Responsible application of artificial intelligence to surveillance: What prospects?1
Abstract
Artificial Intelligence (AI) is one of the most significant of the information and communications technologies being applied to surveillance. AI’s proponents argue that its promise is great, and that successes have been achieved, whereas its detractors draw attention to the many threats embodied in it, some of which are much more problematic than those arising from earlier data analytical tools.
This article considers the full gamut of regulatory mechanisms. The scope extends from natural and infrastructural regulatory mechanisms, via self-regulation, including the recently-popular field of ‘ethical principles’, to co-regulatory and formal approaches. An evaluation is provided of the adequacy or otherwise of the world’s first proposal for formal regulation of AI practices and systems, by the European Commission. To lay the groundwork for the analysis, an overview is provided of the nature of AI.
The conclusion reached is that, despite the threats inherent in the deployment of AI, the current safeguards are seriously inadequate, and the prospects for near-future improvement are far from good. To avoid undue harm from AI applications to surveillance, it is necessary to rapidly enhance existing, already-inadequate safeguards and establish additional protections.
1.Introduction
The scope for harm to arise from Artificial Intelligence (AI) has been recognised by technology providers, user organisations, policy-makers and the public alike. On the other hand, effective management of the risks inherent in its application has been much less apparent. Many users of Information & Communications Technologies (ICT) for surveillance purposes have been successful in avoiding meaningful regulation of their activities. This article undertakes an assessment of the prospects of AI’s use for surveillance being brought under control.
The subjects of surveillance that are considered in this article are primarily people, both individually and collectively, but also things and spaces around which and within which people move. Other contexts, such as in public health and seismology, are not considered. A substantial body of knowledge exists about surveillance (e.g. Rule, 1974; Gandy, 1993; Lyon, 2001; Marx, 2016). The original sense of the word, adopted from French, was of ‘watching over’. It was once inherently physical, spatial and visual (Bentham, 1791, Foucault 1975/1977) That limitation has been long since overcome, with enormous developments over the last century in the surveillance of sound, communications, data, and personal experience. It has become routine to locate and track people in physical space, and their digital personae in virtual space (Clarke, 2014b).
Infrastructure to support surveillance has become pervasive, around the environments in which people live, work and play, and even with people, on people, and in people. Surveillance can be conducted retrospectively, or contemporaneously, or even in an anticipatory manner, threateningly referred to as ‘predictive’. It has given rise to circumspect constructs such as ‘surveillance society’ (Marx, 1985; Gandy, 1989; Lyon, 2001), ‘the panoptic sort’ (Gandy, 1993, 2021), ‘ubiquitous transparency’ (Brin, 1998), ‘location and tracking’ (Clarke, 2001; Clarke & Wigan, 2011), ‘sousveillance’ (from below rather than above, by the weak of the powerful – Mann et al., 2003), ‘equiveillance’ (Mann, 2005), ‘uberveillance’ (both comprehensive and from within – Michael & Michael, 2007; Clarke, 2010), ‘surveillance capitalism’ (Zuboff, 2015) and the ‘digital surveillance economy’ (Clarke, 2019a). An 1800-word review of surveillance, intended to provide the reader with background to the analysis in this article, is in Clarke (2022).
An overview of AI is provided, firstly in the abstract, then moving on to sub-fields of AI with apparent relevance to surveillance. An appreciation of the characteristics of the technologies enables the identification of disbenefits and risks involved in AI’s application to surveillance. A review is then undertaken of the ways in which control might be exercised. Particular attention is paid to the wave of publishing activity during the period 2015–21 in the area of ‘Principles for Responsible AI’. The analysis draws on a previously-published, consolidated super-set of Principles.
Almost all of the publications to date are ‘Guidelines’. This provides a justification for individuals and organisations inclined towards greater care in the deployment of technology, but it lacks enforceability, and in most cases has little impact on AI practice. A critique is provided of the proposal of 21 April 2021 of the European Commission, which appears to be a world-first initiative to establish formal regulation of a bespoke nature for AI applications. The analysis suggests that the provisions appear to be so weak, and the exemptions so broad, that enactment of the proposal, while it would provide window-dressing for AI-using organisations, would not deliver any significant protections for the public. The article concludes with an assessment of the prospects of effective control being achieved over AI applications to surveillance even by organisations with limited market and institutional power, let alone by large corporations and government agencies.
2.AI in support of surveillance
This section provides an overview of the origins and the ambiguous and contested nature of AI. The fields that appear to have particular relevance to surveillance are then outlined. That provides a basis for identifying the disbenefits and risks that AI applications to surveillance appear to embody.
2.1AI in the abstract
The term Artificial Intelligence was coined in the mid-20th century, based on “the conjecture that every aspect of learning or any other feature of intelligence can in principle be so precisely described that a machine can be made to simulate it” (McCarthy et al., 1955). The word ‘artificial’ implies ‘artefactual’ or ‘human-made’. Its conjunction with ‘intelligence’ leaves open the question as to whether the yardstick is ‘equivalent to human’, ‘different from human’ or ‘superior to human’. Conventionally (Albus, 1991; Russell & Norvig, 2003; McCarthy, 2007):
Artificial Intelligenceis exhibited by an artefact if it:
(1) evidences perception and cognition of relevant aspects of its environment; (2) has goals; and (3) formulates actions towards the achievement of those goals.
Histories of AI (e.g. Russell & Norvig, 2009, pp. 16–28; Boden, 2016, pp. 1–20) identify multiple strands and successive re-visits to much the same territory. Over-enthusiastic promotion has always been a feature of the AI arena. The revered Herbert Simon averred that “Within the very near future – much less than twenty-five years – we shall have the technical capability of substituting machines for any and all human functions in organisations.
The last decade has seen an(other) outbreak. Spurred on by the hype, and by the research funding that proponents’ promises have extracted, AI has excited activity in a variety of fields. Some of potential significance are natural language understanding, image processing and manipulation, artificial life, evolutionary computation aka genetic algorithms, and artificial emotional intelligence.
AI intersects with robotics, to the extent that the software installed in a robot is justifiably regarded as artificially intelligent. Robotics involves two key elements:
• programmability, implying computational or symbol-manipulative capabilities that a designer can combine as desired (a robot is a computer); and
• mechanical capability in the form of actuators, enabling it to act on its environment rather than merely function as a data processing or computational device (a robot is a machine).
Two further, frequently-mentioned elements of robotics are sensors, to enable the gathering of data about the devices’s environment; and flexibility, in that the device can both operate using a range of programs, and manipulate and transport materials in a variety of ways. Where robotics incorporates AI elements, additional benefits may be achieved, but the disbenefits and risks are considerably greater, because of the inherent capacity of a robot to act autonomously in the real world (Clarke, 2014a), and the temptation and tendency for the power of decision and action to be delegated to the artefact, whether intentionally or merely by accident.
2.2Claims regarding AI applications to surveillance
Considerable scepticism is necessary when evaluating the claims of AI’s successes. This applies in all domains, but none moreso than surveillance. Civil society analyses of AI in surveillance depend very heavily on media reports that repeat the content of corporate and government media releases and that are highly superficial in their depiction of the underlying technologies. Typical of this vacuity is the assertion, unsupported by any evidence, that many workplace surveillance tools are “powered by artificial intelligence” (Cater & Heikkilä, 2021).
In a widely-read study, Feldstein (2019) identified relevant ‘AI surveillance techniques’ as being smart city/safe city platforms, facial recognition systems and smart policing (p. 16). The author was, however, unable to provide much detail about actual techniques used, beyond the ubiquitous example of facial recognition (e.g., Heikkilä, 2021). The limited technical information that is publicly available reflects the strong tendency of the operators of surveillance to obscure the nature of their activities, and in some cases even the fact of their conduct. The reasons underlying the obfuscation appear to include institutional cultures of secrecy, intellectual property considerations, weaknesses in the technologies such that transparency would enable effective countermeasures, and/or the technology’s ineffectiveness for the claimed purpose.
One of the few concrete examples that Feldstein was able to provide was “iBorderCtrl
A further feature of AI applications to surveillance is the vagueness with which the term ‘AI’ is used. From some descriptions, it could be inferred that the longstanding techniques of pattern recognition are being applied, e.g. to the extraction of useful data from images of vehicle registration-plates and faces. In other cases, the intention of product promoters may be to claim that machine learning is being employed, e.g. to perform trawls of data collections, and of mergers of disparate data collections, with the intention of detecting anomalies that create suspicion about people, objects or places. On the other hand, most forms of surveillance were developed independently of AI. They may be being enhanced using AI features; but they may merely be having gloss added through the unjustified appropriation of the AI tag to refer to an ‘advanced’ or merely ‘the latest’ version of a product.
A case in point is another of Feldstein’s few named instances: “The idea behind smart policing is to feed immense quantities of data into an algorithm – geographic location, historic arrest levels, types of committed crimes, biometric data, social media feeds – in order to prevent crime, respond to criminal acts, or even to make predictions about future criminal activity” (p. 20). The example provided was the PredPol predictive analytics program; but the description on the company’s websites (PredPol, 2021) makes clear that it is not an AI product.
2.3Fields of AI potentially relevant to surveillance
Given that supplier obfuscation severely hampers the independent evaluation of existing products, an analysis is reported here that is based on the generic characteristics of AI technologies. This approach identifies several fields of AI that have apparent potential for application to surveillance.
Many AI fields involve ‘pattern recognition’, for which four major components are needed: “data acquisition and collection, feature extraction and representation, similarity detection and pattern classifier design, and performance evaluation” (Rosenfeld & Wechsler, 2000, p. 101).
Pattern recognition can be applied in a variety of contexts. Those relevant to surveillance include:
• spatial patterns and image analysis (e.g. Gose et al., 1996), relevant to the monitoring of spaces and objects;
• human biometrics images, which has surveillance applications to fingerprints (e.g., Cole, 2004), iris-scans (Daugman, 1998) and facial recognition (Ryan et al., 2009);
• speech (e.g., O’Shaughnessy, 2008), relevant to audio-surveillance;
• written language (Indurkhya & Damerau, 2010), relevant to the monitoring of communications recorded, made available, and read in textual form;
• data, in the forms of data mining or data analytics (e.g. Pal & Mitra, 2004), relevant to dataveillance.
The last of these requires closer attention. Common features of the classical approaches to pattern-recognition in data have been that:
(1) data is posited to be a sufficiently close representation of some real world phenomenon;
(2) that data is processed by software;
(3) inferences are drawn from that data processing;
(4) the inferences are claimed to have relevance to the understanding or management of the phenomenon.
The software that is used may be developed in a number of different ways. An algorithm is a procedure, or set of steps. The steps may be serial and invariant. Alternatively, and more commonly, the steps may also include repetition constructs (e.g. ‘perform the following steps until a condition is fulfilled’) and selection constructs (e.‘perform one of the following sets of steps depending on some test’). From about 1965 until some time after 2000, the preparation of computing software was dominated by languages designed to enable the convenient expression of algorithms, sometimes referred to as procedural or imperative languages. Software developed in this manner represents a humanly-understandable solution to a problem, and hence the rationale underlying an inference drawn using it can be readily expressed. Techniques that express algorithms do not qualify as AI, unless the resulting artefact fulfils the conventional criteria identified in the previous section: evidence of perception, cognition and goal-seeking behaviour.
Other approaches to developing software exist (Clarke, 1991). Two that are represented as being AI techniques are highly relevant to the issues addressed in the present analysis. The approach adopted in rule-based ‘expert systems’ is to express a set of rules that apply within a problem-domain. A classic rule-example is:
If <Person> was born within the UK or any of <list of UK Colonies> between <date
When software is developed at this level of abstraction, a model of the problem-domain exists; but there is no explicit statement of a particular problem or a solution to it. In a simple case, the reasoning underlying an inference that is drawn in a particular circumstance may be easy to provide, whether to an executive, an aggrieved person upset about a decision made based on that inference, or a judge. However, this may not be feasible where data is missing, the rulebase is large or complex, the rulebase involves intertwining among rules, the rulebase embodies some indeterminacies and/or decision-maker discretions exist. Inferences drawn from rule-based schemes in surveillance contexts, e.g. for suspicion-generation about individuals who may be involved in bomb-making, and in so-called ‘predictive policing’, are subject to these challenges.
A further important software development approach is (generically) machine learning (sometimes referred to as AI/ML), and (specifically) connectionist networks or artificial neural networks (ANNs). ANNs originated in the 1940s in the cognitive sciences, prior to the conception of AI (Medler, 1998). They have subsequently been co-opted by AI researchers and are treated as an AI technique. The essence of neural network approaches is that tools, which were possibly developed using a procedural or imperative language, are used to process examples taken from some problem-domain. Such examples might comprise the data relevant to 5% of all applicants for UK passports during some time-period who were born in, say, Jamaica, including the results of the applications.
The processing results in a set of weights on such factors as the tool treats as being involved in drawing the inference. Although the tool may have been developed using a procedural or imperative language implementing an algorithm, the resulting software that is used to process future cases is not algorithmic in nature. The industry misleadingly refers to it as being algorithmic, and critics have adopted that in terms such as ‘algorithmic bias’; but the processing involved is empirically-based, not algorithmic, and hence more appropriate terms are empirical bias and sample bias.
A critical feature of ANNs is a-rationality, that is to say that there is no reasoning underlying the inference that is drawn, and no means of generating an explanation any more meaningful than ‘based on the data, and the current weightings in the software, you don’t qualify’. The approach is referred to as ‘machine learning’ partly because the means whereby the weightings are generated depend on the collection of prior cases that are fed to the tool as its ‘learning set’. Hence, in the (to many people, somewhat strange) sense of the term used in this field, the software ‘learns’ the set of weightings. In addition, the system may be arranged so as to further adapt its weightings (i.e. ‘keep learning’), based on subsequent cases.
There are two different patterns whereby the factors and weightings can come about (DeLua, 2021). The description above was of supervised learning, in that the factors were fed to the tool by a supervisor (‘labelled’ or ‘tagged’), and in each case the ‘right answer’ was provided within the data. In the case of unsupervised learning, on the other hand, there are no labels, and ‘right answers’ are not provided with the rest of the data. The tool uses clusterings and associations to create the equivalent of what a human thinker would call constructs, but without any contextual information, ‘experience’ or ‘common sense’ about the real world that the data purports to relate to. On the one hand, ‘unsupervised learning’ is touted as being capable of discovering patterns and relationships that were not previously known; but, on the other, this greatly exacerbates the enormous scope that already exists with ‘supervised learning’ for inferences to be drawn that bear little or no relationship to the real world.
The vagaries of ‘tagging’ and even moreso of automated construct creation, coupled with the a-rationality of all AI/ML and its inherently mysterious and inexplicable inferencing, leads people who are not AI enthusiasts to be perturbed and even revulsed by the use of ANNs to make decisions that materially affect people. The issues are all the more serious when ML-based inferencing is conducted in surveillance contexts, because of the severity of the consequences for the unjustly-accused, the absence of a rationale for the inference, the strong tendencies in the system towards reversal of the onus of proof, and the near-impossibility in such circumstances of prosecuting one’s innocence.
2.4Disbenefits and risks of AI
A great many claims have been made about the potential benefits AI might offer. Many of these feature vague explanations of the process whereby the benefits would arise. A proportion of the claims have some empirical evidence to support them, but many are mere assertions in media releases, without the support of independent testing. The analysis reported here is concerned with the downsides: disbenefits, by which is meant impacts that are predictable and harmful to some party, and risks, that is to say harmful impacts that are contingent on particular conditions arising in particular circumstances.
Pattern-matching of all kinds is inherently probabilistic rather than precise. It results in inferences that include false-positives (wrongly asserting that a match exists) and false-negatives (wrongly asserting the absence of a match). When used carefully, with inbuilt and effective safeguards against misinterpretation, benefits may arise and disbenefits and risks may be manageable. Where safeguards are missing or inadequate, the likelihood that disbenefits and risks will arise, and even dominate, increases rapidly. For example, where facial recognition is used for identity authentication, low-quality pattern-matching may cause only limited harm when a device refuses its owner permission to use it, but some alternative authentication mechanism such as a password is readily available. On the other hand, there are many other circumstances in which no alternative is available, the scope for error is high, and serious harm can arise. This is common with uses for identification of individuals presumed to be within populations for which biometric measures have already been recorded, such as at border-crossings.
As regards AI generally, the disbenefits and risks have been presented in many different ways (e.g., Scherer, 2016, esp. pp. 362–373; Yampolskiy & Spellchecker, 2016; Duursma, 2018; Crawford, 2021). Clarke (2019b) identifies five factors underlying concerns about AI:
• Artefact Autonomy, arising from software making decisions on the basis of automated inferences, and even taking action by means of actuators under the artefact’s direct control. This can be applied as an enhancement to CCTV, for example, where directional control is programmed, e.g. to follow and zoom in on rapid movement within the device’s field of view. Many kinds of action are possible that are more directly harmful to individuals’ interests.
• Unjustified Assumptions about Data, including its quality and its correspondence with the real-world phenomena it is assumed to represent. The risk of inappropriate outcomes is compounded when the data is drawn from different sources that had different original purposes, different attitudes to quality, and different attributed meanings for data-items.
• Unjustified Assumptions about the Inferencing Process, due to the unsuitability of data as input to the particular inferencing process, failure to demonstrate both theoretically and empirically the applicability of the process to the particular problem-category or problem-domain, and/or assertions that empirical correlation unguided by theory is enough, and that rational explanation is an unnecessary luxury (e.g., Anderson, 2008; LaValle et al., 2011; Mayer-Schoenberger & Cukier, 2013).
• Opaqueness of the Inferencing Process. In many circumstances, as with AI/ML, this may be empirically-based a-rationality.
• Irresponsibility, in that none of the organisations in the AI supply-chain are subject to effective legal constraints and obligations commensurate with the roles that they play. In the currently-typical context of a long chain of technology providers and a network of inter-operating service providers, there is ample opportunity for plausible deniability, finger-pointing and hence universal liability avoidance.
The fourth of these, the lack of access to reasoning underlying inferences, has particularly serious implications (Clarke, 2019b, pp. 428–429). Where no rationale for the outcome exists and none can be convincingly constructed, no humanly-understandable explanation can be provided. The process may also be impossible to replicate, because parameters affecting it may have since changed and the prior state may not be able to be reconstructed. This means that the process may not be able to be checked by an independent party such as an auditor, judge or coroner, because records of the initial state, intermediate states and triggers for transitions between states, may not exist and may not be able to be re-constructed, such that the auditability criterion is failed.
Where an outcome appears to be in error, the factors that gave rise to it may not be discoverable, and undesired actions may not be correctable. These factors combine to provide entities that have nominal responsibility for a decision or action with an escape clause, in a manner similar to force majeure: AI’s opaqueness may be claimed to be a force that is beyond the capacity of a human entity or organisation to cope with, thereby absolving it of responsibility. In short, every test of due process and procedural fairness may be incapable of being satisfied, and accountability destroyed. Surveillance, with its inherent tendency to generate suspicion and to justify the exercise of power, is a particularly dangerous application for AI tools that, by their nature, absolve the operator from accountability.
In summary, “AI gives rise to errors of inference, of decision and of action, which arise from the more or less independent operation of artefacts, for which no rational explanations are available, and which may be incapable of investigation, correction and reparation” (Clarke, 2019b, p. 426).
The second of the five factors relates to problematic aspects of the data. In respect of AI-based data analytics, the quality of outcomes is dependent on many features of data that need to reach a threshold of quality before they can be reliably used to draw inferences (Wang & Strong, 1996; Shanks & Darke, 1998; Piprani & Ernst, 2008; summarised in Clarke 2016 into 13 factors).
As regards the third of the five factors, process quality, all data analytics techniques embody assumptions about the form that the data takes (such as the scale against which it is measured), and its quality, and the reliability of the assumptions made about the associations between the data and some part of the real world. Text-books on data analytics teach almost nothing about the need for, and the techniques that need to be applied to deliver, assurance of inferencing quality. This gives rise to challenges in relation to the use of the inferences drawn by data-analytical processes from data-sets. For inferences to be reliable, and decisions and actions taken based on those inferences equitable, there is a need for:
• reality testing, to gain insight into the reliability of the data as a representation of relevant real-world entities and their attributes;
• safeguards against mis-match between the abstract data-world and the real world in which impacts arise;
• mechanisms to ensure the reasonableness and proportionality of decisions made and actions taken based on the inferences; and
• processes whereby decisions can be contested.
Yet, despite the substantial catalogue of problems with data meaning, data quality, and inconsistencies among data-sets, data analytics teaching and practice invest a remarkably small amount of effort into quality assurance. That is the case even with long-established forms of data analytics. The reason such cavalier behaviour is possible is discussed in the following section.
AI/ML-based data analytics, on the other hand, is inherently incapable of addressing any of these issues. Further, the opacity issue overlays all of the other problems. Pre-AI, genuinely ‘algorithmic’ inferencing is capable of delivering explanations, enabling the various elements of accountability to function. Rule-based ‘expert systems’ dilute explainability. AI/ML inferencing, on the other hand, comprehensively fails the explainability test, and undermines accountability.
Procedural fairness has long been a requirement in the hitherto conventional environment of human-made or at least human-mediated decisions, for which courts demand a rational explanation. In the new world of AI, and particularly AI/ML, decisions are being imposed and actions taken that are incapable of being explained and justified before a court of law. The need for effective regulatory mechanisms is clear. What is far less clear is how protective mechanisms can be structured, and whether they are in place, or at least emergent.
3.Regulatory alternatives
AI may have very substantial impacts, both good and ill, both intended and accidental, and both anticipated and unforeseen. Building on the above review, this section presents an analysis of the regulatory spectrum, to support assessment of whether the threats inherent in AI applied to surveillance can be dealt with appropriately. The regulatory framework proposed in Clarke (2021a) is applied, in particular the Regulatory Layers in s.2.2, presented in graphical form in Fig. 1.
Figure 1.
A hierarchy of regulatory mechanisms.
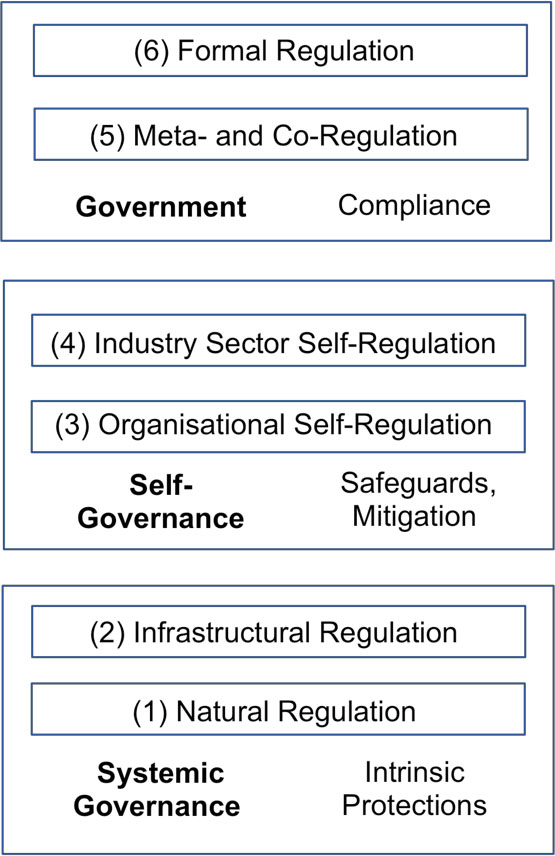
The foundational layer, (1) Natural Regulation, is a correlate of the natural control processes that occur in biological systems. It comprises natural influences intrinsic to the relevant socio-economic system, such as countervailing power by those affected by an initiative, activities by competitors, reputational effects, and cost/benefit trade-offs. It is incumbent on any party that argues for regulatory intervention to demonstrate that such natural influences as exist are inadequate to prevent harms arising. In the case of AI, marketing energy and unbounded adopter enthusiasm exist, and surveillance is an idea in good standing. These appear to go close to entirely negating the effects of natural regulatory processes.
The second-lowest layer, (2) Infrastructural Regulation, is exemplified by artefacts like the mechanical steam governor. It comprises particular features of the infrastructure that reinforce positive aspects and inhibit negative aspects of the relevant socio-economic system. A popular expression for infrastructural regulation in the context of IT is ‘[US] West Coast Code’ (Lessig, 1999; Hosein et al., 2003), which posits that software, and more generally architecture, have regulatory impact. Another such notion is security-by-design (Anderson, 2020). If privacy-by-design (Cavoukian, 2009) is ever articulated, and graduates beyond aspirational status, it would also represent a Layer (2) intervention. However, it appears very challenging to embed safeguards within AI-based software and artefacts (Clarke, 1993).
At the uppermost layer of the regulatory hierarchy, (7) Formal Regulation exercises the power of a parliament through statutes and delegated legislation such as Regulations. Laws demand compliance with requirements that are expressed in more or less specific terms, and are complemented by sanctions, enforcement powers and resources, and actual enforcement. Lessig (1999) refers to formal regulation as ‘[US] East Coast Code’.
Formal regulation appears to be the most logical approach when confronted by a threat of the magnitude that AI may prove to be. However, IT providers and insufficiently critical user organisations clamour for the avoidance of constraints on innovation. Corporate power has been instrumental over many decades in greatly reducing regulatory commitment in many jurisdictions and in many contexts. De-regulation and ‘better regulation’ movements have achieved ratcheting back of existing controls, commonly followed by unacceptable levels of harm, stimulating clumsy re-regulation (Braithwaite & Drahos, 1999). Safeguards have also been avoided through the outsourcing of both activities and responsibilities, including the use of low-regulation havens, and jurisdictional arbitrage. In the public sector, key factors include the drift from subcontracting, via comprehensive outsourcing, to public-private partnerships, and on towards the corporatised state (Schmidt & Cohen, 2014). A particular factor that appears to have largely ‘flown under the radar’ to date is the conversion of locally-installed software products to remotely-provided services (AI as a Service – AIaaS), of which IBM’s Watson was an early exemplar.
Several intermediate forms lie between the informal and formal ends of the regulatory hierarchy. Examples of (3) Organisational Self-Regulation include internal codes of conduct and ‘customer charters’, and self-restraint associated with expressions such as ‘business ethics’ and ‘corporate social responsibility’ (Parker, 2002). Layer (4) Industry Sector Self-Regulation involves schemes that express technical or process standards, codes of conduct or of practice or of ethics, and industry Memoranda of Understanding (MoUs). These commonly lack much impact, because organisations use them primarily as means to create an appearance of safeguards and thereby avoid formal regulatory activity. Braithwaite (2017) notes that “self-regulation has a formidable history of industry abuse of privilege” (p. 124). The conclusion of Gunningham & Sinclair (2017) is that ‘voluntarism’ is generally an effective regulatory element only when it exists in combination with ‘command-and-control’ components.
Other, intermediate forms have emerged that have been claimed to offer greater prospects of achieving regulatory objectives. These are clustered into layer (6) Meta- and Co-Regulation. In many areas, convincing arguments can reasonably be made by regulatees to the effect that government is poorly placed to cope with the detailed workings of complex industry sectors and/or the rate of change in industries’ structures, technologies and practices. Hence, the argument proceeds, parliaments should legislate the framework, objectives and enforcement mechanisms, but delegate the articulation of the detailed requirements (Ayres & Braithwaite, 1992; Parker, 2007). In practice, examples of effective layer (6) designs are uncommon, because the interests of regulatees dominate, advocates for the nominal beneficiaries lack influence and in many cases are not even at the table, and the powers of regulators are so weak that the resulting ‘enforceable Codes’ are almost entirely ineffective. For this reason, the framework in Fig. 1 also identifies the commonly-experienced layer (5) Pseudo Meta- and Co-Regulation
Despite the sceptical tone of the above analysis, several techniques in the mid-layers (3) to (6) of the hierarchy might make contributions, if they are elements within a complex of safeguards. Organisational risk assessment and management is one such technique. However, it considers risks only from the viewpoint of the organisation itself. For example, the focus of the relevant ISO Standards series (31000) is on ‘managing risks faced by organizations’. Harm to stakeholders is only within-scope where the stakeholder has sufficient power to undermine fulfilment of the organisation’s objectives. It is highly desirable that risk assessment and management processes also be conducted for those stakeholders that have legitimacy but lack power (Achterkamp & Vos, 2008). Although multi-stakeholder risk assessment is feasible (Clarke, 2019b), it remains highly unusual.
Another approach to identifying or anticipating potential harm, and devising appropriate safeguards, is impact assessment. This is a family of techniques that has matured in the environmental context, is understood in the privacy arena in theory but is to date very poorly applied (Clarke, 2009), and is emergent in broader areas of social concern (Becker & Vanclay, 2003). Impact assessment has also been described in the specific context of surveillance (Wright & Raab, 2012). However, there is no impetus for any such process to be undertaken, and little chance is evident of such approaches assisting powerless stakeholders harmed by AI.
A further possible source of protection might be application of ‘the precautionary principle’ (Wingspread, 1998). Its strong form exists in some jurisdictions’ environmental laws, along the lines of: “When human activities may lead to morally unacceptable harm that is scientifically plausible but uncertain, actions shall be taken to avoid or diminish that potential harm” (TvH, 2006). In the context of AI, on the other hand, the ‘principle’ has achieved no higher status than an ethical norm to the effect that: ‘If an action or policy is suspected of causing harm, and scientific consensus that it is not harmful is lacking, then the burden of proof falls on those taking the action’.
Finally, the notion of ‘ethically-based principles’ has been popular during the latter part of the decade to 2020, with a wave of ‘Principles for Responsible AI’ published. The documents range from trivial public relations documents from ICT corporations to serious-minded proposals from policy agencies and public interest advocates. Several catalogues have been developed, and analyses undertaken, e.g. Zeng et al., (2019), Clarke, (2019c) and Jobin et al., (2019). The second of those analyses developed a consolidated super-set of 50 Principles. This was then used as a basis for assessing the coverage of multiple further sets. The conclusion from that assessment was that “The main impression is of sparseness, with remarkably limited consensus [even on quite fundamental requirements]” (Clarke, 2019c, p. 415).
The likelihood of any combination of Layer (1)–(5) elements providing effective protection for public interests against the ravages of AI appears very low. What, then, are the prospects of effective interventions at Layers (6) and (7), Formal, Meta- and Co-Regulation?
4.The possibility of formal regulation of AI in surveillance
A new phase was ushered in by a proposal for statutory intervention published by the European Commission (EC) in April 2021. This is sufficiently significant that the Proposal is evaluated here as a proxy for formal regulation generally.
4.1The European Commission’s proposal
The EC’s announcement was of “new rules and actions for excellence and trust in Artificial Intelligence”, with the intention to “make sure that Europeans can trust what AI has to offer”. The document’s title was a ‘Proposal for a Regulation on a European approach for Artificial Intelligence’ (EC, 2021), and the draft statute is termed the Artificial Intelligence Act (AIA).
The document of 2021 is formidable, and the style variously eurocratic and legalistic. It comprises an Explanatory Memorandum, pp. 1–16, a Preamble, in 89 numbered paragraphs on pp. 17–38, and the proposed Regulation, in 85 numbered Articles on pp. 38–88, supported by 15 pages of Annexes.
A first difficulty the document poses is that the term “AI System” is defined in a manner inconsistent with mainstream usage. It omits various forms of AI (such as natural language understanding, robotics and cyborgisation), and encompasses various forms of data analytics that are not AI (specifically, “statistical approaches, Bayesian estimation, search and optimization methods”. These pre-date the coinage of the term ‘AI’ in 1955, and are commonly associated with operations research and data mining/‘data analytics’). A more descriptive term for the proposed statute would be ‘Data Analytics Act’.
The EC proposes different approaches for each of four categories of AI (qua data analytics), which it terms ‘Levels of Risk’: unacceptable, high, limited and minimal. A few “AI Practices” would be prohibited (Art. 5). A number of categories of “High-Risk AI Systems” would be subject to a range of provisions (Arts. 6–7, 8–51, Annexes II-VII). A very limited transparency requirement would apply to a small number of categories of “AI Systems” (Art. 52). All other “AI Systems” would escape regulation by the AIA (although not other law that may be applicable in particular circumstances, such as human rights law and the GDPR).
The consolidated set of 50 Principles was used to assess the sole category to which safeguards would apply, “High-Risk AI Systems”. A comprehensive report is provided in an unpublished working paper (Clarke, 2021b). Applying a scoring technique explained in Clarke (2021b), the EC Proposal was found to be highly deficient, scoring only 14.7/50. Of the 50 Principles, 25 scored nothing, and a further 8 achieved less than 0.5 on a scale of 0.0 to 1.0. The foundational Themes 1-4 achieved only 4%, 0%, 33% and 28% of their possible scores, for a total of 20%. The only 3 of the 10 Themes with a Pass-level score are 8 (Exhibit Robustness and Resilience – 68%), 5 (Ensure Consistency with Human Values and Human Rights – 60%), and 9 (Ensure Accountability for Obligations – 50%).
During the ‘ethical guidelines’ phase, the EC’s contribution, the “Ethics Guidelines for Trustworthy AI” prepared by a “High-Level Expert Group on Artificial Intelligence” (EC, 2019) had achieved easily the highest score against the consolidated set of 50 Principles, with 74%. The disjunction between the EC Proposal (EC 2021) and the earlier ‘Ethics Guidelines’ is striking. Key expressions in the earlier document, such as ‘Fairness’, ‘Prevention of Harm’, ‘Human Autonomy’, ‘Human agency’, ‘Explicability’, ‘Explanation’, ‘Well-Being’ and ‘Auditability’, are nowhere to be seen in the body of the 2021 Proposal, and ‘stakeholder participation’ and ‘auditability’ are not in evidence.
The conclusion reached by the assessment was that “the EC’s Proposal is not a serious attempt to protect the public. It is very strongly driven by economic considerations and administrative convenience for business and government, with the primary purposes being the stimulation of the use of AI systems. The public is to be lulled into accepting AI systems under the pretext that protections exist. The social needs of the affected individuals have been regarded as a constraint not an objective. The draft statute seeks the public’s trust, but fails to deliver trustworthiness” (Clarke, 2021b).
The analysis reported in this article was undertaken during the third and fourth quarters of 2021, and reflects the original, April 2021 version of the EU Draft Bill. The EU subsequently published a ‘Compromise Text’, on 29 November 2021. This has not been evaluated. Initial impressions are, however, that the dominance of economic over social objectives has been consolidated; and new exemptions have been created that appear to increase the level of irresponsibility. The use of AI in research is now to be exempted. So are contributors to product development. Of even greater concern is that ‘general purpose AI’ technology is also now out-of-scope. The core of every AI application is thereby free of quality constraints, and its creators are free from any form of liability. The second version appears to be an even greater breach of public trust than the first.
4.2The proposal’s implications for AI and surveillance
Assessment was undertaken of the extent to which the EC’s Proposal affects AI applications to surveillance. Relevant extracts from EC (2021) are provided in an Annex to this article.
Of the four categories of Prohibited AI Practices (Art. 5), two are related to surveillance: (c) Social scoring (“the evaluation or classification of the trustworthiness of natural persons
In addition, scope exists for ‘gaming’ the regulatory scheme, because, a nominally “prohibited AI practice” can be developed, deployed in particular contexts, then withdrawn, without a prior or even contemporaneous application for authorisation, let alone approval. Moreover, any Member State can override the prohibition (Art.5(4)). Hence many systems in these categories would achieve exemption from the scheme.
Multiple ‘High-Risk AI Systems’ (Arts. 6–7, 8–51, Annexes II-VII, particularly III) are also relevant to surveillance. Some instances of “1. Biometric identification and categorisation of natural persons” (Annex III-1) are nominally defined as within-scope. However, the provisions appear likely to be subject to lengthy legal interpretation, and a great many systems may achieve exemption-by-design. Some spatial surveillance is also defined to be within this category, as “2. Management and operation of critical infrastructure”, but only where the system is a “safety component” in “the management and operation of road traffic and the supply of water, gas, heating and electricity”. If the system, for example, draws inferences about people’s usage of roads, or households’ usage of energy, it is not high-risk, and hence subject to no regulatory protections under the EC Proposal.
More positively, a system is subject to some conditions if it is “5.
However, even for those systems that do not fit into the array of escape-clauses, the statutory obligations (Arts. 8–29) are very limited in comparison with those in the consolidated set of 50 Principles. Further, most such systems are either absolved from undergoing conformity assessment (Art. 41–43, 47) or are subject to mere self-assessment. It appears that considerably more effort may be expended in finding ways to avoid the requirements than in complying with them.
Finally, of the four categories of AI systems to which a limited transparency obligation applies (Art. 52), two are related to surveillance, but are heavily qualified: 2. “an emotion recognition and/or detection system” except where “permitted by law to detect, prevent and investigate criminal offences, unless those systems are available for the public to report a criminal offence”, and 3. “a biometric categorisation system”, separately described as “an AI system to determine association with (social) categories based on biometric data”, except where “permitted by law to detect, prevent and investigate criminal offences”.
The large majority of applications of AI to surveillance would be entirely unaffected by the EC Proposal should it be enacted in anything resembling its current form. This includes many applications of AI that lie very close to the boundary of what the EC considers should be prohibited, and many applications that the EC considers to be ‘high-risk’. Even those ‘high-risk’ applications that are subject to the new law would be subject to very weak requirements. Advocates for the public interest are justified in treating the EC Proposal with derision, both generally in respect of AI, and specifically in relation to the application of AI to surveillance.
5.Conclusions
There is strong evidence that data analytics practices in general are not subject to adequate safeguards for public interests, even before AI’s incursions into the field. One prominent example is the RoboDebt scandal in Australia, in which a new AUD 1 billion system both incorrectly and illegally imposed automatically-generated debts on welfare-recipients, resulting in serious impacts on half a million individuals, AUD 2 billion of repayments, and withdrawal of the scheme (Clarke, 2020). In another case, 20,000 false accusations by the taxation authority of fraudulent drawing of child benefits resulted in the Dutch government resigning (Erdbrink, 2021).
The present article has summarised the many signs of alarm about the damage AI can do, both generally, and specifically when applied to surveillance. To the formal evidence can be added the implicit recognition by AI proponents that the public has much to fear, in that they have undertaken a ‘charm offensive’ involving good news stories about AI applications, and utterances of ‘principles’ further glossed by the word ‘ethical’.
A review of the many forms that regulation can take found nothing outside the uppermost layers of formal regulation that would appear at all likely to deliver meaningful safeguards for the public against AI. Until the second quarter of 2021, there was very little evidence of formal regulation being emergent. The first such proposal, from the EC, when reviewed against a consolidated set of ‘principles for responsible AI’, has been found to be extremely poor.
Given these inadequacies, and the power of the government agencies and corporations that apply surveillance, the current prospects of effective control being achieved over AI applications to surveillance are extremely low. The history of deregulatory/regulatory cycles suggests that, unless very prompt action is taken to elevate both the urgency and the quality of proposals, regulatory protections will come long after the damage has commenced, and in the form of ill-considered, kneejerk reactions to the damage arising during the early, ‘wild west’ phase of deployment.
Acknowledgments
This article had the benefit of substantial comments from two reviewers, which materially assisted the author in clarifying and tightening the analyses.
The Open Access publication of this paper was supported by the Panelfit project. This project has received funding from the European Union’s Horizon 2020 research and innovation programme under grant agreement No 788039. This document reflects only the author’s view and the Agency is not responsible for any use that may be made of the information it contains.
Annex
Extracts of Passages Relevant to Surveillance from the European Commission’s Proposed Regulatory Scheme for ‘AI’ (EC 2021) are available at: http://rogerclarke.com/DV/AIP-S-SurveillancePassages-210927.pdf.
References
[1] | Achterkamp, M.C., & Vos, J.F.J. ((2008) ). Investigating the Use of the Stakeholder Notion in Project Management Literature: A Meta-Analysis. International Journal of Project Management, 26: , 749-757. |
[2] | Albus, J. S. ((1991) ). Outline for a theory of intelligence. IEEE Trans. Systems, Man and Cybernetics, 21: (3), 473-509. http://citeseerx.ist.psu.edu/viewdoc/download?doi=10.1.1.410.9719&rep=rep1&type=pdf. |
[3] | Anderson, C. ((2008) ). The End of Theory: The Data Deluge Makes the Scientific Method Obsolete. Wired Magazine, 16: (7), http://archive.wired.com/science/discoveries/magazine/16-07/pb_theory. |
[4] | Anderson, R. ((2020) ). Security Engineering: A Guide to Building Dependable Distributed Systems, 3rd Edition, Wiley. |
[5] | Ayres, I., & Braithwaite, J. ((1992) ). Responsive Regulation: Transcending the Deregulation Debate, Oxford Univ. Press. |
[6] | Bacchi, U. ((2021) ). EU’s lie-detecting virtual border guards face court scrutiny. Reuters, https://www.reuters.com/article/europe-tech-court-idUSL8N2KB2GT. |
[7] | Becker, H., & Vanclay, F. ((2003) ). The International Handbook of Social Impact Assessment. Cheltenham: Edward Elgar. |
[8] | Bentham, J. Panopticon; or, the Inspection House, London, 1791. |
[9] | Boden, M. ((2016) ). AI: Its Nature and Future, Oxford University Press. |
[10] | Braithwaite, J. ((2017) ). Types of responsiveness. Chapter 7 in Drahos, 117-132. http://press-files.anu.edu.au/downloads/press/n2304/pdf/ch07.pdf. |
[11] | Braithwaite, J., & Drahos, P. ((1999) ). Ratcheting Up and Driving Down Global Regulatory Standards. Development, 42: (4), 109-114. http://citeseerx.ist.psu.edu/viewdoc/download?doi=10.1.1.1070.9909&rep=rep1&type=pdf. |
[12] | Breyer, P. ((2021) ). EU-funded technology violates fundamental rights. About Intel. https://aboutintel.eu/transparency-lawsuit-iborderctrl/. |
[13] | Brin, D. ((1998) ). The Transparent Society, Addison-Wesley. |
[14] | Campbell, Z., Chandler, C.L., & Jones, C. ((2020) ). Sci-fi surveillance: Europe’s secretive push into biometric technology. The Guardian. https://www.theguardian.com/world/2020/dec/10/sci-fi-surveillance-europes-secretive-push-into-biometric-technology. |
[15] | Cater, L., & Heikkilä, M. ((2021) ). Your boss is watching: How AI-powered surveillance rules the workplace. Politico, 27. https://www.politico.eu/article/ai-workplace-surveillance-facial-recognition-software-gdpr-privacy/. |
[16] | Cavoukian, A. ((2009) ). Privacy by Design: The 7 Foundational Principles. Privacy By Design, 2010. http://www.privacybydesign.ca. |
[17] | Clarke, R. ((1991) ). A Contingency Approach to the Application Software Generations. Database, 22: (3), 23-34. PrePrint at http://www.rogerclarke.com/SOS/SwareGenns.html. |
[18] | Clarke, R. ((1993) ). Asimov’s Laws of Robotics: Implications for Information Technology. IEEE Computer, 26: (12), 53-61 and 27,1 (January 1994), pp. 57-66. PrePrint at http://www.rogerclarke.com/SOS/Asimov.html. |
[19] | Clarke, R. ((2001) ). Person-Location and Person-Tracking: Technologies, Risks and Policy Implications. Information Technology & People, 14: (2), 206-231. PrePrint at http://www.rogerclarke.com/DV/PLT.html. |
[20] | Clarke, R. ((2009) ). Privacy Impact Assessment: Its Origins and Development. Computer Law & Security Review, 25: (2), 123-135. PrePrint at http://www.rogerclarke.com/DV/PIAHist-08.html. |
[21] | Clarke, R. ((2010) ). What is Uberveillance? (And What Should Be Done About It?). IEEE Technology and Society, 29: (2), 17-25. PrePrint at http://www.rogerclarke.com/DV/RNSA07.html. |
[22] | Clarke, R. ((2014) a). What Drones Inherit from Their Ancestors. Computer Law & Security Review, 30: (3), 247-262. PrePrint at http://www.rogerclarke.com/SOS/Drones-I.html. |
[23] | Clarke, R. ((2014) b). Promise Unfulfilled: The Digital Persona Concept, Two Decades Later. Information Technology & People, 27: (2), 182-207. PrePrint at http://www.rogerclarke.com/ID/DP12.html. |
[24] | Clarke, R. ((2016) ). Big Data, Big Risks. Information Systems Journal, 26: (1), 77-90. PrePrint at http://www.rogerclarke.com/EC/BDBR.html. |
[25] | Clarke, R. ((2019) a). Risks Inherent in the Digital Surveillance Economy: A Research Agenda. Journal of Information Technology, 34: (1), 59-80. PrePrint at http://www.rogerclarke.com/EC/DSE.html. |
[26] | Clarke, R. ((2019) b). Why the World Wants Controls over Artificial Intelligence. Computer Law & Security Review, 35: (4). 423-433. PrePrint at http://www.rogerclarke.com/EC/AII.html. |
[27] | Clarke, R. ((2019) c) . Principles and Business Processes for Responsible AI. Computer Law & Security Review, 35: (4), 410-422. PrePrint at http://www.rogerclarke.com/EC/AIP.html. |
[28] | Clarke, R. ((2020) ). Centrelink’s Big Data ‘Robo-Debt’ Fiasco of 2016-20. Xamax Consultancy Pty Ltd, 2018-20. http://www.rogerclarke.com/DV/CRD17.html. |
[29] | Clarke, R. ((2021) a). A Comprehensive Framework for Regulatory Regimes as a Basis for Effective Privacy Protection. Proc. 14th Computers, Privacy and Data Protection Conference (CPDP’21), Brussels, 27-29 January 2021, PrePrint at http://rogerclarke.com/DV/RMPP.html. |
[30] | Clarke, R. ((2021) b). The EC’s Proposal for Regulation of AI: Evaluation against a Consolidated Set of 50 Principles. Xamax Consultancy Pty Ltd, August 2021. http://www.rogerclarke.com/DV/AIP-EC21.html. |
[31] | Clarke, R. ((2022) ). Responsible Application of Artificial Intelligence to Surveillance: What Prospects? Xamax Consultancy Pty Ltd, December 2021, PrePrint at http://rogerclarke.com/DV/AIP-S.html#S. |
[32] | Clarke, R., & Wigan, M. ((2011) ). You Are Where You’ve Been: The Privacy Implications of Location and Tracking Technologies. Journal of Location Based Services, 5: (3-4), 138-155. PrePrint at http://www.rogerclarke.com/DV/YAWYB-CWP.html. |
[33] | Cole, S.A. ((2004) ). History of Fingerprint Pattern Recognition. Ch.1, pp. 1-25, in Ratha N., & Bolle R. (eds.) ‘Automatic Fingerprint Recognition Systems’, SpringerLink, (2004) . |
[34] | Crawford, K. ((2021) ). Atlas of AI’ Yale University Press. |
[35] | Daugman, J. ((1998) ). History and Development of Iris Recognition. http://www.cl.cam.ac.uk/users/jgd1000/history.html. |
[36] | DeLua, J. ((2021) ). Supervised vs. Unsupervised Learning: What’s the Difference? IBM, 12 March 2021. https://www.ibm.com/cloud/blog/supervised-vs-unsupervised-learning. |
[37] | DI (2019). AI Ethics Principles. Department of Industry, Innovation & Science, https://www.industry.gov.au/data-and-publications/building-australias-artificial-intelligence-capability/ai-ethics-framework/ai-ethics-principles. |
[38] | Drahos, P. (ed.) ((2017) ) Regulatory Theory: Foundations and applications. ANU Press, 2017. https://press.anu.edu.au/publications/regulatory-theory#pdf. |
[39] | Duursma ((2018) ). The Risks of Artificial Intelligence. Studio OverMorgen, May 2018. https://www.jarnoduursma.nl/the-risks-of-artificial-intelligence/. |
[40] | EC (2019). Ethics Guidelines for Trustworthy AI. High-Level Expert Group on Artificial Intelligence, European Commission, April 2019. https://ec.europa.eu/newsroom/dae/document.cfm?doc_id=58477. |
[41] | EC (2021). Document 52021PC0206. European Commission, viewed 14 July 2021. https://eur-lex.europa.eu/legal-content/EN/HIS/?uri=COM:2021:206:FIN. |
[42] | Erdbrink, T. ((2021) ). Government in Netherlands Resigns After Benefit Scandal. The New York Times, 15. https://www.nytimes.com/2021/01/15/world/europe/dutch-government-resignation-rutte-netherlands.html. |
[43] | Feldstein, S. ((2019) ). The Global Expansion of AI Surveillance. Carnegie Endowment for International Peace. 2019. https://carnegieendowment.org/files/WP-Feldstein-AISurveillance_final1.pdf. |
[44] | Foucault, M. ((1977) ). Discipline and Punish: The Birth of the Prison. Peregrine, London, 1975, trans. 1977. |
[45] | Gandy, O.H. ((1989) ). The Surveillance Society: Information Technology and Bureaucratic Social Control. Journal of Communication, 39: (3). https://www.dhi.ac.uk/san/waysofbeing/data/data-crone-gandy-1989.pdf. |
[46] | Gandy, O.H. ((1993) ). The Panoptic Sort: Critical Studies in Communication and in the Cultural Industries. Westview, Boulder CO. |
[47] | Gandy, O.H. ((2021) ). The Panoptic Sort: A Political Economy of Personal Information. Oxford University Press. |
[48] | Gose, E., Johnsonbaugh, R., & Jost, S. ((1996) ). Pattern recognition and image analysis. Prentice Hall. |
[49] | Gunningham, N., & Sinclair, D. ((2017) ). Smart Regulation, Chapter 8 in Drahos (2017), pp. 133-148. http://press-files.anu.edu.au/downloads/press/n2304/pdf/ch08.pdf. |
[50] | Heikkilä, M. ((2021) ). The rise of AI surveillance. Politico, 26 May 2021. https://www.politico.eu/article/the-rise-of-ai-surveillance-coronavirus-data-collection-tracking-facial-recognition-monitoring/. |
[51] | Hendry, J. ((2021) ). Telstra creates standards to govern AI buying, use. itNews, 15 July 2021. https://www.itnews.com.au/news/telstra-creates-standards-to-govern-ai-buying-use-567005. |
[52] | Hosein, G., Tsavios, P., & Whitley, E. ((2003) ). Regulating Architecture and Architectures of Regulation: Contributions from Information Systems. International Review of Law, Computers and Technology, 17: (1): 85-98. |
[53] | iBorderCtrl ((2016) )‘IBorderCtrl: The Project’ iBorderCtrl, 2016. https://www.iborderctrl.eu/The-project |
[54] | Indurkhya, N., & Damerau, F.J. (eds.) ((2010) ). Handbook of natural language processing. CRC Press. |
[55] | Jobin, A., Ienca, M., & Vayena, E. ((2019) ). The global landscape of AI ethics guidelines. Nature Machine Intelligence, 1: , 389-399. doi: 10.1038/s42256-019-0088-2. |
[56] | Kurzweil, R. ((2005) ). The Singularity is Near. Viking Books. |
[57] | LaValle, S., Lesser, E., Shockley, R., Hopkins, M.S., & Kruschwitz, N. ((2011) ). Big Data, Analytics and the Path From Insights to Value. Sloan Management Review (Winter 2011Research Feature), 21 December 2010. http://sloanreview.mit.edu/article/big-data-analytics-and-the-path-from-insights-to-value/. |
[58] | Lessig, L. ((1999) ). Code and Other Laws of Cyberspace. Basic Books. |
[59] | Lyon, D. ((2001) ). Surveillance Society: Monitoring in Everyday Life. Open University Press. |
[60] | McCarthy, J. ((2007) ). What is artificial intelligence? Department of Computer Science, Stanford University, November 2007. http://www-formal.stanford.edu/jmc/whatisai/node1.html. |
[61] | McCarthy, J., Minsky, M.L., Rochester, N., & Shannon, C.E. ((1955) ). A Proposal for the Dartmouth Summer Research Project on Artificial Intelligence. Reprinted in AI Magazine, 27: (4). https://www.aaai.org/ojs/index.php/aimagazine/article/viewFile/1904/1802. |
[62] | Mann, S. ((2005) ). Equiveillance: The equilibrium between Sur-veillance and Sous-veillance. Opening Address, Computers, Freedom and Privacy. http://wearcam.org/anonequity.htm. |
[63] | Mann, S., Nolan, J., & Wellman, B. ((2003) ). Sousveillance: Inventing and Using Wearable Computing Devices for Data Collection in Surveillance Environments. Surveillance & Society, 1: (3), 331-355. https://ojs.library.queensu.ca/index.php/surveillance-and-society/article/view/3344/3306. |
[64] | Marx, G.T. ((1985) ). The Surveillance Society: The Threat of 1984-Style Techniques. The Futurist, June 1985, pp. 21-26. http://web.mit.edu/gtmarx/www/futurist_surv_soc.pdf. |
[65] | Marx, G.T. ((2016) ). Windows into the Soul: Surveillance and Society in an Age of High Technology. Uni. of Chicago Press. |
[66] | Mayer-Schonberger, V., & Cukier, K. ((2013) ). Big Data: A Revolution That Will Transform How We Live, Work and Think. John Murray. |
[67] | Medler, D.A. ((1998) ). A Brief History of Connectionism. Neural Computing Surveys, 1: (2), 18-72. https://citeseerx.ist.psu.edu/viewdoc/download?doi=10.1.1.86.7504&rep=rep1&type=pdf. |
[68] | Michael, K., & Michael, M.G. (eds.) ((2007) ). From Dataveillance to (Uberveillance) and the Realpolitik of the Transparent Society. Proc. 2nd Workshop on Social Implications of National Security, Uni. of Wollongong, October 2007. http://works.bepress.com/kmichael/51/. |
[69] | O’Shaughnessy, D. ((2008) ). Automatic speech recognition: History, methods and challenges. Pattern Recognition, 41: (10), 2965-2979. |
[70] | Pal, S.K., & Mitra, P. ((2004) ). Pattern Recognition Algorithms for Data Mining. Chapman & Hall. |
[71] | Parker, C. ((2007) ). Meta-Regulation: Legal Accountability for Corporate Social Responsibility? in McBarnet D., Voiculescu A., & Campbell T (eds), The New Corporate Accountability: Corporate Social Responsibility and the Law. |
[72] | Piprani, B., & Ernst, D. ((2008) ). A Model for Data Quality Assessment. Proc. OTM Workshops, (5333), 750-759. |
[73] | PredPol ((2021) ). Predictive Policing Technology: The PredPol Algorithm. Predpol, Accessed 28 Dec 2021. https://www.predpol.com/technology/. |
[74] | Rosenfeld, A., & Wechsler, H. ((2000) ). Pattern Recognition: Historical Perspective and Future Directions. Int J Imaging Syst Technol, 11: , 101-116. http://xrm.phys.northwestern.edu/research/pdf_papers/2000/rosenfeld_ijist_2000.pdf. |
[75] | Rule, J.B. ((1974) ). Private lives and public surveillance: Social control in the computer age’ Schocken. |
[76] | Russell, S.J., & Norvig, P. ((2009) ). Artificial Intelligence: A Modern Approach. Prentice Hall, 3rd edition. |
[77] | Ryan, A., Cohn, J., Lucey, S., Saragih, J., Lucey, P., la Torre, F.D., & Rossi, A. ((2009) ). Automated Facial Expression Recognition System. Proc. Int’l Carnahan Conf. on Security Technology, pp. 172-177. https://www.researchgate.net/profile/Jeffrey-Cohn/publication/224082157_Automated_Facial_Expression_Recognition_System/links/02e7e525c3cf489da1000000/Automated-Facial-Expression-Recognition-System.pdf. |
[78] | Scherer, M.U. ((2016) ). Regulating Artificial Intelligence Systems: Risks, Challenges, Competencies, and Strategies. Harvard Journal of Law & Technology, 29: (2), 353-400. http://euro.ecom.cmu.edu/program/law/08-732/AI/Scherer.pdf. |
[79] | Schmidt, E., & Cohen, J. ((2014) ). The New Digital Age: Reshaping the Future of People, Nations and Business. Knopf, 2013. |
[80] | Shanks, G., & Darke, P. ((1998) ). Understanding Data Quality in a Data Warehouse. The Australian Computer Journal, 30: , 122-128. |
[81] | Simon, H.A. ((1960) ). The Shape of Automation. reprinted in various forms, 1960, 1965, quoted in Weizenbaum J. (1976), pp. 244-245. |
[82] | TvH: Telstra Corporation Limited v Hornsby Shire Council. NSWLEC 133, 101-107, 113, 125-183 (2006). http://www.austlii.edu.au/au/cases/nsw/NSWLEC/2006/133.htm. |
[83] | Wang, R.Y., & Strong, D.M. ((1996) ). Beyond Accuracy: What Data Quality Means to Data Consumers. Journal of Management Information Systems, 12: (4), 5-33. |
[84] | Wingspread ((1998) ). Wingspread statement on the precautionary principle. Science & Environmental Health Network. https://www.sehn.org/precautionary-principle-understanding-science-in-regulation. |
[85] | Wright, D., & Raab, C.D. ((2012) ). Constructing a surveillance impact assessment. Computer Law & Security Review, 28: (6), 613-626. https://www.dhi.ac.uk/san/waysofbeing/data/data-crone-wright-2012a.pdf. |
[86] | Yampolskiy, R.V., & Spellchecker, M.S. ((2016) ). Artificial Intelligence Safety and Cybersecurity: a Timeline of AI Failures. arXiv. https://arxiv.org/pdf/1610.07997. |
[87] | Zeng, Y., Lu, E., & Huangfu, C. ((2019) ). Linking Artificial Intelligence Principles. Proc. AAAI Workshop on Artificial Intelligence Safety (AAAI-Safe AI 2019), 27 January 2019. https://arxiv.org/abs/1812.04814. |
[88] | Zuboff, S. ((2015) ). Big other: Surveillance capitalism and the prospects of an information civilization. Journal of Information Technology, 30: (1), 75-89. https://cryptome.org/2015/07/big-other.pdf. |
Appendices
Author biography
Roger Clarke is Principal of Xamax Consultancy Pty Ltd, Canberra. He is also a Visiting Professor associated with the Allens Hub for Technology, Law and Innovation in UNSW Law, and a Visiting Professor in the Research School of Computer Science at the Australian National University.
http://rogerclarke.com
https://scholar.google.com.au/citations?user=V3s6CWYAAAAJ