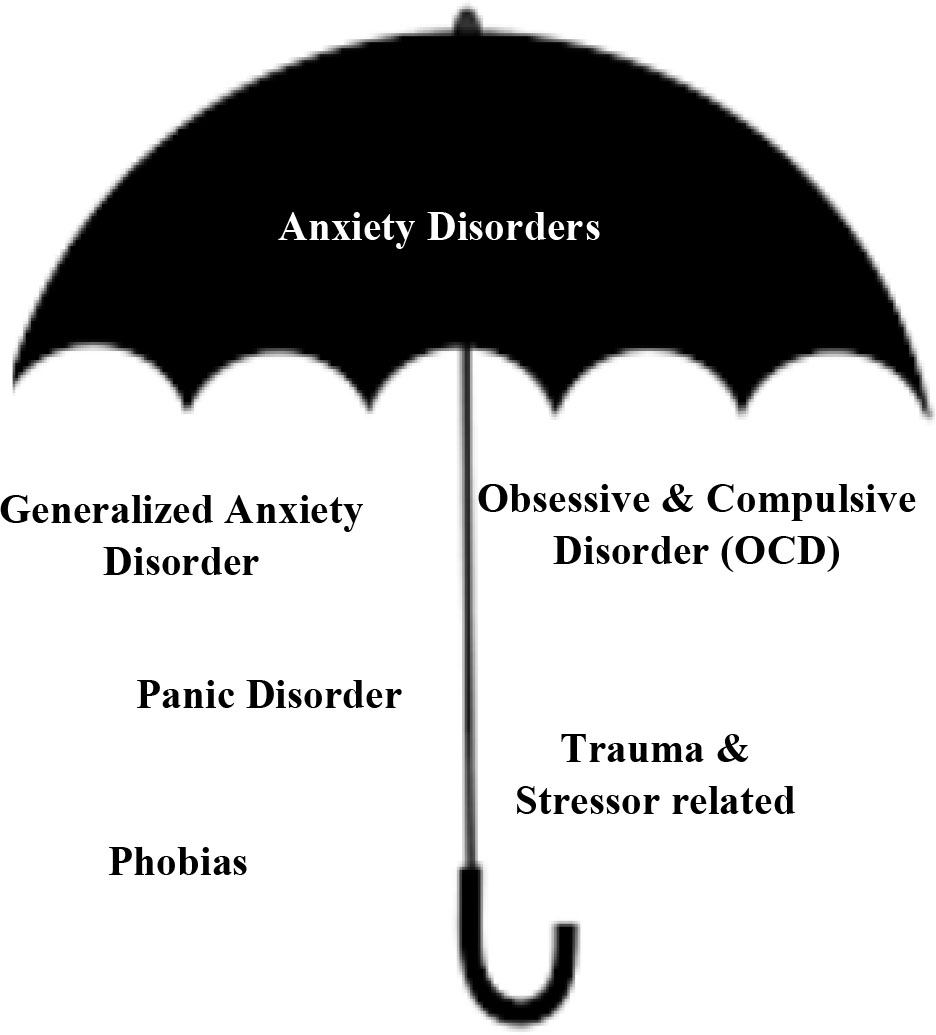
Figure 5.
Types of mood disorders.
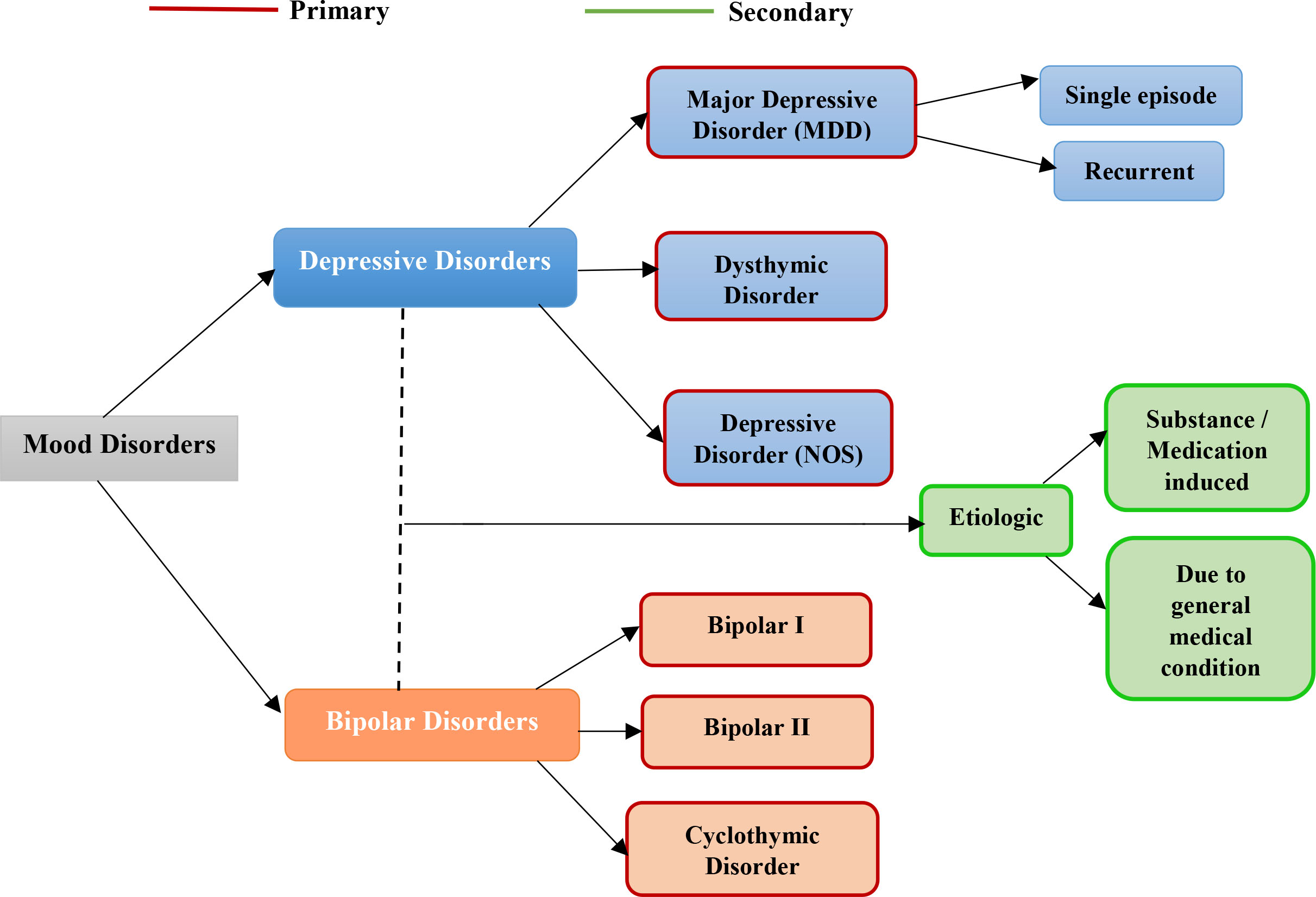
The following symptoms are some prominent markers of anxiety disorder:
• Difficulty in concentrating and carrying routine work.
• Restlessness.
• Excessive worrying & stress.
• Irrational phobias (fear triggered by a specific stimulus or situation, like fear of animals, heights, blood, natural events, situations).
• Significant weight gain/weight loss.
• Altered sleep pattern (insomnia, hypersomnia, parasomnia-sleep terrors, sleep walking, sleep talking, nightmare).
• Changed eating habits (anorexia or binge-eating).
• Separation fear.
• Adjustment difficulties.
• Obsessions – unrealistic thoughts or mental impulses.
2.3.2Mood disorder
Mood disorder or mood affective disorders [10] refer to the broad group of conditions where alteration in a person’s mood is the key feature. The words emotion and mood are often used interchangeably but emotions are generally episodic, phasic and short-term in comparison to mood which is continuous and long-term. Basically, the emotional effects may include changes in mood, motivation or enjoyment. Two major states of mood disorders exist: depressive and bipolar [11] (Fig. 5). A negatively activated mood can quantify to a manic and a depressed mood is known as a major depressive disorder (commonly known as clinical depression). For moods which cycle between mania and depression, the condition is known as bipolar disorder (BD).
The symptoms of mood disorders may include:
• Feelings of hopelessness or helplessness.
• Trouble sleeping or daytime sleepiness.
• Fatigue or low energy.
• Low self-esteem or self-confidence.
• Trouble concentrating or making decisions.
• Excessive guilt.
• Running away or threats of running away from home.
• Irritability, hostility, or aggression.
• Suicidal thoughts.
• Frequent physical complaints (for example, headache, stomachache, or tiredness) that don’t get better with treatment.
• Loss of interest in usual activities or activities that were once enjoyed.
• Ongoing sad, anxious, or “empty” mood.
• Panic buying, spending sprees.
• Significant weight gain/weight loss.
• Altered sleep pattern (insomnia, hypersomnia, parasomnia-sleep terrors, sleep walking, sleep talking, nightmare).
• Changed eating habits (anorexia or binge-eating).
3.Related studies
Artificial Intelligence has been reported to supplement clinical practice in various mental healthcare studies [12]. Pertinent studies on the potential use of machine learning (ML) algorithms in assessing mental illness are available too. In 2015, Zhou et al. [13] used logistic regression on data collected from social media activities through various trackers like web cam video tracking to analyze the eye blinking, head movement, pupillary variation to know the mental health status of a person. In the same year, Lim & Chia [14] used artificial neural network (ANN), K-nearest Neighbor and Linear Discriminant Analysis (LDA) to identify the cognitive stress from the EEG signals and showed that K-nearest neighbor performs better than ANN and LDA with accuracy of 72%. In 2016, Kessler et al. [15] used machine learning model to predict major depressive disorder (MDD) in 1056 respondents. In 2017, Reece et al. [16] used random forest classifier to train on the Twitter data of 204 users to predict the depression, PTSD disorder. Srividya et al. [17] used various supervised learning algorithms for identifying the mental health on the dataset collected from 656 people of different age groups through questioners in 2018. In 2019, Aggarwal et al. [18] used leave one out cross validation coupled with synthetic minority oversampling technique to identify a special type of psychological disorder known as Internet Gaming disorder from the data collected from PUBG an online gaming application platform. The most recent work on detecting psychological disorders using machine learning was proposed by Buettner et al. [19] in 2020, where they used random forest on EEG recording of 10 minutes only to identify the sleep disorders with the accuracy of 90% and in their subsequent study [20] they used EEG signals to detect schizophrenia using random forest only with accuracy of 96.77%.
Epidemics often scar the communities for life. Haunting memories, grief and struggle to regain normalcy make the process challenging. Prominent psychological studies on survivors and health care workers during various viral epidemics like SARS, Zika virus and Ebola are available in literature. Maunder et al. [21] reported the psychosomatic and work-related impact of SARS outbreak in an academic hospital. Verma et al. [22] reported the psychological effects on health care workers post SARS. In 2015, Yadav and Rawal [23] studied the mental health status of Ebola survivors in Western Africa. Shultz et al. [24] provided insights to the common mental disorders (anxiety and depressive disorders, posttraumatic stress disorder) within the affected nations of West Africa during the Ebola outbreak. In 2016, Oliveira et al. [25] studied the psychological health of mothers of neonates with congenital Zika virus syndrome (ZVS). In 2018, de Souza et al. [26] studied the impact of mental health of parents with babies born with ZVS. Hossain et al. [27] reports the adverse effects on mental health because of quarantine and isolation for infection prevention.
Recent studies on impact of coronavirus disease 2019 (COVID-19) on mental health and need of psychological intervention have been reported [28, 29, 30]. Liu [31] presents the mental health effects of COVID-19 pandemic in China. Both cross-sectional and focused studies (isolation of elderly [32], dementia care [33], medical staff [34] and home confinement on children [35]) are available. Lai et al. [36] identified degree of symptoms and testified mental health outcomes of 1257 health care workers in China who are treating COVID-19 patients. It reported the need of intervention and support to frontline nurses. Huang & Zhao [37] conducted a web-based cross-sectional survey to find generalized anxiety, depressive signs and sleep quality during COVID-19 epidemic in China. Park & Park [38] emphasized the need for mental health care during the COVID-19 outbreak in Korea. The studies are mostly country-specific for China, Korea or United Kingdom. The novelty of this paper is two-fold. Firstly, the study assesses the mental outcomes during the ongoing COVID-19 crisis and enforced lockdown in India and secondly it proffers an artificial intelligence-based solution to tackle the psychological epidemic it may lead too. Machine learning algorithms can facilitate discovery of significant behavioral biomarkers to help mental healthcare practitioners in deciding if a patient has probability of developing a specific psychological syndrome. Additionally the algorithms can aid in tracking the efficiency of a treatment plan. The psychological disorder prediction tool is thus proposed in view of the same.
4.Psychological disorder prediction (PDP) tool
This research proffers a cognitive model for psychological disorder predictive analytics. The proposed Psychological Disorder Prediction (PDP) tool uses a supervised machine learning algorithm to train the model to classify the participants into two pre-defined, anxiety and mood disorder categories. The tool aims to suggest individuals who may need psychological support or interventions. The architecture of the PDP tool is shown in Fig. 6.
Figure 6.
Architecture of PDP tool.
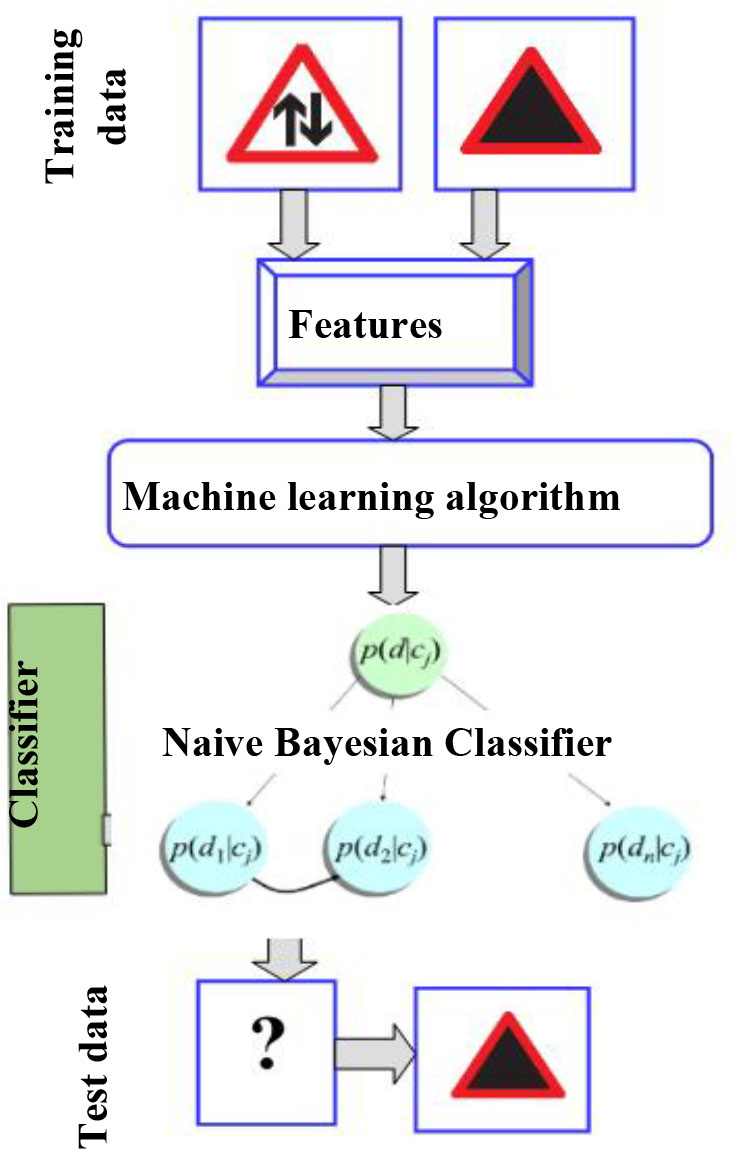
4.1Participant dataset creation and feature extraction
Generically, there are four different data collection techniques – observation, questionnaire, interview and focus group discussion [39]. In this work, the dataset was prepared by evaluating the responses of an online mental health questionnaire. The response were recorded from 26
Figure 7.
Gender distribution.
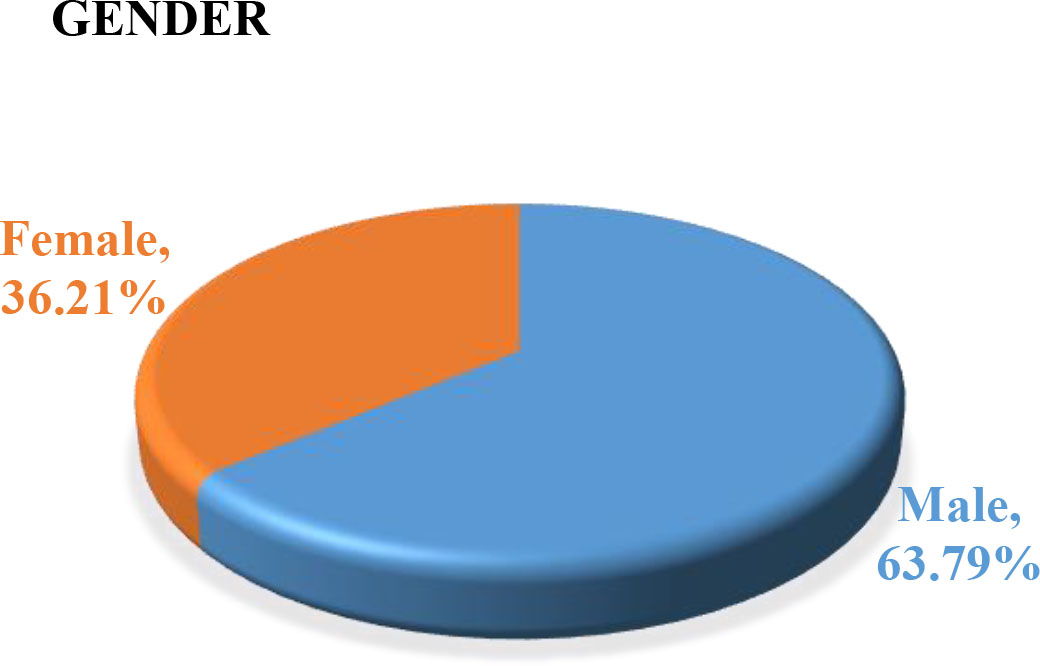
Figure 8.
Age distribution.
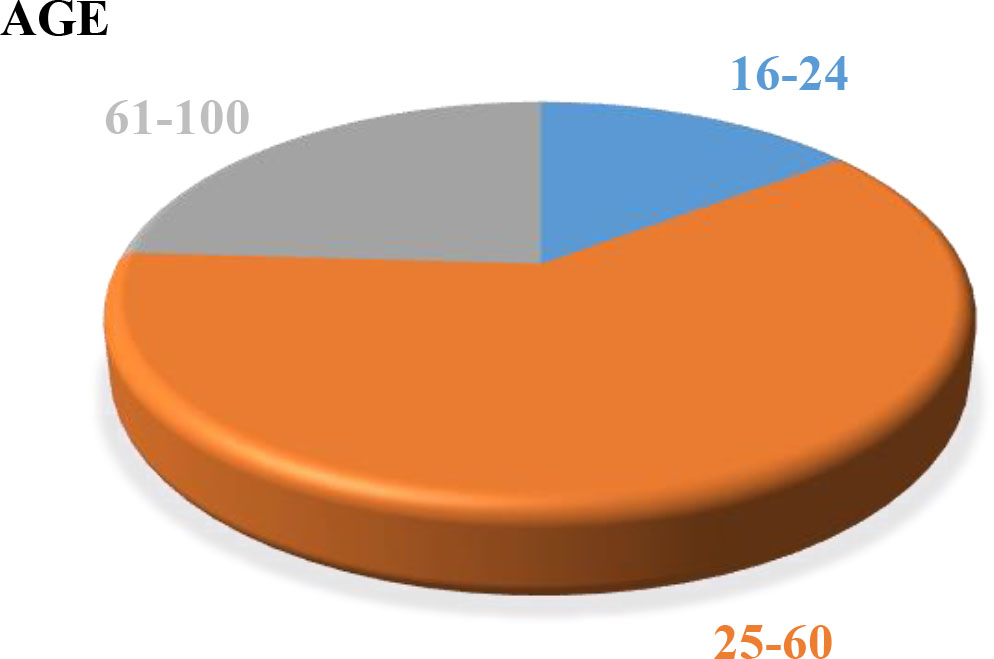
Figure 9.
Occupation distribution.
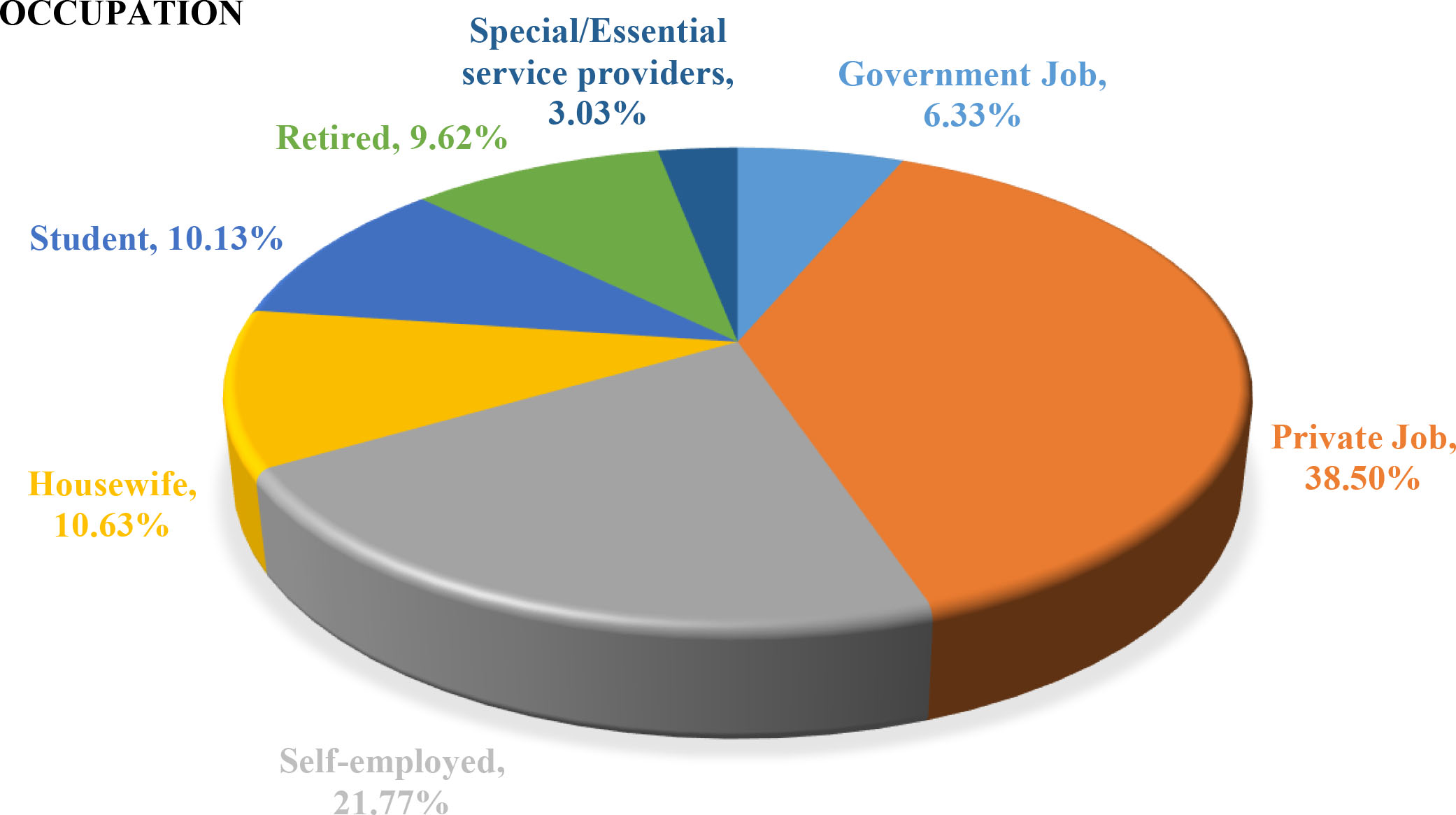
Table 1
MHQ COVID-19 symptomatic markers for the respective disorders
No. | Are you experiencing (symptoms):- | Feeling description | Disorder type |
---|---|---|---|
i. | Separation from loved ones | Separation anxiety disorder | Anxiety disorder |
ii. | Loss of freedom | Feeling threatened and anxious | Anxiety disorder |
iii. | Boredom | Feeling of restlessness, depression is stacked boredom | Anxiety disorder |
iv. | Stigmatization | Exposure to stressful/traumatic event | Anxiety disorder |
v. | Washing/cleaning compulsion | Obsessive-compulsive disorder | Anxiety disorder |
vi. | Phobia that you have COVID-19 | Specific phobias, health anxiety | Anxiety disorder |
vii. | Financial stress | Adjustment disorder (sudden change/loss/disappointment) | Anxiety disorder |
viii. | Job stress | Adjustment disorder (sudden change/loss/disappointment) | Anxiety disorder |
ix. | Panic buying/compulsive shopping | Mania | Mood disorder |
x. | Irritability | Depressive episode | Mood disorder |
xi. | Lack of interest in activity previously enjoyed | Depressive episode | Mood disorder |
xii. | Suicidal thoughts/thoughts of self-harm | Depressive episode | Mood disorder |
xiii. | Unpredictable mood swings | Depressive episode | Mood disorder |
xiv. | Feeling of guilt/worthlessness | Depressive episode | Mood disorder |
xv. | Lack of concentration | Depressive episode | Mood disorder |
xvi. | Significant weight gain/loss | Depressive episode | Anxiety disorder/mood disorder |
xvii. | Altered sleep habits (less sleep, more sleep, no change, disturbed sleep, sleep walking/sleep taking) | Sleep-wake disorder | Anxiety disorder/mood disorder |
xviii. | Altered eating habits (don’t each much, eating more-binge-eating, no change) | Feeding-eating disorder | Anxiety disorder/mood disorder |
Generally, to measure depression a benchmark Patient Health Questionnaire, PHQ-9 [40] which consists of nine symptomatic questions to detect depression and its severity is used. But as the intent of this work was to identify the onset of specific psychological disorders, namely anxiety and mood disorders during this corona crisis, a COVID-19 Mental Health Questionnaire (MHQ COVID-19) is designed. This web-based questionnaire consisted of questions characterizing 18 prominent symptomatic markers for the respective disorders. As discussed earlier, there is a significant overlap in the symptoms of both the disorders but the cumulative results of symptoms were used to predict the final class of disorder. The following Table 1 depicts the symptomatic queries with their respective descriptions and disorder categories:
Each symptom is used as feature to train the classifier which can predict the disorder type. The feature vector is created using these 18 symptomatic markers where the feature value is set to ‘1’ on the presence of symptom, else it is set to ‘0’.
4.2Binary classification using supervised machine learning
Supervised learning is the learning process when the outcome variable is known, and that this information is explicitly used in training (supervised). Classification in machine learning (ML) is the task of segregating instances of input into various classes based on previous training input [41]. It is a supervised task, wherein a number of labelled training instances are given to the ML classifier as input. The classifier learns from the training sample. Then the performance is tested on a sample of test instances.
In this work, a multinomial Naïve Bayes Classifier [42] is used. Naive Bayes is a family of probabilistic algorithms that take advantage of probability theory and Bayes’ Theorem to predict the category of a sample. It predicts a class by taking strong independence assumptions over features/predictors. Every feature/predictor
(1)
where,
A naive Bayes classifier which uses a multinomial distribution for each of the features is referred to as a Multinomial Naive Bayes. NB is an easy to implement, simple, scalable and a fast technique. It is a generative model as compared to logistic regression (LR), decision trees (DT) or support vector machines (SVM) which are discriminative. With the small training data, LR and DT model estimates may over fit the data. An interesting finding is that NB can perform better when there is low amount of training data. Another interesting characteristic of NB is that, when computational time is a critical factor these classifiers may be quickly learned from a dataset by means of the original inductors.
Figure 10.
Distribution of symptomatic markers in MHQ COVID-19.
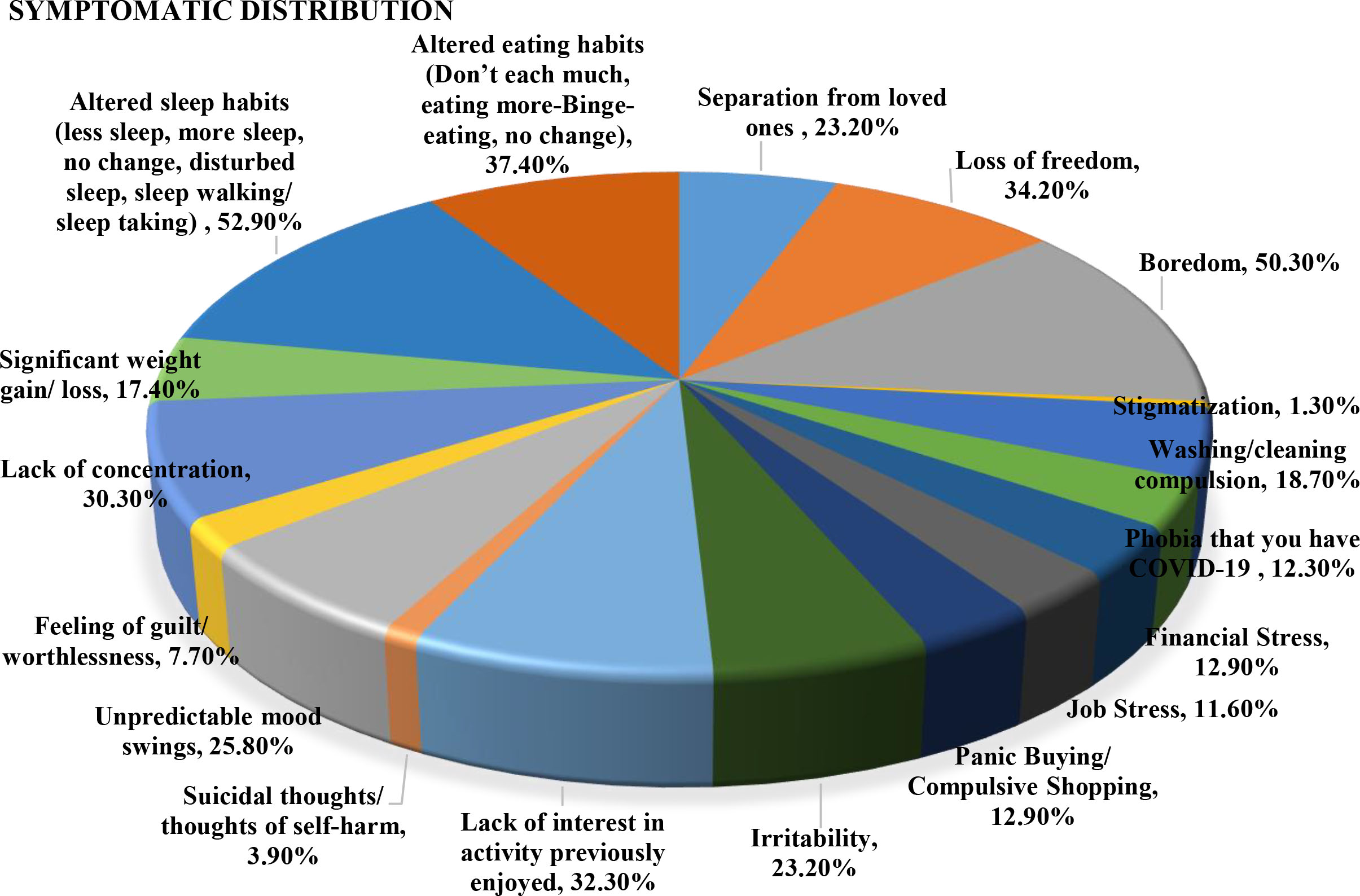
5.Results and discussion
The result assessment is divided into two subsections. Firstly the outcomes of the web-based MHQ COVID-19 is presented in Section 5.1 followed by the evaluation of the proposed PDP tool in the next Section 5.2.
5.1MHQ COVID-19 outcomes
The result of survey clearly indicate the adverse effects on mental health because of lockdown for infection prevention. Almost 86% of the participants had self-reported symptoms of anxiety and depression. The distribution of symptomatic markers is shown in Fig. 10. The top five in descending order of distribution were altered sleep habits, boredom, altered eating habits, loss of freedom and lack of interest in activity previously enjoyed.
The separation of 395 records into anxiety and mood disorder types was 60.75% for AD and 39.24% for mood. It was also observed that the number of females with MD were more in comparison to males. Figures 11 and 12 show the Male-female case distribution for MD and AD respectively.
Figure 11.
Male-female distribution for MD.
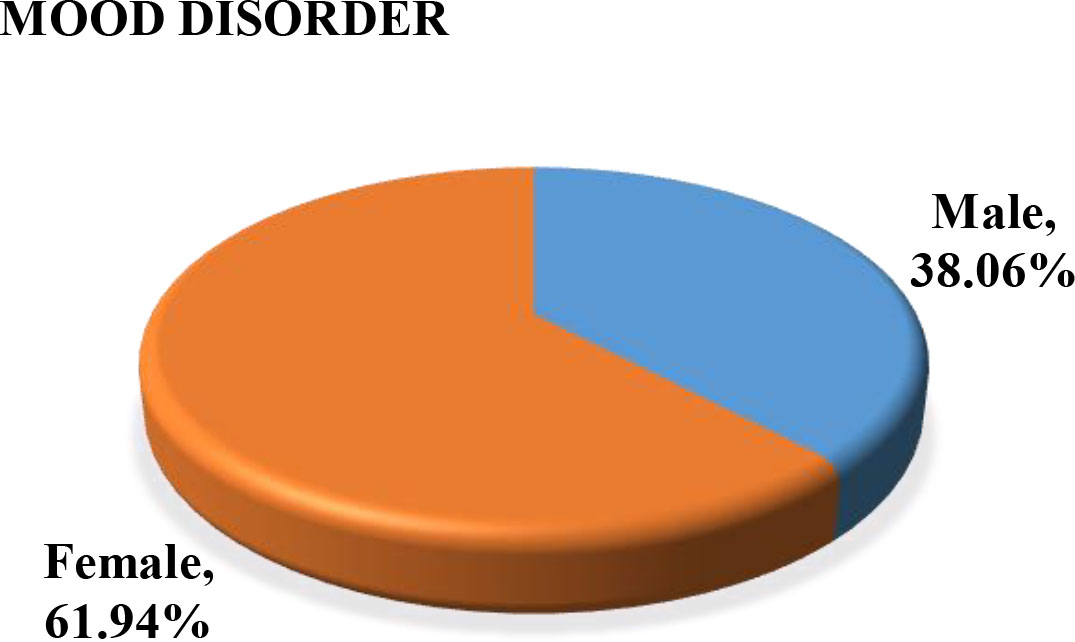
Figure 12.
Male-female distribution for AD.
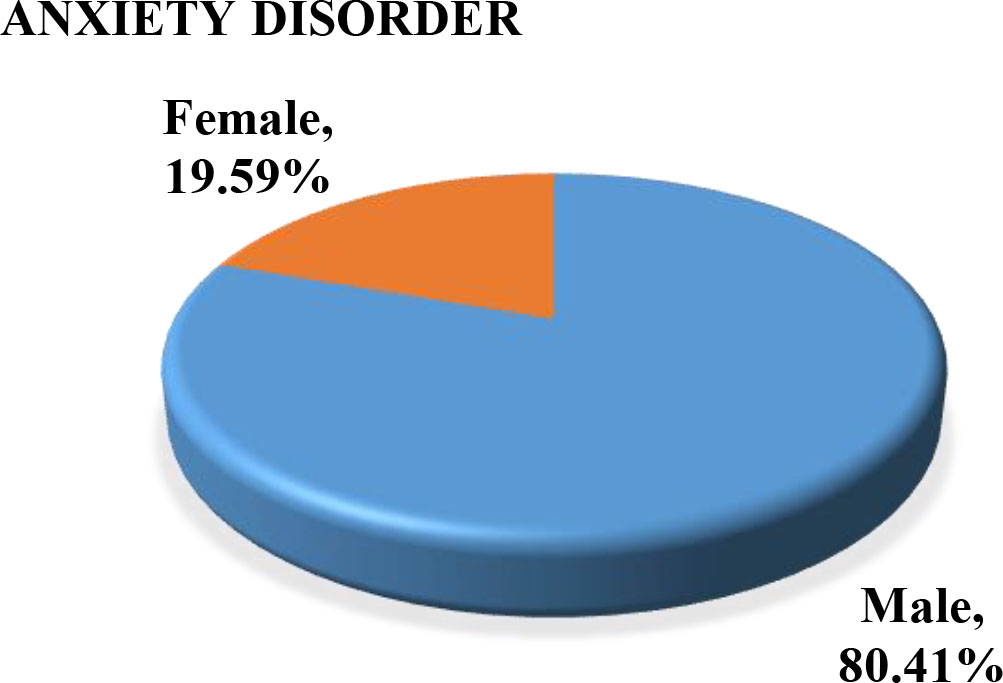
It was also noted that out of 80.41% male with AD, nearly 50% were in private job and 20% were self-employed where as in case of MD out of 61.94% females around 42% were housewife followed by 26% in private job. The student category had the minimal number of AD cases. Also, out of the 45 female and 27 male NRIs the distribution of MD and AD in females was 53.4% and 46.6% respectively, whereas NRI men had nearly equal distribution of disorders. The results are clearly indicative of mass onset of Coro-anxiety and the need of early intervention to immobilize this big silent killer.
5.2Performance of PDP tool
The proposed PDP tool was evaluated for classification performance using to ROC-AUC curve. The participant dataset of 395 records was labelled for anxiety and mood disorder. It comprised of 240 records labelled as AD and 155 labelled as MD. The classification results were assessed by partitioning the dataset into training and test sets. 10-fold cross-validation was performed to create a validation set and find the best parameters. The training has been performed on 80% of the dataset. The prediction results are shown using the confusion matrix (Fig. 13). It helps to visualize the proportions between the predicted and true label for both the datasets to calculate the performance of the developed model using some evaluation metrics.
Figure 13.
Confusion matrix for classifier performance.
Predicted class | |||
---|---|---|---|
Positive | Negative | ||
Actual class | Positive | True Positive (TP) | False Negative (FN) |
Negative | False Positive (FP) | True Negative (TN) |
To evaluate the performance of the classifier, we calculated precision, recall and accuracy [43], where
(2)
(3)
(4)
Figure 14 depicts the confusion matrix of the PDP classifier. An accuracy of 92.15% was achieved. The recall and precision values were 0.9485 and 0.9208 respectively.
Figure 14.
Confusion matrix for PDP tool.
Predicted class | |||
---|---|---|---|
AD | MD | ||
Actual class | AD | 221 | 19 |
MD | 12 | 143 |
The ROC-AUC curve of the proposed PDP tool is shown in Fig. 15. ROC stands for Receiver Operating Characteristic. ROC is a probability curve which is created by plotting the true positive rate (TPR) against the false positive rate (FPR) at various threshold settings. Area under the ROC curve (i.e. AUC) tells how much model is capable of distinguishing between classes. The model that has higher TRP for each point of FPR will give better performance, that is higher the AUC, better the model is at predicting true class labels with max probability.
Table 2
Comparative analysis of different classifiers
Classifier | Precision | Recall | Accuracy |
---|---|---|---|
NB | 0.9208 | 0.9485 | 92.15% |
DT | 0.8250 | 0.8733 | 89.10% |
SVM | 0.9202 | 0.9470 | 91.86% |
K-NN | 0.9170 | 0.9480 | 91.85% |
LR | 0.9162 | 0.9270 | 91.80% |
Figure 15.
ROC-AUC curve of PDP tool.
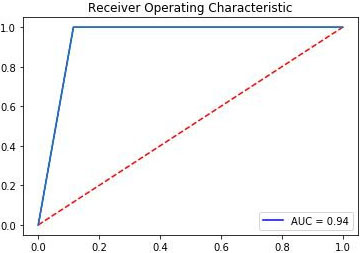
A comparative analysis with four other classification algorithms, namely, Decision Tree (DT), Support vector machine (SVM), k-nearest neighbor (K-NN) and Logistic Regression (LR) was also done to compare it with the Naïve Bayes Classifier (NB). It was observed that NB outperformed all the other classifiers in terms of accuracy (Fig. 16).
Figure 16.
Accuracy of classifiers.
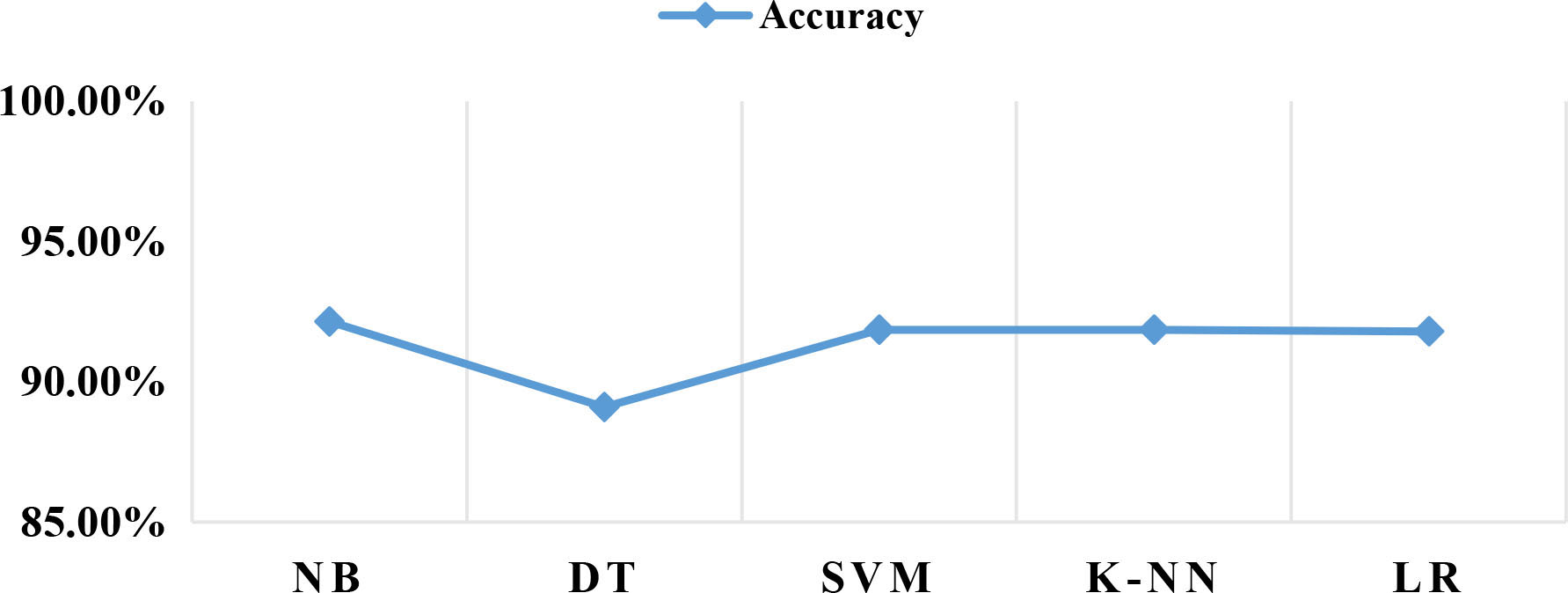
The comparative analysis of the classification algorithms is given in Table 2.
The least precision obtained was for the DT classifier and correspondingly a lower accuracy tree. This is because tree splitting is locally greedy and therefore DT is more likely to get stuck in local optima.
6.Conclusion
The corona pandemic is accelerating at an exponential rate. India entered a total lockdown after spikes in cases. Lockdowns are just a beginning. People are exposed to intense psychological trauma, both individually and collectively, caused not only by the direct consequences of the pandemic – bereavement and illness – but also by indirect, and non-trivial consequences such as economic recession, job loss, pay-cuts, separation from loved ones due to travel bans, post-traumatic and quarantine stress. We used a cross-sectional, survey-based method to find to understand the negative effect of lockdown on population’s mental health. A machine learning based psychological disorder tool was designed to assess the need of early intervention and support. The performance of the tool is motivating and as the tool generate insights for delivering a near real-time intelligence, it can be integrated to the psychological first aid framework during the COVID-19 crisis.
The limitations of the study is that it was carried out for a period of one week and lacked longitudinal follow-up. There has been a steep rise in the number of cases and the death toll has increased too. Amid these rising coronavirus cases in India, the emotions can further be triggered spiking depression and anxiety. Also, the lockdown has further been extended to first week of May. Therefore, long-term psychological consequences need to be probed. Though 85% of the respondents exhibited clinically meaningful symptoms but a response-bias may still exist. As there is overlap in symptoms of both the disorders, data from wearable sensors and biological markers can be used do enable a fine-grain prediction. Further, the use of ensemble classifiers to improve the performance can be investigated too. Additionally, the study relies on hand-crafted features which is efficient with the current data size, the use of deep learning can be a promising future direction if large data is available as these can learn hierarchical representation of features and improve the training performance.
Notes
Acknowledgments
I am thankful to Gp. Capt. Anurag Kumar (Retd.), Indian Air Force, India and Dr. Alpa Kumar (MBBS, FRCGP), GP, London, United Kingdom for their insights and support. I thank all the participants for their contribution and cooperation.
References
[1] | Himma KE. The concept of information overload: A preliminary step in understanding the nature of a harmful information-related condition. Ethics and Information Technology. (2007) ; 9: (4): 259-272. |
[2] | Luxton DD. Artificial intelligence in psychological practice: Current and future applications and implications. Professional Psychology: Research and Practice. (2014) ; 45: (5): 332. |
[3] | Luxton DD. An introduction to artificial intelligence in behavioral and mental health care. in: Artificial Intelligence in Behavioral and Mental Health Care. (2016) ; 1-26. |
[4] | Doraiswamy PM, Blease C, Bodner K. Artificial intelligence and the future of psychiatry: Insights from a global physician survey. Artificial Intelligence in Medicine. (2020) ; 102: : 101753. |
[5] | Lai CC, Shih TP, Ko WC, Tang HJ, Hsueh PR. Severe acute respiratory syndrome coronavirus 2 (SARS-CoV-2) and corona virus disease-2019 (COVID-19): The epidemic and the challenges. International Journal of Antimicrobial Agents. (2020) ; 105924. |
[6] | Menon VK. Prediction of number of cases expected and estimation of the final size of coronavirus epidemic in India using the logistic model and genetic algorithm. arXiv preprint arXiv: 2003.12017. (2020) . |
[7] | Kumar S. Biochemistry of the Brain. in: Examining Biological Foundations of Human Behavior. (2020) ; 47-59. IGI Global. |
[8] | World Health Organization. Depression and other common mental disorders: Global health estimates. World Health Organization; (2017) . |
[9] | Tyrer P, Baldwin D. Generalised anxiety disorder. The Lancet. (2006) ; 368: (9553): 2156-2166. |
[10] | Hirschfeld RM. The Mood Disorder Questionnaire: A simple, patient-rated screening instrument for bipolar disorder. Primary care Companion to the Journal of Clinical Psychiatry. (2002) ; 4: (1): 9. |
[11] | Malhi GS, Bassett D, Boyce P, Bryant R, Fitzgerald PB, Fritz K, Hopwood M, Lyndon B, Mulder R, Murray G, Porter R. Royal Australian and New Zealand college of psychiatrists clinical practice guidelines for mood disorders. Australian & New Zealand Journal of Psychiatry. (2015) ; 49: (12): 1087-1206. |
[12] | Graham S, Depp C, Lee EE, Nebeker C, Tu X, Kim HC, Jeste DV. Artificial intelligence for mental health and mental illnesses: An overview. Current Psychiatry Reports. (2019) ; 21: (11): 116. |
[13] | Zhou D, Luo J, Silenzio VM, Zhou Y, Hu J, Currier G, Kautz H. Tackling mental health by integrating unobtrusive multimodal sensing. InTwenty-Ninth AAAI Conference on Artificial Intelligence. (2015) . |
[14] | Lim CK, Chia WC. Analysis of single-electrode EEG rhythms using MATLAB to elicit correlation with cognitive stress. International Journal of Computer Theory and Engineering. (2015) ; 7: (2): 149. |
[15] | Kessler RC, van Loo HM, Wardenaar KJ, Bossarte RM, Brenner LA, Cai T, Ebert DD, Hwang I, Li J, de Jonge P, Nierenberg AA. Testing a machine-learning algorithm to predict the persistence and severity of major depressive disorder from baseline self-reports. Molecular Psychiatry. (2016) ; 21: (10): 1366-1371. |
[16] | Reece AG, Reagan AJ, Lix KL, Dodds PS, Danforth CM, Langer EJ. Forecasting the onset and course of mental illness with Twitter data. Scientific Reports. (2017) ; 7: (1): 1-1. |
[17] | Srividya M, Mohanavalli S, Bhalaji N. Behavioral modeling for mental health using machine learning algorithms. Journal of Medical Systems. (2018) ; 42: (5): 88. |
[18] | Aggarwal S, Saluja S, Gambhir V, Gupta S, Satia SP. Predicting likelihood of psychological disorders in Player Unknown’s Battlegrounds (PUBG) players from Asian countries using supervised machine learning. Addictive Behaviors. (2020) ; 101: : 106132. |
[19] | Buettner R, Grimmeisen A, Gotschlich A. High-performance diagnosis of sleep disorders: A novel, accurate and fast machine learning approach using electroencephalographic data. in: Proceedings of the 53rd Hawaii International Conference on System Sciences. (2020) . |
[20] | Buettner R, Beil D, Scholtz S, Djemai A. Development of a machine learning based algorithm to accurately detect schizophrenia based on one-minute EEG recordings. in: Proceedings of the 53rd Hawaii International Conference on System Sciences. (2020) . |
[21] | Maunder R, Hunter J, Vincent L, Bennett J, Peladeau N, Leszcz M, Sadavoy J, Verhaeghe LM, Steinberg R, Mazzulli T. The immediate psychological and occupational impact of the 2003 SARS outbreak in a teaching hospital. Cmaj. (2003) ; 168: (10): 1245-1251. |
[22] | Verma S, Mythily S, Chan YH, Deslypere JP, Teo EK, Chong SA. Post-SARS psychological morbidity and stigma among general practitioners and traditional Chinese medicine practitioners in Singapore. Ann Acad Med Singapore. (2004) ; 33: (6): 743-748. |
[23] | Yadav S, Rawal G. The current mental health status of Ebola survivors in Western Africa. Journal of Clinical and Diagnostic Research: JCDR. (2015) ; 9: (10): LA01. |
[24] | Shultz JM, Baingana F, Neria Y. The 2014 Ebola outbreak and mental health: Current status and recommended response. Jama. (2015) ; 313: (6): 567-568. |
[25] | dos Santos Oliveira SJ, Dos Reis CL, Cipolotti R, Gurgel RQ, Santos VS, Martins-Filho PR. Anxiety, depression, and quality of life in mothers of newborns with microcephaly and presumed congenital Zika virus infection: A follow-up study during the first year after birth. Archives of Women’s Mental Health. (2017) ; 20: (3): 473-475. |
[26] | de Souza LE, de Lima TJ, Ribeiro EM, Pessoa AL, Figueiredo TC, Lima LB. Mental health of parents of children with congenital Zika virus syndrome in Brazil. Journal of Child and Family Studies. (2018) ; 27: (4): 1207-1215. |
[27] | Hossain MM, Sultana A, Purohit N. Mental health outcomes of quarantine and isolation for infection prevention: A systematic umbrella review of the global evidence. Available at SSRN 3561265. (2020) . |
[28] | Duan L, Zhu G. Psychological interventions for people affected by the COVID-19 epidemic. The Lancet Psychiatry. (2020) ; 7: (4): 300-302. |
[29] | Xiang YT, Yang Y, Li W, Zhang L, Zhang Q, Cheung T, Ng CH. Timely mental health care for the 2019 novel coronavirus outbreak is urgently needed. The Lancet Psychiatry. (2020) ; 7: (3): 228-229. |
[30] | Liu D, Ren Y, Yan F, Li Y, Xu X, Yu X, Qu W, Wang Z, Tian B, Yang F, Yao Y. Psychological impact and predisposing factors of the coronavirus disease 2019 (COVID-19) pandemic on general public in China. |
[31] | Ho CS, Chee CY, Ho RC. Mental Health Strategies to Combat the Psychological Impact of COVID-19 Beyond Paranoia and Panic. Annals of the Academy of Medicine, Singapore. (2020) ; 49: (1): 1. |
[32] | Yang Y, Li W, Zhang Q, Zhang L, Cheung T, Xiang YT. Mental health services for older adults in China during the COVID-19 outbreak. The Lancet Psychiatry. (2020) ; 7: (4): E19. |
[33] | Wang H, Li T, Barbarino P, Gauthier S, Brodaty H, Molinuevo JL, Xie H, Sun Y, Yu E, Tang Y, Weidner W. Dementia care during COVID-19. The Lancet. (2020) . |
[34] | Chen Q, Liang M, Li Y, Guo J, Fei D, Wang L, He L, Sheng C, Cai Y, Li X, Wang J. Mental health care for medical staff in China during the COVID-19 outbreak. The Lancet Psychiatry. (2020) ; 7: (4): E15-e16. |
[35] | Wang G, Zhang Y, Zhao J, Zhang J, Jiang F. Mitigate the effects of home confinement on children during the COVID-19 outbreak. The Lancet. (2020) ; 395: (10228): 945-947. |
[36] | Lai J, Ma S, Wang Y, Cai Z, Hu J, Wei N, Wu J, Du H, Chen T, Li R, Tan H. Factors associated with mental health outcomes among health care workers exposed to coronavirus disease 2019. JAMA Network Open. (2020) ; 3: (3): E203976. |
[37] | Huang Y, Zhao N. Generalized anxiety disorder, depressive symptoms and sleep quality during COVID-19 epidemic in China: A web-based cross-sectional survey. medRxiv. (2020) . |
[38] | Park SC, Park YC. Mental health care measures in response to the 2019 novel coronavirus outbreak in Korea. Psychiatry Investigation. (2020) ; 17: (2): 85. |
[39] | Chaleunvong K. Data collection techniques. Training Course in Reproductive Health Research Vientine. (2009) . |
[40] | Gilbody S, Richards D, Barkham M. Diagnosing depression in primary care using self-completed instruments: UK validation of PHQ-9 and CORE-OM. Br J Gen Pract. (2007) ; 57: (541): 650-652. |
[41] | Alzubi J, Nayyar A, Kumar A. Machine learning from theory to algorithms: An overview. in: Journal of Physics: Conference Series. (2018) ; 1142: (1): 012012. IOP Publishing. |
[42] | Xu S, Li Y, Wang Z. Bayesian multinomial Naïve Bayes classifier to text classification. in: Advanced Multimedia and Ubiquitous Engineering. (2017) ; 347-352. Springer, Singapore. |
[43] | Powers DM. Evaluation: From precision, recall and F-measure to ROC, informedness, markedness and correlation. |