Special issue on evidence-based government: secure, transparent and responsible digital governance
1.Introduction
This special issue is a collection of papers from the 18
In this open-data-driven society, where the data is disseminated, re-used and re-purposed, as well as connected and fused, researchers need to investigate the impact of open data and the risks of the “open data society.” What are suitable policies to govern the data dissemination, distribution, reuse and repurposing? What are different types of data governance models, and what are their relative advantages and disadvantages? What new risks and opportunities does an open data society face? What are the enabling factors and risks of the data-driven policy making paradigm? Can citizens be made more aware of and accountable for their own privacy by developing technology-driven detection mechanisms of privacy risks?
For this special issue, we feature articles that cover theoretical, practical, and technical approaches to solving issues arising when disseminating, reusing, repurposing and hyper-connecting different sources of big data (including open government data, social data, sensor data, and ubiquitous data) for the purposes of innovation and transformation of government and society.
2.Evidence-based government
Evidence-based government is dependent on data-driven governance. It requires the data to be transparent. The data creates the basis for services and information sources that are personalized and easy to act on by individual citizens, thus promoting their possible participation in government decision making. Participation by citizens can create participatory governance, where citizens’ voices and opinions are taken into consideration in policy making and better decision making. This model of data-driven government can best be modeled and explained by a value creation and transformation chain.
2.1Data-driven value transformation chain
The digital society has created an abundance of data, so called Big Data, and the data-driven discoveries, innovations and transformations enabled by Big Data have given rise to the data-driven economy. The idea of the value chain (Porter 1985) is defined as a chain of activities that gives the products more “added value” in a manufacturing (or service) organization. The data-driven economy can be viewed as a value transformation chain, as illustrated in Fig. 1.
Figure 1.
Data-driven value transformation chain.
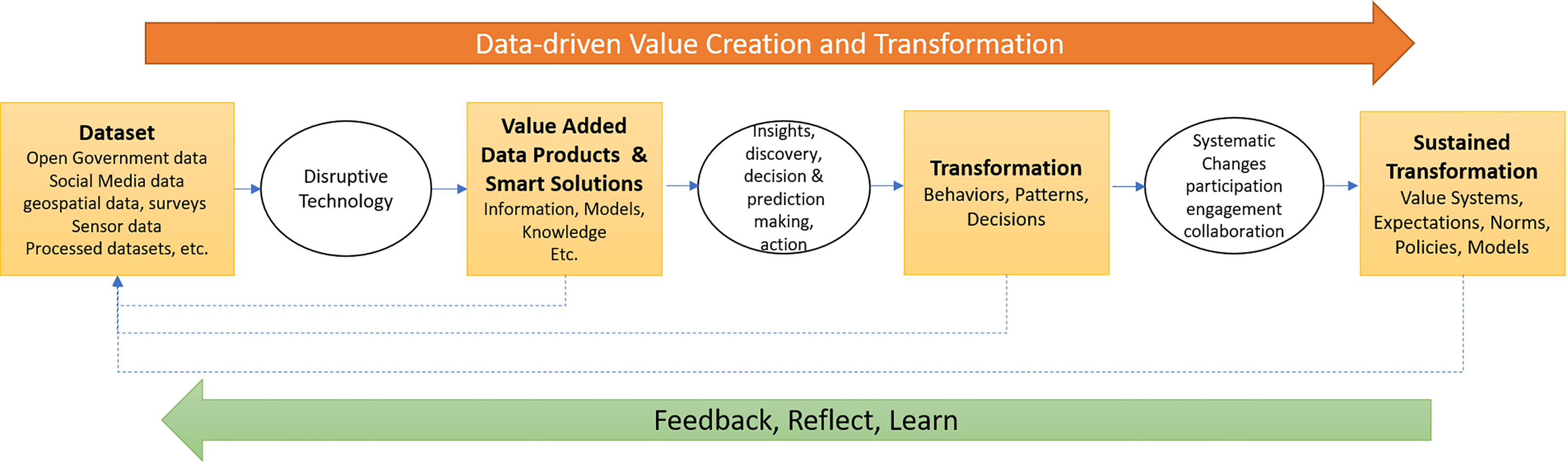
The raw data sets are generated by a myriad of sources, including government public and administrative records (so-called Open Government Data), citizens’ social media data, movement/locational data from mobile devices, transaction data, surveillance data, etc. The digital economy and digital society are relentlessly generating diverse sources and types of data. Disruptive, smart and innovative technologies (e.g. Fan et al. 2015) utilize this data to create value-added data products, that reveal insights, patterns, association rules and other forms of transferable knowledge or prediction models.
These value-added data products help citizens, governments and other stakeholders to gain insights and to make new discoveries to allow more accurate predictions, better decision making processes and efficient and effective administrative actions. These, in turn, will generate transformed behaviors, improved processes (e.g., backend operations or front end services), regular patterns of actions, and fact-based decision making strategies. The participations and collaborations amplify the effect of individual citizens’ or small groups’ transformations, leading to sustained and systemic changes in society’s value systems, accepted norms, expectations, governing rules and policies, collectively shifting the paradigms of governing, decision making, institutional frameworks and societal behaviors.
At each stage of the data transformation chain, the behaviors and actions generated can serve as feedback to earlier stages, to evaluate the actions or transformations, and to serve as new input to the value chain, resulting in an improvement of the effectiveness of the overall system.
This data-driven value transformation chain (data value chain, in short) is different from the life cycle of data transformation which is a process of cleaning data for higher analytics (Curry 2016). It can apply to any vertical domain, e.g. businesses, healthcare institutions, educational institutions, branches of government, etc., and when applied to the government domain, this value transformation chain can create novel citizen services, new smart city products, new ways to identify and implement critical policies, new governance models where citizen engagement and collaborations are key components, as well as new paradigms and up-to-date norms to govern organizations and people (Osborne 2010).
In order for the data-driven value chain to work effectively, researchers need to address some of the risks in creating values in the data value chain. They should ensure the data and computational technologies are secure, protective of private information, and be in compliance with all relevant standards and regulations. If needed, the data and innovative approaches need to be auditable. Overcoming these risks will ensure that the data-driven value transformation chain works efficiently and responsibly.
2.2Article summaries
In this special issue, selected articles cover various topics on the data transformation value chain that creates evidence-based government, including:
• Public service production models;
• Smart city governance models;
• Detecting and understanding policy change;
• New ways of making security policies and strategies using crowdsourcing;
• De-identification policies and risk distribution models; and
• Identifying compliance and auditing elements of government Information and Communication Technology (ICT).
In relation to Value Creation Models, Antonio Cordella and Andrea Paletti compare four different modes of public service production according to their operational capabilities and their related logics of value creation. First, the bureaucracy and manufacturing logic of value creation includes in-house value creation models and joined-up value creation models. Second, the co-production and the service logic of production considers crowdsourced production models and open-sourced production models. For each mode of public service production, they identify the associated benefits, risks and possible solutions that can be deployed to mitigate the risks. The authors suggest that there are no “good or bad” ways to produce services, but different ways to produce value using different configurations of ICT and other resources, and the need to decide about the most suitable way to produce a service should take into consideration the necessary operational capabilities and the need to control the final outcome.
In Smart Governance Models: Authors, Peter Perycheck and Gabriela Viale Pereira conducted a literature review that focuses on smart governance. Smart governance involves the intelligent use of ICT, such as social media, to improve decision-making through better collaboration among different stakeholders, i.e. governments and citizens. It also involves openness as a factor that increases citizen engagement and support for the development of new governance models for smart government. Smart governance may also have an important role in smart city initiatives, which require complex interactions between governments, citizens and other stakeholders. Based on the literature review, this paper coins a definition of ‘smart city governance’ and contributes to developing a framework for building new, smart governance models addressing the challenges of the digital society, collaborative governance, information sharing, citizen engagement, transparency and openness.
The article Policy Changes focusses on using text mining analytics, and Lee and Kim have performed a longitudinal study on institutional changes of economic policies. The concept changes over two decades showing that policy changes occur not necessarily through any external, exogenic factors and regardless of the stability of internal institutions and external environments. The analytical results show that policy elites constantly compete with their past equivalents to influence the “policy package” required to acquire and maintain legitimate authority, while responding to the government’s needs for creative innovation and change. Thus, this data-driven case study of Korean governments shows that value creation and transformation are continuous and dynamic, caused by internal policy competition and the pressure to be more innovative.
In Crowdsourcing for Cybersecurity Policy Innovation, Chatfield and Reddick present the Pentagon’s vulnerability reward program (VRP) or” bug bounty program”, which crowdsources software bug detection to the cybersecurity community and which fits with the US national cybersecurity policy. Their case study results show the feasibility of the government adoption and implementation of the innovative concept of VRP, to enhance the government cybersecurity posture, as well as to address the need to develop sophisticated cybersecurity policies and enhanced cybersecurity capabilities. Public engagement with the vulnerability identification is a way of transforming the national cybersecurity policy making, as shown in the data-driven value creation and transformation chain.
The article Privacy risks and risk distribution models assesses the the data-driven value chain in relation to the protection of personally identifiable data. Joo et al. present a comparative study on the de-identification policies in the European Union, the United States, Japan, and Korea, all of which are now actively pursuing de-identification policies. The major principles used in de-identification policies are used to compare the policies from different regions. Since there is no perfect de-identification, there are always risks of re-identification. The authors identify the constituencies of the de-identification policies and analyze the roles and responsibilities of each of these constituencies. This study may serve as a step toward providing the theoretical basis for distributing burdens and responsibilities arising from the de-identification policies.
In ICT Governance for Compliance Verification, it is argued that in any evidence-based government copious numbers of Government ICT assets are used and innovated daily. Based on a survey of the literature, international and national regulations, and best practices bodies, Clara et al. present and discuss common elements that are considered important to guide regulatory compliance verification of ICT management practices. They provide a methodology of identifying and extracting elements from compliance guidelines, regulations, standards, and academic documents, and use them to audit the ICT compliance. They show a case study of a Brazilian Federal public organization to which they apply these compliance elements for measuring the organization’s compliance and the audit levels for the used software.
3.Summary
We hope that this collection of articles will provide digital government researchers, students and government practitioners with an opportunity to reflect on the ways in which evidence-based government depends on data generated and consumed through the data-driven transformation value chain. The data and data products have the power to accomplish transformations in all the products of the data-driven value creation and transformation chain, including services, behaviors, actions, policies, expectations, norms, and governance standards. The articles presented here provide the reader with an in-depth treatment of topics related to evidence-based government, with an ambition of contributing to a more secure, transparent and responsible digital governance future.
Acknowledgments
We acknowledge the individual authors for their valuable contributions of their original research work to this special issue and their cooperation with the rigorous peer review process. We sincerely thank all the anonymous reviewers who spent their valuable time to review the articles and to improve the quality of the special issue. We extend our thanks to the journal Editors-in-Chief and IOS Press for their support and encouragement for this publication. This work was partially funded by National Research Foundation of Korea (NRF-2017S1A3A2066084).
References
[1] | Curry, E. ((2016) ). The Big Data Value Chain: Definitions, Concepts, and Theoretical Approaches. In: Cavanillas J., Curry E., Wahlster W. (eds) New Horizons for a Data-Driven Economy. Springer. |
[2] | Fan, S., Lau Raymond, Y.K. & Zhao, J. Leon ((2015) ). Demystifying Big Data Analytics for Business Intelligence Through the Lens of Marketing Mix, Big Data Research, Volume 2, Issue 1. |
[3] | Osborne, S.P. (2010) (ed.). The New Public Governance: Emerging Perspectives on the Theory and Practice of Public Governance, Routledge. |
[4] | Porter, M.E. ((1985) ). Competitive Advantage: Creating and Sustaining Superior Performance. New York. |
Appendices
Appendix
Special Issue Editors:
Soon Ae Chun, CUNY
Nabil R. Adam, Rutgers University
Beth Simone Noveck, NYU & Yale University