NLM’s sponsorship of research in biomedical informatics (1985–2016)
Abstract
The U.S. National Library of Medicine’s (NLM) funding for biomedical informatics research in the 1980s and 1990s focused on clinical decision support systems, which were also the focus of research for Donald A.B. Lindberg M.D. prior to becoming NLM’s director. The portfolio of projects expanded over the years. At NLM, Dr. Lindberg supported various large infrastructure programs that enabled biomedical informatics research, as well as investigator-initiated research projects that increasingly included biotechnology/bioinformatics and health services research. The authors review NLM’s sponsorship of research during Dr. Lindberg’s tenure as its Director. NLM’s funding significantly increased in the 2000’s and beyond. Authors report an analysis of R01 topics from 1985–2016 using data from NIH RePORTER. Dr. Lindberg’s legacy for biomedical informatics research is reflected by the research NLM supported under his leadership. The number of R01s remained steady over the years, but the funds provided within awards increased over time. A significant amount of NLM funds listed in RePORTER went into various types of infrastructure projects that laid a solid foundation for biomedical informatics research over multiple decades.
1.Introduction
Research in biomedical informatics is the cornerstone for advances in the way computers and systems can impact the health of millions of people. In addition to stimulating a sea-change in biomedical informatics research during his tenure at the U.S. National Library of Medicine (NLM), Donald A.B. Lindberg M.D. contributed to a significant increase in biomedical research in general. He enabled world-wide free-of-charge dissemination of scientific peer-reviewed abstracts from the biomedical literature through PubMed [1]. Under his leadership, the NLM also made available numerous other databases and knowledge bases, which serve as foundations for entire fields such as toxicology and molecular biology. His experience as a biomedical researcher helped him understand the importance of foundational informatics research and development [2].
During Dr. Lindberg’s tenure, NLM developed a significant infrastructure that engaged the extramural research community. From his own experience in conducting research at an intersection of disciplines, and by assembling a talented team that could bring projects to the finish line, Dr. Lindberg enabled generations of investigators to explore a wide range of biomedical informatics research topics with funding from NLM.
This chapter briefly reviews Dr. Lindberg’s legacy as a pioneer in pathology informatics research to provide context for the focus on decision support systems in his early years at NLM, and his work on hospital information systems that influenced his role as a critical enabler for other investigators [2]. NLM influenced the fields of biomedical informatics and library science in numerous ways. It implemented services and tools that helped investigators worldwide accelerate their research. This chapter initially highlights aspects of Dr. Lindberg’s own research before he became NLM’s director. To provide context about the environment in which NLM investigator initiated R01s evolved over the years, the chapter also cites major NLM programs that impacted the biomedical research community, such as PubMed, the National Center for Biotechnology Information (NCBI) portfolio of biomedical databases, and ClinicalTrials.gov. Finally, the authors present a novel analysis of NLM’s funding trends for extramural research from 1985–2016.
2.Research in clinical decision support systems
Dr. Lindberg had hands-on experience in computer applications in a hospital setting long before he was selected to lead NLM [2]. A pathologist by training, Dr. Lindberg spent his research years developing new algorithms and tools for the analysis of various types of clinical pathology data. One of his first indexed articles, published in 1963, dealt with the automatic measurement and processing of microbiology data [3]. In 1964, he published an article that went beyond pathology, entitled “Computer Generated Hospital Diagnosis File [4]”. A few years later he discussed computer-assisted collection, evaluation, and transmission of Hospital Laboratory Data [5]. His 1968 book, entitled “The Computer and Medical Care”, targeting an audience of readers who understood hospitals but did not necessarily understand computers, was reviewed in the journal Medical Care [6]. The reviewer pointed out that “Dr. Lindberg details a working data system using punched cards and teletype printers” and that he addressed quality improvement: “The ability of the computer to prevent and detect errors by improving quality control in the hospital laboratory is documented”. The review concludes “this is a most worthwhile book… Dr. Lindberg is recognized as a leader in his field, and he has made a real contribution in making his work more readily available to those wishing to know more about the computer and medical care [6]”.
As mentioned in the book review, Dr. Lindberg’s contributions were not limited to pathology. In 1968, he published one of the first articles on the automated analysis of electrocardiograms EKGs [7]. It required an additional 20 years for automated EKG analysis to become mainstream in medicine: only in 1988 did Medicare approve reimbursement for automated EKG analysis [8]. Coincidentally, this was the same year in which, given the growing importance of molecular biology and databases, the U.S. National Center for Biotechnology Information (NCBI) was founded under Dr. Lindberg’s tenure at NLM [9].
Before Dr. Lindberg was sworn as NLM Director in 1984, he had expanded his research portfolio outside of pathology. He published a paper on a computer-based drug information system in 1974 [10]. His last major contribution before becoming NLM’s director was an expert system named AI/RHEUM, designed to serve as a consultant system for rheumatology [2]. Having spent over 20 years as a pioneer in a field that was relatively unknown at the time probably helped Dr. Lindberg appreciate the importance of extramural funding and the impact the U.S. National Institutes of Health (NIH) could have for biomedical informatics.
3.NLM director’s support for research infrastructure
In 2010, Dr. Lindberg published an overview of NLM’s history [11]. NLM’s direct contribution to extramural research came from the different initiatives outlined below.
3.1.Infrastructure for biomedical research
The impact that NLM had on biomedical research and healthcare is reflected in the growth of the scientific literature itself. By making abstracts from that expanding literature freely available worldwide, NLM increased its international standing and relevance [1]. Early on, when the Internet did not exist and library collections consisted of paper-based books and journals, networked academic medical centers were not common. Integrated Academic Information Management Systems (IAIMS) was a concept created before Dr. Lindberg became NLM director but was realized during his tenure [12,13]. In this initiative, NLM played a central role in information networked systems. The fact that this seems logical and unsurprising today is a testament on how the idea really took flight. The significance is that the whole biomedical community started to become more familiar with computers. MEDLINE, the largest database of biomedical literature in the world, was popularized by the application Grateful Med, a search engine for MEDLINE, which made it easy for clinicians and researchers to browse the scientific literature to find articles that would improve care or accelerate their research [14,15].
Another application launched under Dr. Lindberg’s tenure was ClinicalTrials.gov, which helped with registration of clinical trials and with displaying information about their eligibility criteria, study design, etc. [16]. While several applications relating to publications or studies were launched, additional sources of data in NCBI were growing fast, and the center became a critical source of information for a growing molecular biology research community around the world [17]. The Internet-based PubMed application serves as a portal and search engine and is undoubtedly the most popular application in the history of NLM, the NIH, or any other resource for biomedical researchers [18]. Clinicians and researchers can support their decision making based on articles they find on PubMed. All major health sciences breakthroughs are documented in the pages of journals indexed for PubMed. Still under Dr. Lindberg’s directorship, NLM launched PubMed Central, where full articles are deposited and made freely available to anyone [19].
In addition to infrastructure that benefitted biomedical researchers worldwide, the NLM supported infrastructure that specifically enabled the acceleration of biomedical informatics research.
3.2.Infrastructure for biomedical informatics research
NLM utilized both intramural and extramural researchers to build a framework that would impact research nationwide [20]. The NLM’s Unified Medical Language System (UMLS) project is documented elsewhere in this book [21]. The project’s goal was to enable interoperability among systems by putting into place shared vocabularies and standards. Another important Lindberg infrastructure initiative was NLM’s involvement in the interagency High Performance Computing (HPC) and Communications Program (HPCC) [21,22]. The development of an “information superhighway” for biomedical data was also among NLM’s flagship initiatives, where again the extramural scientific community was engaged in developing new applications that would move data faster into facilities supported by the NLM where data could be processed and analyzed. This included research in telemedicine, electronic health record systems, and virtual reality in many institutions across the country. Taken together, these research infrastructure efforts were prescient of the acclaimed, contemporary FAIR principle: make data and tools findable, accessible, interoperable and reusable [23].
4.NLM’s direct funding for biomedical informatics research
In a NLM Board of Regents meeting in 2014, Valerie Florance Ph.D., Director of Extramural Programs, reviewed NLM’s research funding from 1984–2014 [24]. Florance suggested “By 1985, 50 percent of the research grants NLM awarded had medical decision support as a focus”. Florance also noted NLM’s long-range plans expanded the scope of its grant programs and recent plans emphasized biotechnology as well as the need for research on fundamental issues and methods in medical informatics. She added that “By 1998, 44 percent of grants were for biotechnology research”.
NLM’s 2000 Long Range Plan further “expanded research to include consumer health information, patient-specific data, and access to knowledge-based information”. Grants became larger during the first decade of the 21st century, Florance noted. She explained success rates have gone “up and down”, and NLM, which was considered the only supporter of informatics at NIH had company. NIH programs such as Big Data to Knowledge (BD2K) and other NIH institutes sponsored biomedical informatics research.
To provide insightful details regarding funded NLM extramural R01 projects, the authors analyzed data that NIH makes publicly available. The analysis collected the NLM-funded projects accessible through the NIH RePORTER API V2.0 released in 2021 with valid new project cost and keyword indexing terms information from Fiscal Year (FY) 1985 (the earliest year available in NIH RePORTER) to FY 2016, as demonstrated in Fig. 1A (all years mentioned in the rest of this section are FYs unless otherwise stated) [25,26]. In general, the number of funded NLM R01 projects remained around 13, while the overall number of NLM projects increased. On the other hand, the average project cost for an R01 increased steadily, while the overall average cost increased more sharply after 2007 (Fig. 1B).
Fig. 1.
Statistics of NLM projects from 1985 to 2016. (A) Number of projects. (B) Average project amounts. The NLM All Funded Projects included both extramural and intramural projects.
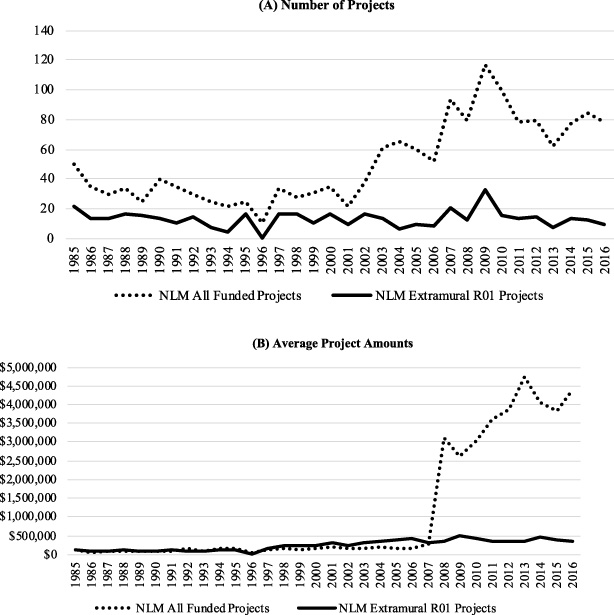
The number of project keyword indexing terms, as shown in Fig. 2, increased significantly after 2007 because of a system change from the Computer Retrieval of Information on Scientific Projects (CRISP) system to the Research, Condition, and Disease Categorization (RCDC) process, as described below [27–29]:
“Beginning with projects funded in FY 2008, project terms are concepts derived by mining the text of a project’s title, abstract, specific aims, and investigator’s stated public health relevance. For projects funded in fiscal years prior to 2008, the project terms in RePORTER are the same terms used in the NIH CRISP system that RePORTER replaces. See the Research, Condition, and Disease Categorization Process for a complete description of this text mining process.”
The authors split the years into six periods for further project indexing term analysis (the areas split by the vertical lines in Fig. 2). Authors excluded 1996 (in which no terms were provided for any project in RePORTER) and included 1985 in the first period (because the total number of years, after excluding 1996, is not divisible by five). The project indexing terms before and after the system change are not comparable [29]:
“Term searches that span fiscal years before and after 2008 will not be comparable. There is no simple and direct association between the CRISP terms used prior to 2008 and the project concepts derived through text mining in 2008 and later years”.
Therefore, all of the subsequent analysis results of the last two periods (i.e., “2007–2011” and “2012–2016”) are also not directly comparable with the results from earlier periods.
Fig. 2.
Average project terms from 1985 to 2016, split into six five-year periods (excluding 1996, and the first period contains six years). The last two periods (“2007–2011” and “2012–2016”, marked with an asterisk symbol “∗”) contain project terms collected using a new system, thus having a significantly higher number of average project terms [29].
![Average project terms from 1985 to 2016, split into six five-year periods (excluding 1996, and the first period contains six years). The last two periods (“2007–2011” and “2012–2016”, marked with an asterisk symbol “∗”) contain project terms collected using a new system, thus having a significantly higher number of average project terms [29].](https://content.iospress.com:443/media/isu/2022/42-1/isu-42-1-isu210137/isu-42-isu210137-g002.jpg)
For each period shown in Fig. 2, the analysis involved Principal Component Analysis (PCA) with 95% variance coverage and identified the top three Principal Components (PCs) [30,31]. Then, the analysis progressed to include Expectation Maximization (EM) clustering based on K-Means algorithm [32,33]. The details of the approach are as follows: the authors initially set the K (i.e., number of clusters) to one, split the data into ten folds, and then performed EM for ten times to compute the average log-likelihood of the clustering results in these ten folds [34]. Then, the analysis increased K by one and repeated the above process, to see if the log-likelihood increased. This increment of K continued until the log-likelihood decreased. The largest K before the decreasing log-likelihood was used as the final number of clusters. Using the method above, authors clustered the projects based on the three PCs. In all periods the algorithm generated K = 2 clusters, while in the “2007–2011” period there are K = 5 clusters being created. In the 3-Dimensional PC space, the projects in the first four periods are sparser while the ones in the last two periods are denser, reflecting the difference after the system change. Authors used the Java-based Weka library to conduct the analysis [35].
The analysis further extracted the top ten project indexing terms in each cluster of projects for each period based on their frequency (Table 1 and Table 2). Again, the set of project terms in the first four periods are different from the ones in the last two periods because of the system change. To compare the results across all periods, the authors manually reviewed and evaluated the project terms. Artificial Intelligence (AI- vertically-shaded in Table 1 and Table 2) was mentioned frequently between 1985–1995, and then less mentioned between 1997–2011, and finally fell out of the top ten. Meanwhile, genomics (horizontally shaded in Table 1 and 2) were frequently mentioned from 1985 to 1990, and then became prevalent again from 2007 to 2011. Also, “data”, “base”, “databases”, and “data bases” were all valid project terms in the new system (i.e., in the last two periods), nevertheless “data” and “base” appear more frequently than the other two.
Table 1
Top one to five project indexing terms in each cluster, as they appear in NIH RePORTER
![]() |
[i] Each row represents a cluster of projects in the corresponding period, and the project terms were ranked based on their frequency. The vertically shaded ones are related to Artificial Intelligence (AI), and the horizontally-shaded ones are related to genomics. The results of the last two periods (“*”) have different set of project terms because of the system change.
Table 2
Top six to ten project indexing terms in each cluster
![]() |
[i] The notations are the same as that of Table 1.
A strong emphasis on computer-assisted systems for clinical decision making was clear until 2006, and genome-wide studies constituted a well-defined cluster in 2007–2011.
However, this analysis has limitations because it does not always confirm the findings described in the 2014 NLM Board of Regents minutes [24]. For example, biotechnology terms did not appear frequently until a decade ago, and then only genome-wide terms appeared in one cluster. Disaster preparedness does not feature as a frequent term and health services research does not appear at all after 2007. Spurious terms such as “British Isles” appear in the list of frequent terms, even before an automated term capture system was put in place. This may just be an artifact of selecting only the top ten terms, or of the inclusion of wrong data in RePORTER. As indicated previously, the methods by which terms were assigned for each grant changed in 2007, so comparisons between pre and post-2007 trends are not warranted. The automated project terms assignment also seems not to be discriminating since the most frequent terms include general descriptions such as “data” or “computers.”
Nevertheless, the analysis confirms that R01s on certain topics were funded steadily during the decades in which Dr. Lindberg directed NLM, and that from the 2000s onward the amount of research funding increased significantly.
This chapter provides overviews of three decades of NLM funding for research and research infrastructure that involved the extramural community. The impact is palpable not only in terms of continued resources that expanded the depth and scope of the biomedical informatics community. The NLM programs expanded and supported the number of faculty members and trainees in biomedical informatics nationally. The NLM will continue to have a profound impact on the field of biomedical informatics, and Dr. Lindberg’s legacy will live on.
Acknowledgements
T.-T. Kuo is partly funded by the National Human Genome Research Institute (NHGRI) of the U.S. NIH under Award Number R00HG009680, the U.S. NIH (R01GM118609, R01HL136835, R01HG011066), and UCSD Academic Senate Research Grant RG100836. L. Ohno-Machado is funded by the U.S. NIH (R01GM118609, R01HL136835, R01HG011066). The content is solely the responsibility of the authors and does not necessarily represent the official views of the NIH.
References
[1] | K.A. Smith, Free MEDLINE access worldwide. in: Transforming Biomedical Informatics and Health Information Access: Don Lindberg and the National Library of Medicine, B.L. Humphreys, R.A. Logan, R.A. Miller and E.R. Siegel (eds), IOS Press, Amsterdam, (2021) . |
[2] | L.C. Kingsland III and C.A. Kulikowski, A scientific mind embraces medicine: Donald Lindberg’s education and early career. in: Transforming Biomedical Informatics and Health Information Access: Don Lindberg and the National Library of Medicine, B.L. Humphreys, R.A. Logan, R.A. Miller and E.R. Siegel (eds), IOS Press, Amsterdam, (2021) . |
[3] | D.A. Lindberg and G.R. Reese, Automatic measurement and computer processing of bacterial growth data, Biomedical Sciences Instrumentation 1: : ((1963) ), 11–20. |
[4] | D.A. Lindberg, G.R. Reese and C. Buck, Computer generated hospital diagnosis file, Missouri Medicine 61: ((1964) ), 581. |
[5] | D.A. Lindberg, Collection, evaluation, and transmission of hospital laboratory data, Methods of Information in Medicine 6: (03) 97–107. doi:10.1055/s-0038-1636364. |
[6] | S. Brunjes, Medical Care 7: (5) ((1969) ), 414–416. |
[7] | D.A. Lindberg and P.R. Amlinger, Automated analysis of the electrocardiogram, Missouri Medicine 65: (9) ((1968) ), 742–745. |
[8] | H. Smulyan, The computerized ECG: Friend and foe, The American Journal of Medicine 132: (2) ((2019) ), 153–160. doi:10.1016/j.amjmed.2018.08.025. |
[9] | D.R. Masys and D.A. Benson, Don Lindberg and the creation of the National Center for Biotechnology Information. in: Transforming Biomedical Informatics and Health Information Access: Don Lindberg and the National Library of Medicine, B.L. Humphreys, R.A. Logan, R.A. Miller and E.R. Siegel (eds), IOS Press, Amsterdam, (2021) . |
[10] | S. Garten, C.E. Mengel, W.E. Stewart and D.A. Lindberg, A computer-based drug information system, Missouri Medicine 71: (4) ((1974) ), 183–186. |
[11] | D.A. Lindberg, The national library of medicine, World Neurosurg 74: (1) ((2010) ), 46–48. doi:10.1016/j.wneu.2010.04.014. |
[12] | D.A. Lindberg, R.T. West and M. Corn, IAIMS: An overview from the National Library of Medicine, Bulletin of the Medical Library Association 80: (3) ((1992) ), 244. |
[13] | N.M. Lorenzi and W.W. Stead, NLM and the IAIMS initiative: Cross-institutional academic/advanced systems contributing to the evolution of networked information and resources. in: Transforming Biomedical Informatics and Health Information Access: Don Lindberg and the National Library of Medicine Ion, B.L. Humphreys, R.A. Logan, R.A. Miller and E.R. Siegel (eds), IOS Press, Amsterdam, (2021) . |
[14] | D.A. Lindberg, The NLM and Grateful Med: promise, public health, and policy, Public Health Reports 111: (6) ((1996) ), 552. |
[15] | J.L. Dorsch, J.G. Faughnan and B.L. Humphreys, Grateful Med: Direct access to MEDLINE for health professionals with personal computers. in: Transforming Biomedical Informatics and Health Information Access: Don Lindberg and the National Library of Medicine, B.L. Humphreys, R.A. Logan, R.A. Miller and E.R. Siegel (eds), IOS Press, Amsterdam, (2021) . |
[16] | A.T. McCray and N.C. Ide, Design and implementation of a national clinical trials registry, Journal of the American Medical Informatics Association 7: (3) ((2000) ), 313–323. doi:10.1136/jamia.2000.0070313. |
[17] | K. Smith, , A brief history of NCBI’s formation and growth. The NCBI handbook [Internet]. 2013. August 23, 2021. Available from: https://www.ncbi.nlm.nih.gov/books/NBK148949/. |
[18] | National Library of Medicine. PubMed celebrates its 10th anniversary! 2006. August 23, 2021. Available from: https://www.nlm.nih.gov/pubs/techbull/so06/so06_pm_10.html. |
[19] | National Library of Medicine. MEDLINE, PubMed, and PMC (PubMed Central): How are they different? 2020. August 23, 2021. Available from: https://www.nlm.nih.gov/bsd/difference.html. |
[20] | B.L. Humphreys and M.S. Tuttle, Transforming Biomedical Informatics and Health Information Access: Don Lindberg and the National Library of Medicine.B.L. Humphreys, R.A. Logan, R.A. Miller and E.R. Siegel (eds), IOS Press, Amsterdam, (2021) . |
[21] | M.J. Ackerman, S.E. Howe and D.R. Masys, Don Lindberg, high performance computing and communications, and telemedicine. in: Transforming Biomedical Informatics and Health Information Access: Don Lindberg and the National Library of Medicine, B.L. Humphreys, R.A. Logan, R.A. Miller and E.R. Siegel (eds), IOS Press, Amsterdam, (2021) . |
[22] | D.A. Lindberg and B.L. Humphreys, High-performance computing and communications and the national information infrastructure: New opportunities and challenges, Journal of the American Medical Informatics Association 2: (3) ((1995) ), 197. doi:10.1136/jamia.1995.95338873. |
[23] | M.D. Wilkinson, M. Dumontier, I.J. Aalbersberg, G. Appleton, M. Axton, A. Baak , The FAIR guiding principles for scientific data management and stewardship, Scientific Data ((2016) ), 3. doi:10.1038/sdata.2016.18. |
[24] | National Library of Medicine. Minutes of the Board of Regents, May 13, 2014. 2014. August 24, 2021. Available from: https://www.nlm.nih.gov/od/bor/514BORminutes.pdf. |
[25] | NIHRePORT. NIH RePORTER API V2.0. August 17, 2021. Available from: https://api.reporter.nih.gov/?urls.primaryName=V2.0. |
[26] | NIHRePORT. NIH RePORTER. August 12, 2021. Available from: https://reporter.nih.gov. |
[27] | A.H. Bair, L.P. Brown, L.C. Pugh, L.C. Borucki and D.L. Spatz, Taking a bite out of CRISP. Strategies on using and conducting searches in the Computer Retrieval of Information on Scientific Projects database, Comput. Nurs. 14: (4) ((1996) ), 218–224, quiz 25-6. |
[28] | NIHRePORT. RCDC Process. August 17, 2021. Available from: https://report.nih.gov/funding/categorical-spending/rcdc-process. |
[29] | NIHRePORT. NIH RePORT frequently asked questions (FAQs). August 12, 2021. Available from: https://report.nih.gov/faqs. |
[30] | S. Wold, K. Esbensen and P. Geladi, Principal component analysis, Chemometrics and Intelligent Laboratory Systems 2: (1-3) ((1987) ), 37–52. doi:10.1016/0169-7439(87)80084-9. |
[31] | M. Hall and G. Schmidberger, WEKA principal components. August 17, 2021. Available from: https://weka.sourceforge.io/doc.dev/weka/attributeSelection/PrincipalComponents.html. |
[32] | P. Cheeseman and J. Stutz, Bayesian classification (AutoClass): Theory and results, Advances in KDD ((1996) ). |
[33] | J.A. Hartigan and M.A. Wong, Algorithm AS 136: A k-means clustering algorithm, Journal of the Royal Statistical Society Series C (Applied Statistics) 28: (1) ((1979) ), 100–108. doi:10.2307/2346830. |
[34] | M. Hall, E. Frank and E.M. Weka, August 17, 2021. Available from: https://weka.sourceforge.io/doc.dev/weka/clusterers/EM/.html. |
[35] | M. Hall, E. Frank, G. Holmes, B. Pfahringer, P. Reutemann and I.H. Witten, The WEKA data mining software: An update, ACM SIGKDD Explorations Newsletter 11: (1) ((2009) ), 10–18. doi:10.1145/1656274.1656278. |