Research data management in the age of big data: Roles and opportunities for librarians
Abstract
In the age of “big data,” scientific researchers are increasingly struggling with how to manage, organize, and make sense of the vast amount of data that often characterizes scientific research in the 21st century. With their expertise in knowledge management, information professionals can be valuable collaborators for research teams facing these challenges. This article discusses just a few of the myriad opportunities for librarians and other information professionals to become involved with research teams and provide valuable support for the management, analysis, and preservation of research data.
1.Introduction
The ways that scientific research are practiced have shifted fundamentally in the last several decades. Researchers of the 21st century often rely on large digital datasets, and sometimes they are using data that they themselves did not gather, but that they obtained from public sources for reuse. Researchers in many fields must comply with new policies from funders and journals that require them to share their data or write data management plans, tasks that most researchers have not had to undertake in the past. Whatever a researcher’s particular field of study, chances are good that the ways that research is conducted have substantively changed in the last several years. As a result, researchers may find that they need new skills and knowledge to work most effectively and take advantage of the new opportunities that this age of data-driven research presents.
In the face of this rapidly evolving research landscape, where can researchers look for assistance with their emerging needs? In many cases, librarians and information professionals can assist researchers who need guidance regarding many different aspects of working with data. Given librarians’ expertise in knowledge management and their longstanding role in the research cycle, librarians are well-situated to provide guidance and even collaborate with research teams on various aspects of data management and data science. This paper considers how research has evolved in the age of big data and how librarians and other information professionals can respond to researchers’ emerging needs.
2.The age of big data
The term “big data” is often used as a buzzword in discussions of the challenges involved in research data management, but what exactly makes data big? How big must the data get to be thought of as big data? Most importantly, how is “big data” different from “small data,” and why do these differences matter to researchers and others who work with research data management?
One useful definition involves four dimensions that typify big data: volume, velocity, variety, and veracity, or the four V’s [18]. Volume describes the scale of data; there is no single defined amount that delineates big data from small data, but the term big data is generally applied to datasets large enough that traditional data processing and storage techniques cannot be used. For example, techniques like parallel or distributed computing involve several computers working in concert with each other to analyze data, enabling analysis of datasets that would be too large for a single processor to handle [10]. Velocity refers to the speed at which data are generated; data are being generated all around us, all day, every day, at an unprecedented rate. Indeed, it has been estimated that 2.5 quintillion bytes of new data are generated every single day [19]. Variety refers to the many different types of data that may comprise a dataset. A single dataset may contain a wide variety of data, including images, audio and video, free text, structured data, and more. Finally, veracity is included in the four V’s to emphasize the importance of ensuring the reliability and integrity of data. With concerns about research accountability and transparency increasing in the wake of several high-profile cases of irreproducible research, and as data sharing and reuse become more commonplace, it is more important than ever that data be error-free and accurate.
The growth of data in general, and scientific research data in particular, has been driven by a number of social and technological factors. First, new technologies have made it possible to gather data more quickly and cheaply than ever before. The trajectory of genome sequencing exemplifies the ways that technology has contributed to a dramatic increase in available data. The first human genome was sequenced in 2003, under the auspices of the Human Genome Project; doing so took about ten years and cost about $2.7 billion [25]. Less than fifteen years later, we can now do that same work in just twenty-six hours [22], and we have reached the much-sought-after $1,000 price tag for sequencing a human genome [6]. The dramatic decreases in sequencing price and time are impressive in their own right, bringing us closer to achieving the promise of medical treatments that are more effective and cause fewer side effects because they are tailored to our personal genome. Making these advances even more impressive is the fact that genome sequencing has far exceeded the standard set by Moore’s Law, which suggests that computing power typically doubles every two years. As Fig. 1 demonstrates, genome sequencing costs are far below what might be expected using the assumptions in Moore’s Law, particularly since 2008 [40]. Not only can we generate data more quickly and cheaply than ever before, but we can also afford to store it, as data storage costs have also dramatically decreased in recent years. Cloud storage solutions like those provided by Google and Amazon make it easy and cheap to store unprecedented amounts of data [15].
New technologies have also led to an increase in the amount of “born-digital” data – materials that are originally created in digital form, rather than those that are created as analog data and subsequently digitized. For example, the widespread adoption of electronic health records means increasingly easy access to a wealth of patient data that was once difficult to utilize because it sat in hand-written paper files in clinicians’ offices [7]. Digitizing all of those records would have been an unreasonably difficult and time-consuming task, but now that patient data are gathered in electronic form as a matter of course, secondary research use is feasible (though patient privacy remains a concern). New types of data arising out of the internet, such as social media data, also have sometimes surprising research potential. Researchers have used social media sites like Twitter and Facebook for unexpected research purposes, like pharmacovigilance [1,4] and disease surveillance [5,16].
Not only do we live in an age of big data, but much of that data is freely and widely available. As of this writing, the Registry of Research Data Repositories (re3data) lists over fifteen hundred repositories providing access to a variety of different types of data, much of it with unrestricted access [32]. Researchers who wish to share their data (and those who wish to locate it) now have many choices about where to do so. A wide variety of subject-specific repositories make it easy for researchers to locate data that will be relevant to them or to find a home for the data they would like to share. These repositories’ scopes range from the very broad (such as DataONE, which hosts Earth and environmental data in general [9]), to the very specific (such as Mouse Genome Informatics, which accepts, as its name would imply, data related to the mouse genome [35]). Some universities and research institutions host data repositories on behalf of their researchers, such as University of Minnesota’s DRUM repository [38]. Often, these institutional repositories are run under the auspices of (or at least with the involvement of) the university library. General repositories like figshare [14] and Dryad [11] are subject-agnostic and have few restrictions on data formats, standards, or scope. Together, these many repositories, with their different scopes and focuses, provide access to a wealth of data.
Fig. 1.
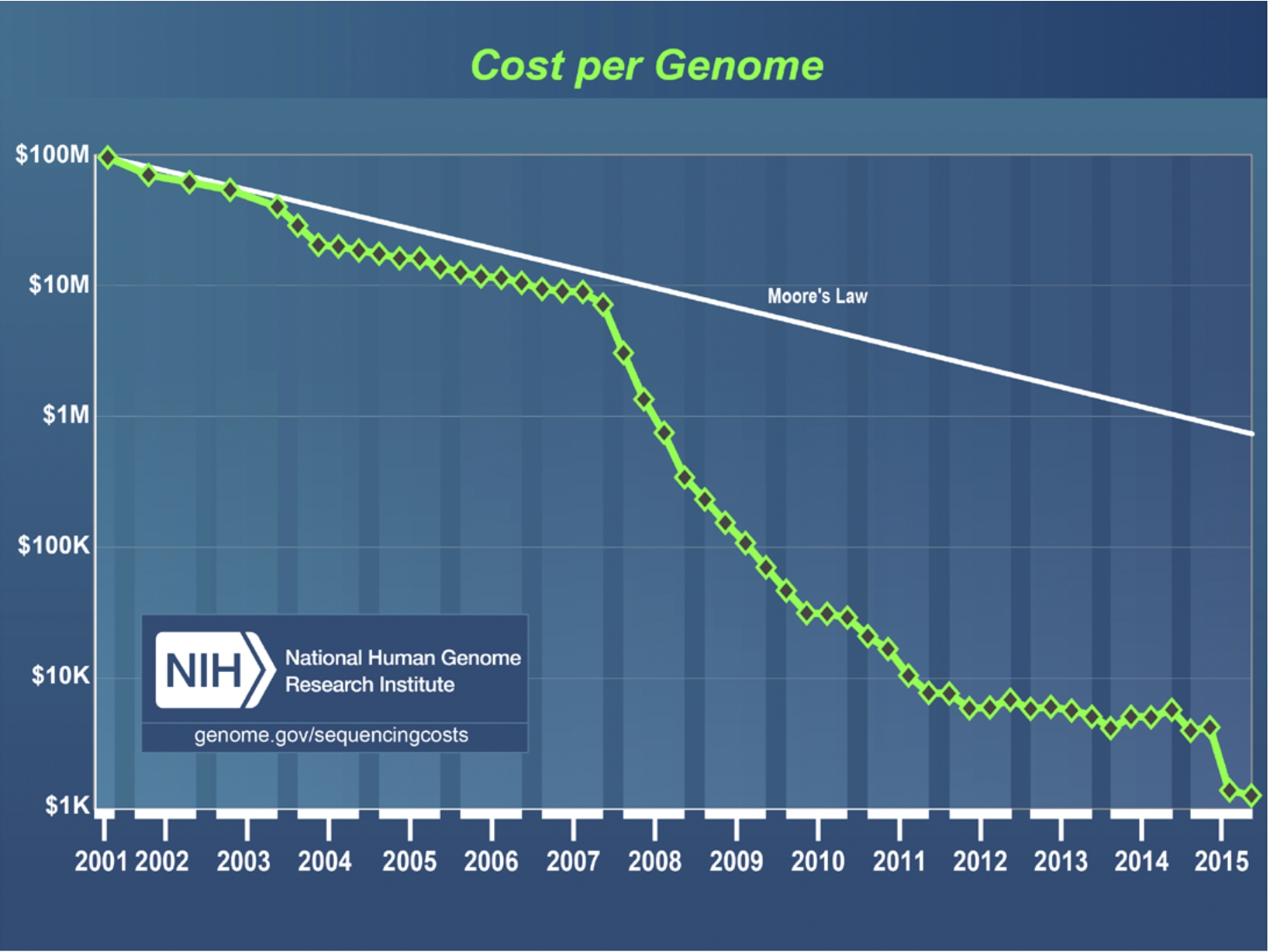
The vast amount of data already available in these repositories demonstrates that many researchers are open to sharing their data, though research has demonstrated that researchers’ willingness to share their data may vary widely depending on a variety of factors, including their field of research, years of experience in the field, and type of funding [13,31,34]. However, regardless of whether a researcher feels open to sharing their data or not, chances are good that most researchers will soon be required to do so. Many major journals, including the Nature journals [30], the Science journals [2], and the PLOS journals [33] have adopted data sharing policies; typically, these policies require that data related to the article be publicly available at the time of the article’s publication. In addition, many federal and private funders have also adopted data sharing policies. In 2013, the United States Office of Science and Technology Policy (OSTP) issued a memo to heads of federal agencies that fund $100 million or more in research and development annually. This memo directed the agencies to develop policies addressing dissemination of federally-funded research findings to the public, in the form of both journal articles reporting on those findings and the digital data arising from that research [17]. Though not all federal agencies have yet released their new policies in response to this memo, researchers should expect that they will soon be required to make their data somehow publicly accessible if they receive federal funding. The National Institutes of Health, for example, has issued a summary of their proposed policy, which indicates that all NIH-funded researchers, regardless of funding level or mechanism, will be required to submit a data management and sharing plan [26].
Are researchers ready to adapt to these various technological, cultural, and policy changes? A growing body of research suggests that, among researchers individually and the scientific community as a whole, the answer is often no. In 2012, the NIH convened a Data and Informatics Working Group to provide “expert advice on the management, integration, and analysis of large biomedical research datasets” [27]. Their report identified a variety of challenges facing the biomedical research community as research becomes more data-driven, including lack of infrastructure and standards to facilitate sharing data, gaps in training for researchers, and a need for new software and other IT solutions for handling data. Two years later, our team conducted research at the NIH Library to help inform the development of our Data Services training curriculum; respondents generally considered data management and related tasks highly relevant to their work, but indicated that they did not have a correspondingly high level of expertise in carrying out these tasks [12]. These issues are not unique to NIH researchers; researchers working at many different institutions and in many different fields of research face similar challenges in working with data effectively.
3.Library-based research data services
Many libraries that serve research communities have begun to develop services to support researchers’ data-related needs. Developing such services can be daunting for libraries just getting started with providing such services; framing services within the model of the research data life cycle can be a helpful approach for determining what services to develop, how to market them to the research community, and how to gauge success of the service. Although a variety of research data life cycle models exist [8,36,39], most generally contain variations on several common stages as follows:
Planning (potentially including creation of a formal data management plan, or DMP, to meet funder requirements),
Data collection or acquisition,
Data analysis or interpretation (including data visualization),
Data preservation and curation, and
Data sharing.
Understanding how researchers interact with their data at various points in the life cycle, as well as recognizing pain points for researchers, can help librarians develop targeted services to meet the particular needs of researchers at their institutions. In addition, freely available resources exist that can be useful at several stages of the life cycle; these resources can be of use to researchers directly, but may also be beneficial to librarians who have less experience in working with research data and would like to develop these skills.
Here I focus on four specific areas where the NIH Library (and other libraries) has established services: data management planning, data reuse, data visualization, and data sharing. Because the NIH Library’s Data Services program is relatively small (one full-time staff member dedicated primarily to Data Services) compared to the number of users it serves (though not all of the NIH’s more than eighteen thousand employees are researchers, a significant number do use the Library’s services), our approach emphasizes classroom training to provide researchers the skills they need to work successfully with data [28].
3.1.Planning
When the National Science Foundation (NSF) adopted its Data Management Plan (DMP) requirement in 2011, it was one of the first United States funders to do so [29]. The NSF’s policy requires all researchers seeking NSF funding to submit a plan about how they would make their data publicly available in accordance with the NSF’s data sharing policy, as well as how they would manage their data throughout the life of the project. Now, five years later, many federal and private funders have followed suit and created their own DMP and data sharing requirements. Even when researchers are not required to submit a formal DMP, determining how data will be organized, managed, preserved, and possibly shared can be a crucial first step in determining the answer to crucial logistical questions, such as how much funding will be needed and what type and amount of digital storage media will be required.
Many libraries have begun providing support to researchers who need to write a DMP, offering advice on how to write such a document and providing guidance on how local resources, like institutional repositories, can help researchers meet the obligations of data sharing policies. Because the DMP is a requirement for many grant-seekers, providing support for DMPs seems like a logical first step for libraries. In addition, providing library interventions at the planning stage of the research process can be much more effective than trying to assist researchers after they have already gathered their data and run into problems associated with poor data management practices. Though the NIH does not currently require submission of DMPs, the NIH Library’s Data Services curriculum emphasizes the importance of planning before beginning a research project to avoid these sorts of problems. Additional training and support will also be offered when the NIH’s DMP policy goes into effect.
For researchers who must write a DMP and the librarians who provide support for DMPs, the Data Management Planning Tool (DMPTool: see https://dmptool.org/) can provide useful guidance and demystify a potentially confusing process [37]. The DMPTool provides free, interactive support for writing DMP plans customized to several different funders. As it is frequently updated to reflect changing funder requirements, the DMPTool can be a useful and authoritative source for guidance on writing a DMP. Especially as additional funders begin requiring DMPs in response to the Office of Science and Technology (OSTP) memo on dissemination of research results, researchers will likely benefit from guidance on how to write an effective DMP.
3.2.Data reuse
Now that researchers have begun to share their data more freely, and repositories are increasingly available to facilitate access to those data, it’s easier for researchers to take advantage of existing data that they can reuse to address their own research questions. Indeed, it’s often possible for researchers to conduct an entire study using solely publicly-available data, without ever having to generate their own data. Though some critics have negatively labeled those who seek to reuse existing data as “research parasites” [21] data reuse can actually be beneficial to the research community and the broader public. Reusing existing data for new purposes potentially increases the return on the original funding investment; a 2014 study found that sharing data yielded additional returns of up to twelve times the original investment [3]. In the setting of biomedical research, data reuse can also shorten the lag between “bench” and “bedside,” or the time it takes to move from an initial scientific discovery to actual clinical use, currently estimated to be about seventeen years [23]. The goal of translational medicine is shortening that gap, and data sharing can play an important role in that process by cutting out the time it takes to gather data and allowing researchers to go straight to analysis.
Though researchers may have access to a wealth of shared data, many are not aware of available data resources. Even if they do wish to reuse existing datasets, researchers may be unsure of how to locate them, gain access to them, and utilize them for their research. Librarians with expertise in searching data resources and familiarity with the types of data those resources contain can be very helpful to researchers who are having difficulty locating existing datasets.
The NIH Library’s Data Services program provides assistance in locating datasets and, when necessary, guidance in negotiating access to closed data, consultation on data sharing agreements, and assistance with “wrangling” messy data. Even when datasets are organized in accordance with best practices, data rarely come ready for a researcher to use out of the box. Migration to a different format may be necessary when the original data collector used proprietary software. Even data in open formats may need work to recode variables, reorganize, or otherwise process data to move them from an organization that made sense for the original collector’s purposes to an organization that makes sense for the purposes of the researcher who will be reusing them. To address this challenge, the NIH Library’s training program emphasizes instruction in R, an open-source scripting and statistical programming language that, along with Python, is among the most commonly-used languages for scientific programming.
3.3.Data visualization
Though not part of the data management process strictly speaking, data visualization is an important skill for working effectively with data. Visualizations are often used to convey final research results and demonstrate the researcher’s findings, but data visualization may also be exploratory in nature, providing a means to visually identify patterns that might otherwise have been difficult to discern in complicated data. Network visualizations can reveal unexpected connections among individuals in a system, such as genes or proteins in a biological process or human beings in a social network. Time series visualizations can demonstrate the progression of a complex process over time, making it possible to better understand causal relationships and interconnected effects. Heat maps of complex datasets, like gene microarray data, make it possible to instantly see patterns through color variations, rather than sifting through thousands of individual numeric data points. These are only a few of the many types of visualizations that researchers can employ to elucidate meaning in their data and easily convey that meaning to others.
Data visualization might not initially seem like a natural fit for librarians, but in fact, libraries can be a logical place to situate data visualization services. Visualization services in libraries are in some ways analogous to the “makerspaces” that libraries have begun to offer, which provide space and tools for users to explore, innovate, and create using tools like 3D printers and digital design tools [20]. Many of the tools used in other stages of the data life cycle, such as R and Python, can be used for visualization, so librarians who develop expertise in these tools may find it a natural extension to provide visualization support. Finally, many librarians have expertise in visualization tools because they use them to analyze and visualize library-related data, such as bibliometrics data.
At the NIH Library, data visualization support is primarily offered in the form of training sessions. These sessions are taught by a variety of staff who have expertise in different aspects of visualization and different visualization tools, including Tableau, R, and specialized visualization tools such as Gephi for network visualization. In addition, the Library’s Technology Hub (which provides cutting-edge technology tools, like 3D printers, and software, like the Adobe Creative Suite) houses a sixty-five inch interactive data visualization touchscreen. Library users can use this touchscreen to interactively explore existing visualizations or create their own.
3.4.Data sharing
As this article has demonstrated, the scientific research ecosystem has increasingly emphasized the importance of sharing research data. Funder and journal policies that require data sharing are new to many researchers, and those who have not been accustomed to sharing their data may need guidance in best practices for doing so. Researchers’ needs can be as simple as determining an appropriate repository for storing data or as complex as de-identifying human subject data. Preparing data for submission to a repository can be a time-consuming and perplexing task; some repositories have very specific requirements about formatting and metadata. For example, the “Formatting Your Submission” section alone of The GenBank Submission Handbook contains over five thousand words and nine figures, with instructions as specific as “Do not put spaces around the ‘=’” [24] For users who have never submitted before, the process can be daunting, and having guidance can be helpful. Thus, the NIH Library provides consulting services for researchers who need guidance on how and where to share their data.
In some cases, researchers may wish to self-host their data on their laboratory’s website or in another convenient and publicly-accessible location. Though doing so may be a feasible and in some cases desirable choice, it is important that researchers recognize the ongoing commitment to curating and maintaining access to their data. In addition, researchers should consider how those who reuse the data will cite and refer back to the dataset. Digital object identifiers (DOIs) are becoming a standard for data citation. Because they are persistent, unique identifiers, DOIs have advantages over Uniform Resource Locators (URLs) typically associated with websites. The NIH Library is piloting a DOI minting service for NIH researchers who would like to obtain a DOI for their dataset (or other research-related digital objects, like software or code). This service will allow researchers to maintain control over their data by self-hosting, but still enable them to obtain a persistent, unique identifier that can be used to cite or share their data.
4.Opportunity ahead
Librarians around the world have had initial success in offering services to support researchers’ data-related needs, and these needs will likely only increase as time goes on. Increasingly, funders and journals are requiring data sharing and the submission of data management plans; even if they don’t already, researchers in many fields should expect that they will be required to comply with such policies within the next few years. As a result of such policies, the amount of freely- and publicly-available research data continues to increase exponentially. Researchers will likely need assistance in learning how to access and utilize these datasets.
Data science and data-intensive research are frequently team-based and interdisciplinary, relying on the varied expertise of many different collaborators. Librarians can potentially play an important role in such research, bringing expertise in information and knowledge management. At a time when many libraries face challenges to funding and must demonstrate their continued relevance, expanding services to encompass research data management and other data science services may be a useful way to provide novel types of support to their user communities.
About the author
Lisa Federer is a Research Data Informationist at the National Institutes of Health Library, where she provides training and support for researchers engaged in data science and data-intensive research. She received her Master of Library and Information Science from the University of California Los Angeles (UCLA) and professional certifications in data science from Georgetown University and data visualization from New York University (NYU).
References
[1] | N. Alvaro, M. Conway, S. Doan, C. Lofi, J. Overington and N. Collier, Crowdsourcing Twitter annotations to identify first-hand experiences of prescription drug use, Journal of Biomedical Information 58: ((2015) ), 280–287. doi:10.1016/j.jbi.2015.11.004. |
[2] | American Association for the Advancement of Science, Science: editorial policies [Internet], 2016 [cited 2016 April 11]. Available from http://www.sciencemag.org/authors/science-editorial-policies. |
[3] | N. Beagrie and J. Houghton, The value and impact of data sharing and curation [Internet], 2014 [cited 2016 April 11]. Available from http://repository.jisc.ac.uk/5568/1/iDF308_-_Digital_Infrastructure_Directions_Report%2C_Jan14_v1-04.pdf. |
[4] | P. Carbonell, M.A. Mayer and A. Bravo, Exploring brand-name drug mentions on Twitter for pharmacovigilance, Studies in Health Technology and Informatics 210: ((2015) ), 55–59. |
[5] | L.E. Charles-Smith, T.L. Reynolds, M.A. Cameron, M. Conway, E.H. Lau, J.M. Olsen, J.A. Pavlin, M. Shigematsu, L.C. Streichert, K.J. Suda and C.D. Corley, Using social media for actionable disease surveillance and outbreak management: A systematic literature review, PloS One 10: (10) ((2015) ), e0139701. |
[6] | H.E. Check, Technology: The $1,000 genome, Nature [Internet], 2014 [cited 2016 April 4]. Available from http://www.nature.com/news/technology-the-1-000-genome-1.14901. |
[7] | P. Coorevits, M. Sundgren, G.O. Klein, A. Bahr, B. Claerhout, C. Daniel, M. Dugas, D. Dupont, A. Schmidt, P. Singleton, G. De Moor and D. Kalra, Electronic health records: New opportunities for clinical research, Journal of Internal Medicine 274: (6) ((2013) ), 547–560. doi:10.1111/joim.12119. |
[8] | Data Curation Centre, DCC curation lifecycle model [Internet], 2016 [cited 2016 April 11]. Available from http://www.dcc.ac.uk/resources/curation-lifecycle-model. |
[9] | DataONE, DataONE: Data Observation Network for Earth [Internet], 2016 [cited 2016 April 11]. Available from https://www.dataone.org/. |
[10] | C. Dobre and F. Xhafa, Parallel programming paradigms and frameworks in big data era, International Journal of Parallel Programming 42: (5), 710–738. doi:10.1007/s10766-013-0272-7. |
[11] | Dryad, Dryad Digital Repository [Internet], 2016 [cited 2016 April 11]. Available from http://datadryad.org/. |
[12] | L.M. Federer, Y.-L. Lu and D.J. Joubert, Data literacy training needs of biomedical researchers, Journal of the Medical Library Association 104: (1) ((2016) ), 52–57. doi:10.3163/1536-5050.104.1.008. |
[13] | L.M. Federer, Y.-L. Lu, D.J. Joubert, J. Welsh and B. Brandys, Biomedical data sharing and reuse: Attitudes and practices of clinical and scientific research staff, PloS One 10: (6) ((2015) ), e0129506. doi:10.1371/journal.pone.0129506. |
[14] | figshare, figshare [Internet], 2016 [cited 2016 April 11]. Available from, http://figshare.com. |
[15] | K. Finley, Google just made near-infinite storage cheap and easy, Wired [Internet], 2015 [cited 2016 April 4]. Available from http://www.wired.com/2015/03/google-nearline/. |
[16] | F. Gesualdo, G. Stilo, A. D’Ambrosio, E. Carloni, E. Pandolfi, P. Velardi, A. Fiocchi and A.E. Tozzi, Can Twitter be a source of information on allergy? Correlation of pollen counts with tweets reporting symptoms of allergic rhinoconjunctivitis and names of antihistamine drugs, PloS One 10: (7) ((2015) ), e0133706. doi:10.1371/journal.pone.0133706. |
[17] | J.P. Holdren, Increasing access to the results of federally funded scientific research [Internet], 2013 [cited 2016 April 11]. Available from https://www.whitehouse.gov/sites/default/files/microsites/ostp/ostp_public_access_memo_2013.pdf. |
[18] | IBM Big Data & Analytics Hub, The four V’s of big data [Internet], 2016 [cited 2016 April 4]. Available from http://www.ibmbigdatahub.com/infographic/four-vs-big-data. |
[19] | IBM Software, Bringing big data to the enterprise [Internet], 2016 [cited 2016 April 4]. Available from http://www-01.ibm.com/software/data/bigdata/what-is-big-data.html. |
[20] | Institute of Museum and Library Services, Talking points: Museums, libraries, and makerspaces [Internet], 2014 [cited 2016 April 26]. Available from https://www.imls.gov/assets/1/AssetManager/Makerspaces.pdf. |
[21] | D.L. Longo and J.M. Drazen, Data sharing, New England Journal of Medicine 374: (3) ((2016) ), 276–277. doi:10.1056/NEJMe1516564. |
[22] | C. Maldarelli, We can now sequence a whole human genome in 26 hours, Popular Science [Internet], 2015 [cited 2016 April 4]. Available from http://www.popsci.com/scientists-can-now-sequence-whole-genome-in-26-hours. |
[23] | Z.S. Morris, S. Wooding and J. Grant, The answer is 17 years, what is the question: Understanding time lags in translational research, Journal of the Royal Society of Medicine 104: (12) ((2011) ), 510–520. doi:10.1258/jrsm.2011.110180. |
[24] | National Center for Biotechnology Information, Formatting your submission, 2014 [cited April 26, 2016], in: The GenBank Submissions Handbook [Internet], National Library of Medicine, Bethesda, MD [cited April 26, 2016]. Available from http://www.ncbi.nlm.nih.gov/books/NBK53702/. |
[25] | National Human Genome Research Institute, The Human Genome Project completion: Frequently asked questions [Internet], 2010 [cited 2016 April 4]. Available from https://www.genome.gov/11006943. |
[26] | National Institutes of Health, National Institutes of Health plan for increasing access to scientific publications and digital scientific data from NIH funded scientific, research 2015 [cited 2016 April 11]. Available from https://grants.nih.gov/grants/NIH-Public-Access-Plan.pdf. |
[27] | National Institutes of Health Data and Informatics Working Group, Draft report to the Advisory Committee to the Director [Internet], 2012 [cited 2016 April 11]. Available from http://acd.od.nih.gov/Data%20and%20Informatics%20Working%20Group%20Report.pdf. |
[28] | National Institutes of Health Library, NIH Library: Data services [Internet], 2016 [cited 2016 April 11]. Available from http://nihlibrary.campusguides.com/dataservices/. |
[29] | National Science Foundation Office of Budget, Finance and Award Management, Dissemination and sharing of research results [Internet], 2011 [cited 2016 April 11]. Available from http://www.nsf.gov/bfa/dias/policy/dmp.jsp. |
[30] | Nature Publishing Group, Policies: Availability of data, material and methods [Internet], 2016 [cited 2016 April 11]. Available from http://www.nature.com/authors/policies/availability.html. |
[31] | H.A. Piwowar, Who shares? Who doesn’t? Factors associated with openly archiving raw research data, PloS One 6: (7) ((2011) ), e18657. doi:10.1371/journal.pone.0018657. |
[32] | re3data.org, Registry of Research Data Repositories [Internet], 2016 [cited 2016 April 4]. Available from http://service.re3data.org/search. |
[33] | L. Silva, EveryONE: PLoS One Community Blog [Internet], 2014 [cited 2016]. Available from http://blogs.plos.org/everyone/2014/02/24/plos-new-data-policy-public-access-data-2/. |
[34] | C. Tenopir, S. Allard, K. Douglass, A.U. Aydinoglu, L. Wu, E. Read, M. Manoff and M. Frame, Data sharing by scientists: Practices and perceptions, PloS One 6: (6) ((2011) ), e21101. |
[35] | The Jackson Laboratory, Mouse Genome Informatics (MGI) [Internet], 2016 [cited 2016 April 11]. Available from http://www.informatics.jax.org/. |
[36] | UK Data Service, Research data lifecycle [Internet], 2016 [cited 2016 April 11]. Available from https://www.ukdataservice.ac.uk/manage-data/lifecycle. |
[37] | University of California Digital Library, DMPTool [Internet], 2016 [cited 2016 April 11]. Available from https://dmptool.org/. |
[38] | University of Minnesota Libraries, Data Repository for the U of M (DRUM) [Internet], 2016 [cited 2016 April 11]. Available from https://www.lib.umn.edu/datamanagement/drum. |
[39] | University of Virginia Library Research Data Services, Steps in the data life cycle [Internet], 2016 [cited 2016 April 11]. Available from http://data.library.virginia.edu/data-management/lifecycle/. |
[40] | K.A. Wetterstrand, DNA sequencing costs: Data from the NHGRI Genome Sequencing Program (GSP) [Internet], 2016 [cited 2016 April 4]. Available from https://www.genome.gov/sequencingcosts/. |