Introduction to special issue algorithmic transparency in government: Towards a multi-level perspective
Abstract
The editorial sets the stage for the special issue on algorithmic transparency in government. The papers in the issue bring together transparency challenges experienced across different levels of government, including macro-, meso-, and micro-levels. This highlights that transparency issues transcend different levels of government – from European regulation to individual public bureaucrats. With a special focus on these links, the editorial sketches a future research agenda for transparency-related challenges. Highlighting these linkages is a first step towards seeing the bigger picture of why transparency mechanisms are put in place in some scenarios and not in others. Finally, this introduction present an agenda for future research, which opens the door to comparative analyses for future research and new insights for policymakers.
1.Introduction
Previous research shows that machine-learning technologies and the algorithms that power them hold huge potential to make government services fairer and more effective, and ‘freeing’ decision-making from human subjectivity (Margetts & Dorobantu, 2019; Pencheva et al., 2018). Algorithms today are used in many public service contexts, from health care to criminal justice. For example, within the legal system algorithms can predict recidivism better than criminal court judges (Kleinberg et al., 2017). Such changes have a profound impact on public administrations as they increasingly use algorithms for decisionmaking and service delivery. Scholars have argued, that the introduction of algorithms in decision-making procedures can cause profound shifts in the way bureaucrats make decisions (Peeters & Schuilenberg, 2018; Van der Voort et al., 2019), and that algorithms may affect broader organizational routines and structures (Meijer & Grimmelikhuijsen, 2021).
At the same time, critics highlight several dangers of algorithmic decision-making. They argue that algorithms can produce decisions or recommendations that disproportionately negatively affect disadvantaged groups and that they are simply not accurate in their predictions (Boyd & Crawford, 2012; Barocas & Selbst, 2016; Giest & Samuels, 2020). For example, several local governments in the Netherlands implemented a system that used a machine-learning algorithm (Systeem Risicoindicatie, SyRI) to detect potential welfare fraud amongst benefit recipients. Privacy activists objected to this system and in 2020 it was judged in a court of law to be ‘discriminatory’ and ‘not transparent’. Here, vulnerable citizens, often with a migrant background, were unfairly profiled and suspected of welfare fraud (Huisman, 2019).
A major issue underlying this machine bias is the lack of algorithmic transparency (Janssen & Van den Hoven, 2015; Kroll et al., 2016). Algorithms – and the datasets that feed them – tend to suffer from a lack of accessibility and a lack of explainability (Lepri et al., 2018). This is due to the fact that algorithms used in government decisions are often inaccessible because they are developed by commercial parties that consider them intellectual property (Mittelstadt et al., 2016). Machine-learning algorithms further produce decisional outcomes that may not make sense to people (Burrell, 2016). This so-called ‘black box problem’ of algorithmic decision-making makes perceived bias or unfair algorithms hard to scrutinize and contest. This is problematic because when public servants make decisions based on these non-transparent algorithms, this comes at the very real risk of making (unintentional) biased decisions which could erode public trust in government (Whitakker et al., 2018). In short, for automated decision-making explainability and accessibility are prominent challenges when tasks are delegated to complex algorithmic systems (Ananny, 2016; Sandvig et al., 2016; Giest, 2019; Dencik & Kaun, 2020).
To date, various conceptual pieces have been published that highlight the potential impact of algorithms on the way government works (e.g. Agarwal, 2018; Pencheva et al., 2018) and on decision-making of individual bureaucrats (Young et al., 2020). At the same time these authors call for more transparent and accountable algorithms (e.g. Busuioc, 2020). It is time to complement these critical reflections and conceptual analyses with empirical research in the field of public administration to gain a better understanding of how algorithms and algorithmic transparency affect governmental routines and decisions.
Thus, as scholars of public administration, there is a need to ‘get up to speed’ with the rapid rise of algorithms in government decision-making. Such analyses can be sobering and indicate that some of the more sweeping claims surrounding algorithms need a reality check. For instance, Van de Voort et al. (2019) highlight, using case study research, that data analysts who provide algorithmic predications have limited influence and are often simply bypassed by policy makers. However, how algorithmic transparency affects public administration – although it is often touted as important – is rarely investigated.11
This special issue on algorithm transparency addresses this imbalance and presents six excellent contributions to sharpen our conceptual and empirical understanding of algorithms in government. Furthermore, the issue includes a very insightful book review of Yeung and Lodge’s edited volume Algorithmic Regulation (2019). The articles vary in terms of their theoretical perspective and methodology, but overall, they show the importance of a multi-level perspective on algorithm-use in public administration.
In this introductory article we set the stage for the special issue on algorithmic transparency in government. From a bird eye’s point of view there are two main take-aways from the six contributions to this special issue. Firstly, they paint a nuanced and perhaps a slightly more optimistic picture of algorithmic decision-making and transparency in government. Algorithmic decision-making does not have to be opaque and does not unequivocally lead to diminished decisional discretion. Machine learning algorithms can even be enablers of transparency in government decisions. Secondly, the articles in this issue bring together transparency challenges experienced across the different analytical levels of government, namely the macro-, meso-, and micro-levels. This highlights the importance of looking at government algorithm-use from various levels in order to uncover the connections between the national level contexts, institutional structures, organizational policies as well as the behavior of individual bureaucrats. With a special focus on these findings, the editorial sketches a future research agenda for transparency-related algorithmic challenges
2.Developing a multilevel analytical perspective on algorithm-use in public administrations
The current landscape covering algorithmic applications in government is spread across different fields of research that range from legal dimensions related to European GDPR regulations (e.g. Goodman & Flaxman, 2017; Kaminski & Malgieri, 2020) to social psychology aspects surrounding individual agency on the receiving end of automated decisions (e.g. Wagner, 2019; Gran et al., 2020). These publications are often disjointed in separate journals and fields. In this issue, we offer a view on the different levels involved in these dynamics. The articles address transparency challenges of algorithm-use at the macro-, meso- and micro-level. This distinction is a common way to classify research in public administration and management and entails the specific level of analysis of a study (e.g. Jilke et al., 2019; Roberts, 2020). Building on this work this can be linked to algorithms and algorithmic transparency as follows:
• The macro level describes phenomena from an institutional perspective: which national systems, regulations and cultures play a role in algorithmic decision-making? For example, Peeters and Schuilenberg (2018) analyze how predictive algorithms transform existing norms and rules in the justice system.
• The meso level mostly pays attention to the organizational and team level: what kind of organizational and group strategies are developed and how do government organizations deal with algorithmic transparency in general? One example is a chapter by Meijer and Grimmelikhuijsen (2021) on “algorithmization” in which the authors argue that the introduction of a new algorithm in a government organization triggers various dimensions of change in organizational policies, structures and informational relations.
• The micro level focuses on individual attributes, such as beliefs, motivation, interactions and behaviors. For instance, how do individual street-level bureaucrats use algorithms and do transparent algorithms lead to different/better decisions by these bureaucrats? For instance, Zouridis, Van Eck and Bovens (2020) study algorithmic discretion of street-level bureaucrats and find that discretionary power does partially shift to software and IT developers. However, this depends on the level and type of automation in the specific decision-making context.
All three levels of analyses are covered in the special issues on algorithmic transparency. We argue that 1) there are specific transparency challenges at each level and 2) future research needs to look into the interactions between the three levels.
2.1Macro-level contributions
The macro-level describes algorithms and algorithmic transparency in a European and national regulatory context.
First, Ahonen and Erkkilä focus on institutional aspects in the Finnish context for their contribution. They utilize a conceptual angle around rhetoric and semantic fields to analyze the dynamics of formulating regulation addressing algorithmic transparency. Through document analysis and interviews, they uncover that European Union and Finnish laws and regulations are intertwined in complex ways in that there are binding standards, such as the GDPR, but member states retain leeway in how specific policies are formulated and implemented. At the national level, there are further ideational and conceptual struggles for power when it comes to the interpretation of legislation concerning algorithmic and other automatic decision-making in government. These findings contribute to the larger discussion in two important ways: First, that there are constraining and enabling factors embedded in European and national frameworks in combination with the member state tradition of the public sphere when it comes to transparency of algorithms and algorithmic decision-making. This leads to a call for more comparative studies on this topic. And second, the authors emphasize the need for looking at the role of expert advisory bodies for future research to have additional oversight and control while potentially offering insights into the process itself.
Secondly, Ingrams employs a technical approach to increase institutional transparency of public commenting in a law-making process in the United States. The article applies an unsupervised machine learning analysis of thousands of public comments submitted to the United States Transport Security Administration on a 2013 proposed regulation for the use of new full body imaging scanners in airports. The algorithm highlights salient topic clusters in the public comments which could help policymakers to understand open public comment processes. This shows that algorithms should not (only) be subject to transparency but can also be used as a tool for transparency in government decision-making.
2.2Meso-level contributions
For the meso-level, we include organizational and managerial strategies with regard to algorithmic transparency.
The article by Criado, Valero, and Villodre highlights the interaction between the meso and micro level by focusing on a program implemented in the Spanish region of Valencia that affects decision discretion of individual bureaucrats. The authors zoom in on the black box problem of algorithms, addressing accessibility and explicability issues. This is done in the context of the implementation of the Spanish SALER Early Warning System, which prevents irregularities in the Valencian regional body. The authors provide insights into the discretionary space of bureaucrats working with the system through interviews and show that the system is seen as a support structure by bureaucrats that produces humanly comprehensible responses. This positive account of such a system is traced back in the article to the fact that SALER was initially defined as a decision support system rather than as a decision-making system and system output does not equal the final decision. Also, the authors identify pathways for achieving algorithmic transparency by civil servants in developing algorithms or to develop auditing processes within the organization. Overall this article offers a somewhat more optimistic account of the impact of algorithms and algorithmic transparency.
Bannister and Connolly provide a conceptual contribution to better understand the potential risks associated with algorithms and AI use in government. They argue that these risks are currently not well understood and that various types of algorithms pose different types of risks to individuals and groups in society. Arguably the most important contribution is the development of a three-dimensional risk management framework including transparency, comprehensibility and impact. According to the authors demands for transparency should be tied to potential impact of an algorithm. High levels of algorithmic transparency may be demanded for algorithms with life altering impact, such as decisional algorithms that aid judicial sentencing or medical treatment. The authors argue we should have calibrated responses in terms of transparency and accountability to manage the risks associated with algorithm-aided decisions.
2.3Micro-level contribution
Finally, the micro-level addresses behavioral dimensions of bureaucrats working with algorithmic systems.
The article by Bullock, Young and Wang focuses on this analytical level by looking at the relationship between artificial intelligence, discretion and bureaucratic form in public organizations. The authors specifically look at the standardization dynamics facilitated by technology use and its effect on discretionary power of bureaucrats and highlight the nuances visible in such a setting. Bullock et al. point towards a continuum of bureaucracy from street to system-level, which shapes the organizational context for decision making and task execution. Based on this conceptual framework, the article presents a case analysis of Policing and Public Health Insurance Administration. The cases describe how the use of AI affects discretionary space and how both organizational and task characteristics influence the uses of AI. A key contribution of this discussion is that AI can, but does not necessarily, encourage a linear transitioning into a system-level bureaucracy. Hence, processes of automation in government organizations are not linear nor monotonic. This raises future research questions around the feedback loop between AI changing bureaucracies and bureaucracies changing AI applications.
Another article that focuses on the micro-level dynamics of algorithmic systems is by Peeters looking at the variation in ‘human-algorithm interaction’. Through a systematic literature review, Peeters highlights the role of agency that is built into algorithms due to them being designed by humans as well as various behavioral dynamics in the human-algorithm interaction, such as using algorithms as a convenient default for human decision-making. In addressing the different scenarios that play out in the human-algorithm interaction, the article is able to draw two major conclusions: First, human agents are rarely fully ‘out of the loop’ and levels of oversight and override designed into algorithms should be understood as a continuum, and second, bounded rationality, satisficing behavior, automation bias and frontline coping mechanisms play a crucial role in the way humans use algorithms in decision-making processes. For future research, Peeters thus suggests taking a closer look at the behavioral mechanisms in combination with identifying relevant skills of bureaucrats in dealing with algorithms.
Finally, the book review by Lorenz of a volume edited by Yeung and Lodge (Algorithmic Regulation) shows that this edited volume provides much needed insights into regulatory issues on algorithms by analyzing them from an ethical, legal and managerial point of view. In the final section of the volume authors acknowledge the lack of explainability and accountability associated with algorithmic decision-making. This issue is explored mostly from a legal approach and building on this notion we offer some more empirical insights with this special issue.
Table 1 highlights the transparency challenges and future research questions in line with the levels identified.
Table 1
Overview contributions specified by level of analysis
Level | Main findings |
---|---|
Macro-levelEuropean and national regulatory context |
|
Meso-levelOrganizational and managerial structure |
|
Micro-levelBehavior and decision-making of bureaucrats |
|
3.Concluding remarks and future research
The contributions in this special issue shed light on different dimensions of algorithmic transparency, which range from the regulatory context at the European and national level, to organizational policies and discretionary spaces of individual bureaucrats. What becomes obvious in this discussion is that the different levels, the macro-, meso-, and micro-levels are linked and need to be viewed in coherence to achieve more transparency for algorithm use in government. For instance, bureaucratic behavior is not only determined by the algorithmic tools they work with, but also by the regulatory and organizational context as well as their personal experience and the training provided.
Overall, these contributions paint a nuanced and perhaps a more optimistic picture of algorithmic decision-making and transparency in government. Processes of algorithmization do not have to lead to opaqueness nor do they have to lead to less discretion and programmed bias. Algorithmic tools can even be used to create more transparency of otherwise unclear decision-processes. Furthermore, the authors in this issue offer ways forward to improve algorithmic usage and increase transparency. Here, it is important to address these issues at all levels of analysis, if we are to provide accountable oversight of algorithmic decision-making and to use algorithms in the most effective way. Building on the contributions in this special issue we identify the following key areas for improvement in Fig. 1.
Figure 1.
Areas for improvement of algorithmic transparency and decision-making.
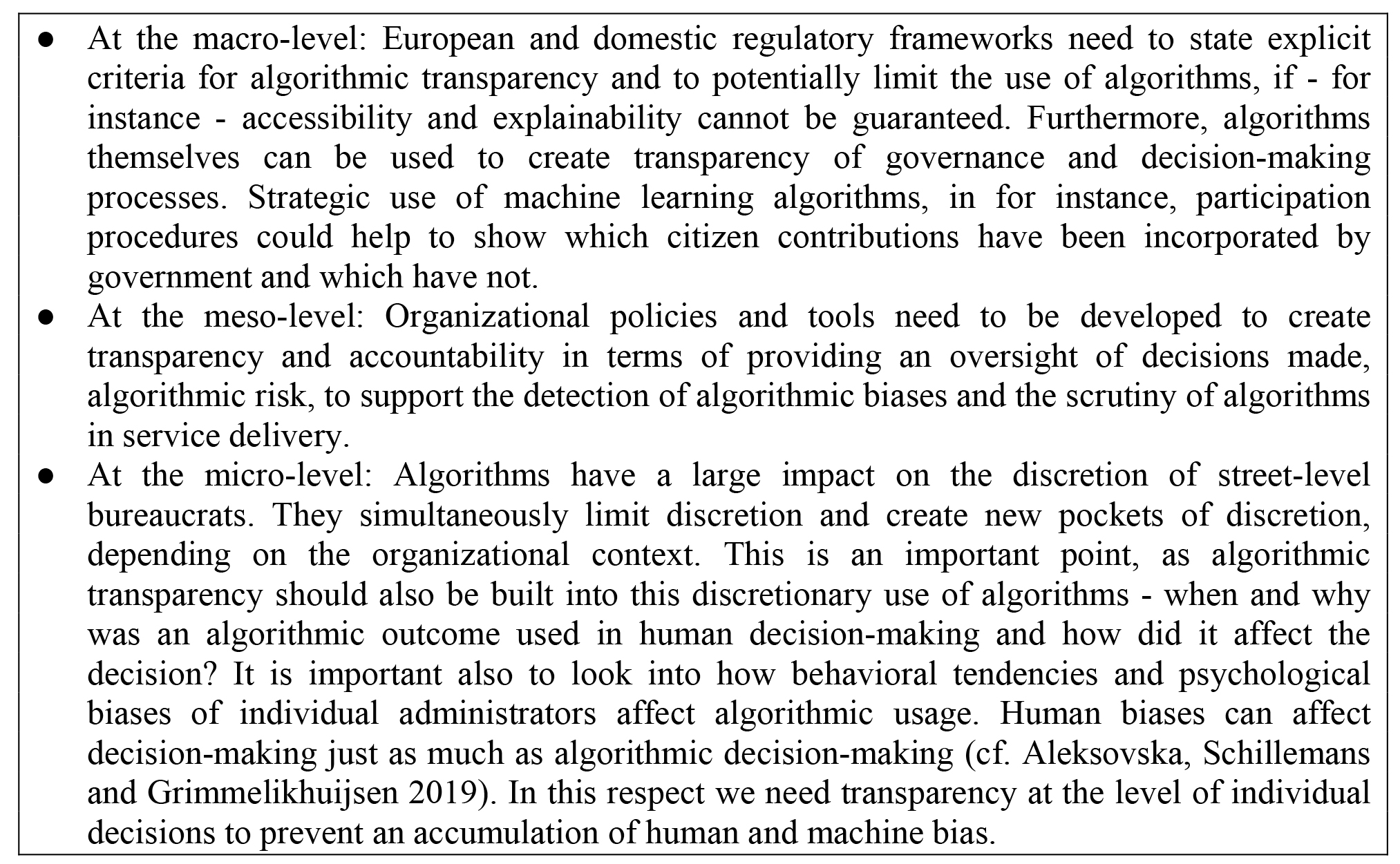
The contributions in this special issue also yield new important research questions. We argue that future research should focus on two broad areas. First, we need to flesh out the effects, dynamics and determinants of algorithmic decision-making and transparency at all levels of analysis, in order to get a better understanding of how algorithms have evolved in government and public service settings. Important questions are:
• What are enabling and constraining factors in the regulatory and institutional environment (macro) surrounding the deployment of algorithms?
• Which organizational tools and strategies will enable proper scrutiny of algorithmic transparency (meso)?
• What behavioral tendencies affect algorithm use and how does algorithmic transparency trigger the desired behavioral response amongst bureaucrats and how will it affect citizen attitudes towards government (micro)?
Secondly, the contributions in this issue indicate that the dynamics between the three levels need to be better understood. For instance, we know that individual behavior is constrained and enabled by regulatory context and existing administrative norms. Future research could look into how these macro-level factors shape the use of algorithms. At the same time micro-level decisions will slowly but surely cause shifts at the macro level. For instance, algorithmic transparency in government decisions will lead to different expectations of algorithms usage amongst bureaucrats and citizens. These expectations may result in new norms and a change in regulatory frameworks. How and whether such shifts take place is subject to debate and needs further empirical investigation. Figure 2 highlights the potential relations among all levels.
Figure 2.
Studying algorithms and algorithmic transparency from multiple levels of analyses.
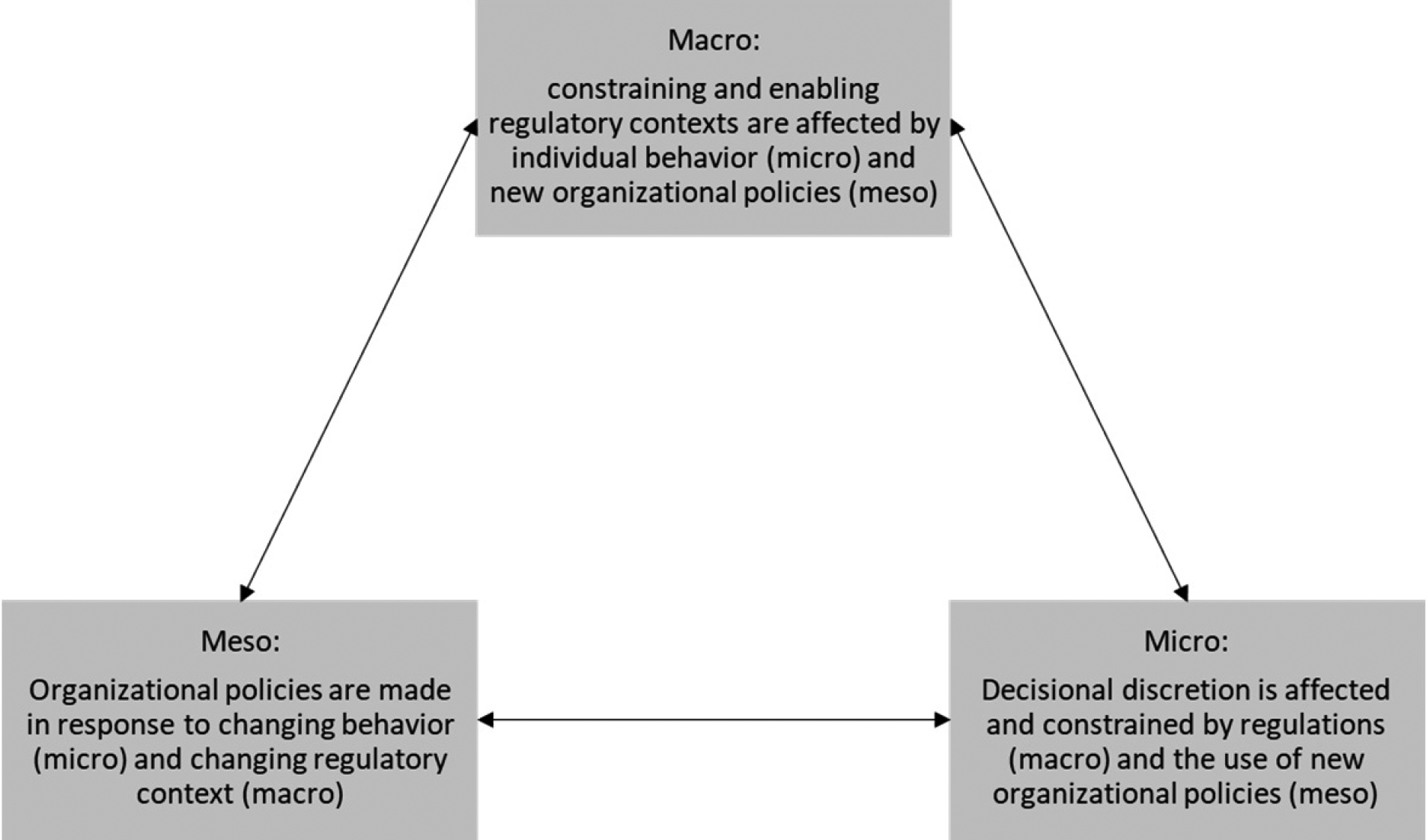
These research questions provide ample material for future research by raising questions around the interplay of regulatory and organizational contexts as well as the intersection of bureaucrats and algorithms where research on built-in transparency mechanisms is lacking. In some ways this resembles research on government transparency in general. Scholars find different dynamics and effects of government transparency depending on the institutional and policy context (e.g. Meijer, 2013; De Fine Licht, 2014; Grimmelikhuijsen et al., 2020). It is likely that a similar contextual approach is needed to understand algorithmic transparency. Ultimately, the costs and benefits of transparency will vary across policy fields, algorithmic or AI decision-making type as well as the organizational features in which such systems are embedded. To advocate the responsible and transparent use of algorithms future research should look into the interplay between these different levels and contexts.
It is clear that the use of algorithms in the delivery of government services is an ongoing development which will expand in the next few years and it is critical that these developments are embedded in democratic norms of accountability, trust, oversight and transparency. To do so we need to analyze algorithms from multiple levels.
Notes
1 There have been (empirical) investigations but so far these are not applied in a public sector context (e.g. Kizilcec, 2016).
Acknowledgments
We would like to thank the editors-in-chief of Information Polity for making this special issue possible in the pages of the journal. We are also grateful for their guidance and feedback along the way. Furthermore, thank you to all the reviewers who provided excellent and elaborate feedback. Their time and effort in these already stressful and busy times is very much appreciated.
References
[1] | Agarwal, P.K. ((2018) ). Public administration challenges in the world of AI and bots. Public Administration Review, 78: (6), 917-921. |
[2] | Ahonen, P., & Erkkilä T. ((2020) ). Transparency in algorithmic decision-making: Ideational tensions and conceptual shifts in Finland. Information Polity, 4. |
[3] | Aleksovska, M., Schillemans, T., & Grimmelikhuijsen, S. ((2019) ). Lessons from five decades of experimental and behavioral research on accountability: a systematic literature review. Journal of Behavioral Public Administration, 2: (2). |
[4] | Ananny, M. ((2016) ). Toward an ethics of algorithms: convening, observation, probability, and timeliness. Science, Technology, & Human Values, 41: (1), 93-117. |
[5] | Bannister, F., & Connolly, R. ((2020) ). Administration by algorithm: a risk management framework. Information Polity, 25: (4). |
[6] | Burrell, J. ((2016) ). How the machine ‘thinks’: understanding opacity in machine learning algorithms. Big Data & Society, 3: (1), 1-12. doi: 10.1177/2053951715622512. |
[7] | Barocas, S., & Selbst, A.D. ((2016) ). Big data’s disparate impact. California Law Review, 104: (3), 671-732. |
[8] | Bullock, J., Young, M., & Wang, Y.-F. ((2020) ). Artificial Intelligence, Bureaucratic Form, and Discretion in Public Service. Information Polity, 4. |
[9] | Burrell, J. ((2016) ). How the machine ‘thinks’: understanding opacity in machine learning algorithms. Big Data & Society, 3: (1), 1-12. |
[10] | Busuioc, M. Accountable Artificial Intelligence: Holding Algorithms to Account. Public Administration Review. doi: 10.1111/puar.13293. |
[11] | Crawford, K., & Boyd, D. ((2012) ). Critical questions for big data. Information, Communication & Society, 15: (5), 662-679. |
[12] | Criado, J.I., Valero, J., & Villodre, J. ((2020) ). Artificial Intelligence and discretionary decision-making. An analysis of SALER algorithmic early warning system to promote transparency and fight corruption. Information Polity, 4. |
[13] | Dencik, L., & Kaun, A. ((2020) ). Datafication and the welfare state. Global Perspectives, 1: (1). |
[14] | Goodman, B., & Flaxman, S. ((2017) ). European union regulations on algorithmic decision-making and a “right to explanation”. AI Magazine, 38: (3), 50-57. |
[15] | Giest, Sarah. ((2019) ). Decision-making with algorithms, On the digital state. In: Schinkelshoek Jan and Aalt Willem Heringa (Eds.), Groot onderhoud of kruimelwerk. Montesquieu Institute, EAN 9789462906853. |
[16] | Giest, S., & Samuels, A. ((2020) ). ‘For good measure’: data gaps in a big data world. Policy Sciences, 53: , 559-569. |
[17] | Gran, A.-B., Booth, P., & Bucher, T. ((2020) ). To be or not to be algorithm aware: a question of a new digital divide? Information, Communication & Society, doi: 10.1080/1369118X.2020.1736124. |
[18] | Grimmelikhuijsen, S.G., Piotrowski, S.J., & Van Ryzin, G.G. ((2020) ). Latent transparency and trust in government: unexpected findings from two survey experiments. Government Information Quarterly, 37: (4), 101497. |
[19] | Huisman, C. ((2019) , June 27). SyRI, het fraudesysteem van de overheid, faalt: nog niet één fraudegeval opgespoord. De Volkskrant retrieved from https://www.volkskrant.nl/nieuws-achtergrond/syri-het-fraudesysteem-van-de-overheid-faalt-nog-niet-een-fraudegeval-opgespoord∼b789bc3a/ on Oct 29, 2020 |
[20] | Ingrams, A. ((2020) ). A machine learning approach to open public comments for policymaking. Information Polity, 25: (4). |
[21] | Janssen, M., & van den Hoven, J. ((2015) ). Big and open linked data (BOLD) in government: a challenge to transparency and privacy? Government Information Quarterly, 32: (4), 363-368. |
[22] | Jilke, S., Olsen, A.L., Resh, W., & Siddiki, S. ((2019) ). Microbrook, mesobrook, macrobrook. Perspectives on Public Management and Governance, 2: (4), 245-253. |
[23] | Kaminski, M.E., & Malgieri, G. ((2020) ). Multi-layered explanations from algorithmic impact assessments in the GDPR. In Proceedings of the 2020 Conference on Fairness, Accountability, and Transparency, pp. 68-79. |
[24] | Kizilcec, R.F. ((2016) ). How much information? Effects of transparency on trust in an algorithmic interface. In Proceedings of the 2016 CHI Conference on Human Factors in Computing Systems, pp. 2390-2395. ACM. |
[25] | Kleinberg, J., Lakkaraju, H., Leskovec, J., Ludwig, J., & Mullainathan, S. ((2017) ). Human decisions and machine predictions. The Quarterly Journal of Economics, 133: (1), 237-293. |
[26] | Kroll, J.A., Barocas, S., Felten, E.W., Reidenberg, J.R., Robinson, D.G., & Yu., H. ((2016) ). Accountable algorithms. University of Pennsylvania Law Review, 165: (3), 633-706. |
[27] | Lepri, B., Oliver, N., Letouzé, E., Pentland, A., & Vinck, P. ((2018) ). Fair, transparent, and accountable algorithmic decision-making processes. Philosophy & Technology, 31: (4), 611-627. doi: 10.1007/s13347-017-0279-x. |
[28] | Margetts, H., & Dorobantu, C. ((2019) ). Rethink government with AI. Nature, 568: , 163-165. |
[29] | Meijer, A. ((2013) ). Understanding the complex dynamics of transparency. Public Administration Review, 73: (3), 429-439. |
[30] | Meijer, A.J., & Grimmelikhuijsen, S.G. ((2021) ). Responsible and Accountable Algorithmization: How to Generate Citizen Trust in Governmental Usage of Algorithms. In Schuilenburg, M., & Peeters, R. (eds.) The Algorithmic Society: Technology, Power, and Knowledge. Routledge. |
[31] | Mittelstadt, B.D., Allo, P., Taddeo, M., Wachter, S., & Floridi, L. ((2016) ). The ethics of algorithms: mapping the debate. Big Data & Society, 3: (2), 2053951716679679. |
[32] | Peeters, R. ((2020) ). The Agency of Algorithms: Understanding Human-Algorithm Interaction in Administrative Decision-Making. Information Polity, 4. |
[33] | Peeters, R., & Schuilenburg, M. ((2018) ). Machine justice: governing security through the bureaucracy of algorithms. Information Polity, 23: (3), 267-280. |
[34] | Pencheva, I., Esteve, M., & Mikhaylov, S.J. ((2018) ). Big Data and AI – A transformational shift for government: So, what next for research? Public Policy and Administration. doi: 10.1177/0952076718780537. |
[35] | Roberts, A. ((2020) ). Bridging levels of public administration: how macro shapes meso and micro. Administration & Society, 52: (4), 631-656. |
[36] | Sandvig, C., Hamilton, K., Karahalios, K., & Langbort, C. ((2016) ). When the algorithm itself is a racist: diagnosing ethical harm in the basic components of software. International Journal of Communication, 10: , 4972-4990. |
[37] | Van der Voort, H.G., Klievink, A.J., Arnaboldi, M., & Meijer, A.J. ((2019) ). Rationality and politics of algorithms. Will the promise of big data survive the dynamics of public decision making? Government Information Quarterly, 36: (1), 27-38. |
[38] | Wagner, B. ((2019) ). Liable, but not in control? Ensuring meaningful human agency in automated decision-making systems. Policy & Internet, 11: (1), 104-122. |
[39] | Whittaker, Meredith, Kate Crawford, Roel Dobbe, Genevieve Fried, Elizabeth Kaziunas, Varoon Mathur, Sarah Myers West, Rashida Richardson, Jason Schultz, Oscar Schwartz. ((2018) ). AI Now report 2018, New York University. |
[40] | Yeung, K., & Lodge, M. ((2019) ). Algorithmic Regulation. Oxford: Oxford University Press. |
[41] | Young, M.M., Bullock, J.B., & Lecy, J.D. ((2019) ). Artificial discretion as a tool of governance: a framework for understanding the impact of artificial intelligence on public administration. Perspectives on Public Management and Governance, 2: (4), 301-313. |
[42] | Zouridis, S., van Eck, M., & Bovens, M. ((2020) ). Automated discretion. In Evans, T., & Hupe, P. (eds.) Discretion and the quest for controlled freedom, pp. 313-329. Cham: Palgrave Macmillan. |