A Systematic Literature Review of MABAC Method and Applications: An Outlook for Sustainability and Circularity
Abstract
Multiple Criteria Decision-Making (MCDM) is one of the most reliable and applicable decision-making tools to address real-life complex and multi-dimensional problems in accordance with the concepts of sustainable development and circular economy. Although there have been several literature reviews on several MCDM methods, there is a research gap in conducting a literature review on the Multi-Attributive Border Approximation area Comparison (MABAC) as a useful technique to deal with intelligent decision-making systems. This study attempts to present a comprehensive literature review of 117 articles on recent developments and applications of MABAC. Future outlook is provided considering challenges and current trends.
1Introduction
Decision-making is one of the most significant and vital activities of management to achieve organizational goals, which is highly dependent on its quality. It comprises accurate definition of objectives, finding various possible solutions and assessing their feasibility based on limitations, evaluating the outcomes, and finally, choosing and implementing the best solution. Decision-making is known as the essence of management based on the experts’ points of view. It should be pointed out that, however, decision-making problems have been complicated by an increased level of complexity, compelling decision-makers to go through new technologies and tools that simplify the processes supporting decision-making (Biswas et al., 2022; Pérez-Gladish et al., 2020; Rani et al., 2021; Torkayesh et al., 2021a).
Multiple Criteria Decision-Making (MCDM) approaches include a variety of decision-making methods which have been applied and developed for many years at various educational and industrial levels, particularly in the fields of management, Industrial Engineering (IE) and Operations Research (OR). MCDM methods are classified into two main groups: Multiple Objective Decision-making (MODM) techniques for finding the best possible solution in the design phase, and Multiple Attribute Decision-Making (MADM) techniques for choosing the best alternative. Since the practical applications of MADM are higher than MODM, a large number of MADM approaches have been offered by scholars over the last 60 years and this trend will continue. The decision-making problems regarding MADM include several main components such as the main goal(s), criteria (sometimes accompanied with sub-criteria) or a set of performance measures, decision-maker or a team of decision-makers, and a set of decision alternatives along with a set of unknown and a set of output results. Nowadays, there are many opportunities to extend the existing MADM techniques in order to make them more efficient in tackling complex and intelligent decision-making systems.
On the other hand, the sustainable development concept along with the circular economy approach has recently stuck out for governments, industries and researchers to conduct their decision-making processes. In fact, handling these non-ignorable issues along with other decisions leads to multi-dimensional complex decision-making. Due to this, this study tries to address these concepts in line with the application of MCDM approaches where Multi-Attributive Border Approximation area Comparison (MABAC) is regarded as one of the most potent methods to cope with intelligent decision-making systems (Gong et al., 2020). Next sections review the MABAC from a comprehensive perspective to address different real-world applications.
1.1Decision-Making for Sustainability and Circularity
Sustainability is being able to keep up with a perceptible behaviour for an unknown period of time. In order to present a clear definition, three areas related to economic, environmental and social aspects should be addressed at the same time. Effective economic decision-making has always been a critical issue in private or public organizations.
The built-in connection between the environment and economic growth originates from the study performed by Nordhaus (1991), where he asserted that the reduction of emissions will restrict its effect and guarantee a higher economic growth in the future. In the last two decades, the research community has been keeping track and modelling the relationships between economic growth, environmental sustainability and human welfare as a basic topic.
Over the years, the significant increase of economic activities and vast amounts of consumption have created difficulties for long-term planning and hindered sustainable management from emerging and growing sufficiently (Colapinto et al., 2019). Hence, it has become more and more essential to integrate the interests of the different stakeholders associated with or influenced by long-term planning actions, in order to provide a balance between their requirements including economic development along with the environmental, social and future generations. Sustainability decision-making models in this regard can be taken into account as a baseline condition to reach sustainable development, such that economic representatives often go for decision-making processes in order to enhance their organizations’ performance (Carayannis et al., 2018). Figure 1 represents the annual evolution of scientific attempts on MCDM applications to address Sustainable Development Goals (SDGs) between 2016 and 2020 and according to 143 articles reviewed by Sousa et al. (2021). As can be seen, a steep upward trend has started from 2019 which demonstrates the significance of sustainability to be addressed in decision-making problems. Sousa et al. (2021) also defined the 2030 agenda framework for different classifications of SDGs to be addressed with MCDM methodologies.
Fig. 1
Annual scientific production from 2016 to 2020 on the application of MCDM methods to address SDGs (Sousa et al., 2021).
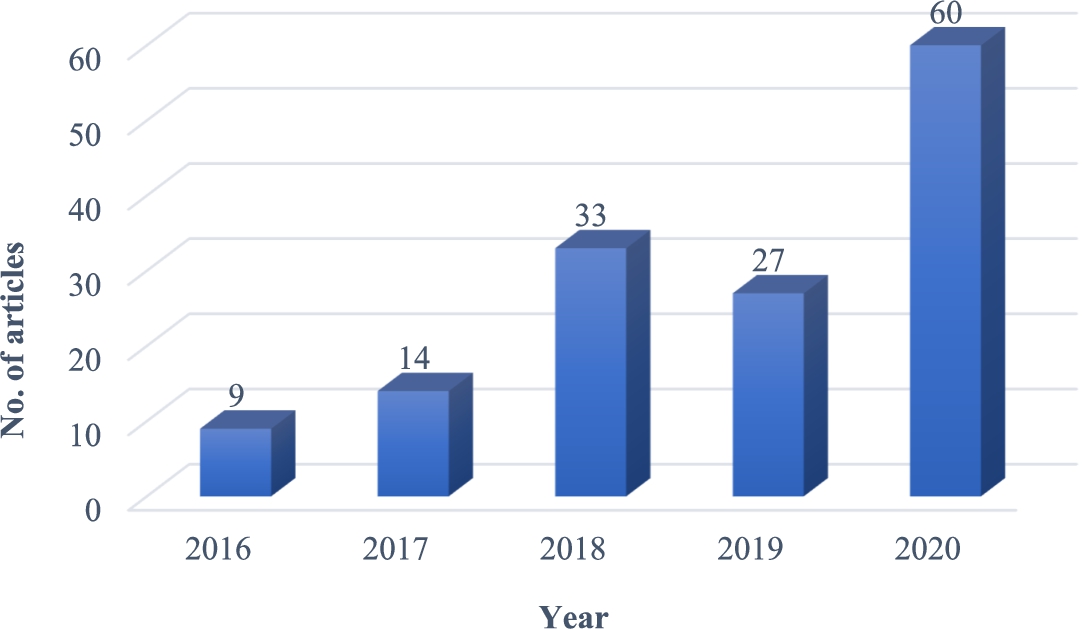
The decision-makers (policymakers and public/private performers) seek appropriate trade-offs, while adapting economic, social and environmental criteria or objectives (Yazdani et al., 2020a). Accordingly, each stakeholder has to treat diverse and contradictory criteria/objectives, giving rise to complex and intelligent MCDM (Torkayesh et al., 2021). Plenty of researchers have counted on and utilized the MADM and MODM models as formal and standard methodologies using the obtainable technical information to balance and assess stakeholder values, develop solutions and enhance environmental and social sustainability. One of the most-addressed fields is Supply Chain Management (SCM) (Haleh and Hamidi, 2011; Liao et al., 2020; Wang and Hsu, 2012).
In the meantime, and with the search for alternative solutions as the means to create more sustainable economies, the circular economy concept emerged. The circular economy aims not only for the reasonable usage of resources but also to minimize the demand for their generation and the re-utilization of those already in effect (Machado and Davim, 2020). Following this framework, it replaces the linear economic end-of-life approach with new circular streams through re-utilization, restoration and rehabilitation within integrated and fundamental processes. The circular economy, in supporting the dissociation of economic growth from proliferating resource consumption, a relationship otherwise recognized as unavoidable, reformulates the functional flows of production chains and causes doubts about the hegemonic model coming from wastage and disposal.
For establishing any circular economy, one decisive aspect comprises the means of waste management (Ali et al., 2021; Lahri et al., 2021). According to this framework, recycling plays a key and fundamental role in terms of transforming such waste into new/raw materials/products with the possibility of re-utilization. Given the applicability of recycling for this process, there are major demands to significantly uplift the waste recovery rate, mainly in cities and the major suburbs. For example, the utility of integrated multi-criteria evaluation to provide a circular city can be illustrated in Fig. 2.
Consistent with sustainable development, there is increasing agreement on the necessity of a gradual and continuous transition to a more sustainable economic growth and almost all countries are encountering this emerging challenge (Mardani et al., 2020). This proposed mechanism sets clear targets for waste reduction in all steps of the value chain from production to consumption, recovery, repair and re-manufacturing, recycling and waste management, and secondary raw material that affect the development of the economy. Furthermore, this approach provides a long-term direction for recycling and waste management.
Over the last few years, the application of MCDM techniques for evaluating circular economy perspective and sustainability issues has increased sharply, and to the best of our knowledge, we can claim that these methodologies can be efficiently utilized to deal with these emerging concepts in decision-making problems since they have revealed their usefulness to address a large variety of environmental and management problems.
1.2Contributions
The application of MCDM techniques in sustainable development and circular economy is much less mature than its application in other fields of study. The MABAC technique is known as one of the most novel MADM techniques and the literature has not maintained the same rate of progress with the quick extension of knowledge in this field. Consequently, there is still a need for a comprehensive review study on the applications of the MABAC method with an outlook for sustainability issues in different areas of applications as one of the most efficient methods in expert decision-making. This research systematically reviews the previous efforts paid off to investigate the applicability of MABAC in decision-making problems. It gives and adds notable insights into the literature of MABAC by taking into account a variety of novel perspectives in the evaluation of articles, including the classification of the published studies based on their time trends, journals, locations, application areas, MCDM goals and other joint MCDM approaches.
1.3Structure of the Article
The structure of the remaining sections is as follows. Section 2 introduces the MABAC in detail. Section 3 reviews the most relevant studies in the literature about MABAC in terms of different criteria. Applications of MABAC are investigated in Section 4. Section 5 presents methodological and managerial insights. Section 6 concludes the research and discusses the main findings and challenges, and finally gives a useful outlook including recommendations for future studies.
2MABAC Method
Pamučar and Ćirović (2015) developed the MABAC method in 2015, and to date, this multi-criteria methodology has found wide application for tackling numerous real-world problems. The main advantages of the MABAC multi-criteria framework include:
The algorithm of the MABAC method is represented in Fig. 3.
Fig. 3
MABAC method algorithm.
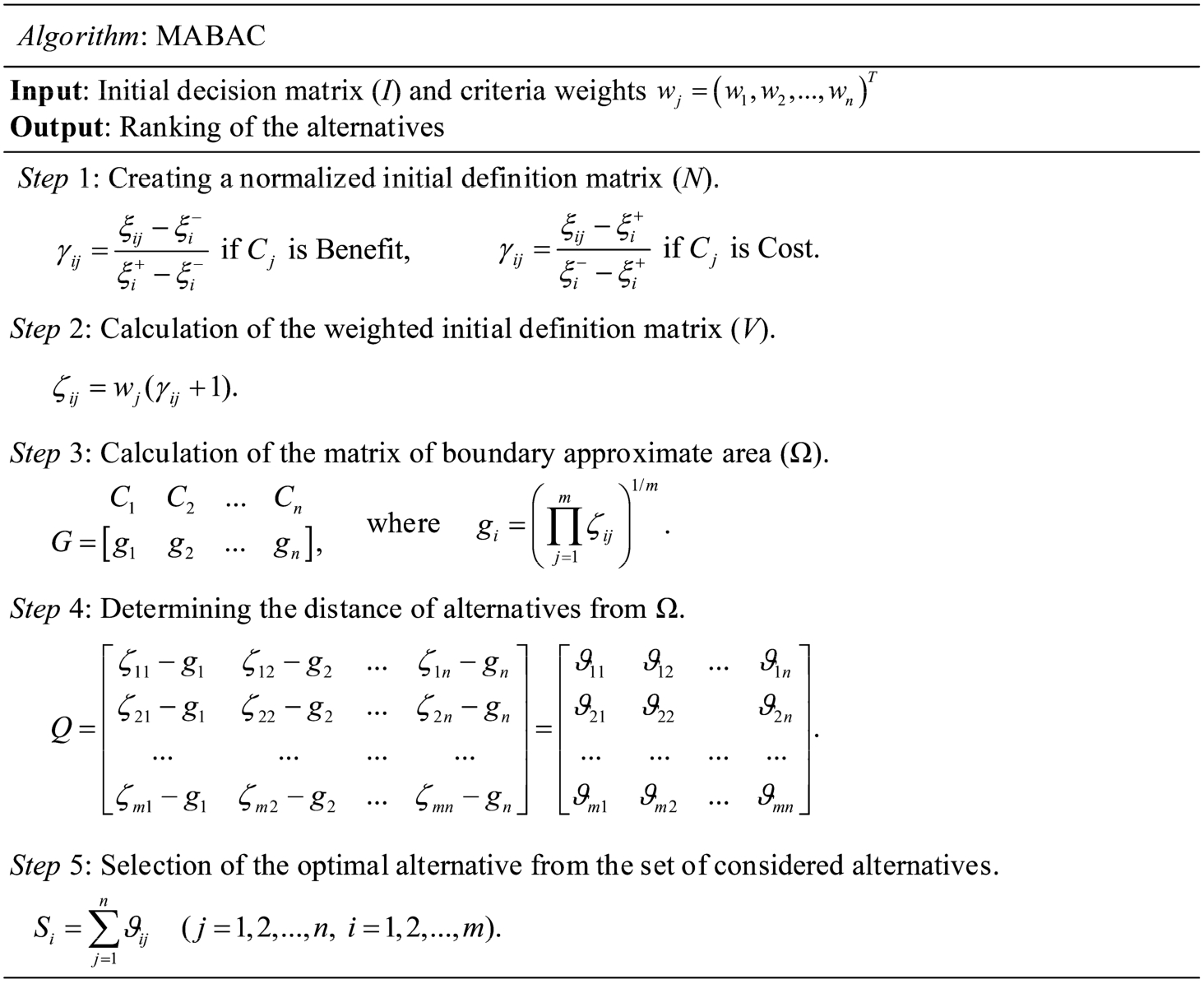
The mathematical formulation of the MABAC method is based on determining the distance of alternatives from the boundary approximate area (Ω). Based on the defined distances of alternatives from Ω, all alternatives are classified into two sets, which we call the upper approximate area (
Since the ideal alternative is in
3Literature Survey
Fig. 4
Approximate areas of the MABAC method.
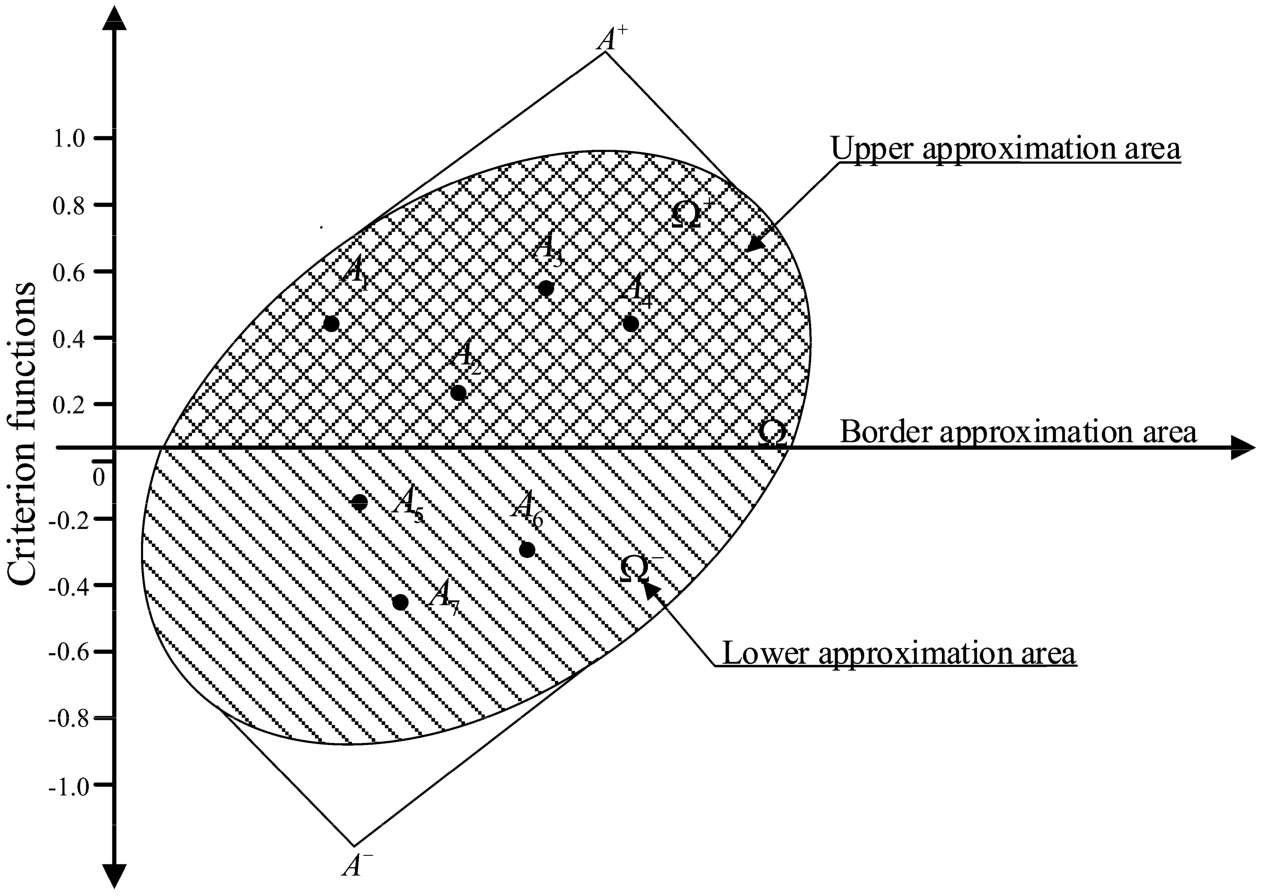
3.1Research Methodology
Over recent decades, MCDM methods have attracted high interest to be used in order to reliably address complicated decision-making problems. MABAC technique is one of the recently developed MCDM methods to address the ranking of several alternatives with respect to several decision criteria under a multi-aspect environment. Due to the high applicability of the MABAC method, a large number of articles have investigated different types of complicated decision-making problems using different versions of the MABAC. Figure 5 shows the steps conducted to search articles through different databases until the end of May 2022. Finally, 117 articles were selected for the literature review process.
For this purpose, all 117 selected articles are reviewed under different trends. First, articles are studied based on their published year, location of contributing authors and co-authors, journals that articles are published in, methods combined with the MABAC, uncertainty sets implemented for the MABAC, and most importantly, different applications of the MABAC in different industries and fields.
Fig. 5
Article search methodology.
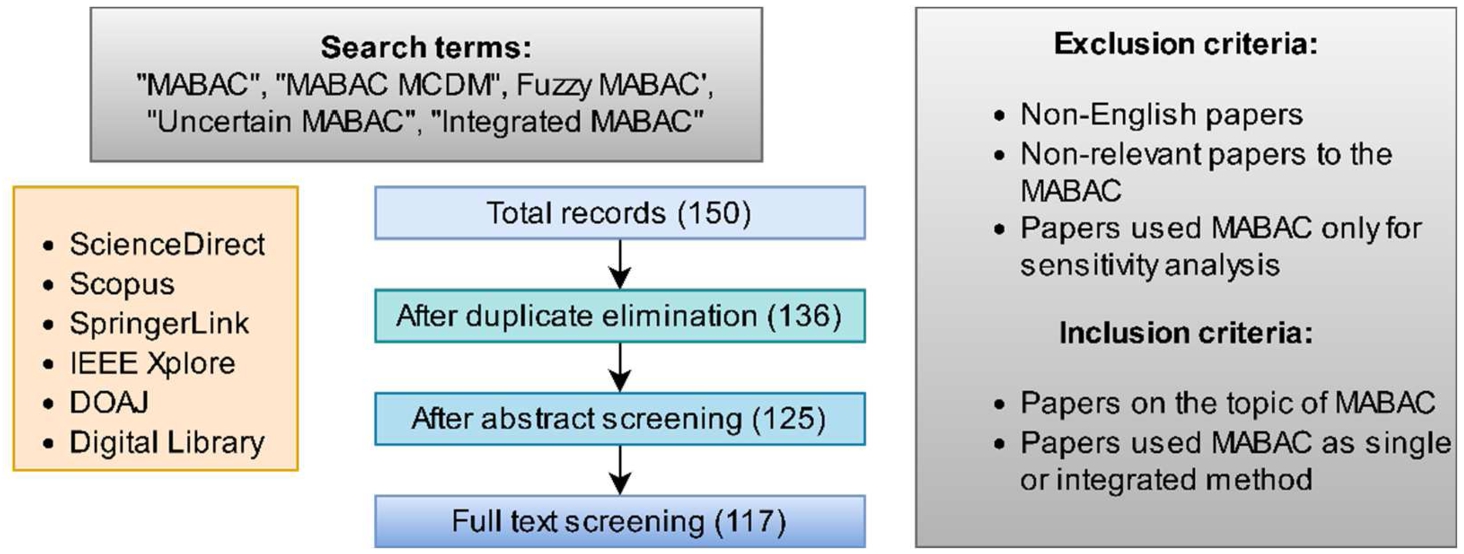
3.2Time Trend
MABAC method has been one of the most frequently developed MCDM methods since its development in 2015 (Pamučar and Ćirović, 2015). The growing rate of articles using the MABAC ascertains the demand for reliable decision-making tools for complex and multi-aspect decision-making problems. Figure 6 presents the distribution of published studies which have employed the MABAC method as a single decision-making model or part of an integrated decision model since 2015. According to Fig. 6, records show an important increase in the number of studies after 2017 which followed an increasing trend until 2022.
Fig. 6
Timeline of MABAC-based studies.
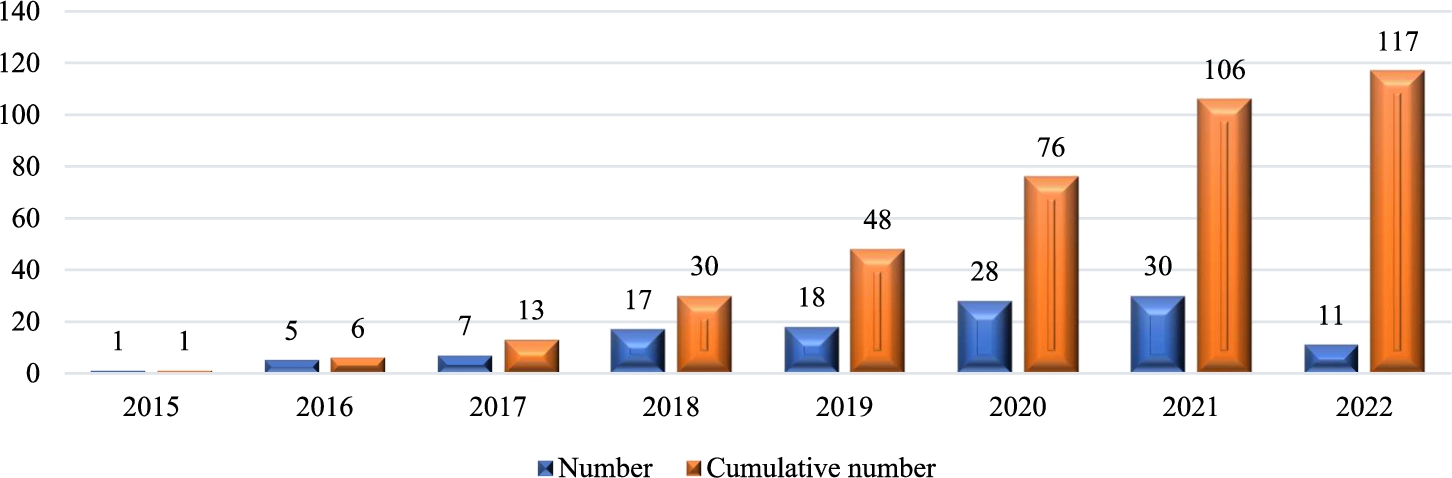
3.3Location Distribution
In order to highlight the contribution of countries which have used the MABAC method as a single MCDM or as a part of an integrated MCDM model, the location of authors of all the papers is considered. According to Fig. 7, it can be observed that countries like China, Serbia, and India are countries in which most of the studies are carried out to tackle different MCDM problems using the MABAC method. Location distribution of published articles indicates how more complicated decision-making problems are subjected to be tackled using the MCDM concept. Based on our meta-analysis results, at least one author from China contributed to 55 articles, at least one author from Serbia contributed to 26 articles, and at least one author from India contributed to 25 articles. Lithuania, Turkey, Bosnia and Herzegovina, Iran, Chile, Canada, USA, Spain, Vietnam, and UK are countries in which authors have contributed to more than one article.
Fig. 7
Locational distribution of authors publishing MABAC studies.
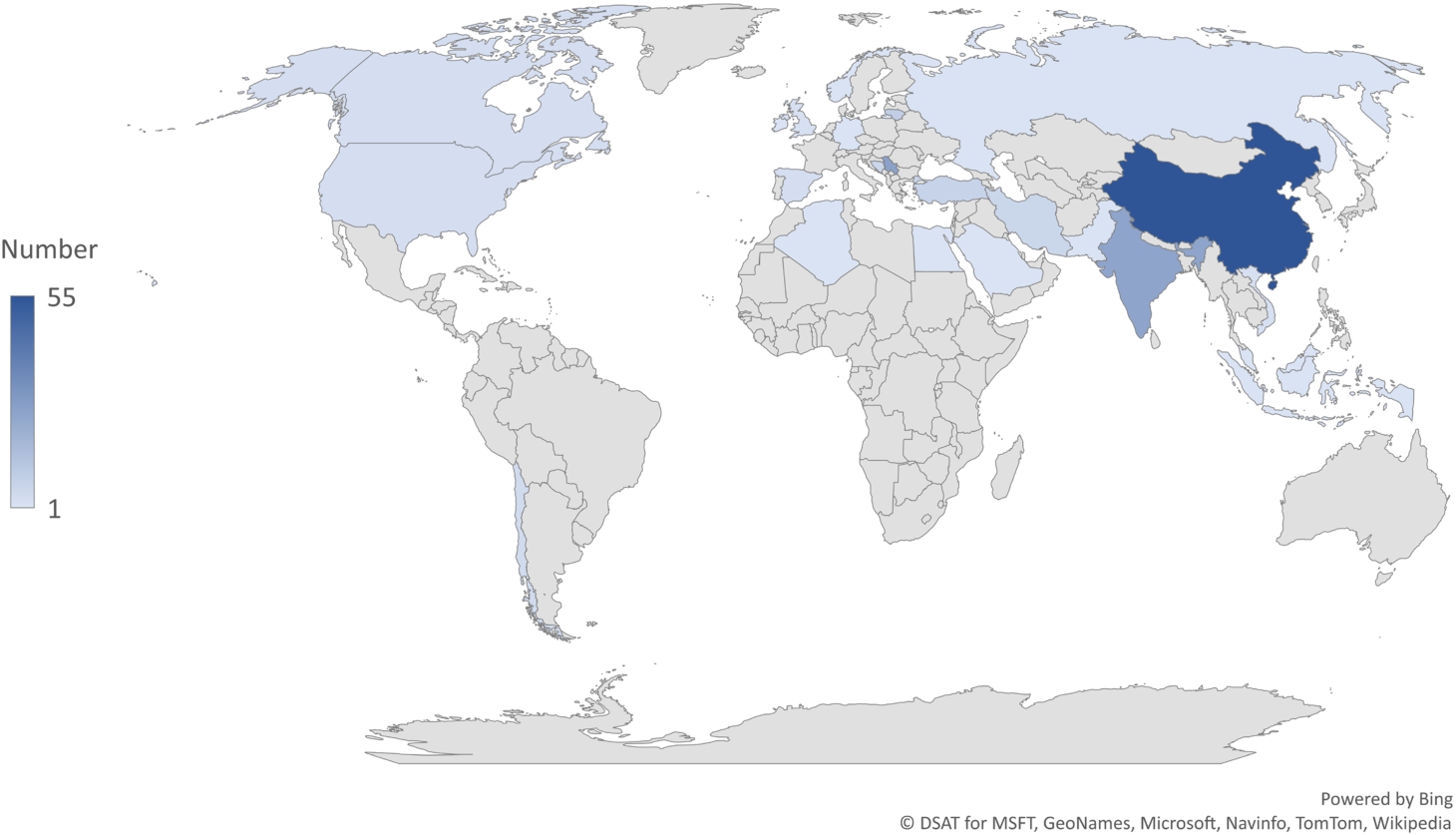
Fig. 8
VOSviewer network analysis based on co-authorship in terms of published documents.

In order to provide further information regarding the locational distribution of authors applying the MABAC method, VOSviewer (version 1.6.18) developed by Van Eck and Waltman (2010) is used. In this regard, two networks are depicted based on the co-authorship analysis in terms of countries considering the total number of published documents and citations of the published documents. Figure 8 represents the co-authorship analysis weighted by the total number of documents published in each country. According to Fig. 9, China and Serbia share most of the published studies which have used MABAC. An important insight from Fig. 8 is about the high link strength of Serbia compared to China. Although China holds a higher share of published studies, Serbia has the highest co-authorship degree with a total of 17 countries, whereas China has co-authorship with 10 countries. This analysis shows how Serbian researchers are in favor of collaboration on various research studies with partners from different countries.
Fig. 9
VOSviewer network analysis based on co-authorship in terms of citations of published documents.

In a similar way, Fig. 9 shows the co-authorship analysis based on the total number of citations from each country. Although Serbia holds a lower number of published studies compared to China (almost half), Serbia’s total number of citations is very close to China’s total citation. Unlike Fig. 8, we observe that India, Bosnia and Herzegovina and Lithuania have been shown in a more highlighted way due to their high citations. The high total citation of China is due to its high number of published studies; however, a large proportion of Serbia’s citations are related to the very first paper which introduced MABAC by Pamučar and Ćirović (2015).
3.4Journal Distribution
As illustrated in Fig. 5, 117 articles passed the search processing framework in order to be considered for the review process. Among 117 articles, our list includes 115 journal articles, one online digital library, and one conference. Decision-making: Applications in Management and Engineering, Journal of Intelligent & Fuzzy Systems, International Journal of Intelligent Systems, Expert Systems with Applications, and Computational and Applied Mathematics are the main targets of decision-making studies which used the MABAC method. Selected 117 articles for the review process are published in 65 journals which shows how complicated decision-making problems exist in various research fields (see Table 1).
Table 1
Journal distribution of published MABAC studies.
No. | Journal | # of studies |
1 | Decision Making: Applications in Management and Engineering | 10 |
2 | Journal of Intelligent & Fuzzy Systems | 9 |
3 | International Journal of Intelligent Systems | 8 |
4 | Expert Systems with Applications | 5 |
5 | Computational and Applied Mathematics | 4 |
6 | Computers & Industrial Engineering | 4 |
7 | Applied Soft Computing | 3 |
8 | Operational Research in Engineering Sciences: Theory and Applications | 3 |
9 | Sustainability | 3 |
10 | Economic Research-Ekonomska Istraživanja | 2 |
11 | Engineering Applications of Artificial Intelligence | 2 |
12 | Informatica | 2 |
13 | International Journal of Environmental Research and Public Health | 2 |
14 | International Journal of Fuzzy Systems | 2 |
15 | International Journal of Information Technology & Decision Making | 2 |
16 | International Journal of Machine Learning and Cybernetics | 2 |
17 | Journal of Ambient Intelligence and Humanized Computing | 2 |
18 | Journal of Cleaner Production | 2 |
19 | Kybernetes | 2 |
20 | Socio-Economic Planning Sciences | 2 |
21 | Soft Computing | 2 |
22 | Algorithms | 1 |
23 | ArXiv ID | 1 |
24 | Axioms | 1 |
25 | Biomass and Bioenergy | 1 |
26 | Complex & Intelligent Systems | 1 |
27 | Defence Science Journal | 1 |
28 | Defence Technology | 1 |
29 | Energy | 1 |
30 | Evolution in Computational Intelligence | 1 |
31 | Expert Systems | 1 |
32 | Facta Universitatis, Series: Mechanical Engineering | 1 |
33 | Fundamenta Informaticae | 1 |
34 | Fuzzy Systems and Knowledge Discovery | 1 |
35 | Granular Computing | 1 |
36 | Green Supplier Evaluation and Selection: Models, Methods and Applications | 1 |
37 | IEEE Access | 1 |
38 | Information Sciences | 1 |
39 | International Journal of Production Economics | 1 |
40 | International Journal of Production Research | 1 |
41 | International Journal of Strategic Property Management | 1 |
42 | Journal of Air Transport Management | 1 |
43 | Journal of Enterprise Information Management | 1 |
44 | Journal of Industrial and Management Optimization | 1 |
45 | Journal of Mathematics | 1 |
46 | Journal of Multiple-Valued Logic & Soft Computing | 1 |
47 | Journal of The Institution of Engineers | 1 |
48 | Land Use Policy | 1 |
49 | Management Science Letters | 1 |
50 | Materials Today: Proceedings | 1 |
51 | Mathematics | 1 |
52 | Neural Computing and Applications | 1 |
53 | Neutrosophic Sets and Systems | 1 |
54 | OPSEARCH | 1 |
55 | Plos One | 1 |
56 | Proceedings of the Institution of Mechanical Engineers, Part O: Journal of Risk and Reliability | 1 |
57 | Renewable Energy | 1 |
58 | Research in Transportation Business & Management | 1 |
59 | Research Journal of Textile and Apparel | 1 |
60 | Symmetry | 1 |
61 | Technological and Economic Development of Economy | 1 |
62 | Transport and Telecommunication | 1 |
63 | Tunnelling and Underground Space Technology | 1 |
64 | Vojnotehnički glasnik | 1 |
65 | Waste Management | 1 |
Table 1 presents detailed information on journals, online libraries, and conferences which have published different versions of the MABAC method for different applications.
3.5Methodology & Uncertainty
Considering the high complexity of decision-making problems, new MCDM methods are continuously being developed to increase the capacity of decision-makers in expressing their opinions. Generally, MCDM based decision-making tools can be categorized into two types of singular MCDM-based methods, and integrated MCDM-based methods. In the context of integrated MCDM-based methods, we investigated MCDM models that are used in integrated form with the MABAC method. The major contribution of such methods is to calculate the weight vector of criteria as well as generate integrated ranking results. Table 2 presents the frequency of different MCDM methods that are used next to MABAC to tackle different complicated problems. Best-Worst Method (BWM), as one of the most well-known MCDM methods, is frequently used with the MABAC method in 14 articles. Furthermore, Analytical Hierarchy Process (AHP), DEcision-MAking Trial and Evaluation Laboratory (DEMATEL), and Shannon Entropy are used as MCDM methods integrated with the MABAC. With a short look at Table 2, it is understood that most of the MCDM approaches integrated with the MABAC are specific methods developed for the weight determination process.
Table 2
Frequency of methods combined with MABAC.
No. | Methods | # of studies | References |
1 | BWM | 14 | (Chakraborty et al., 2020a; Luo and Xing, 2019; Muravev and Mijic, 2020; Pamucar et al., 2019; Pamučar et al., 2018a; Wu et al., 2019) |
2 | AHP | 12 | (Biswas and Das, 2019; Bojanic et al., 2018; Božanić et al., 2018; Božanić et al., 2016; Büyüközkan et al., 2020; Gupta et al., 2019; Pamučar et al., 2018b; Roy et al., 2018; Sharma et al., 2018; Vasiljević et al., 2018) |
3 | DEMATEL | 9 | (Agarwal et al., 2020; Can and Toktas, 2018; Debnath et al., 2017; Gigović et al., 2017, 2016; Pamučar and Ćirović, 2015; Shi et al., 2017; Yazdani et al., 2020) |
4 | Shannon Entropy | 9 | (Biswas and Das, 2018; Chakraborty et al., 2020b; Fan et al., 2020; Liu et al., 2019; Mishra et al., 2020a; Verma, 2021; Wei et al., 2020a, 2020b; Zhu et al., 2018) |
5 | TOPSIS | 5 | (Gupta et al., 2019; Milosavljević et al., 2018; Noureddine and Ristic, 2019; Peng and Dai, 2018) |
6 | ANP | 4 | (Chatterjee et al., 2017; Gigović et al., 2017; Hashemizadeh et al., 2021) |
7 | CPT | 4 | (Chatterjee et al., 2017; Gigović et al., 2017; Hashemizadeh et al., 2021) |
8 | CRITIC | 4 | (Wei et al., 2019) |
9 | FUCOM | 4 | (Božanić et al., 2019; Bozanic et al., 2020; Noureddine and Ristic, 2019; Nunić, 2018) |
10 | SWARA | 4 | (Liu et al. (2020a); Vesković et al. (2018)) |
11 | WASPAS | 4 | (Gupta et al., 2019; Pamucar et al., 2019; Peng and Dai, 2017) |
12 | COPRAS | 2 | (Hashemizadeh et al., 2021) |
13 | ELECTRE | 2 | (Ji et al., 2018; Milosavljević et al., 2018) |
14 | LBWA | 2 | (Gupta et al., 2019; Pamucar et al., 2019; Peng and Dai, 2017) |
15 | PIPRECIA | 2 | (Biswas, 2020) |
16 | PROMETHEE | 2 | (Luo and Xing, 2019) |
17 | TODIM | 2 | (Hashemizadeh et al., 2021; Liu et al., 2020b) |
18 | AFSA | 1 | (Bose et al., 2020) |
19 | ARAS | 1 | (Bose et al., 2020) |
20 | CCSD | 1 | (Bose et al., 2020) |
21 | Choquet Integral Geometric | 1 | (Peng and Yang, 2016) |
22 | EAC | 1 | (Peng and Yang, 2016) |
23 | EDAS | 1 | (Peng et al., 2017) |
24 | FMEA | 1 | (Liu et al., 2019) |
25 | GRA | 1 | (Hashemizadeh et al., 2021) |
26 | IRN | 1 | (Hashemizadeh et al., 2021) |
27 | ITARA | 1 | (Gong et al., 2020) |
28 | MAIRCA | 1 | (Adar and Delice, 2019) |
29 | RPR | 1 | (Gupta et al., 2019; Pamucar et al., 2019; Peng and Dai, 2017) |
30 | SWOT | 1 | (Büyüközkan et al., 2020) |
31 | Weighted Power Average | 1 | (Liang et al., 2020) |
32 | Weighted Bonferroni Distance | 1 | (Wang et al., 2018) |
Another important point regarding the integration of MCDM methods is reflected in the implementation of uncertainty sets to enhance the possibility of expressing uncertain, vague, and incomplete information in decision-making process. 98 out of 117 reviewed studies have implemented the MABAC method under different types of uncertainty sets. Triangular fuzzy set has the highest share among all uncertainty sets. Hesitant fuzzy set and rough theory, are the second and third frequently used uncertain models with the MABAC, respectively. Table 3 presents a piece of detailed information about the 32 different types of uncertainties that are implemented for the MABAC method to address different MCDM problems.
Table 3
Integration of uncertainty sets into MABAC.
No. | Uncertainty | # of studies |
1 | No Uncertainty Method | 19 |
2 | Triangular Fuzzy Set | 13 |
3 | Hesitant Fuzzy Set | 10 |
4 | Rough Theory | 9 |
5 | Interval-Valued Intuitionistic Fuzzy Set | 8 |
6 | Intuitionistic Fuzzy Set | 7 |
7 | Interval Type-2 Fuzzy Set | 4 |
8 | Probabilistic Linguistic Set | 4 |
9 | D Numbers | 3 |
10 | Fermatean Fuzzy Environment | 3 |
11 | Picture Fuzzy Set | 3 |
12 | Z Numbers | 3 |
13 | 2-Tuple Linguistic Neutrosophic | 2 |
14 | Bipolar Fuzzy Set | 2 |
15 | Bipolar Neutrosophic Linguistic | 2 |
16 | Grey Theory | 2 |
17 | Linguistic Neutrosophic Set | 2 |
18 | q-Rung Orthopair Fuzzy Set | 2 |
19 | Single-Valued Neutrosophic Linguistic Set | 2 |
20 | Spherical Fuzzy Sets | 2 |
21 | Trapezoidal Fuzzy Set | 2 |
22 | Type-2 Neutrosophic Numbers | 2 |
23 | Exponential Fuzzy | 1 |
24 | Interval 2-Tuple Linguistic Cloud Model | 1 |
25 | Interval Type-2 Trapezoidal Fuzzy Set | 1 |
26 | Interval-Valued Pythagorean Fuzzy Set | 1 |
27 | Linguistic Distribution | 1 |
28 | Linguistic Spherical Fuzzy | 1 |
29 | Picture 2-Tuple Linguistic Set | 1 |
30 | Probability Multi-Valued Neutrosophic Set | 1 |
31 | Pythagorean Fuzzy Set | 1 |
32 | Trapezoidal Fuzzy Neutrosophic Set | 1 |
4Applications of MABAC
In this section, selected articles are categorized and reviewed with respect to their applications and fields in different industries. Based on the findings of the initial review process, we identified 11 applications that MABAC and its extensions are used to address different decision-making problems. Identified categories and yearly distribution of articles in each category is shown in Fig. 10.
Fig. 10
Detailed survey of MABAC studies based on application.
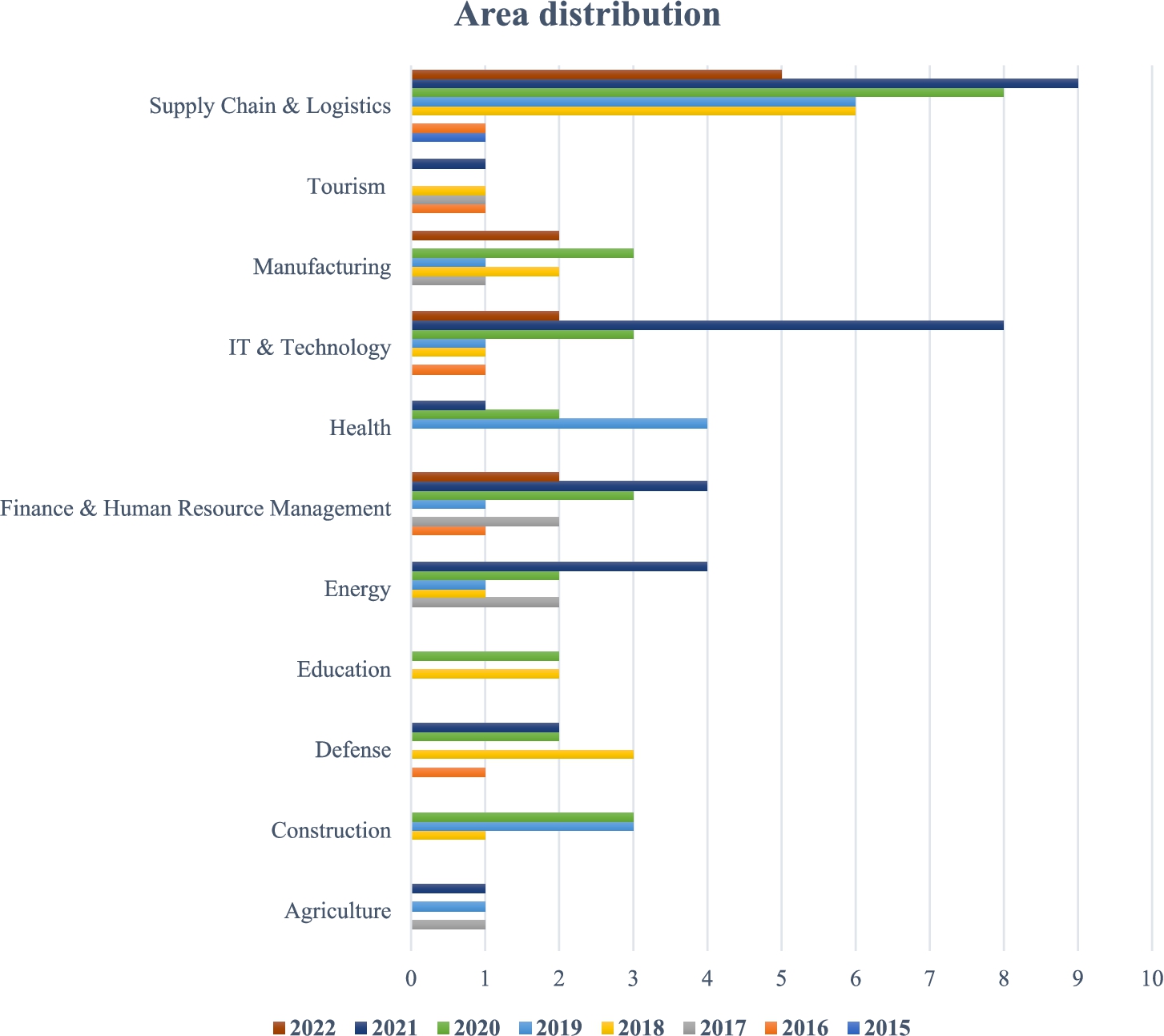
Figure 10 illustrates that supply chain and logistics is the most frequent application category that MABAC and its extensions have used to address. Energy management, finance and human resource management, defence industry, and tourism industry are other important application categories that use MABAC to tackle different decision-making problems.
In order to investigate how interesting these applications have been for other researchers, google scholar citations of each application category are collected (end of May 2022). According to Table 4, supply chain and logistics have the highest number of citations in comparison to other application categories. Energy management, finance and human resource management, defence industry, and tourism industry are other application categories with 359, 325, 256, and 218 citations, respectively.
4.1Agriculture
The agriculture industry and sustainable farming are considered fundamental areas that cover economic, environmental, ecological, and social aspects. The growing rate of farm and crop production with high productivity using high-tech and modern devices extends the agricultural sector’s economic, environmental, and social boundaries. Therefore, different agricultural processes go through various decision-making procedures to determine a specific policy. MCDM methods have been applied in several directions for agricultural uses, such as in location selection, portfolio evaluation, and assessment of edible and other products (Golfam et al., 2019; Jafari Shalamzari et al., 2019; Qureshi et al., 2018; Zamani et al., 2020). Land location selection problems aim to select appropriate lands based on their applicability for specific agricultural activities. Strategic decision-making for agricultural activities is of high significance where different elements of an agricultural project are evaluated and investigated through a wide range of decision analysis processes. Table 5 presents a summary of agriculture studies, which used different versions of the MABAC method for decision-making purposes.
Use of agrochemicals, fragmented crop health management, harvesting practices, integrated crop health solutions, utilization of bio-chemicals on the agro-product, industrial heat consumption, total electricity consumed, biomass resources availability, land price, and workforce price are considered as essential factors.
Table 4
Google Scholar citations of application areas.
No. | Area | Citations |
1 | Agriculture | 59 |
2 | Construction | 150 |
3 | Defence | 256 |
4 | Education | 207 |
5 | Energy | 359 |
6 | Finance & Human Resource Management | 325 |
7 | Health | 163 |
8 | IT & Technology | 104 |
9 | Manufacturing | 78 |
10 | Tourism | 218 |
11 | Supply Chain & logistics | 844 |
Table 5
Applications of MABAC for the agriculture industry.
References | Case study | Methodology | Objective |
(Debnath et al., 2017) | India | G-DEMATEL-MABAC | Evaluation of strategic project portfolios of agricultural by-products |
(Wu et al., 2019) | China | MABAC-BWM | Location selection of agroforestry biomass cogeneration project |
(Wu et al., 2019) | Bosnia and Herzegovina | MABAC-PIPRECIA | Evaluation of rapeseed varieties |
4.2Construction
The construction sector has been considered one of the oldest industries globally and is recognized for being traditional and underdeveloped in many areas such as information technology, SCM, innovation, and risk assessment (Al-Werikat, 2017). Construction projects must be accomplished in complicated dynamic environments that are mostly characterized by risk and uncertainty, and the construction object’s life cycle is full of various risks (Schatteman et al., 2008; Zavadskas et al., 2010). As a result, construction projects face problems that cause delays and failure to satisfy the determined time frame and cost. Many studies illustrate numerous pieces of evidence that many construction projects fail to achieve their budget, time, and even quality goals. It is necessary to assess and analyse the complexity of managing risk policies in different projects appropriately. In this regard, risk assessment in construction projects should be applied differently in each project to evaluate risks in various activities of the project. It also can be considered a vital part of the MCDM process. A summary of the objective, methods used, and possible case study is summarized in Table 6.
Table 6
Applications of MABAC for construction management.
References | Case study | Methodology | Objective |
(Chatterjee et al., 2018) | – | D-ANP-MABAC | Evaluation of risks related to construction projects due to the dynamic nature of risk factors |
(Wang et al., 2019) | – | MABAC | Safety assessment of construction projects due to risk factors |
(Božanić et al., 2019) | Serbia | TFS-FUCOM-MABAC | Location selection for constructing a single-span bailey bridge |
(Liang et al., 2019a) | China | TFS-MABAC | Assessment of risks related to rock burst to identify the most critical areas and risks |
(Pamucar et al., 2020) | Spain | TFNS-Dombi Aggregator-MABAC | Evaluation of suppliers for a construction project in a resilient supply chain |
(Wang et al., 2020) | China | q-ROFS-MABAC | Assessment of several projects in order to determine the most suitable project |
(Liang et al., 2020) | China | LD-Weighted Power Average-MABAC | Emergency decision-making for the failure of construction projects related to waterlogging disasters |
4.3Defence Industry
The defence industry’s promotion is a viable strategic option for countries to endogenize digitization technologies and, therefore, nationalize the necessary means to be the protagonist of their development (Ambros, 2017). Military operations contain essential activities that have numerous risks from various perspectives. However, these operations are conducted for multiple purposes, categorized as the lowest damage taken and the maximum damage given (Karaşan et al., 2019). Therefore, since the defence industry has to deal with many uncertainties, including multi-criteria and many alternatives, it is worthwhile to utilize the MCDM methodologies.
The military decision-making process is an analytical process for troops’ movements, designing operations, logistics, or air defence planning. Logistics is considered one of the significant challenging areas in the defensce industry, and provider selection is one of the most complicated logistical management problems. As a result, numerous studies have been done to develop methods for evaluating transport service providers. In Table 7, we provided a short review of the MABAC methods in the defence industry.
Table 7
Applications of MABAC for the defence industry.
References | Case study | Methodology | Objective |
(Božanić et al., 2016) | Serbia | TFS-AHP-MABAC | Location selection for preparing laying-up positions used for facilities designed for concealment, protection, and maneuvering of military ships |
(Božanić et al., 2018) | Serbia | TFS-AHP-MABAC | Location selection of deep wading as a means of crossing the river by tanks |
(Bojanic et al., 2018) | Serbia | TFS-AHP-MABAC | Selection of best firing position of the guided anti-tank missile battery in a defence operation |
(Pamučar et al., 2018a) | Serbia | R-BWM-MABAC | Selection of suitable firefighting helicopters |
(Muravev and Mijic, 2020) | Serbia | BWM-MABAC | Provider selection for defence operations |
(Bozanic et al., 2020) | Serbia | Z-FUCOM-MABAC | Location selection of brigade command post during combat operations |
(Bozanic et al., 2020) | Serbia | LBWA-MABAC | Selection of fire position of mortar units |
4.4Education
Education is defined as systematic efforts that society builds up to deliver the knowledge, attitude, value, and skill out of their group members to boost individuals’ potential and changes that occurred in themselves. Therefore, education is the foundation of a society that brings economic wealth, political stability, and social prosperity (Idris et al., 2012). Nowadays, the educational sector has to deal with many challenges such as technology, which comes with its downsides, lack of educational innovation, government policies and spending, educational resources, teaching quality, assessment, and attainment.
Webpages are considered standard information that has been gradually improving over the years. Educational institutions utilize this resource to guarantee that the best quality of information transmission is achieved. According to the importance of university websites’ quality, teaching quality is also an essential factor in evaluating educational institutions. Undergraduate Teaching Audit and Evaluation (UTAE) is a fundamental element of the quality assurance and monitoring system. It examines whether universities have succeeded in their targets or not, which could be regarded as a complicated MCDM problem. The summary of these studies is shown in Table 8.
Table 8
Applications of MABAC for the education sector.
References | Case study | Methodology | Objective |
(Peng and Dai, 2018) | – | SVNLS-TOPSIS | Evaluation of books for the educational sector. |
(Pamučar et al., 2018b) | Bosnia and Herzegovina | R-AHP-MABAC | Evaluation of web pages. |
(Liu and Zhang, 2020) | – | HFS-CCSD-MABAC | Supplier selection for an educational system. |
(Gong et al., 2020) | China | q-ROFS-ITARA-MABAC | Evaluation of teaching audit systems. |
4.5Energy
In this modern world, energy is required for a society’s sustainable development (Amer and Daim, 2011). The global fossil fuel reserves are bounded and unevenly distributed, leading to severe economic and political conflicts. Also, fossil energy availability is not the primary concern; the main problem lies in the impacts caused by the CO2 produced when these massive amounts of remaining fossil fuels are used. Therefore, energy crises and environmental issues have elicited increasing attention. Hence, energy-saving has become necessary.
It is crucial to develop alternative technologies and other energy resources, e.g., renewable energies, gas-to-liquid, biomass-to-liquid, syncrude from oil sands, and waste-to-energy technologies for the production of fuels for different usages. As a result, most countries have developed new energy security policies to reduce energy costs and increase energy sustainability, which plays an essential role in the country’s economic growth and social development, and the quality of people’s lives (Kaiser et al., 2013). Table 9 presents a short review of the MABAC methods for energy management.
Table 9
Applications of MABAC for energy management.
References | Case study | Methodology | Objective |
(Shi et al., 2017) | China | I2TLCM-DEMATEL-MABAC | Health waste disposal technology selection based on several stakeholders |
(Wang et al., 2018) | China | PFS-Weighted | Evaluation of energy performance contracting projects |
(Gigović et al., 2017) | Bonferroni Distance-MABAC | ||
(Adar and Delice, 2019) | Serbia | GIS-DEMATEL-ANP-MABAC | Selection of suitable locations for wind farms |
(Rahim et al., 2020) | Turkey | HFS-MAIRCA-MABAC | Selection of waste treatment alternative for medical waste |
(Zhang et al., 2020) | Malaysia | BNLS-MABAC | Evaluation of renewable sustainable energy |
(Hashemizadeh et al., 2021) | – | P2TLS-MABAC | Selecting the renewable energy power generation project |
(Shi et al., 2017) | – | TFS-ANP-TODIM-COPRAS-GRA | Evaluation of renewable energy investment risk assessment |
4.6Finance & Human Resource Management
Human Resource Financial Management (HRFM) is defined as a framework to develop and propose a new approach to deal with the issues, which remain unanswered by HRM and FM. HRM is defined as a process of placing the right person at the right place and at the right time, while FM is considered the process of making financial decisions based on the data gathered by accounting.
HRM keeps its prime concentration on developing human resources, and financial management can canalize companies’ investments and expenditures to maximize their profits (Chaudhary and Prasad, 2010). The HR and finance departments work towards one ultimate common goal of achieving a higher level of performance and profitability. In today’s competitive environment, the world of HRM is changing more rapidly than can be imagined. Constant environmental changes indicate that HR managers have to deal with constant challenges. They should respond by taking advantage of gradual yet reflective changes in this area’s nature, current practices, and overall HRM policies, mission, and vision.
To address the numerous quantitative and qualitative criteria that influence HRM’s affordability and sustainability, MCDM is a suitable technique that allows the quantitative and qualitative criteria to be incorporated into one evaluation process. Several financial decision problems are MCDM problems, and the tools utilized in the field of MCDM contribute both to the quality of the FM process and the quality of the resulting decisions. A review of related studies is provided in Table 10.
Table 10
Applications of MABAC for finance and human resource management.
References | Case study | Methodology | Objective |
(Peng and Yang, 2016) | – | PS-Choquet Integral Geometric-MABAC | Investment selection to invest in internet companies in the stock market |
(Peng and Dai, 2017) | – | HFS-WASPAS-COPRAS-MABAC | Numerical examples in investment selection |
(Peng et al., 2017) | – | IVFS-EDAS-MABAC | Numerical examples in investment selection |
(Liang et al., 2019b) | China | IFS-MABAC | Employment of outstanding foreign teachers |
Verma (2021) | – | IFS-Entropy-MABAC | Personnel selection in the human resource department |
(Estiri et al., 2020) | Iran | DEMATEL-MABAC | Prioritization of human resource practices |
(Irvanizam et al., 2020) | Indonesia | TFNS-MABAC | Investment selection in one of five technology enterprises |
(Irvanizam et al., 2020) | China | CPT-MABAC | Buying a house |
(Irvanizam et al., 2020) | Serbia | RPR-WASPAS-MABAC | Evaluation of infrastructure projects |
4.7Healthcare
Healthcare is a broad term that pertains to a system that contains the maintenance and improvement of medical services to cater to people’s medical demands. The role of healthcare systems in the quality of life and social welfare in modern society has been well recognized (Hashemkhani Zolfani et al., 2020; Yazdani et al., 2020c). There are many participants involved in healthcare delivery, each having their concerns and interests (Mosadeghrad, 2014). The healthcare system has many sub-systems that operate at different levels (outside of the organization, stakeholders, suppliers, technologies, organization, program level, and point of care), each with specific goals, resources (financial, human, and equipment), and processes (formal and informal). As a result, complexity is increasingly being embraced in healthcare, which brings uncertainties and risks.
In the following, to increase patient safety, health information technology (HIT) has been introduced. HIT indicates numerous opportunities for improving and transforming healthcare systems, including improving clinical outcomes, decreasing human errors, facilitating care coordination, utilizing developed equipment, improving practice efficiencies, and tracking data over time (Alotaibi and Federico, 2017). Patient safety improvement and the quality of healthcare delivery regarding the HIT concept have received considerable attention. Proposing a new algorithm for patient-centered treatment selection, evaluation, and selection of best suppliers for hi-tech medical products and equipment are some areas combined with various MCDM methods to improve and facilitate care coordination. A summary of the evidence of MABAC approach usage in healthcare systems is provided in Table 11.
Table 11
Applications of MABAC for healthcare management.
References | Case study | Methodology | Objective |
(Jia et al., 2019) | China | IFRS-MABAC | Supplier selection for medical devices |
(Wei et al., 2019) | China | PLS-CRITIC-MABAC | Supplier selection of medical consumption products |
(Liu et al., 2019) | China | IVIFS-FEMA-Entropy-MABAC | Risk analysis of radiation therapy in health centres |
(Hu et al., 2019) | China | IVIFS-MABAC | Selection of appropriate medical treatment for patient-centered care |
(Liu et al., 2020c) | China | BFS-SWARA-MABAC | Risk assessment of occupational health and safety measurements |
(Büyüközkan et al., 2020) | Turkey | HFS-SWOT-AHP-MABAC | Evaluation of health tourism strategies |
(Büyüközkan et al., 2020) | – | BWM-MABAC | Facility procurement |
4.8IT & Technology
The business world has been adopting new technologies, which has quickly affected every feature of how businesses operate. Technology has provided an innovative and better approach to manage a business in the last decades, making transactions faster, more effective and more convenient. Information technology (IT) systems emerge in the form of many technologically advanced devices. It helps deliver critical information to managers to make crucial decisions regarding their organization’s operations. It involves studying and applying computers and any type of telecommunications that store, retrieve, analyse, transmit, manipulate, and send information.
Some of the biggest challenges currently are information security, cloud computing, risk management and governance, technology selection, integration and up-gradation, resource management, hiring, communication, fraud monitoring, and business continuity/disaster recovery. Dealing with the above-mentioned technological challenges by making decisions based on various MCDM and MADM methods has been accompanied by significant results. Thanks to these methods, the above-mentioned technological challenges have been successfully addressed.
The proliferation and extensive use of information and communication technologies (ICTs) are revolutionizing the approach people and companies work. They can help people and organizations substantially decrease the cost of their business processes and operations (Taylor, 2015). ICTs contain every communication device and application imaginable, such as phones, computer hardware and software, and web applications. The final goal for a company is to be able to use communication technology productively. An outline of the paramount results of IT and technology studies using the MABAC methods is highlighted in Table 12.
Table 12
Applications of MABAC for IT & Technology.
References | Case study | Methodology | Objective |
(Roy et al., 2016) | India | IT2TFS-MABAC | Evaluation of a software company to hire a software engineer |
(Ji et al., 2018) | China | SVNLS-ELECTRE-MABAC | Outsourcing provider selection for an IT company |
(Luo and Xing, 2019) | China | LNS-BWM-PROMOTHEE-MABAC | Personnel selection for an IT company |
(Mishra et al., 2020b) | – | IFS-MABAC | Evaluation of smart phones |
(Liu et al., 2020a) | – | HFS-MABAC | Security evaluation of marine ecological systems |
(Mishra et al., 2020a) | India | IVIFS-Entropy-MABAC | Assessment of usability and capability of programming languages |
(Mishra et al., 2020a) | – | EAC-GIBWM-MABAC | Selection of data products |
4.9Manufacturing
Manufacturing industries have traditionally played a vital role in countries’ economic development and improvement as they employ the workforce and produce items needed by strategic importance sectors such as national infrastructure and defence (Haraguchi et al., 2017). Manufacturing systems include the manufacturing and processing of items and result in either creation of new goods or in value addition. It is described as broad-spectrum with technological advancements, which primarily focuses on improving and developing productivity by considering the optimal number of materials, technology, methods, machinery, and labour.
Since several manufacturing alternatives are available for each stage, selecting a manufacturing process for any product or service is complicated as different manufacturing processes vary in their performance, productivity, and economics. The selection of convenient alternatives from the group of contrasting and diverse manufacturing system stages can be achieved through MCDM techniques. Therefore, MCDM models take a unique and essential place in manufacturing systems (Ghaleb et al., 2020). Excellence in developing and accomplishing manufacturing processes that prepare unique production capabilities with quality and cost advantages can determine market success and the key to future competitiveness in manufactured products because this strategy cannot be easily duplicated. These motivate the manufacturing companies to struggle along several product dimensions, including design, manufacturing process, utilization of various technologies, methods and tools, and others.
The type of manufacturing process chosen depends on the facility, the staff, and the information systems available. Altogether, while manufacturers worldwide have reached new standards in technology adoption and equipment innovation, many strain points hinder optimization, efficiency, and even safety. These main points lead to risks and uncertainties in manufacturing systems. Table 13 presents a summary of manufacturing studies addressed by the MABAC method.
Table 13
Applications of MABAC for manufacturing.
References | Case study | Methodology | Objective |
(Chatterjee et al., 2017) | India | MABAC | Machining process selection |
(Zhu et al., 2018) | China | IVPFS-Entropy-MABAC | Evaluation and assessment of potential product failure risks |
(Nunić, 2018) | – | FUCOM-MABAC | Assessment of PVC manufacturing centres |
(Can and Toktas, 2018) | Turkey | TFS-DEMATEL-MABAC | Assessment of potential warehouse risks |
(Chakraborty et al., 2020b) | – | R-Entropy-MABAC | Machining process selection |
(Agarwal et al., 2020) | India | DEMATEL-MABAC | Evaluation of jute fibers for manufacturing purposes |
(Bose et al., 2020) | India | ARAS-MABAC | Material selection |
(Bose et al., 2020) | India | BWM-MABAC | Optimizing the wear parameter of CrN/TiAlSiN coating |
4.10Tourism
Tourism is a cultural, societal, and economic factor that involves people’s movement to other countries or locations for individual or professional purposes. It is a multi-aspect commercial activity with immense job creation potential by its labour-intensive nature, revenue creation through tax collection from the hotel sectors, gaining massive foreign exchange and prelation of cross-cultural apprehension and cooperation, business opportunities for entrepreneurs, and economic development of the country (Arshad et al., 2018).
Some of the issues raised in this regard are service quality, tourism destination, ecotourism, marketing, location selection, security, and medical condition. MCDM approaches have been proposed to help experts make the best choices in the tourism industry (Mardani et al., 2016). Table 14 gives a short review of the MABAC methods in the tourism industry.
Table 14
Applications of MABAC for the tourism industry.
References | Case study | Methodology | Objective |
(Gigović et al., 2016) | Serbia | TFS-GIS-DEMATEL-MABAC | Estimation and mapping the suitability classes of ecotourism potential sites |
(Yu et al., 2017) | – | IT2FS-MABAC | Hotel selection using an online tourism platform |
(Roy et al., 2018) | India | R-AHP-MABAC | Location selection for medical tourism sites |
(Roy et al., 2018) | India | IRN-SWARA-MABAC | Evaluation of tourism websites |
4.11Supply Chain & Logistics
A supply chain is defined as the network of all the individuals, organizations, resources, activities, and technologies involved in creating and selling a product or service. It includes everything from the supplier’s delivery of source materials to the manufacturer through its eventual delivery to the end-user. Nowadays, markets have changed drastically with the growth in collaboration between competitors, supply chain partners, outsourcing, integrated supply chain systems, and innovation and technology advancement. As a result, mapping out an supply chain is essential in performing an external analysis in a strategic planning process (Arawati, 2011).
The global marketplace provides tremendous opportunities for companies to reach strategic competitiveness through effective SCM. Companies have been increasingly interested in efficient SCM because they have met extreme competition due to decreased product life cycle time, varying customer demands, and rising manufacturing and transportation costs. This made companies recognize the vital role of SCM to reach organizational purposes via speeding up innovations and product launching to the dynamic market, choosing the most appropriate supplier, green supply chain, improving customer value, optimizing the use of resources, reducing different types of costs such as production, inventory, transportation, and finally enhancing profitability (Moosivand et al., 2019). SCM challenges are MCDM issues because, in the entire supply chain cycle, various criteria related to each sub-criterion of the supply chain cycle should be investigated. To manage the entire supply chain, each criterion’s relationship should be identified, which impacts the performance of the supply chain. Finally, based on the criteria determined, decisions should be made. Therefore, decision-making is critical in managing the supply chain cycle, and SCM is an MCDM problem (Khan et al., 2018).
The selection of potential suppliers has been known as one of the essential concerns that companies face while maintaining a strategically competitive position. Therefore, it is necessary to commit to fair assessment and supplier selection during the phase of purchasing, which can primarily affect the product’s final price. Traditionally, supply chain was primarily considered based on economic aspects, but companies are becoming much more concerned about environmental problems. Green Supply Chain Management (GSCM) with environmental protection concepts increases attention from practitioners and researchers (Mishra et al., 2019).
As mentioned before, material selection has been known as one of the crucial stages in the designing phase in SCM. Evaluation and selection of the optimum material for a product are crucial for companies to survive in today’s fiercely competitive environment. Xue et al. (2016) believed that material selection problems in product design could be considered within the framework of MCDM. They introduced a novel approach based on IVIFSs and MABAC methods for handling material selection problems with incomplete weight information.
Logistics have been known as the backbone of global trade, consisting of a network of transportation, warehousing, and inventory of raw materials and goods. Logistics process management has always been a complicated task, but with time it becomes even more labourious. Some key challenges facing the logistics process are as followed: choosing the most appropriate transportation system, reduction of transport costs, compliance with regulations, streamlining operations, offering segmented, personalized services and workforce management.
There are also several aspects of decision-making in the transportation system, such as determining clean technology vehicles. Besides, the mobility sector containing all kinds of transportation systems is dealing with global challenges regarding green environmental issues. It is also worth considering that smart customers do not always make their purchasing decisions using structured cost comparison approaches and are concerned with new technologies that inherently raise fuel efficiency and reduce Greenhouse Gas (GHG) emissions such as CO2 and better air quality. Some of these studies of the MABAC for supply chain and logistics are highlighted in Table 15.
Table 15
Applications of MABAC for supply chain & logistics.
References | Case study | Methodology | Objective |
(Pamučar and Ćirović, 2015) | – | TFS-DEMATEL-MABAC | Logistics and handling resources selection |
(Xue et al., 2016) | China | IVIFS-Linear Programming-MABAC | Material selection for the automobile industry |
(Sharma et al., 2018) | India | R-AHP-MABAC | Evaluation of railway stations |
(Vasiljević et al., 2018) | – | R-AHP-MABAC | Supplier selection in the automobile industry |
(Milosavljević et al., 2018) | Serbia | TOPSIS-ELECTRE-MABAC | Location selection of railroad container terminals |
(Vesković et al., 2018) | Bosnia and Herzegovina | SWARA-MABAC | Evaluation of railway management strategies and models |
(Petrović et al., 2018) | Serbia | MABAC | Logistics policy selection |
(Biswas and Das, 2018) | India | Entropy-MABAC | Hybrid electric vehicle selection |
(Luo and Liang, 2019) | China | LNS-MABAC | Evaluation of roadway support schemes |
(Noureddine and Ristic, 2019) | Serbia | FUCOM-TOPSIS-MABAC | Route selection for transportation of hazardous materials |
(Biswas and Das, 2019) | India | TFS-AHP-MABAC | Assessment of electric vehicles |
(Gupta et al., 2019) | India | TFS-AHP-TOPSIS-WASPAS-MABAC | Green supplier selection in automobile industry |
(Pamucar et al., 2019) | – | R-BWM-WASPAS-MABAC | Evaluation of third-party logistics provider |
(Xu et al., 2019) | China | TFS-MABAC, HFS-MABAC | Green supplier selection for a manufacturing centre |
(Chakraborty et al., 2020a) | India | BWM-MABAC | Evaluation of international airports |
(Liu et al., 2020b) | China | LSFS-TODIM-MABAC | Evaluation of public bicycles |
(Yazdani et al., 2020) | Spain | R-DEMATEL-MABAC | Evaluation of freight systems |
(Wei et al., 2020b) | China | PLS-Entropy-MABAC | Green supplier selection for the retail industry |
(Biswas, 2020) | India | PIPRECIA-MABAC | Measurement of supply chain financial metrics |
(Fan et al., 2020) | China | Z-Entropy-MABAC | Evaluation of third-party logistics provider |
(Wei et al., 2020a) | China | PLS-Entropy-MABAC | Green supplier selection |
(Liu and Cheng, 2020) | China | PMVNS-MABAC | Logistics provider selection |
(Liu and Cheng, 2020) | Egypt | BWM-PROMETHEE II-MABAC | Drug Product Selection |
(Liu and Cheng, 2020) | Turkey | SWARA-MABAC | Evaluation of logistics villages |
(Pamučar and Ćirović, 2015) | Iran | DANP-MABAC | Green Supplier Selection |
5Discussion
MCDM approaches in form of weighting and ranking techniques have attracted a lot of attention from different sectors, specifically the sectors which are directly connected to sustainable development and circular economy. In current societies, achieving SDGs within the Agenda 2030 is considered among the top and highly prioritized governmental and non-governmental organizations in order to meet the required standards. However, the complexity and multi-dimensionality of the problems related to sustainability and circularity create a need for reliable and robust tools to generate reliable solutions, otherwise not only the sustainability and circularity goals would not be met, but also severe and costly consequences may occur. On the other hand, not all relevant problems can be addressed quantitatively; therefore, the utilization of qualitative-based techniques with a user-friendly environment for decision-makers from different sectors is of high significance. MABAC method, as a ranking MCDM approach, is one of the well-established MCDM techniques that can be used for a broad range of sustainability and circularity problems.
According to the results of the review, MABAC technique and its extensions have shown a promising performance in various application areas. As mentioned in Section 2, MABAC provides three main advantages including providing consistent results in the event of a change in the units of measurement, stable solutions in the event of a change in the type of criteria formulation, and a simplified algorithm suitable for large-scale problems. However, MABAC can be improved considering its structural limitations. One of the serious shortcomings in the traditional form of MABAC is its normalization technique which is based on a max-min normalization formula. However, using a single normalization technique may lead to biased solutions. Recently, novel methods such as mulTi-noRmalization mUlti-distance aSsessmenT (TRUST) (Torkayesh and Deveci, 2021) and Double Normalization-based Multiple Aggregation (DNMA) (Liao and Wu, 2020) were developed to mitigate the biasedness of single normalization techniques. Therefore, an improved version of the MABAC can be developed by considering multiple normalization techniques. On the other hand, the complexity of real-life problems and restricted choices based on governmental or non-governmental standards are other concerns that can improve the traditional MABAC. One of the possible ways to empower the traditional MABAC is the utilization of constrained-based criteria in order to include real-life standards within the computations (Abdelli et al., 2019).
The findings of Section 4 represent how successful MABAC has been considering its applicability for diverse application areas. In recent years, sustainable development and circular economy have become very broad terms addressing various types of problems in many application areas. Several key application areas still exist in that MABAC can play a key role in addressing multi-dimensional problems. Transportation and fuel planning are two important application areas that strongly contribute to sustainability and circularity targets related to GHG emissions, and batteries of electric vehicles. To support circularity, MABAC can be used in various decision-making related to recycling and remanufacturing facilities which can mitigate environmental and social impacts and increase economic benefits. Moreover, social sustainability and its targets regarding education, equity, employment, and healthcare can be other application areas in which MABAC can be applied.
6Conclusions
The emergence of concepts like sustainable development and circular economy has raised the need for multi-dimensional complex decision-making tools to enhance the capability of the decision-making process. Recent decades have been known as the golden era of developing MCDM methods in a different form under various uncertainty sets to tackle important complex problems. MABAC is one of the most frequently used methods in different applications over the last 5 years. In this paper, we comprehensively reviewed the development and applications of the MABAC method through its developments and applications. Reviewed papers were categorized into eleven application areas. Apart from application categorization, all papers were systematically reviewed based on different metrics such as publication year, authors, combined methodology, uncertainty set, case study, and journals. A large proportion of papers, 30 out of 117, were published in 2021 in international journals. Authors from China published more than other countries. Journal of “Decision-making: Applications in Management and Engineering” published 10 papers and ranked as the top journal publishing MABAC studies. BWM and AHP entropy are two frequently integrated MCDM models with the MABAC method. Most of the uncertain MABAC studies were implemented under triangular fuzzy sets. Finally, findings indicate that supply chain & logistics is the most important target for decision-making problems solved with a different form of MABAC with 36 papers and a total of 844 Google Scholar citations.
This review represented important points about past development and application of the MABAC method. However, there are manifold ways to both extend the MABAC method and apply it based on the world’s current uncertain and complex environments. Important fields that emerged in recent years are sustainable development and circular economy which can be dealt with MABAC to develop and run expert decision-making models. Waste management is one of the important areas in both concepts of sustainability and circular economy which can be addressed using MABAC. On the other hand, transportation planning, fuel planning, and climate change strategy development are important applications strongly contributing to sustainable development and circular economy where MABAC can be used. Moreover, MABAC has been developed under different fuzzy-based uncertainty sets; however, there exists no study to consider uncertainty in the decision-making environment and the impact of future events on the its process. The concept of stratification is one of the newly introduced topics that can be used within the MABAC method to enhance its capability in making reliable decisions (Pamucar et al., 2023; Torkayesh et al., 2022). In the same context, most of the previous uncertainty sets focused on the incorporation of the uncertainty related to the decision-makers’ opinions. However, all decisions may not be directly implemented based on their opinions whereas experts’ judgments over the decision-makers’ opinions play an important role in finalizing the decision. For this purpose, MABAC can be improved by an extension under extended Z-numbers (Tian et al., 2021) where experts’ judgments on the decision-makers’ opinions are considered in the decision-making process for more reliable and robust solutions. Another important future direction is related to the integration of the MABAC method with big data tools in order to develop big data decision support systems for various complex problems (Maghsoodi et al., 2023; Simic et al., 2022). Finally, the integration of the MABAC method with probabilistic linguistic information is anticipated to be investigated since it is natural for decision-makers to employ probabilistic linguistic information (Liao et al., 2020).
References
1 | Abdelli, A., Hammal, Y., Mokdad, L. ((2019) ). ISOCOV: a new MCDM method to handle value constraints in Web service selection. In: 2019 IEEE Symposium on Computers and Communications (ISCC), Barcelona, Spain, 2019, pp. 1022–1027. https://doi.org/10.1109/ISCC47284.2019.8969589. |
2 | Adar, T., Delice, E.K. ((2019) ). New integrated approaches based on MC-HFLTS for healthcare waste treatment technology selection. Journal of Enterprise Information Management, 32: (4), 688–711. |
3 | Agarwal, S., Chakraborty, S., Chakraborty, S. ((2020) ). A DEMATEL-MABAC-based approach for grading and evaluation of jute fibers. Research Journal of Textile and Apparel, 24: (4), 341–355. |
4 | Al-Werikat, D. ((2017) ). Supply chain management in construction revealed. International Journal of Scientific & Technology Research, 6: , 106–110. |
5 | Ali, S.M., Paul, S.K., Chowdhury, P., Agarwal, R., Fathollahi-Fard, A.M., Jabbour, C.J.C., Luthra, S. ((2021) ). Modelling of supply chain disruption analytics using an integrated approach: an emerging economy example. Expert Systems with Applications, 173: , 114690. |
6 | Alotaibi, Y.K., Federico, F. ((2017) ). The impact of health information technology on patient safety. Saudi Medical Journal, 38: (12), 1173. |
7 | Ambros, C.C. ((2017) ). Defense and development industry: theoretical controversies and implications in industrial policy. Austral: Brazilian Journal of Strategy & International Relations, 6: (11), 132–153. |
8 | Amer, M., Daim, T.U. ((2011) ). Selection of renewable energy technologies for a developing county: a case of Pakistan. Energy for Sustainable Development, 15: (4), 420–435. |
9 | Arawati, A. ((2011) ). Supply chain management, supply chain flexibility and business performance. Journal of Global Strategic Management, 9: (1), 134–145. |
10 | Arshad, M.I., Iqbal, M.A., Shahbaz, M. ((2018) ). Pakistan tourism industry and challenges: a review. Asia Pacific Journal of Tourism Research, 23: (2), 121–132. |
11 | Biswas, S. ((2020) ). Measuring performance of healthcare supply chains in India: a comparative analysis of multi-criteria decision making methods. Decision Making: Applications in Management and Engineering, 3: (2), 162–189. |
12 | Biswas, T., Das, M. ((2018) ). Selection of hybrid vehicle for green environment using multi-attributive border approximation area comparison method. Management Science Letters, 8: (2), 121–130. |
13 | Biswas, T.K., Das, M.C. ((2019) ). Selection of commercially available electric vehicle using fuzzy AHP-MABAC. Journal of The Institution of Engineers (India): Series C, 100: (3), 531–537. |
14 | Biswas, S., Pamucar, D., Kar, S. ((2022) ). A preference-based comparison of select over-the-top video streaming platforms with picture fuzzy information. International Journal of Communication Networks and Distributed Systems, 28: (4), 414–458. |
15 | Bojanic, D., Kovač, M., Bojanic, M., Ristic, V. ((2018) ). Multi-criteria decision-making in A defensive operation of the guided anti-tank missile battery: an example of the hybrid model fuzzy AHP-MABAC. Decision Making: Applications in Management and Engineering, 1: (1), 51–66. |
16 | Bose, S., Mandal, N., Nandi, T. ((2020) ). Comparative and experimental study on hybrid metal matrix composites using additive ratio assessment and multi-attributive border approximation area comparison methods varying the different weight percentage of the reinforcements. Materials Today: Proceedings, 22: , 1745–1754. |
17 | Božanić, D., Pamučar, D., Karović, S. ((2016) ). Use of the fuzzy AHP-MABAC hybrid model in ranking potential locations for preparing laying-up positions. Vojnotehnički glasnik, 64: (3), 705–729. |
18 | Božanić, D., Tešić, D., Milićević, J. ((2018) ). A hybrid fuzzy AHP-MABAC model: application in the Serbian Army–The selection of the location for deep wading as a technique of crossing the river by tanks. Decision Making: Applications in Management and Engineering, 1: (1), 143–164. |
19 | Božanić, D., Tešić, D., Kočić, J. ((2019) ). Multi-criteria FUCOM–Fuzzy MABAC model for the selection of location for construction of single-span bailey bridge. Decision Making: Applications in Management and Engineering, 2: (1), 132–146. |
20 | Bozanic, D., Tešić, D., Milić, A. ((2020) ). Multi-criteria decision making model with Z-numbers based on FUCOM and MABAC model. Decision Making: Applications in Management and Engineering, 3: (2), 19–36. |
21 | Büyüközkan, G., Mukul, E., Kongar, E. ((2020) ). Health tourism strategy selection via SWOT analysis and integrated hesitant fuzzy linguistic AHP-MABAC approach. Socio-Economic Planning Sciences, 74: , 100929. |
22 | Can, G.F., Toktas, P. ((2018) ). A novel fuzzy risk matrix based risk assessment approach. Kybernetes, 47: (9), 1721–1751. |
23 | Carayannis, E.G., Ferreira, J.J., Jalali, M.S., Ferreira, F.A. ((2018) ). MCDA in knowledge-based economies: methodological developments and real world applications. Technological Forecasting and Social Change, 131: , 1–3. |
24 | Chakraborty, S., Dandge, S.S., Agarwal, S. ((2020) a). Non-traditional machining processes selection and evaluation: a rough multi-attributive border approximation area comparison approach. Computers & Industrial Engineering, 139: , 106201. |
25 | Chakraborty, S., Ghosh, S., Sarker, B., Chakraborty, S. ((2020) b). An integrated performance evaluation approach for the Indian international airports. Journal of Air Transport Management, 88: , 101876. |
26 | Chatterjee, P., Mondal, S., Boral, S., Banerjee, A., Chakraborty, S. ((2017) ). A novel hybrid method for non-traditional machining process selection using factor relationship and multi-attributive border approximation method. Facta Universitatis, Series: Mechanical Engineering, 15: (3), 439–456. |
27 | Chatterjee, K., Zavadskas, E.K., Tamošaitienė, J., Adhikary, K., Kar, S. ((2018) ). A hybrid MCDM technique for risk management in construction projects. Symmetry, 10: (2), 46. |
28 | Chaudhary, A., Prasad, S. ((2010) ). Human resource financial management. International Journal of Trade, Economics and Finance, 1: (4), 342. |
29 | Colapinto, C., Jayaraman, R., Abdelaziz, F.B., La Torre, D. ((2019) ). Environmental sustainability and multifaceted development: multi-criteria decision models with applications. Annals of Operations Research, 293: (2), 405–432. |
30 | Debnath, A., Roy, J., Kar, S., Zavadskas, E.K., Antucheviciene, J. ((2017) ). A hybrid MCDM approach for strategic project portfolio selection of agro by-products. Sustainability, 9: (8), 1302. |
31 | Estiri, M., Dahooie, J.H., Vanaki, A.S., Banaitis, A., Binkytė-Vėlienė, A. ((2020) ). A multi-attribute framework for the selection of high-performance work systems: the hybrid DEMATEL-MABAC model. Economic Research-Ekonomska Istraživanja, 34: (1), 970–997. |
32 | Fan, J., Guan, R., Wu, M. ((2020) ). Z-MABAC method for the selection of third-party logistics suppliers in fuzzy environment. IEEE Access, 8: , 199111–199119. |
33 | Ghaleb, A.M., Kaid, H., Alsamhan, A., Mian, S.H., Hidri, L. ((2020) ). Assessment and comparison of various MCDM approaches in the selection of manufacturing process. Advances in Materials Science and Engineering, 2020: , 4039253. |
34 | Gigović, L., Pamučar, D., Lukić, D., Marković, S. ((2016) ). GIS-Fuzzy DEMATEL MCDA model for the evaluation of the sites for ecotourism development: a case study of “Dunavski ključ” region, Serbia. Land Use Policy, 58: , 348–365. |
35 | Gigović, L., Pamučar, D., Božanić, D., Ljubojević, S. ((2017) ). Application of the GIS-DANP-MABAC multi-criteria model for selecting the location of wind farms: a case study of Vojvodina. Serbia. Renewable Energy, 103: , 501–521. |
36 | Golfam, P., Ashofteh, P.-S., Rajaee, T., Chu, X. ((2019) ). Prioritization of water allocation for adaptation to climate change using multi-criteria decision making (MCDM). Water Resources Management, 33: (10), 3401–3416. |
37 | Gong, J.W., Li, Q., Yin, L., Liu, H.C. ((2020) ). Undergraduate teaching audit and evaluation using an extended MABAC method under q-rung orthopair fuzzy environment. International Journal of Intelligent Systems, 35: (12), 1912–1933. |
38 | Gupta, S., Soni, U., Kumar, G. ((2019) ). Green supplier selection using multi-criterion decision making under fuzzy environment: a case study in automotive industry. Computers & Industrial Engineering, 136: , 663–680. |
39 | Haleh, H., Hamidi, A. ((2011) ). A fuzzy MCDM model for allocating orders to suppliers in a supply chain under uncertainty over a multi-period time horizon. Expert Systems with Applications, 38: (8), 9076–9083. |
40 | Haraguchi, N., Cheng, C.F.C., Smeets, E. ((2017) ). The importance of manufacturing in economic development: has this changed? World Development, 93: , 293–315. |
41 | Hashemizadeh, A., Ju, Y., Bamakan, S.M.H., Le, H.P. ((2021) ). Renewable energy investment risk assessment in belt and road initiative countries under uncertainty conditions. Energy, 214: , 118923. |
42 | Hashemkhani Zolfani, S., Yazdani, M., Ebadi Torkayesh, A., Derakhti, A. ((2020) ). Application of a gray-based decision support framework for location selection of a temporary hospital during COVID-19 pandemic. Symmetry, 12: (6), 886. |
43 | Hu, J., Chen, P., Yang, Y. ((2019) ). An interval type-2 fuzzy similarity-based MABAC approach for patient-centered care. Mathematics, 7: (2), 140. |
44 | Idris, F., Hassan, Z., Ya’acob, A., Gill, S.K., Awal, N.A.M. ((2012) ). The role of education in shaping youth’s national identity. Procedia-Social and Behavioral Sciences, 59: , 443–450. |
45 | Irvanizam, I., Zi, N.N., Zuhra, R., Amrusi, A., Sofyan, H. ((2020) ). An extended MABAC method based on triangular fuzzy neutrosophic numbers for multiple-criteria group decision making problems. Axioms, 9: (3), 104. |
46 | Jafari Shalamzari, M., Zhang, W., Gholami, A., Zhang, Z. ((2019) ). Runoff harvesting site suitability analysis for wildlife in sub-desert regions. Water, 11: (9). 1944. |
47 | Ji, P., Zhang, H-y., Wang, J-q. ((2018) ). Selecting an outsourcing provider based on the combined MABAC–ELECTRE method using single-valued neutrosophic linguistic sets. Computers & Industrial Engineering, 120: , 429–441. |
48 | Jia, F., Liu, Y., Wang, X. ((2019) ). An extended MABAC method for multi-criteria group decision making based on intuitionistic fuzzy rough numbers. Expert Systems with Applications, 127: , 241–255. |
49 | Kaiser, P., Unde, R.B., Kern, C., Jess, A. ((2013) ). Production of liquid hydrocarbons with CO2 as carbon source based on reverse water-gas shift and Fischer-Tropsch synthesis. Chemie Ingenieur Technik, 85: (4), 489–499. |
50 | Karaşan, A., Kaya, İ., Erdoğan, M., Özkan, B., Çolak, M. ((2019) ). Evaluation of defense strategies by using a MCDM methodology based on neutrosophic sets: a case study for Turkey. In: International Conference on Intelligent and Fuzzy Systems. |
51 | Khan, S.A., Chaabane, A., Dweiri, F.T. (2018). Multi-criteria decision-making methods application in supply chain management: a systematic literature. Multi-Criteria Methods and Techniques Applied to Supply Chain Management. |
52 | Lahri, V., Shaw, K., Ishizaka, A. ((2021) ). Sustainable supply chain network design problem: using the integrated BWM, TOPSIS, possibilistic programming, and ε-constrained methods. Expert Systems with Applications, 168: , 114373. |
53 | Liang, R-x., He, S-s., Wang, J-q., Chen, K., Li, L. ((2019) a). An extended MABAC method for multi-criteria group decision-making problems based on correlative inputs of intuitionistic fuzzy information. Computational and Applied Mathematics, 38: (3), 112. |
54 | Liang, W., Zhao, G., Wu, H., Dai, B. ((2019) b). Risk assessment of rockburst via an extended MABAC method under fuzzy environment. Tunnelling and Underground Space Technology, 83: , 533–544. |
55 | Liang, X., Teng, F., Sun, Y. ((2020) ). Multiple group decision making for selecting emergency alternatives: a novel method based on the LDWPA operator and LD-MABAC. International Journal of Environmental Research and Public Health, 17: (8), 2945. |
56 | Liao, H., Wu, X. ((2020) ). DNMA: a double normalization-based multiple aggregation method for multi-expert multi-criteria decision making. Omega, 94: , 102058. |
57 | Liao, H., Mi, X., Xu, Z. ((2020) ). A survey of decision-making methods with probabilistic linguistic information: bibliometrics, preliminaries, methodologies, applications and future directions. Fuzzy Optimization and Decision Making, 19: (1), 81–134. |
58 | Liu, H.-C., You, J.-X., Duan, C.-Y. ((2019) ). An integrated approach for failure mode and effect analysis under interval-valued intuitionistic fuzzy environment. International Journal of Production Economics, 207: , 163–172. |
59 | Liu, P., Cheng, S. ((2020) ). An improved MABAC group decision-making method using regret theory and likelihood in probability multi-valued neutrosophic sets. International Journal of Information Technology & Decision Making (IJITDM), 19(05): , 1353–1387. |
60 | Liu, P., Zhang, P. ((2020) ). A normal wiggly hesitant fuzzy MABAC method based on CCSD and prospect theory for multiple attribute decision making. International Journal of Intelligent Systems, 36: (1), 447–477. |
61 | Liu, P., Xu, H., Pedrycz, W. ((2020) a). A normal wiggly hesitant fuzzy linguistic projection-based multiattributive border approximation area comparison method. International Journal of Intelligent Systems, 35: (3), 432–469. |
62 | Liu, P., Zhu, B., Wang, P., Shen, M. ((2020) b). An approach based on linguistic spherical fuzzy sets for public evaluation of shared bicycles in China. Engineering Applications of Artificial Intelligence, 87: , 103295. |
63 | Liu, R., Hou, L.-X., Liu, H.-C., Lin, W. ((2020) c). Occupational health and safety risk assessment using an integrated SWARA-MABAC model under bipolar fuzzy environment. Computational and Applied Mathematics, 39: (4), 1–17. |
64 | Luo, S.-Z., Liang, W.-Z. ((2019) ). Optimization of roadway support schemes with likelihood-based MABAC method. Applied Soft Computing, 80: , 80–92. |
65 | Luo, S.-Z., Xing, L.-N. ((2019) ). A hybrid decision making framework for personnel selection using BWM, MABAC and PROMETHEE. International Journal of Fuzzy Systems, 21: (8), 2421–2434. |
66 | Machado, C., Davim, J.P. ((2020) ). Circular Economy and Engineering: A New Ecologically Efficient Model. Springer Nature. |
67 | Maghsoodi, A.I., Torkayesh, A.E., Wood, L.C., Herrera-Viedma, E., Govindan, K. ((2023) ). A machine learning driven multiple criteria decision analysis using LS-SVM feature elimination: sustainability performance assessment with incomplete data. Engineering Applications of Artificial Intelligence, 119: , 105785. |
68 | Mardani, A., Jusoh, A., Zavadskas, E.K., Kazemilari, M., ((2016) ). Application of multiple criteria decision making techniques in tourism and hospitality industry: a systematic review. Transformations in Business & Economics, 15: (1), 192–213. |
69 | Mardani, A., Kannan, D., Hooker, R.E., Ozkul, S., Alrasheedi, M., Tirkolaee, E.B. ((2020) ). Evaluation of green and sustainable supply chain management using structural equation modelling: a systematic review of the state of the art literature and recommendations for future research. Journal of Cleaner Production, 249: , 119383. |
70 | Milosavljević, M., Bursać, M., Tričković, G. ((2018) ). Selection of the railroad container terminal in Serbia based on multi criteria decision making methods. Decision Making: Applications in Management and Engineering, 1: (2), 1–15. |
71 | Mishra, A.R., Rani, P., Pardasani, K.R., Mardani, A. ((2019) ). A novel hesitant fuzzy WASPAS method for assessment of green supplier problem based on exponential information measures. Journal of Cleaner Production, 238: , 117901. |
72 | Mishra, A.R., Chandel, A., Motwani, D. ((2020) a). Extended MABAC method based on divergence measures for multi-criteria assessment of programming language with interval-valued intuitionistic fuzzy sets. Granular Computing, 5: (1), 97–117. |
73 | Mishra, A.R., Garg, A.K., Purwar, H., Rana, P., Liao, H., Mardani, A. ((2020) b). An extended intuitionistic fuzzy multi-attributive border approximation area comparison approach for Smartphone selection using discrimination measures. Informatica, 32: (1), 119–143. |
74 | Moosivand, A., Ghatari, A.R., Rasekh, H.R. ((2019) ). Supply chain challenges in pharmaceutical manufacturing companies: using qualitative system dynamics methodology. Iranian Journal of Pharmaceutical Research: IJPR, 18: (2), 1103. |
75 | Mosadeghrad, A.M. ((2014) ). Factors influencing healthcare service quality. International Journal of Health Policy and Management, 3: (2), 77. |
76 | Muravev, D., Mijic, N. ((2020) ). A novel integrated provider selection multi-criteria model: the BWM-MABAC model. Decision Making: Applications in Management and Engineering, 3: (1), 60–78. |
77 | Nocca, F., Girard, L.F. ((2018) ). Circular city model and its implementation: towards an integrated evaluation tool. BDC. Bollettino Del Centro Calza Bini, 18: (1), 11–32. |
78 | Nordhaus, W.D. ((1991) ). To slow or not to slow: the economics of the greenhouse effect. The Economic Journal, 101: (407), 920–937. |
79 | Noureddine, M., Ristic, M. ((2019) ). Route planning for hazardous materials transportation: multi-criteria decision making approach. Decision Making: Applications in Management and Engineering, 2: (1), 66–85. |
80 | Nunić, Z. ((2018) ). Evaluation and selection of the PVC carpentry manufacturer using the FUCOM-MABAC model. Operational Research in Engineering Sciences: Theory and Applications, 1: (1), 13–28. |
81 | Pamucar, D., Chatterjee, K., Zavadskas, E.K. ((2019) ). Assessment of third-party logistics provider using multi-criteria decision-making approach based on interval rough numbers. Computers & Industrial Engineering, 127: , 383–407. |
82 | Pamucar, D., Yazdani, M., Obradovic, R., Kumar, A., Torres-Jiménez, M. ((2020) ). A novel fuzzy hybrid neutrosophic decision-making approach for the resilient supplier selection problem. International Journal of Intelligent Systems, 35: (12), 1934–1986. |
83 | Pamucar, D., Gokasar, I., Torkayesh, A.E., Deveci, M., Martínez, L., Wu, Q. ((2023) ). Prioritization of unmanned aerial vehicles in transportation systems using the integrated stratified fuzzy rough decision-making approach with the hamacher operator. Information Sciences, 622: , 374–404. |
84 | Pamučar, D., Ćirović, G. ((2015) ). The selection of transport and handling resources in logistics centers using multi-attributive border approximation area comparison (MABAC). Expert Systems with Applications, 42: (6), 3016–3028. |
85 | Pamučar, D., Petrović, I., Ćirović, G. ((2018) a). Modification of the Best–Worst and MABAC methods: a novel approach based on interval-valued fuzzy-rough numbers. Expert Systems with Applications, 91: , 89–106. |
86 | Pamučar, D., Stević, Ž., Zavadskas, E.K. ((2018) b). Integration of interval rough AHP and interval rough MABAC methods for evaluating university web pages. Applied Soft Computing, 67: , 141–163. |
87 | Peng, X., Yang, Y. ((2016) ). Pythagorean fuzzy Choquet integral based MABAC method for multiple attribute group decision making. International Journal of Intelligent Systems, 31: (10), 989–1020. |
88 | Peng, X., Dai, J. ((2017) ). Hesitant fuzzy soft decision making methods based on WASPAS, MABAC and COPRAS with combined weights. Journal of Intelligent & Fuzzy Systems, 33: (2), 1313–1325. |
89 | Peng, X., Dai, J. ((2018) ). Approaches to single-valued neutrosophic MADM based on MABAC, TOPSIS and new similarity measure with score function. Neural Computing and Applications, 29: (10), 939–954. |
90 | Peng, X., Dai, J., Yuan, H. ((2017) ). Interval-valued fuzzy soft decision making methods based on MABAC, similarity measure and EDAS. Fundamenta Informaticae, 152: (4), 373–396. |
91 | Pérez-Gladish, B., Ferreira, F.A., Zopounidis, C. ((2020) ). MCDM/A studies for economic development, social cohesion and environmental sustainability: introduction. International Journal of Sustainable Development & World Ecology, 28: (1), 1–3. |
92 | Petrović, G.S., Madić, M., Antucheviciene, J. ((2018) ). An approach for robust decision making rule generation: solving transport and logistics decision making problems. Expert Systems with Applications, 106: , 263–276. |
93 | Qureshi, M.R.N., Singh, R.K., Hasan, M.A. ((2018) ). Decision support model to select crop pattern for sustainable agricultural practices using fuzzy MCDM. Environment, Development and Sustainability, 20: (2), 641–659. |
94 | Rahim, N., Abdullah, L., Yusoff, B. ((2020) ). A border approximation area approach considering bipolar neutrosophic linguistic variable for sustainable energy selection. Sustainability, 12: (10), 3971. |
95 | Rani, P., Mishra, A.R., Krishankumar, R., Ravichandran, K., Kar, S. ((2021) ). Multi-criteria food waste treatment method selection using single-valued neutrosophic-CRITIC-MULTIMOORA framework. Applied Soft Computing, 111: , 107657. |
96 | Roy, J., Ranjan, A., Debnath, A., Kar, S. (2016). An extended Multi Attributive Border Approximation area Comparison using interval type-2 trapezoidal fuzzy numbers. arXiv:1607.01254. |
97 | Roy, J., Chatterjee, K., Bandyopadhyay, A., Kar, S. ((2018) ). Evaluation and selection of medical tourism sites: a rough analytic hierarchy process based multi-attributive border approximation area comparison approach. Expert Systems, 35: (1), e12232. |
98 | Schatteman, D., Herroelen, W., Van de Vonder, S., Boone, A. ((2008) ). Methodology for integrated risk management and proactive scheduling of construction projects. Journal of Construction Engineering and Management, 134: (11), 885–893. |
99 | Sharma, H.K., Roy, J., Kar, S., Prentkovskis, O. ((2018) ). Multi criteria evaluation framework for prioritizing Indian railway stations using modified rough ahp-mabac method. Transport and Telecommunication Journal, 19: (2), 113–127. |
100 | Shi, H., Liu, H.-C., Li, P., Xu, X.-G. ((2017) ). An integrated decision making approach for assessing healthcare waste treatment technologies from a multiple stakeholder. Waste Management, 59: , 508–517. |
101 | Simic, V., Ebadi Torkayesh, A., Ijadi Maghsoodi, A. (2022). Locating a disinfection facility for hazardous healthcare waste in the COVID-19 era: a novel approach based on Fermatean fuzzy ITARA-MARCOS and random forest recursive feature elimination algorithm. Annals of Operations Research, 1–46. |
102 | Sousa, M., Almeida, M.F., Calili, R. ((2021) ). Multiple criteria decision making for the achievement of the UN sustainable development goals: a systematic literature. Review and a Research Agenda. Sustainability, 13: (8), 4129. |
103 | Taylor, P. (2015). The importance of information and communication technologies (ICTs): an integration of the extant literature on ICT adoption in small and medium enterprises. International Journal of Economics, Commerce and Management, 3(5). |
104 | Tian, Y., Mi, X., Ji, Y., Kang, B. ((2021) ). ZE-numbers: a new extended Z-numbers and its application on multiple attribute group decision making. Engineering Applications of Artificial Intelligence, 101: , 104225. |
105 | Torkayesh, A.E., Deveci, M. ((2021) ). A mulTi-noRmalization mUlti-distance aSsessmenT (TRUST) approach for locating a battery swapping station for electric scooters. Sustainable Cities and Society, 74: , 103243. |
106 | Torkayesh, A.E., Malmir, B., Asadabadi, M.R. ((2021) a). Sustainable waste disposal technology selection: the stratified best-worst multi-criteria decision-making method. Waste Management, 122: , 100–112. |
107 | Torkayesh, A.E., Zolfani, S.H., Kahvand, M., Khazaelpour, P. (2021b). Landfill location selection for healthcare waste of urban areas using hybrid BWM-grey MARCOS model based on GIS. Sustainable Cities and Society, 102712. |
108 | Torkayesh, A.E., Yazdani, M., Ribeiro-Soriano, D. ((2022) ). Analysis of industry 4.0 implementation in mobility sector: an integrated approach based on QFD, BWM, and stratified combined compromise solution under fuzzy environment. Journal of Industrial Information Integration, 30: , 100406. |
109 | Van Eck, N., Waltman, L. ((2010) ). Software survey: VOSviewer, a computer program for bibliometric mapping. Scientometrics, 84: (2), 523–538. |
110 | Vasiljević, M., Fazlollahtabar, H., Stević, Ž., Vesković, S. ((2018) ). A rough multi-criteria approach for evaluation of the supplier criteria in automotive industry. Decision Making: Applications in Management and Engineering, 1: (1), 82–96. |
111 | Verma, R. ((2021) ) On intuitionistic fuzzy order-α divergence and entropy measures with MABAC method for multiple attribute group decision-making. Journal of Intelligent & Fuzzy Systems, 40: (1), 1191–1217. |
112 | Vesković, S., Stević, Ž., Stojić, G., Vasiljević, M., Milinković, S. ((2018) ). Evaluation of the railway management model by using a new integrated model DELPHI-SWARA-MABAC. Decision Making: Applications in Management and Engineering, 1: (2), 34–50. |
113 | Wang, H.-F., Hsu, H.-W. ((2012) ). A possibilistic approach to the modeling and resolution of uncertain closed-loop logistics. Fuzzy Optimization and Decision Making, 11: (2), 177–208. |
114 | Wang, J., Wei, G., Wei, C., Wei, Y. ((2020) ). MABAC method for multiple attribute group decision making under q-rung orthopair fuzzy environment. Defence Technology, 16: (1), 208–216. |
115 | Wang, L., Peng, J-j., Wang, J-q. ((2018) ). A multi-criteria decision-making framework for risk ranking of energy performance contracting project under picture fuzzy environment. Journal of cleaner production, 191: , 105–118. |
116 | Wang, P., Wang, J., Wei, G., Wei, C., Wei, Y. ((2019) ). The multi-attributive border approximation area comparison (MABAC) for multiple attribute group decision making under 2-tuple linguistic neutrosophic environment. Informatica, 30: (4), 799–818. |
117 | Wei, G., Wei, C., Wu, J., Wang, H. ((2019) ). Supplier selection of medical consumption products with a probabilistic linguistic MABAC method. International Journal of Environmental Research and Public Health, 16: (24), 5082. |
118 | Wei, G., He, Y., Lei, F., Wu, J., Wei, C. ((2020) a). MABAC method for multiple attribute group decision making with probabilistic uncertain linguistic information. Journal of Intelligent & Fuzzy Systems, 39: (1), 1–13. |
119 | Wei, G., He, Y., Lei, F., Wu, J., Wei, C., Guo, Y. ((2020) b). Green supplier selection with an uncertain probabilistic linguistic MABAC method. Journal of Intelligent & Fuzzy Systems, 39: (3), 3125–3136. |
120 | Wu, Y., Yan, Y., Wang, S., Liu, F., Xu, C., Zhang, T. ((2019) ). Study on location decision framework of agroforestry biomass cogeneration project: a case of China. Biomass and Bioenergy, 127: , 105289. |
121 | Xu, X.-G., Shi, H., Zhang, L.-J., Liu, H.-C. ((2019) ). Green supplier evaluation and selection with an extended MABAC method under the heterogeneous information environment. Sustainability, 11: (23), 6616. |
122 | Xue, Y.-X., You, J.-X., Lai, X.-D., Liu, H.-C. ((2016) ). An interval-valued intuitionistic fuzzy MABAC approach for material selection with incomplete weight information. Applied Soft Computing, 38: , 703–713. |
123 | Yazdani, M., Pamucar, D., Chatterjee, P., Chakraborty, S. ((2020) a). Development of a decision support framework for sustainable freight transport system evaluation using rough numbers. International Journal of Production Research, 58: (14), 4325–4351. |
124 | Yazdani, M., Torkayesh, A.E., Chatterjee, P. ((2020) ). An integrated decision-making model for supplier evaluation in public healthcare system: the case study of a Spanish hospital. Journal of Enterprise Information Management, 33: (5), 965–989. |
125 | Yazdani, M., Torkayesh, A.E., Santibanez-Gonzalez, E.D., Otaghsara, S.K. ((2020) c). Evaluation of renewable energy resources using integrated Shannon Entropy–EDAS model. Sustainable Operations and Computers, 1: , 35–42. |
126 | Yu, S-m., Wang, J., Wang, J-q. ((2017) ). An interval type-2 fuzzy likelihood-based MABAC approach and its application in selecting hotels on a tourism website. International Journal of Fuzzy Systems, 19: (1), 47–61. |
127 | Zamani, R., Ali, A.M.A., Roozbahani, A. ((2020) ). Evaluation of adaptation scenarios for climate change impacts on agricultural water allocation using fuzzy MCDM methods. Water Resources Management, 34: (3), 1093–1110. |
128 | Zavadskas, E.K., Turskis, Z., Tamošaitiene, J. ((2010) ). Risk assessment of construction projects. Journal of Civil Engineering and Management, 16: (1), 33–46. |
129 | Zhang, S., Wei, G., Alsaadi, F.E., Hayat, T., Wei, C., Zhang, Z. ((2020) ). MABAC method for multiple attribute group decision making under picture 2-tuple linguistic environment. Soft Computing, 24: (8), 5819–5829. |
130 | Zhu, J., Wang, R., Li, Y. ((2018) ). Failure mode and effects analysis considering consensus and preferences interdependence. Algorithms, 11: (4), 34. |