Citizens’ attitudes towards automated decision-making
Abstract
Public organisations are starting to show an interest in automated decision-making (ADM). So far, existing research focuses on the governmental perspective on this phenomenon. Less attention is paid to citizens’ views on ADM. The aim of this study is to provide empirical insights into citizen awareness of and beliefs about ADM in public-sector services. To this end, we participated in an annual national survey in Sweden carried out by the SOM Institute at Gothenburg University concluding that a minority of the citizens know about the use of ADM in public-sector services. Furthermore, when computers instead of civil servants make decisions in the public-sector, citizens expect decisions by computers to become less legally secure but more impartial. They also expect ADM to take personal circumstances into account to a lesser degree and become less transparent. Finally, we found that citizens with that awareness expect decisions by computers to become more reliable and impartial. Based on our empirical findings in relation to previous research, we suggest four hypotheses on citizen’s awareness and beliefs about public-sector ADM.
1.Introduction
Public-sector digitalisation is a worldwide trend that has been in progress since the 1950’s (Banister & Grönlund, 2017), and advances in IT are continuously pushing the digitalisation of public services into new areas. As recent research literature illustrates (Henriksen, 2018; Ranerup & Henriksen, 2019; Wihlborg et al., 2016), public organisations are starting to show an interest in automated decision-making (ADM). These empirical studies show how, instead of specialised public servants, public administration allows computers to make decisions using algorithms.
ADM is not a unified concept (Le Sueur, 2016). Rather, ADM in the public-sector spans a broad set of solutions. It can include decision-support systems with different degrees of human involvement in the decision-making process, as well as fully automated systems without any human involvement. The latter can also include electronically communicating the outcome of the decision without involving or informing public servants. Thus, this type of technology encompasses a variety of different designs when it comes to the autonomy of the computers in the decision processes. This paper refers to a broad notion of ADM as part of an administrative process where a computer takes the decisions with or without involvement of a public servant. Moreover, we focus on ADM related to public services for citizens, such as computers making decisions about economic support during parental leave.
As Section 2 shows, existing research on ADM in the public-sector is scarce. Moreover, researchers seem to apply a governmental perspective when studying this phenomenon (e.g. Ranerup & Henriksen, 2019; Smith et al., 2010; Snellen, 2002; Wihlborg et al., 2016), mainly analysing how ADM has changed or has the potential to change the internal workings of public administration. Thus, research devotes less attention to an external perspective, in particular, to citizens’ views on ADM and the legitimacy of public administration – although some exceptions can be found (Araujo et al., 2020; Helberger et al., 2020; Miller & Keiser, 2020).
Citizens’ perceptions of public services play a decisive role in government legitimacy (Rothstein & Teorell, 2008), making their beliefs about ADM important. Citizens compare their perception of decision-making (i.e., how it occurs) with values they hold concerning procedural fairness (i.e., how they believe it ought to occur). As a result, citizens who regard decision-making as procedurally fair tend to have greater trust in public administration (Abdelzadeh et al., 2015; Grimes, 2005). Although the increasing use of ADM is a major change that may affect citizens’ view of public administration legitimacy, we have limited knowledge about citizens’ attitudes towards the use of ADM in public-sector services.
The aim of this study is therefore to provide empirical insights into citizen awareness of and beliefs about ADM in public-sector services. To this end we draw on the expectancy-value model (Fishbein & Ajzen, 1975; Fishbein & Ajzen, 2010), assuming that citizens develop attitudes towards objects, such as ADM in public-sector services, based on how strong belief they have about an object having a certain attribute (see Fig. 1, in Section 3). Citizens who are aware of ADM are assumed to base their beliefs on experiences of ADM, while individuals who lack such awareness tend to recall assumptions or beliefs from memory. Thus, based on this model it is expected that citizens differ in their beliefs about ADM having certain attributes, in particular differences based on citizens’ awareness of ADM. We therefore pose the following research questions:
1) Are citizens aware of ADM in public-sector services?
2) What are citizens’ beliefs about ADM in public-sector services?
3) What characterises the relation between citizens’ awareness of and beliefs about ADM in public-sector services?
To answer these research questions, we participated in an annual national survey in Sweden carried out by the SOM Institute at the University of Gothenburg. From a research point of view, this study contributes with a starting point for building a knowledge base about citizens’ awareness of and beliefs about ADM. Based on our empirical results we develop four hypotheses on citizen’s awareness and beliefs about public-sector ADM, that can be used as for future research. Furthermore, although our empirical findings are bound to a specific context, they can help decision makers and policy makers understand citizens’ attitudes towards ADM.
The structure of the remainder of the paper is as follows. In the next section, we address existing research on ADM in public-sector. The third section presents the theoretical framework, and the fourth section focuses on the research method. In the fifth section, we explain the analysis and present our empirical results. The sixth section comprises a discussion of the results and their implications for research and practice, study limitations and ideas for future research. Finally, the paper ends with a set of conclusions.
2.Related research
As stated in the Introduction, existing research on ADM in the publicsector has mainly taken a governmental perspective, and in particular focuses on government officials and legislative issues. Fewer studies have applied a citizens’ perspective on ADM and the legitimacy of public administration (Araujo et al., 2020; Helberger et al., 2020; Miller & Keiser, 2020).
Miller and Keiser (2020) conclude that black citizens are more likely to favour ADM, in the context of a policy agency. These respondents are more likely, in that context, to think that ADM leads to the law being applied accurately. Helberger et al. (2020) explore Dutch citizens’ perception of ADM fairness, and their results indicate “that AI would be fairer than a human in making decisions”, while about one third of the respondents are unable to choose. Their findings show that systematic execution of rules is important for those citizens that perceive ADM as fairer. However, the same infallible execution of these rules, seems to be the main concern of those citizens being critical to ADM. An important argument for the latter category is human’s ability to consider the broader context in decision-making. Araujo et al. (2020) explore differences between perceptions of fairness, risk and usefulness of ADM in three contexts: media, health and justice. They conclude that ADM was often evaluated on par or even better than human decisions. They find no significant difference across the studied contexts with regards to fairness and risk of ADM. When it comes to usefulness in the health context, decision made by ADM is seen as more useful than human decisions.
A focus on government officials is common in studies on ADM (Bruhn, 2015; Ranerup & Henriksen, 2019; Snellen, 2002; Wihlborg et al., 2016). Wihlborg et al. (2016) analyse how the professional role of the street-level bureaucrat is changing as an effect of automation. They show how the automation becomes a ‘co-bureaucrat’ in the relation between government official and citizen. Ranerup and Henriksen (2019) apply a similar perspective in their analysis of how publicsector values come into play in ADM. They conclude that automation of socialassistance decisions in the studied municipality mainly supports the values of professionalism, efficiency and service. Bruhn (2015) analyses how the role of caseworkers changes due to new information and communication technology, such as ADM. His findings show that simple routine work is reduced, leaving caseworkers with complex cases only and worries about de-personalization of tasks.
Snellen (2002) applies a government official’s perspective when discussing how automation of public decisions risks narrowing the discretionary power of the street-level bureaucrat which might lead to disempowering government officials. He concludes that there is no straightforward answer to the question of who the winners and losers are in this development. For street-level bureaucrats, politicians and citizens, he argues that it has much to do with competence and changing structures. Smith et al. (2010) make a similar observation but in relation to automation and accountability. They argue that high-degree automation in public administration requires new forms of transparency and accountability. Also, they conclude that ADM requires a formalisation of the decision-making process. This might constrain human discretion, which potentially can have positive as well as negative effects, depending on the context. In order to manage risk in ADM, Bannister and Connolly (2020) have suggested a risk management framework; in particular, they address the risks associated with the use of artificial intelligence.
Another focus is consequences of ADM for legislation (Coglianese & Lehr, 2017; Kuziemski & Misuraca, 2020; Le Sueur, 2016; Motzfeldt & Næsborg-Andersen, 2017; Schartum, 2016; Suksi, 2020). Le Sueur (2016) argues for the importance of considering the constitutional implications of ADM. For example, he suggests that the computer programmes should be subject to parliamentary oversight to bring them under democratic control. Schartum (2016) took a similar perspective, arguing that the juridical aspects of ADM should be regarded as ‘quasi-legislation’. Following this, there is a need for increased openness and political involvement in these types of systems with digitalised and automated legal and regulatory aspects. Therefore, Schartum (2016) suggests changing legislation to better fit ADM.
Motzfeldt and Næsborg-Andersen (2017) emphasise the importance of strengthening citizens’ legal position during the development of ADM. Kuziemski and Misuraca (2020) analyse legal and policy instrument associated with three ADM systems. They highlight the risks of opacities in these implementations, and that power-related considerations seem disregarded. Suksi (2020) identifies legislative lacunae in the regulation of ADM, and suggests that legislation should be explicit about how far this type of technology can be used in public-sector. Finally, Coglianese and Lehr (2017) present a legal analysis to support agencies that want to use what they call ‘machine-learning algorithms’ for decision-making in public administration. They see the technological advancement in public administration as a fruitful way forward for improving the administrative state. Furthermore, they argue that the risk of ‘black-boxing’ the decision-making process is similar for human decision-making and ADM.
3.Theoretical framework
This study is guided by a theoretical framework that relates awareness and beliefs about ADM. Awareness refers to the cognitive knowledge of the existence of objects and their qualities. With awareness, individuals pay attention to the objects and, as a result, have mental images of them. Developing emotions (e.g. interest or identification) and judgements (e.g. trust or satisfaction) about objects and developing beliefs about them require these cognitive orientations (Lupia, 2016; Zaller, 1990). Beliefs refer to the subjective probability that objects have specific attributes. Individuals therefore expect that objects will be more or less likely to have certain attributes (Fishbein & Ajzen, 1975; Fishbein & Ajzen, 2010). An example is the expectation that decision-making in the public-sector (the object) is likely (the probability) to be impartial (the attribute) when computers make the decisions. This attribution process, by which individuals connect objects to attributes, is crucial when individuals try to understand actors, events or processes in the surrounding context (Heider, 1958; McGraw, 2001). Hence, beliefs are more developed orientations than awareness and consist of cognitive images about the qualities, construction or function of objects.
According to the expectancy-value model, individuals tend to form beliefs about objects when they become aware of the objects (Fishbein & Ajzen, 1975; Fishbein & Ajzen, 2010). The model assumes that individuals develop negative or positive attitudes towards the objects when they relate them to attributes. This attitude formation is the result of pre-existing evaluations of the attributes. For example, an individual who values impartiality will have a more positive attitude towards decision-making that the individual thinks will likely provide impartial decisions. According to the expectancy-value model, an attitude towards an object (A) is the summation of the strength of the belief (
Awareness and cognitive beliefs reside on a subjective (mental) level, while ADM functions reside on an object level. However, we focus on how individuals cognitively acknowledge ADM, which may differ from how the automated processes de facto work. The attributes that individuals expect objects to have do not necessarily correspond to the actual attributes of the objects (Fishbein & Ajzen, 2010; McGraw, 2001). Although awareness and cognitive beliefs are on a different analytical level than ADM, we assume a connection between the subjective and the object levels. For example, the existence of ADM affects (increases) the awareness of or belief about ADM, which modifies the attribution processes.
A paradox arises when exploring awareness using surveys or interviews (Converse, 1964; Tourangeau et al., 2000; Zaller, 1990). When asking people about their awareness, they may become aware of objects that they did not know before. Respondents that were unaware before the inquiry then tend to express beliefs and emotions unconnected to experiences of the object. Instead, they use as part of their attribution process assumptions, beliefs, or emotions that they activate from memory and relate to similar objects. For example, individuals may sample beliefs about computers or decision-making in the public-sector when they answer questions about automated public-sector decision-making. According to this paradox, we expect individuals who are aware of ADM to base their beliefs on direct (e.g. personal) or indirect (e.g. media) experiences of these processes, while individuals who lack such awareness tend to recall assumptions or beliefs from memory. This difference provides us with arguments for a) examining the relationship between awareness and beliefs about ADM, and b) expecting differences in beliefs between aware and unaware individuals.
Figure 1.
Analytical framework.
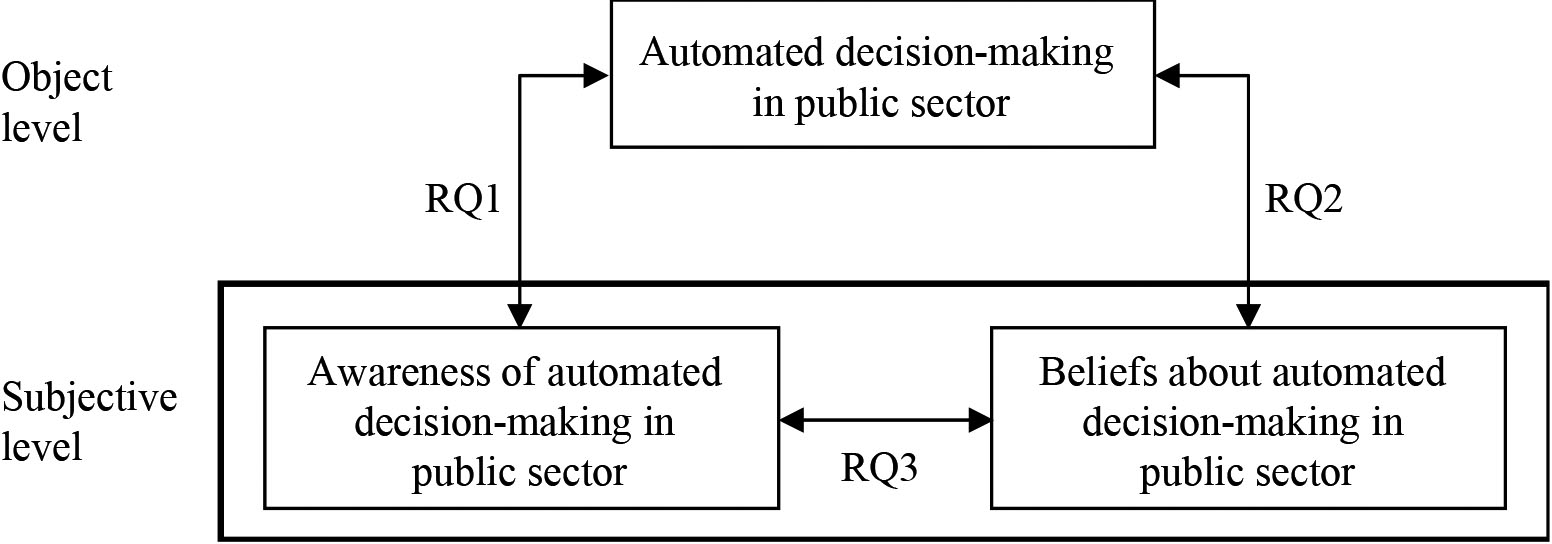
Figure 1 summarises the analytical framework. First, we explore the individuals’ awareness of ADM in the public-sector. In this part, we also analyse similarities and differences between socio-demographic groups (RQ1). Second, we examine cognitive beliefs about ADM in the public-sector (RQ2). Third, we analyse the connection between awareness and beliefs, which empirically tests our expectation about differences in beliefs according to awareness (RQ3). While the first two parts explore the relation between the subjective and object levels, the last part explores relationships on the subjective level.
Although this study is grounded in the analytical framework, the main approach within this framework is inductive in the sense that the ambition is to generate new hypothesises for future research rather than testing theoretically derived hypotheses. Our reason for choosing this inductive approach is that only a few studies have empirically explored awareness and beliefs of ADM before (Araujo et al., 2020; Helberger et al., 2020; Miller & Keiser, 2020), and the research field is thus in need of theoretical development based on empirical results. We use, therefore, a general framework to guide our empirical analyses and formulate based on the empirical results hypotheses for future studies to test.
4.Research method
We used materials from a national survey consisting of 1,746 respondents. The total selected sample included 3,500 persons, which gives a response rate of 50 per cent. The respondents were randomly selected from among persons between 16 and 85 years old, living in Sweden, which represents the population that we assume have direct or indirect experiences of ADM in the public-sector. The sampling included both Swedish and foreign citizens that had a primary address in Sweden, according to the Swedish Tax Agency. Although younger persons tend to respond to a lesser extent than older persons, the socio-demographic aspects of the sample are highly representative (Weissenbilder, 2019).
The Swedish case is interesting to study, because this context is characterized by citizens with high trust for political institutions, a welfare system built on a strong tradition of citizens’ social rights and general welfare, and a public-sector with a low level of corruption. ADM has a long tradition in Swedish agencies, and has been around since the 1970s (RIR 2020:22). The Swedish National Audit Office (RIR 2020:22) investigated 13 Swedish agencies that used ADM in 2019 and in total these agencies made 148 million decisions aimed at citizens and companies. Of these decisions 137 million decisions were part of ADM processes, and 121 million decisions were fully automated. The agencies with highest degree of ADM are the Swedish Social Insurance Agency, the Swedish Transport Agency, and the Swedish Tax Agency. The Swedish Social Insurance Agency has for example fully automated applications and decisions about parental benefits since 2014 (RIR 2020:22). The use of ADM for decisions towards citizens in Swedish municipalities is however a more recent development. One example is Trelleborg municipality, where computers take automated decisions about income support for citizens (Ranerup & Henriksen, 2019; Trelleborgs Allehanda, 2017). The software works similarly to public servants: it logs on to the system and executes specific clicks, configurations and checks before making decisions. In this specific case, automation means rule-based decisions, where a set of predefined rules determine the decisions.
Our items about attitudes towards ADM were included in the 2018 national survey that the SOM Institute at the University of Gothenburg has conducted annually since 1986. For this survey, the institute used a mixed-mode version of mailed paper-based surveys and web surveys to collect the data. We also use materials from this survey to measure background and control variables: gender, age, level of education, citizenship, place of living, sector of employment, political interest, and interpersonal trust (see Table 1). Previous research concludes that these variables affect the degree of political awareness (Lupia, 2016).
To measure awareness of ADM in the public-sector, we used an item that asked the respondents if they had previous knowledge of ADM in the public-sector. This item captures cognitive awareness of the existence of ADM. Given the explorative purpose of the study, and the fact that citizens’ awareness of the use of ADM in public-sector is largely unknown, we have chosen an inclusive question about ADM. Thus, it can include decision-support systems that range from fully automated systems without any human involvement to different degrees of human involvement in the decision-making process. We used four items to measure beliefs about ADM, which indicate how persons expected decision-making in the public-sector would change if computers made the decisions instead of public servants. More concretely, they focus on the extent to which ADM changes the following four aspects of public-sector decision-making: being legally secure,11 impartiality, considerations about the situations of the persons and transparency (see Table A1 in Appendix A). The items were constructed with inspirations from previous studies about attitudes towards decision-making (Abdelzadeh et al., 2015; Grimes, 2005; Hernández, 2016; Smith, 2018). Furthermore, the items were reviewed by experts from the survey program before they were accepted to be included in the survey. In this process, the items were compared with previous surveys and tests of item constructions.
The four selected items are only a limited part of the qualities that research of decision-making has focused on (Hernández, 2016). The selected qualities refer not only to basic aspects of the relationship between the public-sector and citizens in Sweden, but also to basic qualities of public decision-making that the Administrative Procedure Act in Sweden (SFS 2017:900) requires. The law expects the decisions to be legally secure and impartial, to consider personal situations when relevant and to be transparent. Research also claims that these qualities are components of ‘good government’, which has positive effects on different aspects of society (Rothstein, 2011; Rothstein & Teorell, 2008). One of these aspects concerns the legitimacy of the public-sector among citizens. For example, studies have shown strong correlations between impartiality and trust of decision-making within public institutions (Abdelzadeh et al., 2015; Grimes, 2005; Rothstein, 2011). Furthermore, a non-transparent and impenetrable decision-making process tend to reduce citizen trust for the process and the outcome (Pardo & Burke, 2008). Smith et al. (2010) also voice this concern, arguing that transparency is important for securing public-sector accountability in times of ADM. Therefore, we see transparency at the core of ADM in the public-sector. In sum, the four items represent core aspects of quality in public decision-making, which the research indicates are expected to affect the relationship between the public-sector and the citizens, in terms of legitimacy and trust.
To analyse our data, we used three statistical methods. First, we used correlation methods (tau-b and tau-c) to examine bivariate correlations between the items of awareness and background variables (see Table 1) as well as between the survey items (see Tables 3 and 4). The use of these methods is motivated by the scale structure of the items. Both tau-b and tau-c range between
5.Results
5.1Awareness of ADM
The results indicate that only a minority of the respondents were aware of ADM. As Table 1 presents, 80 per cent of the respondents answered that they did not know that computers make decisions within the public-sector. Only 20 per cent answered that they knew about ADM in the public-sector. Below we discuss to what extent the awareness of ADM varied between groups, which previous studies about attitudes toward political institutions (e.g. Ceka & Magalhães, 2016; Lupia, 2016; Zaller, 1990) and perceptions toward ADM have identified to be relevant (e.g. Araujo et al., 2020; Helberger et al., 2020).
Table 1
Awareness of ADM in public-sector (per cent, correlations)
Yes | No | Total | Correlation (significance) |
| |
---|---|---|---|---|---|
All | 20 | 80 | 100 | 1,746 | |
Gender | Tau-b: 0.056 | ||||
Female | 18 | 82 | 100 | (0.019) | 851 |
Men | 22 | 78 | 100 | 892 | |
Age group (years) | Tau-c: -0.069 | ||||
16–19 | 20 | 80 | 100 | (0.002) | 74 |
20–29 | 24 | 76 | 100 | 214 | |
30–39 | 28 | 72 | 100 | 245 | |
40–49 | 16 | 84 | 100 | 256 | |
50–59 | 20 | 80 | 100 | 275 | |
60–69 | 19 | 81 | 100 | 317 | |
70–70 | 16 | 84 | 100 | 289 | |
80–85 | 15 | 85 | 100 | 76 | |
Level of education | Tau-c: 0.102 | ||||
Low | 14 | 86 | 100 | (0.000) | 257 |
Middle-low | 16 | 84 | 100 | 519 | |
Middle-high | 20 | 80 | 100 | 402 | |
High | 25 | 75 | 100 | 503 | |
Citizenship | Tau-c: 0.039 | ||||
Swedish citizens | 19 | 81 | 100 | (0.002) | 1,583 |
Double citizenships | 28 | 72 | 100 | 72 | |
Non-Swedish citizens | 35 | 65 | 100 | 71 | |
Place of living | Tau-c | ||||
Rural area | 13 | 87 | 100 | (0.000) | 257 |
Smaller urban area | 18 | 82 | 100 | 274 | |
Town or bigger urban area | 20 | 80 | 100 | 886 | |
Metropolitan | 26 | 74 | 100 | 284 | |
Sector of employment | Tau-c | ||||
Non-profit organization/foundation | (12) | (88) | (100) | (0.000) | 41 |
Private | 18 | 82 | 100 | 900 | |
Regional government | 14 | 86 | 100 | 116 | |
Local government | 24 | 76 | 100 | 350 | |
Central government | 26 | 74 | 100 | 176 | |
Political interest | Tau-c | ||||
Not at all interested | 20 | 80 | 100 | (0.000) | 126 |
Not particularly interested | 12 | 88 | 100 | 462 | |
Quite a lot interested | 20 | 80 | 100 | 807 | |
Very interested | 30 | 70 | 100 | 323 | |
Interpersonal trust (scale 0–10) | Tau-c | ||||
Low (0–2) | 77 | 23 | 100 | (0.128) | 114 |
Middle (3–7) | 82 | 18 | 100 | 1,007 | |
High (8–10) | 77 | 23 | 100 | 598 |
As Table 1 shows, we identified significant but very weak correlations for how awareness differed between the groups. First, we found men being somewhat more aware of ADM than women. Second, the differences between age groups showed a negative correlation between age and awareness. Younger (20–29 years) and younger middle-age (30–39 years) respondents had a higher awareness of ADM in the public-sector than older groups. Third, education level had a positive correlation with awareness. Persons with a higher education level seem to be more aware than persons with less education; however, the difference is moderate at 11 per cent Fourth, persons with citizenship other than Swedish had a greater awareness of ADM than persons of Swedish citizenship. One possible explanation for this difference is that persons with other citizenship may have had contact with authorities that use ADM to a higher degree than Swedish citizens have had.
Fifth, awareness also varies between places of residence. Individuals living in rural areas have less awareness than persons living in one of the three Metropoles in Sweden (Stockholm, Gothenburg and Malmö). Only 13 per cent of the respondents living in rural areas stated that they had heard about ADM, while the share among persons in the Metropoles was 26 per cent. Sixth, there is a correlation between employment sector and awareness. Individuals employed within local or national government sectors seem somewhat more aware of ADM than persons employed in a regional government sector or the private sector. This difference may indicate that local and national government sectors use ADM to a greater extent than regional government, and persons within the private sector tend to have less contact through their employment with ADM in the public-sector.
Seventh, persons with a higher level of political interest showed greater awareness of ADM than persons with a lower level. The lowest level of awareness appeared among individuals with the next lowest level of political interest. In this group, the awareness of ADM is 18 per cent lower than the group with the highest level of political interest. This result indicates that political interest is positively correlated to the awareness of ADM. Finally, the analysis indicates no correlation between interpersonal trust and awareness of ADM. To summarise, the differences we found between groups according to age, citizenship, place of living, political interest and sector of employment can be considered minor to modest.
5.2Beliefs about ADM
The respondents were asked to answer four statements that describe how ADM affects decision-making in the public-sector, compared to human decision-making (Table 2). The first statement claimed that decisions by computers produce more legally secure decisions than human decision-makers. A minority of the respondents (40 per cent) agreed to some extent or entirely with this statement, while 60 per cent rejected the statement. The second statement was that decisions by computers give more impartial decisions than decisions by public servants. A majority (69 per cent) answered that they agree with this statement, whereas 31 per cent disagree with it. A majority (77 per cent) also agreed with our third statement that decisions by computers take people’s situations into account to a lesser extent. A share of respondents almost equal to the third statement (71 per cent) supported the fourth statement, which claimed that when computers make decisions, the decision-making becomes less transparent than when public servants make the decisions.
Table 2
Awareness of ADM in public-sector (per cent, balance)
Do not agree at all | Hardly agree | Agree to some extent | Agree total | Sum |
| Balance | |
---|---|---|---|---|---|---|---|
…more legally secure decisions | 20 | 40 | 35 | 5 | 100 | 1,588 | –20 |
…more impartial decisions | 12 | 19 | 48 | 21 | 100 | 1,585 | |
…less consideration of people’s situation | 9 | 14 | 32 | 45 | 100 | 1,630 | |
…less transparent decisions | 10 | 19 | 37 | 34 | 100 | 1,604 |
Note: Balance is the share with positive answers (agree total
When we analysed the correlations between the answers to the statements, we found two patterns (Table 3). The first pattern is a strong correlation between the answers to the statement that decisions by computers would give more legally secure decisions and the statement that decisions by computers would result in more impartial decisions. Respondents that agreed with one of the statements also tended to agree with the other. The second pattern is also a strong positive correlation, this time between the answers to the statements that decisions by computers would make decisions that consider people’s situations and that would make the decision-making less transparent. These results indicate that the beliefs about ADM have two dimensions. One dimension concerns the qualities of the decisions (legally secure and impartial), whereas the second dimension concerns the relationship between the decision-making and the citizens (considerations of life situations and transparency).22
Table 3
Correlation between beliefs about ADM in public-sector (tau-b)
Legally secure decisions | Impartial decisions | Less consideration | |
---|---|---|---|
Impartial decisions | 0.52 (0.000) | ||
Less consideration | 0.07 (0.000) | 0.28 (0.000) | |
Less transparency | –0.02 (0.400) | 0.16 (0.000) | 0.59 (0.000) |
5.3Awareness of and beliefs about ADM
Individuals who are aware of ADM might have some cognitions that individuals without awareness lack (Fishbein & Ajzen, 2010; Lupia, 2016; Zaller, 1990). Based on this assumption, the expectation is that beliefs are different between individuals with and individuals without awareness, making it interesting to compare the two groups.
However, the correlation between awareness of and beliefs about ADM indicates mixed empirical results (Table 4). Correlations exist between awareness of ADM and two aspects of beliefs about ADM. First, there is a correlation between awareness and the belief that decisions by computers would make the decision more legally secure. A majority of the individuals with awareness agreed with this statement, while a majority of the individuals without awareness disagreed with the statement. Second, we found a positive correlation between awareness and the belief that decisions by computers would give more impartial decisions. However, a majority in both groups agreed with the statement. The share that agreed is higher in the group with awareness than the group without awareness. Third, concerning the other two aspects – considering life situations in decision-making and making decision-making less transparent – no significant differences appear between the groups. Both groups agreed with the statements to the same extent, even if the shares concerning the statement on transparent decision-making are lower than for the statement on considering life situations. To summarise, the correlation analyses indicate that higher awareness strengthens the beliefs that ADM would result in more legally secure and impartial decisions. However, there are no connections between awareness and the beliefs about taking people’s situation into consideration and decision transparency.
Table 4
Awareness and beliefs about ADM in public-sector (per cent, balance, correlations)
Do not agree not at all | Hardly agree | Agree to some extent | Agree total | Sum |
| Balance | Tau-c (sign.) | |
---|---|---|---|---|---|---|---|---|
…more legally secure decisions | 0.196 | |||||||
With awareness | 9 | 31 | 50 | 10 | 100 | 1,579 | (0.000) | |
Without awareness | 22 | 43 | 31 | 4 | 100 | 1,579 | –30 | |
…more impartial decisions | 0.132 | |||||||
With awareness | 4 | 16 | 49 | 31 | 100 | 1,576 | (0.000) | |
Without awareness | 13 | 20 | 47 | 19 | 100 | 1 576 | ||
…less consideration of the people’s situation | 0.015 | |||||||
With awareness | 4 | 18 | 37 | 41 | 100 | 1,622 | (0.474) | |
Without awareness | 10 | 13 | 30 | 46 | 100 | 1,622 | ||
…less transparent decisions | 0.038 | |||||||
With awareness | 8 | 23 | 40 | 29 | 100 | 1,596 | (0.076) | |
Without awareness | 11 | 16 | 37 | 36 | 100 | 1,596 |
As a second step, we analysed the connection between awareness and beliefs about ADM with multiple regression models (Table 5) that control for the background variables (see Table 1). The dependent variables in these analyses are dummy variables with one category that includes individuals that agreed with the statement (coded as 1) and another category that refers to individuals who disagreed with the statement (coded as 0). As the dependent variables are dummy variables (with two categories), we used logistic regression models to analyse the effects of awareness on beliefs about ADM. The models include eight control variables: age, citizenship, education, employment sector, gender, interpersonal trust, place of living, and political interest.
Table 5
Logistic regression analyses about awareness and beliefs
Dependent variables | Legally secure decisions | Impartial decisions | Less consideration | Less transparency |
---|---|---|---|---|
Exp (B) (significance) | Exp (B) (significance) | Exp (B) (significance) | Exp (B) (significance) | |
Awareness about ADM in publicsector | 2.507 (0.000) | 1.693 (0.002) | 0.899 (0.530) | 0.847 (0.269) |
Control variables included | Yes | Yes | Yes | Yes |
Nagelkerke R | 11.5 | 14.7 | 6.9 | 2.6 |
Number of respondents | 1,386 | 1,385 | 1,423 | 1,405 |
The outcomes of the regression analyses show the same patterns between awareness of and beliefs about ADM as the previous analyses. Individuals who stated they have an awareness of ADM in the public-sector tended to a higher degree agree with the statement that the decision-making by computers leads to more legally secure and impartial decisions than when public servants make the decisions. According to the results, the probability that an individual with awareness would agree with the statement about legally secure decisions is 150 per cent higher than the probability of an individual without awareness. When it comes to impartial decisions, the probability of an individual with awareness agreeing with the statement is 69 per cent higher than the probability of a person without awareness. However, there is no difference between individuals with or without awareness in their beliefs regarding consideration of life situations or transparency. If we relate these findings to the results in Table 3, we conclude that awareness has a connection to beliefs about the qualities of the decisions (legally secure and impartial) but lacks correlation with beliefs about the relationships between the decision-making and the citizens (considerations of personal life situations and transparency).
6.Discussion
6.1Implications for research
Our study complements and extends the findings of the few previous studies that have explored citizens’ attitudes to ADM in public administration (Araujo et al., 2020; Helberger et al., 2020; Miller & Keiser, 2020). We do that by developing four hypotheses, where the intention is to stimulate collective and incremental theory building about citizens’ awareness of and beliefs about ADM in public-sector.
Our results show that very few (only 20 per cent) of those who answered our survey were aware that computers make public-sector decisions in Sweden. Previous research has not explicitly inquired awareness, although Helberger et al. (2020) indirectly capture it when studying fairness. They found that 30 per cent of their respondents were unclear or did not respond who is more just – computers or humans. Thus, our finding is important for electronic government research, and especially research on ADM. We need to consider how this lack of awareness may influence the view of public administration and the relation between the citizen and the public organisation, as well as how it may impact development and implementation of ADM in public organisations.
We explored to what extent citizen awareness of ADM varies among different groups (see Table 1) and found that the identified differences were minor to modest and not strongly associated with any particular group. For example, we conclude that education level is very weakly associated with differences in awareness of ADM. Still this is interesting considering previous findings, where general knowledge (education) has been associated with increased expectations about usefulness of ADM (Araujo et al., 2020). Education has also been positively associated with the likelihood of ADM “being considered fairer than humans” (Helberger et al., 2020). In addition, we conclude that employment sector does not seem to be strongly associated with differences in awareness of ADM. Individuals who work within local and central governments only seemed to show a bit more awareness of ADM than individuals working in regional governments or in the private sector. At same time, Araujo et al. (2020) has associated domain-specific knowledge, i.e., knowledge about algorithms and artificial intelligence, with positive expectations about usefulness and fairness of ADM. Thus, employment sector might not be related to levels of such domain-specific knowledge about ADM.
Our study investigated citizens’ beliefs about ADM in relation to the public values of being legally secure, being impartial, taking people’s situations into consideration and transparency in decision-making. With regards to ADM resulting in decisions being more legally secure, our findings are complex. A majority of those who answered our survey believed that ADM does not support more legally secure decisions. Thus, these results seem to be at odds with Helberger et al. (2020), who found that citizens perceive ADM as fairer decision-makers than humans. They argue that citizens associate an “alleged immunity of algorithmic systems to manipulation”, i.e., legally secure decisions, with fairness of ADM. At the same time, our findings show that citizens being aware of ADM believe that this type of technology contribute to decisions being more legally secure. This led us to our first hypothesis:
H1. Citizens’ awareness of ADM in the public-sector has a positive effect on their belief that the use of ADM makes decisions more legally secure than public servants making the decisions.
We can conclude that citizens believe that ADM leads to more impartial decisions. This is interesting, as impartiality is an important quality for decision-making in public administration (Rothstein & Teorell, 2008), and one core value for electronic government (Bannister & Connolly, 2014). Our results support this view and corroborates earlier findings by Helberger et al. (2020) and Araujo et al. (2020) about citizens perceiving ADM as being on par or fairer than human decision-making. This is also in line with the findings about fairness by Miller and Keiser (2020). In addition, previous research highlights the changing role of the street-level bureaucrat and the importance of citizen centricity in ADM (Ranerup & Henriksen, 2019). At the same time our findings show that beliefs about impartiality are higher among citizens with awareness of ADM. Also, in light of our results showing that citizens believe that a computer making a decision considers the individual to a lesser extent than a human making a decision, we formulate our second hypothesis:
H2. Citizens’ awareness of ADM in the public-sector has a positive effect on their belief that the use of ADM results in more impartial decisions than when public servants make the decisions.
Another important aspect of ADM is transparency (see e.g. M. L. Smith et al., 2010), being seen as a core factor in accountability and, thus, legitimacy. Our results indicate that citizens believe that computers making decisions will do so less transparently. However, we did not find this belief being connected to awareness. This might not be surprising given that Kuziemski and Misuraca (2020) have shown, from the government administration perspective, that transparency is a concern in several ADM initiatives. Thus, the question of transparency and its meaning from a citizen perspective is important for future research to further investigate, in relation to public-sector ADM. This leads to our third hypothesis.
H3. Citizens’ awareness of ADM in the public-sector has no effect on their belief that the use of ADM makes decisions less transparent than when public servants make the decisions.
One value of importance for the relationship between citizens and the public-sector is the need for the public-sector to consider people’s situation instead of relying too much on abstract principles (Jørgensen & Bozeman, 2007). This may be problematic, as a high degree of automation generally requires formalisation and standardisation of the decision-making process (Smith et al., 2010). Our findings show that citizens believe ADM takes people’s situation into account to a lesser extent compared to public servants. Thus, we confirm earlier findings by Helberger et al. (2020), where citizens found the “infallible execution of rules” of ADM concerning. At the same time, we did not find this belief being connected to awareness. We therefore suggest the fourth hypothesis:
H4. Citizens’ awareness of ADM in the public-sector has no effect on their beliefs that the use of ADM takes the situations of people into less account than when public servants make the decisions.
6.2Implications for practice
Our explorative study should be viewed in the context that ADM is a technology that policy-makers are pushing. For example, the European Strategy for Data (European Commission, 2020a) and White Paper on Artificial Intelligence (European Commission, 2020b) lay the foundation “for a society empowered by data” that includes ADM. The compilations presented in the Automating Society Reports (Chiusi et al., 2020; Spielkamp, 2019) show an increasing number of ADM initiatives in Europe – at different stages of implementation.
Our hypotheses can therefore be useful also for practitioners, such as policy-makers in the public-sector. These hypotheses highlight major aspects that should be considered when, for example, Swedish government agencies continue their plans to further develop and implement ADM (RIR 2020:22). When policy-makers decide to use ADM in public administration, they must consider that at least in Sweden, only 20 per cent of those who answered our survey were aware of the use of this technology. Consequently, policy-makers have an important task ahead of them, addressing citizens’ awareness of ADM. At the same time, our hypotheses suggest that citizens’ awareness plays different roles with regards to citizens’ beliefs about ADM and its qualities. Higher awareness of ADM seems to strengthen the beliefs about legally secure and impartial decisions, while there seems to be no such connection to beliefs about transparency and taking people’s situation into consideration. Thus, these connections may play a role in what kind of results one can be expected from raised awareness among citizens about this technology. Given our findings it is more reasonable to expect changes in citizens’ belief about ADM in public-sector being legally secure and impartial than being transparent and taking people’s situation into consideration.
Furthermore, based on our results it is not possible to pinpoint specific target groups for such awareness-raising campaigns; the awareness is equally low across the board (see Table 1). Thus, policy-makers must inform all citizens about the use of ADM in the public-sector to raise awareness in general and inform about ongoing ADM projects that target specific groups of citizens in particular. It is also important that public-sector organisations provide clear information on how decisions are made when communicating them to citizens. Having said that, we do not argue that raising citizens’ awareness of ADM is something easily achieved. As Lupia (2016) discusses, it is not easy to engage people in how government works.
Our findings show that citizens believe the use of ADM results in less transparent decisions and that people’s situation is taken into account to a lesser degree, than when public servants make these decisions. In addition, these beliefs do not seem to be connected to awareness. This suggests that the use of ADM fits certain types of decisions in public administration better than other types. Decisions based on a simple framework, with few and clear rules, are easier to explain to citizens and are therefore suitable candidates for ADM. The case that few and clear rules are easier to explain is probably true also when public servants make the decisions. However, public servants have better possibilities to explain complex rules in their interactions with citizens, compared to situations of citizen interaction with public administration via IT (at least with today’s technology level). Also, decisions with little or no need to consider the situations of people are probably suitable candidates for ADM. From a citizens’ perspective, this mean that government officials should carefully consider which public services they choose for ADM implementation. Having said that, these types of decisions might not be the ones that are most suitable for automated decisions from a governmental and efficiency perspective (Ranerup & Henriksen, 2019). We do not argue that the citizens’ perspective should drive the selection of which decisions to implement using this type of technology. However, it shows the importance of policy-makers being aware of and explicit about the perspective that drives the selection and being aware that there might be conflicts between these perspectives.
Citizens’ beliefs about ADM resulting in decisions being less transparent than when public servants make them might suggest that the next technical leap in ADM – artificial intelligence – introduces new challenges regarding transparency. The basis of today’s implementation of ADM is often a predetermined set of algorithms (see e.g. Ranerup & Henriksen, 2019; Wihlborg et al., 2016). Artificial intelligence introduces possibilities for more dynamic decision-making structures that could evolve over time (Bannister & Connolly, 2020), likely to make such ADM even less transparent than decision-making with a predetermined set of algorithms. This probably suggests that policy-makers need to consider how to make decisions based on artificial intelligence transparent to the citizens before they decide to introduce such technology into ADM.
Finally, citizens’ low awareness of the use of ADM in the public-sector also raises questions about their understanding of what is required for ADM to work, i.e., a topic that is far more complex than having awareness of the phenomenon. When policy-makers frame ADM as a technology it is very much a simplification, with a risk of overlooking the type of data needed, the design of the decisions models implemented and who is deciding about these designs. For example, there might be important privacy concerns with integrating data, and potential bias when building and deciding on the models or using data to train them (Chiusi et al., 2020; Spielkamp, 2019). Thus, ADM is not without potential dark sides as exemplified by Eubanks (2017), and a limited understanding among citizens about the ongoing transition in public-sector risks the debate on how, when, and for what purposes citizens want public-sector to use ADM.
6.3Limitations and future research
This study has limitations that open directions for future research. First, the Swedish context with high level of citizen trust and long tradition of ADM may affect beliefs about ADM. In order to increase generalizability, future studies could use comparative design to test the hypotheses in different contexts and examine the significance of context for the beliefs. Second, our inclusive measure of awareness of ADM does not differentiate between different degrees of automation in the ADM nor between automation of different types of public-sector decisions, which opens for broad interpretations among the respondents. As discussed in the Introduction, existing research has shown ADM spans a broad set of solutions. Thus, future studies could investigate citizens’ awareness using more detailed descriptions of ADM and citizens’ views on different types of decisions in relation to the degree of automation. Such studies could also examine the difference (if any) between citizens’ attitudes to deterministic ADM and non-deterministic ADM using artificial intelligence.
Third, the design of the items used limits the study to analysing four beliefs about ADM. Citizens may have other beliefs than the four we have included in this survey. This question about relevant beliefs among citizens calls for future research. Fourth, our study does not analyse the significance of the beliefs. For example, issues of what beliefs about ADM affect how citizens evaluate the legitimacy of the decisions made by computers are left to future studies. Finally, we focus on the relationship between awareness and beliefs, but to explain why citizens have different beliefs requires more elaborate models and analyses. Thus, an essential topic for future studies is explaining the variations in awareness and beliefs.
7.Conclusions
The aim of this study is to provide empirical insights into citizen awareness and beliefs concerning automated decision-making (ADM) in public-sector services. Based on our empirical findings in relation to previous research, we suggest four hypotheses explicating our results and to stimulate collective and incremental theory building on this topic. Furthermore, based on the empirical analyses, we can draw three conclusions that answer our research questions. First, the use of ADM in public-sector services is known among a minority of the citizens. There are differences in awareness among citizen groups about ADM in the public-sector, but the magnitude of the differences is quite modest. Second, when computers make public-sector decisions instead of public servants, the citizens expect both the quality of decisions and the relationship between citizens and decision-making to change. According to the citizens, decisions become less legally secure and more impartial when computers make them. Citizens also expect decisions by computers to take personal circumstances into account to a lesser degree and become less transparent. Third, citizens with awareness expect decisions made by computers to become more legally secure and impartial. However, there are no correlations between awareness and the other two beliefs that we analysed (consideration of the people’s situation and transparent decision). These conclusions can form a starting point for understanding the citizen view when developing ADM in the public-sector, informing electronic-government initiatives.
Notes
1 Legally secure refers, in this context, to the individuals’ expectations that the legal principles are applied predictably and effectively given the information about the case.
2 The two dimensions are also the outcome of an exploratory factor analysis (EFA) with the four items (see Table A2). The analysis is done with Varimax as rotation method and Kaiser’s criterion with eigenvalues as extraction method. However, we interpret the result from factor analysis with caution, as the KMO-coefficient is not strong (under 0.65), and with awareness of the ordinal structure of the items.
References
[1] | Abdelzadeh, A., Zetterberg, P., & Ekman, J. ((2015) ). Procedural fairness and political trust among young people: Evidence from a panel study of Swedish school students. Acta Politica, 50: (3), 253-278. |
[2] | Araujo, T., Helberger, N. Kruikemeier, S., & de Vreese, C. ((2020) ). In AI we trust? Perceptions about automated decision-making by artificial intelligence. AI & Society, 35: (3), 611-623. |
[3] | Banister, F., & Grönlund, Å. ((2017) ). Information Technology and Government Research: A Brief History. Paper presented at the 50th Hawaii International Conference on System Sciences (HICSS 2017), Hawaii, USA. |
[4] | Bannister, F., & Connolly, R. ((2014) ). ICT, public values and transformative government: A framework and programme for research. Government Information Quarterly, 31: (1), 119-128. |
[5] | Bannister, F., & Connolly, R. ((2020) ). Administration by algorithm: A risk management framework. Information Polity, 25: (4), 471-490. |
[6] | Bruhn, A. ((2015) ). Changing Occupational Roles in Audit Society – The Case of Swedish Student Aid Officials. Nordic Journal of Working Life Studies, 5: (1), 31-50. |
[7] | Ceka, B., & Magalhães, P. C. ((2016) ). How People Understand Demcracy: A Social Domincance Approach. In M. Ferrín & H. Kriesi (Eds.), How European View and Evaluate Democracy. Oxford: Oxford University Press, pp. 90-110. |
[8] | Chiusi, F., Fischer, S., Kayser-Bril, N., & Spielkamp, M. ((2020) ). Automating Society Report 2020. Retrieved from Berlin, Germany. |
[9] | Coglianese, C., & Lehr, D. ((2017) ). Regulating by Robot: Administrative Decision Making in the Machine-Learning Era. The Georgetown Law Journal, 105: , 1147-1223. |
[10] | Converse, P. E. ((1964) ). The nature of belief systems in mass publics. In D. E. Apter (Ed.), Ideology and its discontent New York: Free Press of Glencoe, pp. 206-261 |
[11] | Eubanks, V. ((2017) ). Automating inequality: how high-tech tools profile, police, and punish the poor. New York, NY: St. Martin’s Press. |
[12] | European Commission. ((2020) a). A European data strategy. Retrieved from Brussels. |
[13] | European Commission. ((2020) b). White paper: On Artificial Intelligence – A European approach to excellence and Trust. Retrieved from Brussels: |
[14] | Fishbein, M., & Ajzen, I. ((1975) ). Belief, attitude, intention, and behavior: An introduction to theory and research. Reading: Addison-Wesley. |
[15] | Fishbein, M., & Ajzen, I. ((2010) ). Predicting and Changing Behavior: The Reasoned Action Approach. New York: Psychology Press. |
[16] | Grimes, M. ((2005) ). Democracy’s Infrastructure: The Role of Procedural Fairness in Fostering Consent. Göteborg University, Göteborg. (Göteborg Studies in Politics nr 97). |
[17] | Heider, F. ((1958) ). The Psychology of International Relations. New York: Wiley. |
[18] | Helberger, N., Araujo, T., & de Vreese, C. ((2020) ). Who is the fairest of them all? Public attitudes and expectations regarding automated decision-making. Computer Law & Security Review, 39: , 105456. |
[19] | Henriksen, H. Z. ((2018) ). One step forward and two steps back: e-Government policies in practice. In J. R. Gil-García, T. A. Pardo, & L. F. Luna-Reyes (Eds.), Policy analytics, modelling, and informatics. Innovative Tools for Solving Complex Social Problems. Cham: Springer, pp. 79-97. |
[20] | Hernández, E. ((2016) ). Europeans’ Views of Democracy: The Core Elements of Democracy. In M. Ferrín & H. Kriesi (Eds.), How European View and Evaluate Democracy. Oxford: Oxford University Press. |
[21] | Jørgensen, T. B., & Bozeman, B. ((2007) ). Public Values: An Inventory. Administration & Society, 39: (3), 354-381. |
[22] | Kuziemski, M., & Misuraca, G. ((2020) ). AI governance in the public sector: Three tales from the frontiers of automated decision-making in democratic settings. Telecommunications Policy, 44: (6), 101976. |
[23] | Le Sueur, A. ((2016) ). Robot Government: Automated Decision-Making and its Implications for Parliament. In A. Horne & A. Le Sueur (Eds.), Parliament: Legislation and Accountability. Oxford and Portland, Oregon: Hart Publishing, pp. Chapter 9. |
[24] | Lupia, A. ((2016) ). Uninformed: Why People Know so Little about Politics and What we can do about it. Oxford: Oxford University Press. |
[25] | McGraw, K. M. ((2001) ). Political Accounts and Attribution Processes. In J. H. Kuklinski (Ed.), Citizens and Politics: Perspectives from Political Psychology. Cambridge: Cambridge University Press, pp. 160-197. |
[26] | Miller, S. M., & Keiser, L. R. ((2020) ). Representative Bureaucracy and Attitudes Toward Automated Decision Making. Journal of Public Administration Research and Theory, 1-16. |
[27] | Motzfeldt, H. M., & Næsborg-Andersen, A. ((2017) ). Regulating E-government in Denmark. In J. Vieira Borges & J. C. Dias Rouco (Eds.), Proceedings of the 17th European Conference on Digital Government. Reading, UK: Academic Conferences and Publishing International, pp. 104-109. |
[28] | Pardo, T., & Burke, B. ((2008) ). Government Worth Having: A Briefing on Interoperability for Government Leaders. Retrieved from Albany, NY. |
[29] | Ranerup, A., & Henriksen, H. Z. ((2019) ). Value positions viewed through the lens of automated decision-making: The case of social services. Government Information Quarterly. |
[30] | RIR 2020:22. (2020). Automatiserat beslutsfattande i statsförvaltningen – effektivt, men kontroll och uppföljning brister [Automated decision-making in public administration – effective and efficient, but inadequate control and follow-up]. Retrieved from Stockholm. |
[31] | Rothstein, B. ((2011) ). The quality of government corruption, social trust, and inequality in international perspective. Chicago: University of Chicago Press. |
[32] | Rothstein, B., & Teorell, J. ((2008) ). What is quality of government? A theory of impartial government institutions. Governance, 21: (2), 165-190. |
[33] | Schartum, D. W. ((2016) ). Law and algorithms in the public domain. Nordic Journal of Applied Ethics, 1: , 15-26. |
[34] | Förvaltningslagen [Swedish Public Administration Act], (2017). |
[35] | Smith, A. ((2018) ). Public Attitudes toward Computer Algorithm. Retrieved from Washington. |
[36] | Smith, M. L., Noorman, M. E., & Martin, A. K. ((2010) ). Automating the public sector and organizing accountabilities. Communications of the Association for Information Systems, 26: (1), 1-16. |
[37] | Snellen, I. ((2002) ). Electronic governance: implications for citizens, politicians and public servants. International Review of Administrative Sciences, 68: (2), 183-198. |
[38] | Spielkamp, M. ((2019) ). Automating Society Taking Stock of Automated Decision-Making in the EU. Retrieved from Berlin, Germany. |
[39] | Suksi, M. ((2020) ). Administrative due process when using automated decision-making in public administration: some notes from a Finnish perspective. Artificial Intelligence and Law, 1-24. |
[40] | Tourangeau, R., Rips, L. J., & Rasinski, K. ((2000) ). The psychology of survey response. Cambridge: Cambridge University Press. |
[41] | Trelleborgs Allehanda. ((2017) ). Trelleborg’s robot recieved prize in Stockholm [In Swedish: Trelleborgs robot prisad i Stockholm]. Trelleborgs Allehanda. |
[42] | Weissenbilder, M. ((2019) ). Den nationella SOM-undersökningen 2018. In U. Andersson, B. Rönnerstrand, P. Öhberg, & A. Bergström (Eds.), Storm och Stiltje. Göteborg: Göteborgs Universitet. |
[43] | Wihlborg, E., Larsson, H., & Hedström, K. ((2016) ). “The Computer Says No!” – A Case Study on Automated Decision-making in Public Authorities. Paper presented at the 49th Hawaii International Conference on System Sciences (HICCS). |
[44] | Zaller, J. R. ((1990) ). The Nature and Origins of Mass Opinion. Cambridge University Press: Cambridge. |
Appendices
Authors biographies
Thomas Denk is professor of political science at Örebro University. He received his PhD degree in political science in 1999. His research focuses on political culture, socialization, democratization, diffusion, interdependence, state formation and comparative methodology. He has published several research monographs and articles in international journals. He is currently conducting research about complex interdependence in historical and comparative perspectives.
Karin Hedström is professor in informatics at Örebro University, Sweden. Her research interest is about the role of values for development and use of information systems. Hedström’s research is mainly carried out within the areas of information security and eGovernment. Her research has been published in international journals such as Government Information Quarterly, Strategic Journal of Information Systems, Transforming Government: People, Process and Policy, Scandinavian Journal of Information Systems, and Information Management and Computer Security, among others. Hedström is currently the Chair of IFIP TC 11 Working Group 1 – Information Security Management.
Fredrik Karlsson is a professor of informatics at Örebro University, Sweden. He received his PhD degree in information systems development at Linköping University in 2005. His areas of specialization include electronic government, information security management, method engineering, use of systems development methods and how values can be used to understand different sets of behavior in complex situations. He often conducts research in collaboration with businesses and public organizations. He has published in conference proceedings of CAISE, ISD, ECIS, IFIP SEC among others. His research has also appeared in a variety of information systems journals such as European Journal of Information Systems, Journal of the Association for Information Systems, Strategic Journal of Information Systems and Government Information Quarterly.
Appendix A
Table A1
Survey items about awareness of and beliefs about automated decision-making in public-sector
1. | Municipalities and authorities make more and more decisions that affect citizens by computers rather than public officials. Do you know since before that some decisions in the public-sector are made by computers? | ||||
---|---|---|---|---|---|
2. | To what extent do you agree with the following statements? | ||||
When decisions are made by computers instead of officials, | Do not | Hardly | Agree to | Agree | |
this leads to | agree at all | agree | some extent | total | |
…more legally secure decision |
|
|
|
| |
…more impartial decisions |
|
|
|
| |
…less consideration for people’s situation |
|
|
|
| |
…less transparency in the decisions |
|
|
|
|
Table A2
Factor analyses
Variables |
| Factor 2 Quality of decisions | Extraction | |||
---|---|---|---|---|---|---|
Legally secure decisions | 0.916 | 0.840 | ||||
Impartial decisions | 0.287 | 0.847 | 0.799 | |||
Less consideration | 0.885 | 0.208 | 0.826 | |||
Less transparency | 0.917 | 0.016 | 0.841 | |||
Explained variance (%) | 42.7% | 40.0% | 83.7% | |||
KMO-coefficient (significance) | 0.559 (0.000) |
Exploratory factor analysis with Varimax as the rotation method and principal components analysis with Kaiser criterion (Eigenvalue >1) as the extraction method.