The Concept of AI-Based Algorithm: Analysis of CEUS Images and HSPs for Identification of Early Parenchymal Changes in Severe Acute Pancreatitis
Abstract
(1) Background: Identifying early pancreas parenchymal changes remains a challenging radiologic diagnostic task. In this study, we hypothesized that applying artificial intelligence (AI) to contrast-enhanced ultrasound (CEUS) along with measurement of Heat Shock Protein (HSP)-70 levels could improve detection of early pancreatic necrosis in acute pancreatitis. (2) Methods: Acute pancreatitis
1Introduction
Acute pancreatitis (AP) is one of the most common gastrointestinal disorders, and cases have been steadily increasing over the past few decades (Yadav and Whitcomb, 2010). Abdominal pain is the most commonly presented symptom of AP, but it does not necessarily reflect the severity of the disease (Afghani et al., 2015). The clinical challenge is the early identification of the 20% of patients for whom AP will become severe in a matter of days (Afghani et al., 2015). Usually, the clinical signs of acute pancreatitis are non-specific, with serum amylase and lipase levels correlating poorly with disease severity (Fei and Li, 2017). The severe form of the disease is accompanied by serious complications and is reported to have a high mortality rate (Frossard et al., 2008). The mortality rate of AP, especially the clinically severe form (SAP), can be as high as 15–48.4% (Dellinger et al., 2012). Imaging is recommended to confirm the clinical diagnosis, evaluate the cause of the abdominal pain, and grade the extent and severity of acute pancreatitis (Fei and Li, 2017). There are many etiological factors such as trypsin autodigestion, pancreatic microcirculation malfunction, calcium overload in pancreatic acinar cells, oxygen-free radical injury, and cytokine injury involved in the pathogenesis of severe forms of acute pancreatitis (Frossard et al., 2008). More recently, heat shock proteins (HSPs), particularly HSP-70, have received increasing attention as a stress-responsive protein. It plays a role in the pathogenesis and development of acute pancreatitis (Frossard et al., 2008). The increased expression of HSP-70 associated with pancreatic inflammation may confer a protective effect for the remaining acini after acute pancreatitis (Feng and Li, 2010).
The importance of early identification of AP severity may alter clinical decisions. In addition, speedy detection of pancreatic and/or peripancreatic necrosis, fluid collection, infection may change therapeutic protocols (Steinberg and Tenner, 1994; Bradley, 1993). Therefore, rapid evaluation by contrast enhanced ultrasound (CEUS) of the severe cases of AP might be critical. This is especially relevant when CT is not available, or patient’s condition is too critical. Prompt early identification can reduce morbidity and mortality (Fei and Li, 2017).
Although computed tomography (CT) is considered a gold standard radiological modality for assessing local complications of AP, CEUS could be considered a safe alternative as it is a reliable, non-invasive imaging modality with no radiation exposure and high sensitivity and specificity. If the pancreatic region is clearly visible on ultrasound, CEUS could be used to assess the severity of acute pancreatitis It provides information on the pancreatic parenchyma’s vascularization, detecting areas of non-viable pancreatic tissue, i.e. necrosis. A significant correlation between CEUS and CT was found for pancreatitis CT severity index and the extent of necrosis for the severity of AP. As CT contrast medium is nephrotoxic, it can aggravate AP in animal models by impairing the pancreatic microcirculation (Foitzik et al., 1994; Schmidt et al., 1995; Golea et al., 2010). However, ultrasound contrast agents are non-nephrotoxic, have fewer side effects, and, as reported in literature (Ethridge et al., 2000), the expression of HSP70 is increased after contrast-enhanced ultrasound. Thus, it could be considered as a safe and reliable alternative (Ethridge et al., 2000). The objective of this study is to assess the role of CEUS and HSP-70 for the detection of early pancreatic parenchymal necrosis in AP. Thus, to propose a concept of algorithm for extraction of informative quantitative parameters from CEUS images to be used further for training of the AI-based classifiers for automated classification of pancreas pathologies. Recently, with the development of technology and the evolution of artificial intelligence (AI) and deep neural networks, the computer-aided diagnosis (CAD) is becoming more accessible and accurate (Poce et al., 2024).
2Patients and Methods
2.1Study Design and Patient Population
We have conducted a prospective observational study in the period between November 2018 and December 2020. All patients admitted to the Center of Abdominal Surgery, Emergency Room or Intensive Care Unit at Vilnius University Hospital “Santaros klinikos” (Lithuania) with a diagnosis of acute pancreatitis and onset of the disease within 72 hours were included in this study. Total number of 146
The diagnosis was established on the basis of acute abdominal pain, at least 3-fold elevated levels of serum amylase and typical radiological findings. The contrast-enhanced CT scan was performed on admission, on day 4 to 7 after onset of the disease, to demonstrate the presence of pancreatic necrosis. According to the clinical course and clinical severity scores (Modified Atlanta Classification for Acute Pancreatitis, APACHE II > 7, MODS > 2) patients were stratified into mild, moderate and severe acute pancreatitis groups. Clinical data related to the severity of disease, development of organ dysfunction and/or septic complications was prospectively collected in a standardized fashion. Patients with chronic pancreatitis or repetitive episodes of acute pancreatitis were excluded. Peripheral blood samples from patients were drawn on admission to the hospital. Afterwards, centrifugation serum HSP-70 levels were measured using the standard technique utilizing Human HSP-70 ELISA Kit (Bender MedSystems GmbH, Vienna, Austria).
2.2Measurement of HSP-70 Concentration in Serum Samples
HSP-70 concentration was quantified in the serum using a commercially available enzyme-linked immunoassay (Bender MedSystems GmbH, Vienna, Austria). In brief, human HSP 70 present in the sample binds to antibodies adsorbed to the microwells. Following incubation, unbound biological components are removed during a wash step and a biotin-conjugated anti-HSP70 antibody is added and binds to human HSP70 captured by the first antibody. Following incubation unbound biotin- conjugated anti-human HSP70 antibody is removed during a wash step. Streptavidin conjugated to horseradish peroxidase (HRP) is added and binds to the biotin conjugated anti- human HSP70 antibody. Furthermore, incubation unbound Streptavidin-HRP is removed during a wash step, and serum solution reactive with HRP is added to the wells. A coloured product is formed in proportion to the amount of human HSP-70 present in the sample. The reaction is terminated by addition of acid and absorbance is measured using a spectrophotometer at 450 nm. A standard curve is prepared from 7 human HSP70 standard dilutions and HSP70 sample concentration is determined. All specimens were tested in replicate wells.
2.3The Algorithm for Analysis of CEUS Images
CT scan shows the region of pancreas affected by AP, however, it is a more expensive technique and due to non-portability it is not suitable to be applied in operation room. Therefore, due to portability and lower application costs of CEUS technique, the related ultrasonic CEUS images were analysed further.
For CEUS imaging a non-invasive ultrasound imaging device (Hitachi Arietta 70, Hitachi Aloka Medical, Japan) and ultrasound contrast agent (SonoVue, Bracco, Milan, Italy) were used following the manufacturer’s instructions. To implement the AI-based classifiers for the automated classification of CEUS diagnostic images of clinical pathologies into the different classes, it is necessary to select, extract and calculate a set of quantitative parameters.
The acquired low mechanical index (0,07) contrast harmonic images (movie clips up to 60 seconds) were further post-processed by manually selecting the rough contour of two-dimensional (2D) spatial regions of interest (ROI) (Fig. 1a). Subsequently, the set of quantitative parameters for both regions of parenchyma tissue (healthy and affected by necrosis) were automatically calculated. For healthy tissue, calculation of area
Calculations including image processing and extraction of the set of the quantitative parameters were performed using developed special algorithms implemented in the MATLAB 2020a software (The MathWorks Inc., Natick, MA USA). It is also essential to select and use only statistically significant parameters (
Fig. 1
The workflow of post-processing of acquired CEUS images and AI-based classification for estimating the level of acute pancreatitis.
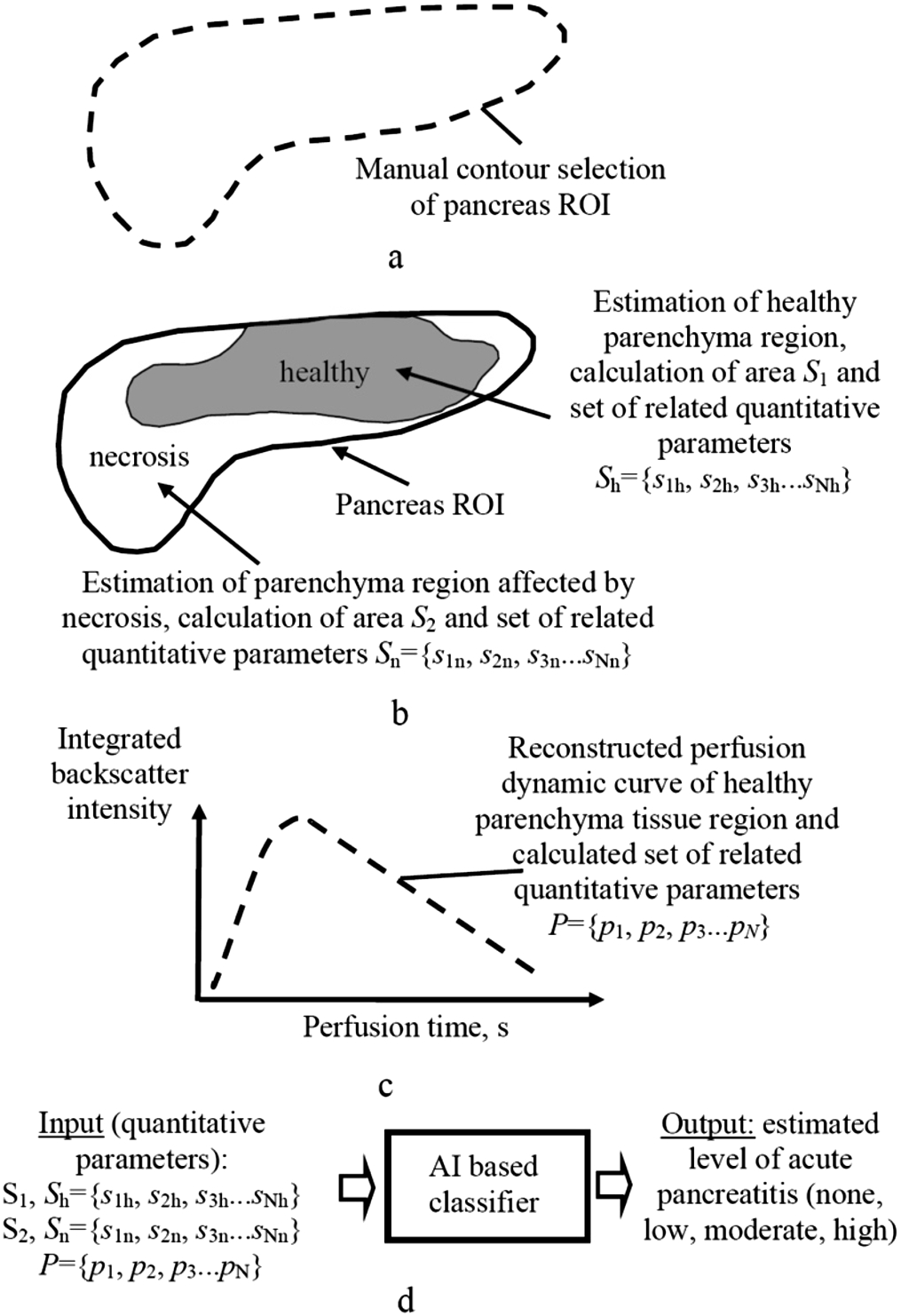
The principle of AI-based classifier for automatic estimation of the level of acute pancreatitis risk (none, low, moderate, or high) was presented as well (Fig. 1d). In computer-aided diagnostics (CAD), many machine learning methods have been used for training and performance evaluation of the classifiers (e.g. linear regression (LR), Naive Bayes (NB), Decision Tree (DT), Neuro-Fuzzy (NF), K – Nearest Neighbour (NN), Fuzzy C-Mean (FCM), Support Vector Machine (SVM), Artificial Neural Network (ANN), etc.), thus potentially useful in the images for pancreas diseases (Saftoiu et al., 2015; Shi et al., 2009; Kotsiantis et al., 2006). Machine learning is a branch of artificial intelligence (AI) focused on building applications that learn from data and improve their accuracy over time without being programmed to do so (Machine Learning, 2021). In the case of applying for classification only the set of quantitative parameters instead of whole images, based on our previous B-mode ultrasonic image analysis and skin cancer detection experience, the classifiers such SVM and LR are expected to be the most accurate (Tiwari et al., 2020).
3Results
3.1Analysis of Perfusion Dynamics and Acute Severe Pancreatitis
The subjects were categorized into two groups (patient and healthy control groups) based on the presence of acute pancreatitis diagnosis on admission. The studied patient for an algorithm establishment was a 28-year-old male with severe acute pancreatitis who was admitted to the ER less than 24 hours after the symptoms started. The diagnosis was confirmed by clinical examination and by ultrasound examination. The patient’s condition became unstable, and he was transferred to the Intensive Care Unit (ICU), where a CT scan (Fig. 2) and CEUS were simultaneously performed.
Fig. 2
28 years old male with severe acute pancreatitis. Contrast-enhanced CT (portovenous phase, the same axial plane as on CEUS image) confirmed acute necrotizing pancreatitis (arrows) with acute necrotic collection (star).
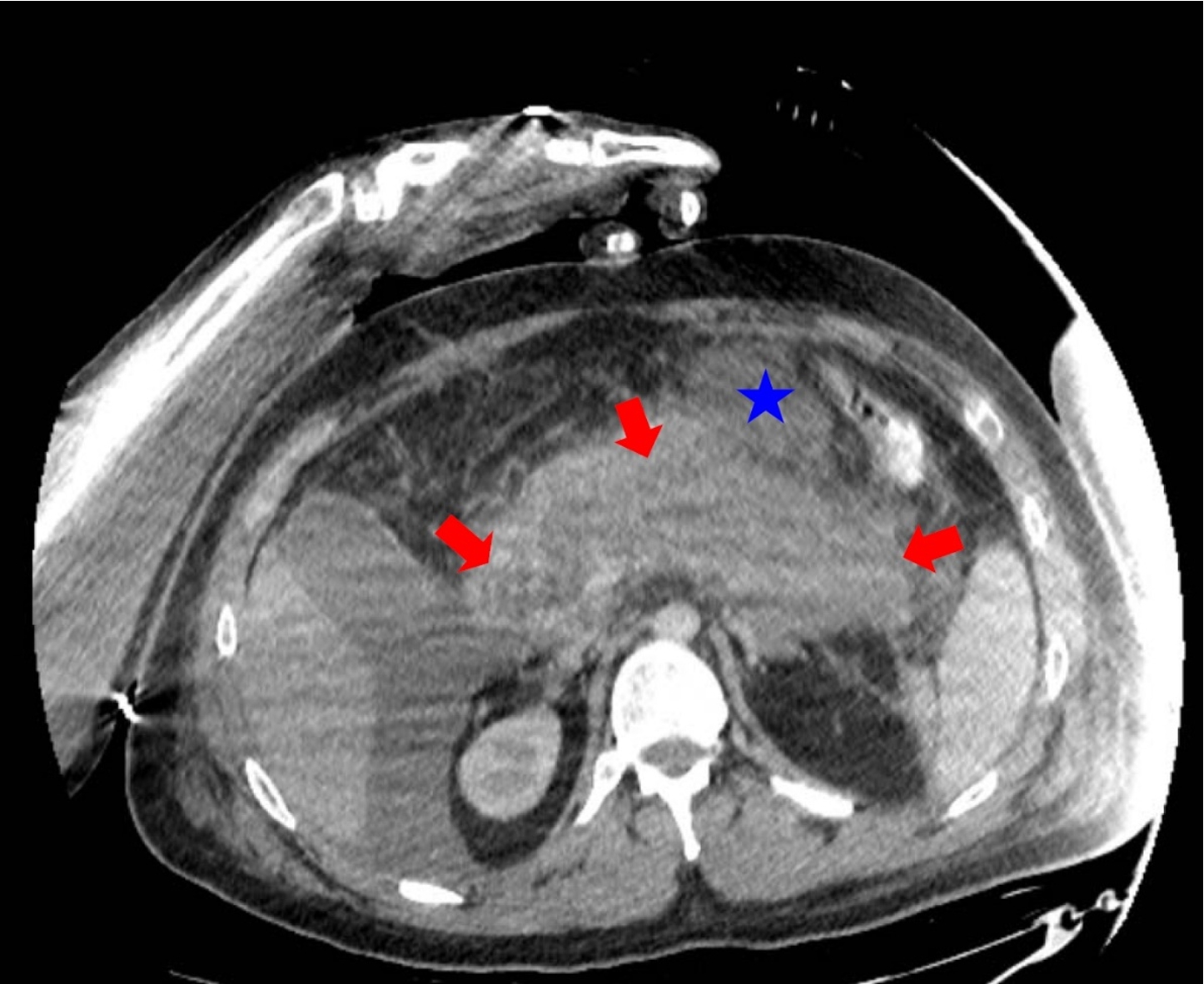
The appropriate sets of quantitative parameters within the selected ROI of each CEUS images were acquired. Automated estimation of region boundaries of healthy parenchyma tissue within the pancreas ROI was performed by appropriate threshold analysis (e.g. Otsu, etc.). During the analysis, it was assumed that during CEUS procedure, the contrast material penetrates regions of healthy parenchyma tissue, with limited penetration in regions affected by necrosis of parenchyma tissue. Further, healthy and impaired areas (affected by necrosis) were automatically estimated and quantitatively evaluated as percentages compared of overall ROI (Figs. 3, 4).
Fig. 3
Example of a CEUS image (15 s after injection of contrast agent) of a healthy pancreas (healthy volunteer – female) and estimated area of a healthy parenchyma (according to the presence of perfusion): a – contrast harmonic image of the pancreas region and manually selected ROI according to the solid red line, b – extracted informative ROI for further automatic detection of healthy parenchyma areas being marked with a solid green line, c – automatically detected area of a healthy parenchyma is binary marked (white) and covers 100.0% of the overall pancreas ROI.
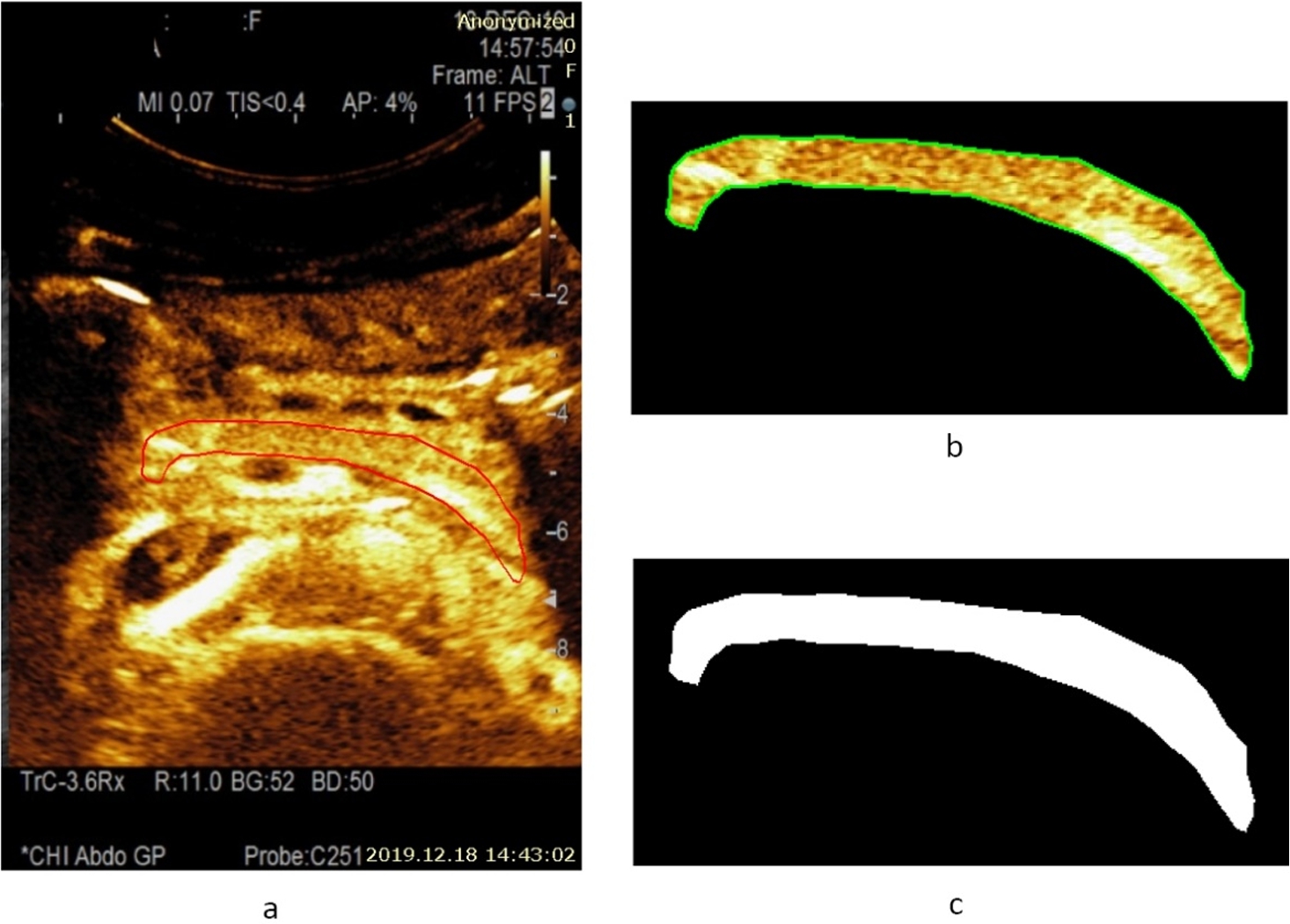
Fig. 4
A CEUS image of a pancreas at 22 s after injection of contrast agent, and estimated area of a healthy parenchyma (according to the presence of perfusion) from a male patient with acute necrotizing pancreatitis: a – contrast harmonic image of the pancreas region and manually selected ROI marked with a solid red line, b – extracted informative ROI for further automatic detection of healthy parenchyma areas marked with a solid green line, c – automatically detected area of a healthy parenchyma (white colour) covering 55.6% of overall pancreas ROI.
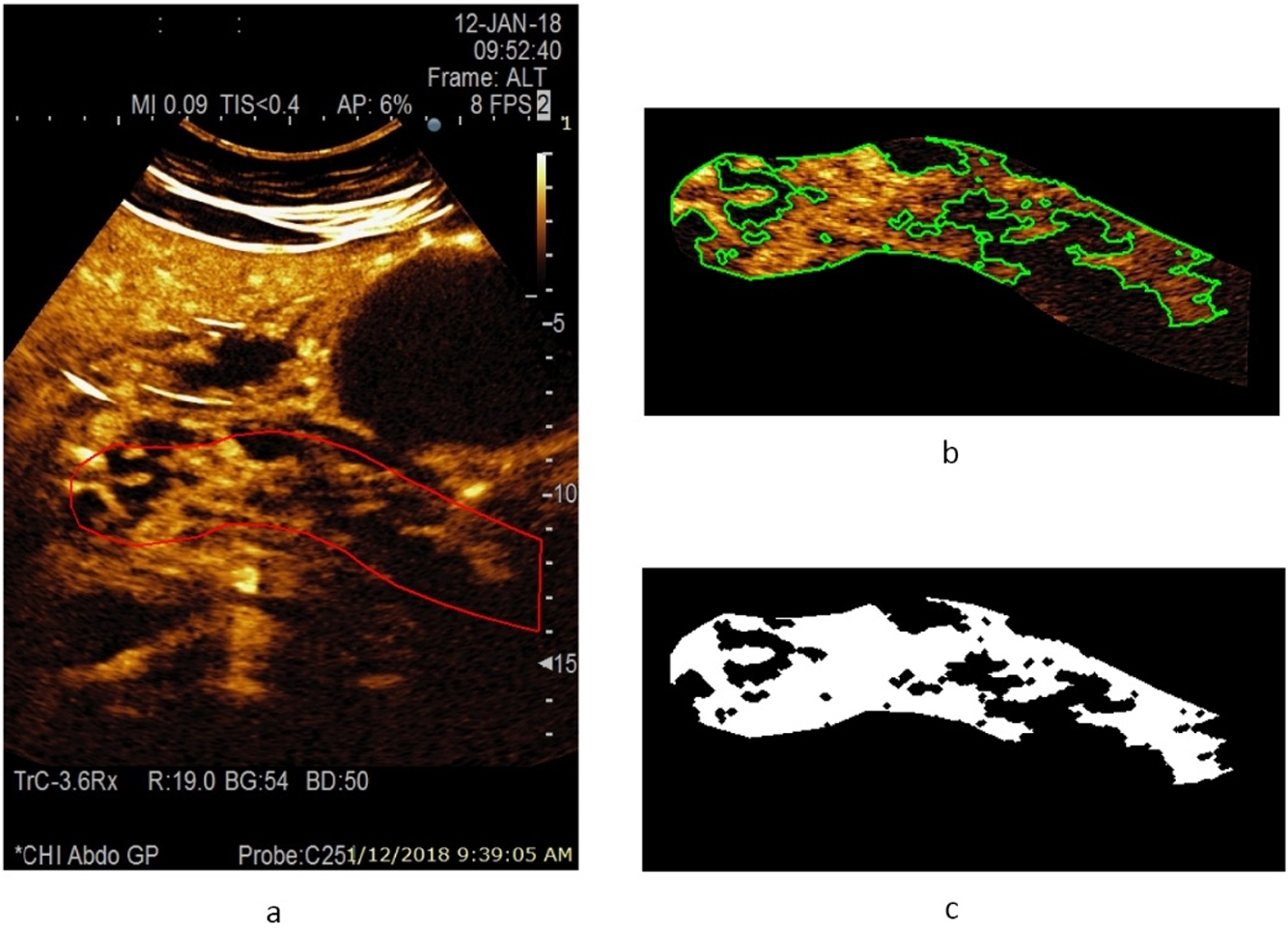
An example of a CEUS image of a healthy pancreas (healthy female volunteer) acquired at 15 s after injection of contrast agent and an estimated area of healthy parenchyma (according to the presence of perfusion) is presented in Fig. 3. The automatically detected area of healthy parenchyma is marked in white, with the area covering 100.0% of the overall pancreas ROI. The CEUS B-mode image of pancreas affected by acute pancreatitis (male patient) acquired 22 s after injection of contrast agent, and an estimated area of healthy parenchyma (according to the presence of perfusion) is presented in Fig. 4. Here, the automatically detected area of healthy parenchyma (white) covers 55.6% of the overall ROI. The remaining part of the tissue is affected by necrosis and covers 44.4% of the overall ROI.
Reconstructed normalized perfusion dynamic curves by approximations of log-normal distributions (Dietrich et al., 2012) for healthy volunteers and patients with pancreas affected by necrosis are presented in Fig. 5. Estimated parameters (Dietrich et al., 2012; Omoto et al., 2017; Saftoiu et al., 2015) from the reconstructed normalized perfusion dynamic curves are presented in Table 1. Here, the Peek value is higher (155.5) and the mean transit time is longer (40.1 s) for a healthy pancreas in a healthy volunteer compared with parenchyma affected by necrosis in a patient with acute necrotizing pancreatitis (46.5 and 34.6 s, respectively).
Fig. 5
Reconstructed normalized perfusion dynamic curves by approximations of log-normal distributions for healthy volunteer and patient with pancreas affected by necrosis.
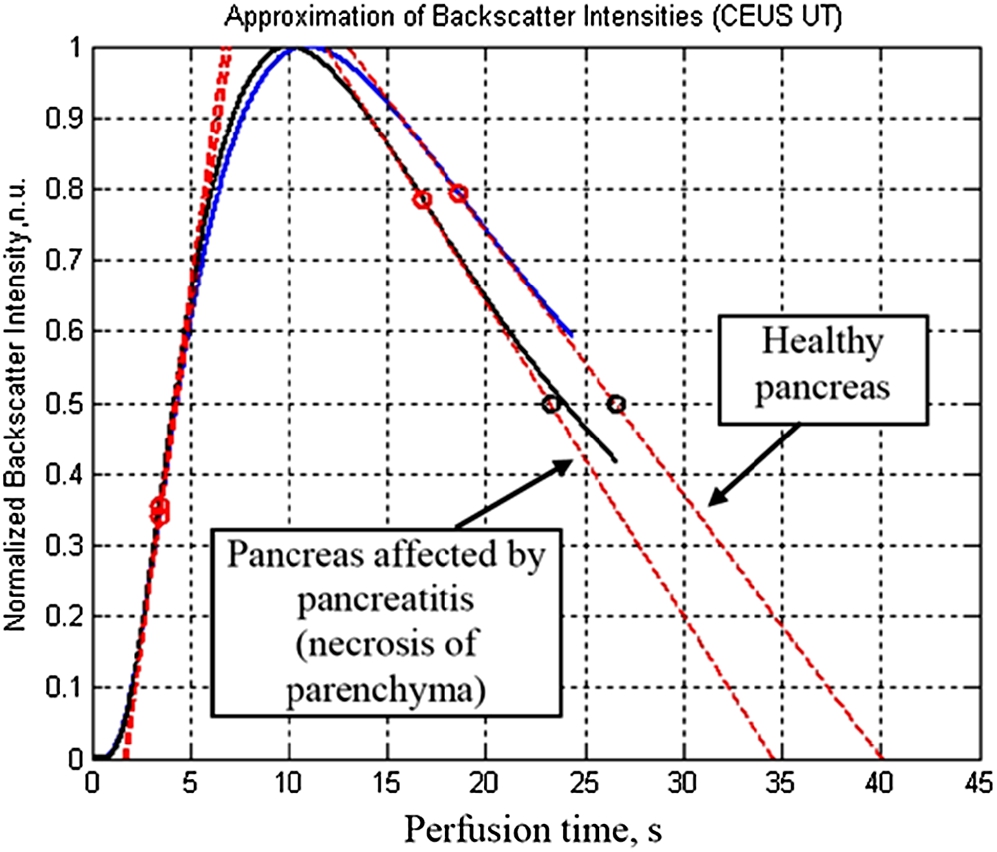
3.2Biochemical Changes and Acute Pancreatitis
In order to validate the reliability of non-invasive techniques (e.g. CEUS), the gold standard technique should be used as well. For example, measures in diagnosing acute pancreatitis are three times increased lipase and amylase levels. Recently, new and novel biomarkers have emerged. HSP-70 has been evaluated for use in the early diagnosis of acute pancreatitis. Bhagat et al. proved that HSP-70 played a vital role in protecting organisms against pancreatitis and found that the high expression of HSP-70 induced by hyperthermia can inhibit trypsinogen activation in cerulein-induced pancreatitis, resulting in its decreased severity. Furthermore, in non-thermally stressed rats, pretreatment of antisense-HSP-70 can aggravate the severity of pancreatitis while reducing the HSP-70 expression (Ethridge et al., 2000). Also, factors such as an accelerated protein degradation rate and post-transcriptional regulation of HSP play a key role in the serum expression levels of HSP-70 proteins, especially in the setting of CEUS in liver tumors (Liu et al., 2010).
Table 1
Estimated quantitative parameters from the reconstructed normalized perfusion dynamic curves.
Parameters | Parenchyma affected by necrosis (patient with acute pancreatitis) | Healthy pancreas of healthy volunteer |
Peek value, arbitrary units | 46.5 | 155.5 |
Time to peek after injection of contrast agent, s | 19 s | 24.2 s |
(10 s after appearance of contrast agent caused reflections) | (10.9 s after appearance of contrast agent caused reflections) | |
Area under the “wash in” curve | 7858 | 29595 |
Mean transit time MTT, s | 34.6 s | 40.1 s |
MTT (50% of peak), s | 23.2 s | 26.5 s |
“Wash in” rate | 9.3 | 28.7 |
“Wash out” rate | 2.1 | 5.7 |
Rise time, s | 8.4 s | 9.3 s |
Fall time, s | 24.6 s | 29.1 s |
Wash in perfusion index | 940.96 | 3175.1 |
4Discussion
In this paper we present two novel AI-based approaches combined with biochemical markers for early AP severity detection. First, automatic adaptive detection of ROI boundaries of the pancreas region within multiple contrast harmonic imaging (CHI) mode images. Second, the compensation of artifacts influences the stability of spatial pancreas tissue position within the detected ROI while comparing with HSP-70 levels. Additional artifacts are caused by physiological movements of patient body tissues due to breathing and pulsation of larger blood vessels and by additional transducer movements caused by the ultrasonography operator during examination and the image acquisition procedure.
Further study results are currently being analysed to process more images of healthy volunteers and patients with acute pancreatitis of various severity levels. Unfortunately, the worldwide outbreak of SARS-Cov-2 makes some limitations of clinical studies with real patients and healthy volunteers. Afterwards, the AI–based classification will be applied to detect the presence of early-stage AP severity. While CEUS remains a reliable non-invasive diagnostic test for detecting morphological changes of the pancreas, evaluating conditions of the vasculature, visualizing contours of pancreas, quantifying perfusion and necrotic areas, it could be used along with CT and magnetic resonance imaging (MRI) (Omoto et al., 2017). Perfusion analysis by evaluating the time-intensity curve at early arterial and late venous phases (wash-in and wash-out) was performed to investigate chronic pseudotumoral pancreatitis and pancreatic cancer. During the study by Gheonea et al. (2013) of 51 patients (chronic pancreatitis 19 and pancreatic cancer 32), the achieved sensitivity and specificity were 93.75% and 89.47%, correspondingly. In addition, during the study of 173 patients (chronic pancreatitis 35 and pancreatic cancer 138), the achieved sensitivity and specificity were 88.6% and 97.8%, respectively. Ardelean et al. have introduced a retrospective study of 197 patients. In 60 examinations of acute pancreatitis, the pancreatic necrosis was diagnosed in 30 cases. The estimated accuracy of necrotic lesions was 97.4%, and authors declared CEUS to be the cost-effective method for detecting pancreatic necrosis, comparable to CT (Ardelean et al., 2014). Omoto et al. presented results of time-intensity curve parameters being used for quantitative perfusion analysis and differentiation of pancreatic carcinomas (Ardelean et al., 2014). The intensity gains at time after of 60 s was enumerated as the most accurate for diagnosis of pancreatic carcinomas. Additionally, CEUS is suitable for differentiation between normal and inflamed tissue, abscesses, cysts, and necrosis (Saftoiu et al., 2015).
The importance of HSP-70 expression after CEUS have been described in liver diseases with the mean density 0.35 while compared in radiofrequency ablation group with 0.31 accordingly (Liu et al., 2010). In addition, Ethridge RT et al. found the increase levels of HSP-70 and HSP-27 expressed after induction of pancreatitis (Ethridge et al., 2000). Furthermore, literature shows that expression of HSP-70 and HSP-27 with pancreatic inflammation may play a protective effect for the remaining acini after an acute pancreatitis episode (Ethridge et al., 2000).
In our study, the algorithm concept and workflow of post-processing of acquired CEUS images was used to estimate sets of quantitative parameters for healthy tissue of pancreas parenchyma and tissue affected by necrosis. Also, the principle of the AI–based classification for estimating the risk level of acute pancreatitis (none, low, moderate, high) was presented to overcome the limitations of ultrasound. Major disadvantages of ultrasound remain the limited visibility of the pancreas and peripancreatic region in a large proportion of patients with severe acute pancreatitis because of the presence of overlying bowel gas, particularly in the case of ileus. The body habitus may also limit the penetration of acoustic waves in obese patients. Additionally, abdominal ultrasound is less accurate in delineating extrapancreatic inflammatory spread within retroperitoneal spaces. Finally, ultrasound is operator-dependent and displayed on a limited number of images that are not easy to comprehend and convey to practicing clinicians. Applying AI and CEUS enhance sensitivity and specificity for the assessment of the early severity of acute pancreatitis.
Authors declare that the small number of patients and additional artifacts in the images are limitations to the study.
5Conclusions
Further studies are now underway to determine whether pairing the CEUS, conventional radiology tests (i.e. CT, MRI) and AI techniques (image acquisition, further sophisticated post-processing, and classification) and biochemical markers (i.e.HSP-70) will result in a valuable new diagnostic and clinical decision support tool in early diagnosis of AP and the presence of pancreas tissue necrosis.
6Patents
A patent application was filed describing the findings in this paper. The patent application number is LT2020 538.
Author Contributions: Conceptualization, A.G. and R.R.; methodology, A.G. and A.S. and R.R.; formal analysis, A.G. and R.R.; investigation, A.G and R.R.; data curation, A.G., and A.S.; writing – original draft preparation, A.G., and R.R.; visualization, R.R.; writing – review and editing of the manuscript, K.S., A.S., G.D., and A.G.; supervision, K.S., G.D. and R.R.
Funding: This research received no external funding.
Institutional Review Board Statement: The study was conducted according to the guidelines of the Declaration of Helsinki and approved by the Institutional Ethics Committee of Vilnius University (protocol code Nr. 158200-17-941-455, date of approval October 3rd, 2017).
Informed Consent Statement: Patient consent was signed by the patient’s relatives due to the critical condition and incapacity of the patient in accordance with the clinical protocol procedures.
Data Availability Statement: The data presented in this study are available on request from the corresponding authors. The data are not publicly available due to patient data privacy EU regulations.
Conflicts of Interest: The authors declare no conflict of interest.
Acknowledgements
We acknowledge the technical support and guidance of Ultrasound Research Institute, Kaunas Technology University, Kaunas, Lithuania.
References
1 | Afghani, E., Pandol, S.J., Shimosegawa, T., Sutton, R., Wu, B.U., Vege, S.S., Gorelick, F., Hirota, M., Windsor, J., Lo, S.K., Freeman, M.L., Lerch, M.M., Tsuji, Y., Melmed, G.Y., Wassef, W., Mayerle, J. ((2015) ). Acute pancreatitis—progress and challenges a report on an international symposium. Pancreas, 44: (8), 1195–1210. https://doi.org/10.1097/MPA.0000000000000500. |
2 | Ardelean, M., Şirli, R., Sporea, I., Bota, S., Martie, A., Popescu, A., Dănilă, M., Timar, B., Buzas, R., Lighezan, D. ((2014) ). Contrast enhanced ultrasound in the pathology of the pancreas – a monocentric experience. Medical Ultrasonography, 16: (4), 325–331. https://doi.org/10.11152/mu.201.3.2066.164.mars12. |
3 | Bradley, E.L. ((1993) ). A clinically based classification system for acute pancreatitis: summary of the International Symposium on Acute Pancreatitis, Atlanta, Ga, September 11 through 13, 1992. Archives of Surgery, 11 Through 13: (5), 586–590. https://doi.org/10.1001/archsurg.1993.01420170122019. 1992, 128. |
4 | Dietrich, C.F., Averkiou, M.A., Correas, J.M., Lassau, N., Leen, E., Piscaglia, F. ((2012) ). An EFSUMB introduction into Dynamic Contrast-Enhanced Ultrasound (DCE-US) for quantification of tumour perfusion. Ultraschall der Medizin, 33: (4), 344–351. https://doi.org/10.1055/s-0032-1313026. |
5 | Dellinger, E.P., Forsmark, C.E., Layer, P., Lévy, P., Maraví-Poma, E., Petrov, M.S., Shimosegawa, T., Siriwardena, A.K., Uomo, G., Whitcomb, D.C., Windsor, J.A., Abu Hilal, M., Abu-Zidan, F.M., Acosta, J.M., Ainsworth, A.P., Aizcorbe Garralda, M., Alagozlu, H., Al’aref, S.J., Albeniz Arbizu, E., Alhajeri, A., Almeida, I.C., Almeida, J.L., Amano, H., Ammori, B.J., Andersson, B., Andersson, R., Andrén-Sandberg, A., Ardengh, J.C., Arroyo-Sanchez, A.S., Arvanitakis, M., Ashley, S.W., Aygencel, G., Ayoub, W.A., Baillie, J., Bala, M., Ball, C.G., Baron, T.H., Barreto, S.G., Basaranoglu, M., Beger, H.G., Bernal Monterde, V., Bharwani, N., Bhasin, D.K., Bong, J.J., Botoi, G., Bruennler, T., Cairoli, E., Carter, C.R., Cernea, D., Chari, S.T., Charnley, R.M., Chooklin, S., Cochior, D., Col, C., Conwell, D.L., Correia, M.I., Dambrauskas, Z., Darvas, K., De Campos, T., De Casasola, G.G., De Waele, J.J., Del Chiaro, M., Delle Fave, G., De-Madaria, E., Di Sebastiano, P., Diuzheva, T.G., Duarte-Rojo, A., Fagenholz, P.J., Farkas, G., Farre Viladrich, A., Fernandez-Del Castillo, C., Friess, H., Frossard, J.L., Gandhi, V., Gardner, T.B., Gloor, B., Gluk, M., Goltsov, V.R., Guevara-Campos, J., Gumbs, A.A., Hackert, T., Hauser, G., Horvath, K.D., Howard, T.J., Igarashi, H., Ioannidis, O., Jaber, S., James, F.E., Jha, R.K., Juneja, D., Kamisawa, T., Kandasami, P., Kantarcioglu, M., Kapoor, V.K., Karakan, T., Kaya, E., Khaliq, A., Kiriyama, S., Kochhar, R., Konstantinou, G.N., Kylänpää, M.L., Lankisch, P.G., Laplaza Santos, C., Lata, J., Leppäniemi, A., Levy, P., Lopez, A., López Camps, V., Lujano-Nicolas, L.A., Lund, H., Lytras, D., Macaya Redin, L., Machado, M.C., Macias Rodriguez, M.A., Mann, O., Maravi-Poma, E., Marincas, M., Marwah, S., Mas, E., Matheus, A.S., Meier, R., Mennecier, D., Mentula, P., Mifkovic, A., Mofidi, R., Mole, D.J., Morris-Stiff, G., Mossner, J., Muftuoglu, M.A., Munsell, M.A., Nathens, A.B., Neri, V., Nöjgaard, C., Nordback, I., Ocampo, C., Oláh, A., Olejnik, J., O’Reilly, D.A., Oria, A., Panek, J., Papachristou, G.I., Parekh, D., Parks, R.W., Passaglia, C., Pearce, C.B., Pellegrini, D., Perez-Mateo, M., Pettila, V., Pezzilli, R., Pitchumoni, C.S., Pongprasobchai, S., Poves Prim, I., Puolakkainen, P., Pupelis, G., Radenkovic, D.V., Rahman, S.H., Regidor Sanz, E., Repiso, A., Rodrigo, L., Rosseland, A., Rydzewska, G., Sánchez-Izquierdo Riera, J.A., Savides, T.J., Scaglione, M., Serrablo, A., Servin-Torres, E., Sethu, I., Sezgin, O., Shankar-Hari, M., Singer, M.V., Sinha, S.K., Sjoberg Bexelius, T., Skipworth, J.R., Soriano, F.G., Sotoudehmanesh, R., Spanier, B.W., Stabuc, B., Steinberg, W., Stroescu, C., Szentkereszty, Z., Takacs, T., Takada, T., Takeda, K., Takeyama, Y., Tang, W., Tanjoh, K., Tarnasky, P.R., Teich, N., Tellado, J.M., Tenner, S., Thomson, A., Tireli, M., Tong, Z., Triantopoulou, C., Uy, M.C., van Geenen, E.J., Velasco Guardado, A., Vettoretto, N., Vollmer, C.M., Wada, K., Warshaw, A.L., Weinbroum, A.A., Wilson, J.S., Wittau, M., Wu, B.U., Wysocki, A.P., Yan Quiroz, E., Yasuda, T., Yu, C., Zerem, E., Zhou, X., Zubia Olazcoaga, F., Zyromski N.J., ((2012) ). Determinant-based classification of acute pancreatitis severity: an international multidisciplinary consultation. Annals of Surgery, 256: (6), 875–880. https://doi.org/10.1097/SLA.0b013e318256f778. |
6 | Echegaray, S., Bakr, S., Rubin, D.L., Napel, S. ((2018) ). Quantitative Image Feature Engine (QIFE): an open-source, modular engine for 3D quantitative feature extraction from volumetric medical images. Journal of Digital Imaging, 31: (4), 403–414. https://doi.org/10.1007/s10278-017-0019-x. |
7 | Ethridge, R.T., Ehlers, R.A., Hellmich, M.R., Rajaraman, S., Evers, B.M. ((2000) ). Acute pancreatitis results in induction of heat shock proteins 70 and 27 and heat shock factor-1. Pancreas, 21: (3), 248–256. https://doi.org/10.1097/00006676-200010000-00005. |
8 | Fei, Y., Li, W.-Q. ((2017) ). Effectiveness of contrast-enhanced ultrasound for the diagnosis of acute pancreatitis: a systematic review and meta-analysis. Digestive and Liver Disease, 49: , 623–629. https://doi.org/10.1016/j.dld.2017.03.017. |
9 | Feng, J.Y., Li, Y.Y. ((2010) ). Alteration and role of heat shock proteins in acute pancreatitis. Journal of Digestive Diseases, 11: (5), 277–283. https://doi.org/10.1111/j.1751-2980.2010.00450.x. |
10 | Foitzik, T., Bassi, D.G., Castillo, Del, C.F., Warshaw, A.L., Rattner, D.W. ((1994) ). Intravenous contrast medium impairs oxygenation of the pancreas in acute necrotizing pancreatitis in the rat. Archives of Surgery, 129: (7), 706–711. https://doi.org/10.1001/archsurg.1994.01420310038006. |
11 | Frossard, J.L., Steer, M.L., Pastor, C.M. ((2008) ). Acute Pancreatitis. The Lancet, 371: (9607), 143–152. https://doi.org/10.1016/S0140-6736(08)60107-5. |
12 | Gheonea, D.I., Streba, C.T., Ciurea, T., Saftoiu, A. (2013). Quantitative low mechanical index contrast-enhanced endoscopic ultrasound for the differential diagnosis of chronic pseudotumoral pancreatitis and pancreatic cancer. BMC Gastroenterology, 13(2). https://doi.org/10.1186/1471-230X-13-2. |
13 | Golea, A., Badea, R., Socaciu, M., Diaconu, B., Iacob, D. ((2010) ). Quantitative analysis of tissue perfusion using contrast-enhanced transabdominal ultrasound (CEUS) in the evaluation of the severity of acute pancreatitis. Medical Ultrasonography, 12: (3), 198–204. |
14 | Haralick, R.M., Dinstein, I., Shanmugam, K. ((1973) ). Textural features for image classification. IEEE Transactions on Systems, Man, and Cybernetics, SMC–3(6): , 610–621. https://doi.org/10.1109/TSMC.1973.4309314. |
15 | Kotsiantis, S.B., Zaharakis, I.D., Pintelas, P.E., ((2006) ). Machine learning: a review of classification and combining techniques. Artificial Intelligence Review, 26: (3), 159–190. https://doi.org/10.1007/s10462-007-9052-3. |
16 | Liu, G.J., Moriyasu, F., Hirokawa, T., Rexiati, M., Yamada, M., Imai, Y. ((2010) ). Expression of heat shock protein 70 in rabbit liver after contrast-enhanced ultrasound and radiofrequency ablation. Ultrasound in Medicine and Biology, 36: (1), 78–85. https://doi.org/10.1016/j.ultrasmedbio.2009.08.001. |
17 | Machine Learning (2021). https://www.ibm.com/cloud/learn/machine-learning. |
18 | Mashayekhi, R., Parekh, V.S., Faghih, M., Singh, V.K., Jacobs, M.A., Zaheer, Radiomic, A. (2020). Features of the pancreas on CT imaging accurately differentiate functional abdominal pain, recurrent acute pancreatitis, and chronic pancreatitis. European Journal of Radiology, 123. https://doi.org/10.1016/j.ejrad.2019.108778. |
19 | Omoto, S., Takenaka, M., Kitano, M., Miyata, T., Kamata, K., Minaga, K., Arizumi, T., Yamao, K., Imai, H., Sakamoto, H., Harwani, Y., Sakurai, T., Watanabe, T., Nishida, N., Takeyama, Y., Chiba, Y., Kudo, M. ((2017) ). Characterization of pancreatic tumors with quantitative perfusion analysis in contrast-enhanced harmonic endoscopic ultrasonography. Oncology, 93: (1), 55–60. https://doi.org/10.1159/000481231. |
20 | Poce, I., Arsenjeva, J., Kielaite-Gulla, A., Strupas, K., Pancreas, D.G. ((2024) ). Segmentation in CT images: state of the art in clinical practice. Baltic Journal of Modern Computing, 12: (1), 1–3. |
21 | Səftoiu, A., Vilmann, P., Dietrich, C.F., Iglesias-Garcia, J., Hocke, M., Seicean, A., Ignee, A., Hassan, H., Streba, C.T., Ioncicə, A.M., Gheonea, D.I., Ciurea, T. ((2015) ). Quantitative contrast-enhanced harmonic EUS in differential diagnosis of focal pancreatic masses (with videos). Gastrointestinal Endoscopy, 82: (1), 59–69. https://doi.org/10.1016/j.gie.2014.11.040. |
22 | Schmidt, J., Hotz, H.G., Foitzik, T., Ryschich, E., Buhr, H.J., Warshaw, A.L., Herfarth, C., Klar, E., ((1995) ). Intravenous contrast medium aggravates the impairment of pancreatic microcirculation in necrotizing pancreatitis in the rat. Archives of Surgery, 221: (3), 257–264. https://doi.org/10.1097/00000658-199503000-00007. |
23 | Shi, Z., He, L., Suzuki, K., Nakamura, T., Itoh, H. ((2009) ). Survey on neural networks used for medical image processing. International Journal of Computational Science, 3: (1), 86–100. |
24 | Steinberg, W., Tenner, S. ((1994) ). Acute pancreatitis. The New England Journal of Medicine, 330: (17), 1198–1210. https://doi.org/10.1056/NEJM199404283301706. |
25 | Tiwari, K.A., Raišutis, R., Liutkus, J., Valiukevičienė, S. ((2020) ). Diagnostics of melanocytic skin tumours by a combination of ultrasonic, dermatoscopic and spectrophotometric image parameters. Diagnostics, 10: (9), 632. https://doi.org/10.3390/diagnostics10090632. |
26 | Yadav, D., Whitcomb, D.C. ((2010) ). The role of alcohol and smoking in pancreatitis. Nature Reviews Gastroenterology & Hepatology, 7: , 131–145. https://doi.org/10.1038/nrgastro.2010.6. |