Federated FAIR principles: Ownership, localisation and regulatory compliance (OLR)
Abstract
FAIR-Guidelines can be enriched to include ownership of data, localisation of storing data and stewardship that respects regulatory frameworks relevant to the jurisdiction. It is proposed that this is referred as FAIR-OLR; OLR referring to Ownership, Localisation and Regulation of data production in jurisdiction.
1.Introduction
This article discusses the use of FAIR (Findable, Accessible, Interoperable and Reusable) Data based on Ownership, Localisation and Regulatory Compliance of data (FAIR-OLR) as a specification of FAIR Guidelines for the use of sensitive data and personal data. The relevance of Ownership, Localisation and Regulatory Compliance (OLR) of data-use was at the core of the research undertaken by the Virus Outbreak Data Network (VODAN)-Africa, aiming to improve health data-visiting of data produced in health clinics in different African countries.
Utilising the Guidelines of FAIR-Data[18], VODAN-Africa investigates how the quality of health-data available for, and from, Africa can be enhanced. Health records in Africa are often missing or lack generalisability, as data from remote or vulnerable communities are not available. A less biased data pipeline of African health records is much needed. [15]
The research seeks to investigate how an ethical quality data pipeline can be produced. In framing the question in this way, it responds to the concern about the current practices of health records being moved out of jurisdictions, without control by the data subject over the life-cycle of the data. In order to be compliant with the European Union (EU) General Data Protection Regulation (GDPR, 2016) [10], which came into force in 2018, and following the FAIR-Data guidelines, investigation of the possibility of curating federated, interoperable and reusable health records is relevant. The GDPR assigns ultimate data-control to the data subject to whom the data refers, and finds that in collaboration with any EU data processor, handling must be GDPR compliant. In order to adhere to GDPR standards, it is necessary that data pertaining to a data subject remain within the jurisdiction where such data is produced. Increasingly, the data ownership is subject to GDPR-equivalent regulatory principles in non-EU geographies, including in Africa [14].
OLR principles emerged in a research that began with the development of a FAIR-data architecture in nine African countries (see Fig. 1). In the second phase the software was deployed as a minimum viable product (MVP) in 88 health facilities in a real-life natural setting [17]. The MVP deploys patient health records from the Out Patient Registry, Ante Natal Care an d COVID Data from facilities in rich metadata-format as machine-actionable and visitable FAIR objects in AllegroGraph [1], a graph producing software, within the clinic where the data is produced. VODAN-Africa achieved a data-visiting, architecture in which data is entered once, can be arranged by algorithmic tools to perform different operations, and allowing for horizontal data-interoperability (within the community) and vertical data-interoperability (with other platforms) [2]. The data can be visited across different regulatory jurisdictions. The investigation concluded that key aspects requiring attention for interoperability in FAIR-compliant architectures are: Data Ownership, Localisation and Regulatory compliance in jurisdiction (OLR). The relevance of ‘OLR’ is briefly described below.
Fig. 1.
The VODAN-A Architecture based on FAIR-OLR principles. The figure was first published in: Van Reisen, M., Oladipo, F., Mpezamihigo, M., Plug, R., Basajja, M., Aktau, A., Purnama Jati, P.H., Nalugala, R., Folorunso, S., Amare, Y.S., Abdulahi, I., Afolabi, O.O., Mwesigwa, E., Taye, G.T., Kawu, A., Ghardallou, M., Liang, Y., Osigwe, O., Medhanyie, A.A., Mawere, M.: Incomplete COVID-19 data: The curation of medical health data by the Virus Outbreak Data Network-Africa. Data Intelligence 4(4) (2022) (2022). doi: https://doi.org/10.1162/dint_e_00166. This figure presents the architecture of the MVP of VODAN-A, with deployment of a data editor in each location where data is produced. The patient data is entered only once, and it is produced with semantically right metadata, in a template based on vodanaportal (onteportal-based) vocabularies and stored in allegrograph. The data is available for several simultaneous use cases, including an internal dashboard of the patient data, and external dashboard of aggregates computed from each location, an expert function to DHIS2 (or alternative Health Information System) and an allgegrograph that can be visited with specific SPARQL queries.
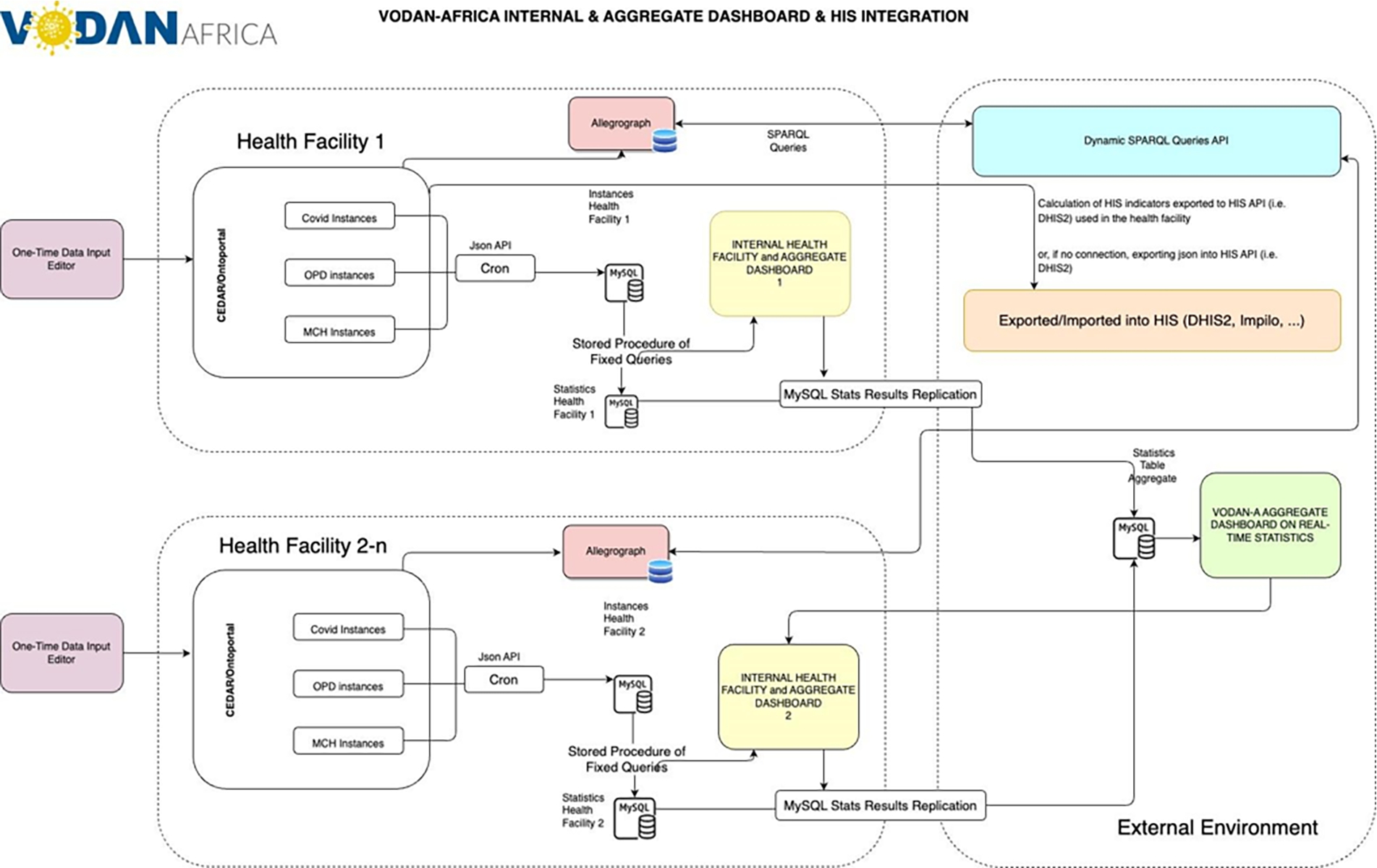
1.1.Ownership
Ownership is a critical principle from which access and control arrangements are derived [6,10]. In VODAN-Africa the data is stored in residence, governed by data use agreements, and compliant with the regulations identified in each locality [14]. In multiple jurisdictions, data-ownership is federated, and requires data-localisation, meaning the repositioning of machine-actionable meta-data held in the repository where the data is produced. The precise definition of data-ownership may differ in the different regulatory frameworks in place for different situations. The ultimate ownership of personal health record resides with the data subject and/or the health facility where the data is produced who consent for data (re)-use. When the data is aggregated – without inclusion of personal information – into statistical data elements, ownership can be transferred. The main aspect regarding ownership of the data principle is that personal data pertains to the data subject and is stewarded by the data producer with consent of the data subject, and this is a basic non-negotiable principle in VODAN-Africa.
1.2.Localisation
Data Localisation is necessary if the standard of data Ownership is to be met. VODAN-A tested the possibility of repositing data in each of the participating health clinics. The data is entered only once and stored in AllegroGraph, or a triple store for semantic linked data, from where it is available for statistical analysis in the local data dashboard. In addition, horizontal interoperability is arranged across health facilities in the community [17]. The VODAN-A dashboard serves to visualise health population trends. Vertical interoperability with other platforms is supported through interoperability schemas [17]. Specific functions, such as an automated computation in a Health Information Systems (HIS) or reporting into District Health Information System 2 (DHIS2) are arranged within the architecture deployed in residence.
The VODAN-A research found that a ‘locale’ for data localisation can differ across different geographies. In some locations, depending on availability of resources, a client-server deployment architecture was followed. In a client-server set-up, the MVP is deployed in a local setting and the issue of ownership is adhered to, through the data ownership structure in the client-server set-up. In other locations, the data resides within the health facility proper. In all cases, the personal health data did not leave the jurisdiction.
1.3.Regulatory compliance in the jurisdiction
Through a federated data-visiting set up, VODAN-A achieved compliance with data according to the regulatory framework in place in each jurisdiction [1]. Due to the sensitive nature of personal health data, full attention must be given to the rights and obligations under the regulatory frameworks in each jurisdiction in which the data is produced, in combination with the obligation to assign rights to the data subject, as part of the founding principles of VODAN-A.
Fig. 2.
OLR feeds into FAIR-Guidelines.
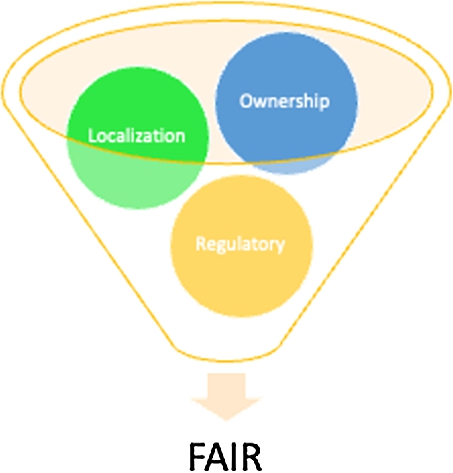
Fig. 3.
The FAIR-house completed with the foundation of localization (federated data) and delivering a rooftop for data ownership and regulatory compliance of data handling.
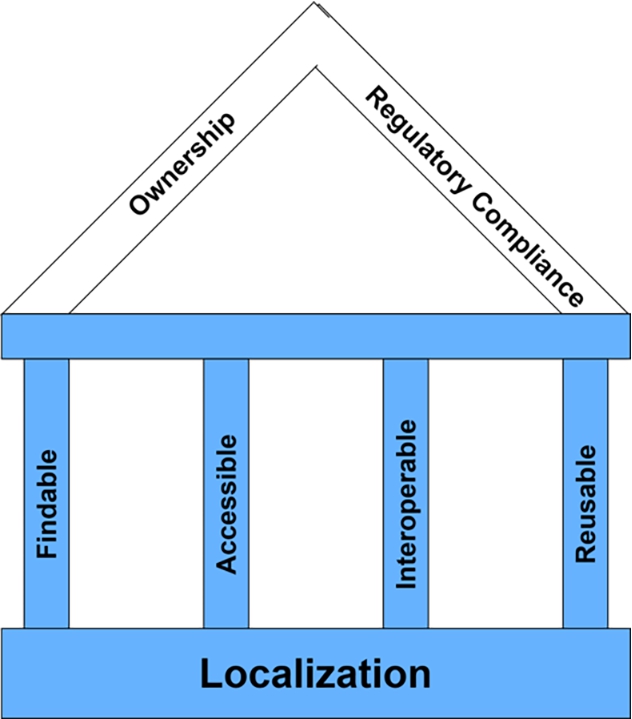
Ownership, Localization and Regulatory Compliance form an inherit part of the data-visiting FAIR-principles (Fig. 2). OLR allows data to be FAIR. OLR enables three critical aspects that are a condition for the FAIR-data architecture (Fig. 3: (i) federated localisation, which is critical for a data architecture where data is findable and associated with its provenance, (ii) data-ownership, which allows such data to remain of value to the data subject and (iii) the regulatory compliance, which allows data-visiting across different juridsictions. These are critical for transitioning FAIR-data curation into a FAIR-based Data Space. The OLR-principles provides the building blocks of the FAIR Africa Health Data Space restulting from ethical quality health data curated in and for the African continent.
2.Ownership, localisation and regulatory compliance: OLR
It is proposed that the acronym FAIR-OLR be used to refer to the set of basic qualities of a health information architecture that allows Ownership, Localisation and Regulatory Compliance (OLR) of the data in the respective jurisdictions. This includes respect for the Health Information System in place.
The distributed concept of FAIR-data under GDPR – at least in any data-setting that collaborates with the EU, makes intrinsic reference to the OLR principles. The purpose of coining FAIR-OLR is to propose that Ownership of Data, Localisation and Regulatory Compliance within each jurisdiction where data is produced is relevant as a consideration under the FAIR-Guidelines. FAIR-OLR refers to data that is federated, connected to the data subject, and under the responsibility of the data authority and processors where data is produced and deposited. The FAIR-OLR set of principles allows for data interoperability and reuse across borders in different jurisdictions and modes of operations, taking full advantage of the available semantic descriptions of the data [4], as well as its contextual qualities. FAIR-OLR advances the ethical and legal principles of personal data protection [11]. FAIR-OLR mitigates against health data being (systematically) removed from the African continent, and contributes to building health infrastructures that generate value from data for better health outcomes.
3.FAIR-OLR governance framework
FAIR-OLR provides a governance framework for FAIR tools and FAIR enabling tools, which are themselves FAIR [see Table 1). FAIR principles have clearly outlined how to make data FAIR. The Go-train, Go-build and Go-change modalities of GO FAIR set a foundation for focusing on how to create awareness, and build tools to move FAIR implementation forward [16]. The authors identified the gap in the governance of FAIR implementation during the design of vertical and horizontal interoperability across jurisdictions.
Table 1
FAIR-OLR principles
FAIR | Ownership | Localisation | Regulatory compliance |
Findable | Owned locally and findable globally | Locally deployable and globally findable | According to the regulatory frameworks in place in each jurisdiction and aligned with globally defined regulatory frameworks. |
Accessible | Defined and allowed locally to be accessed globally | Locally deployable and globally accessible | |
Interoperable | Knowledge and ontologies defined with local ownership | Knowledge and ontologies defined locally and stored globally and with copies stored locally | |
Reusable | Re-usable while ownership is protected | Local storage for global and local re-use |
The governance has local and global dimensions and identifies how local governance frameworks interplay with global frameworks. VODAN-Africa data production and use includes data processing agreements which relate to GDPR in terms of the principles, processes, and standards that a given local implementation follows, and the adherence of these with policy directions in the jurisdictions at hand [3,5,12,13]. In addition to the advantages of data being curated with FAIR-OLR guidelines, the tooling also needs to follow these guidelines. Global FAIR enabling tools need to be governed, as at some point the tools may evolve from being open source to closed source, or from being FAIR to otherwise, or may change standards without prior notice to the FAIR enabling resource using the tools. Looking at ontology services provided by BioPortal, lots of variants emerge (i.e. AgroPortal, VodanPortal); with growth in demand for the services and in the number of requests, such tools will develop.
During localisation there is a trade-off that needs to be made. Deploying globally available tools locally requires data stewardship skills and the availability of resources. There is a need to make what is locally available to be globally findable and accessible. There is also a need for FAIR-tooling to be deployed, owned and governed locally, and relatable globally in a federated manner. The application of the FAIR-OLR principles on the tooling will help to ensure sustainability, avoid downtime, reduce the cost of connectivity and other resource utilisation, and enhance performance. The FAIR-OLR principles foster reuse of existing and emerging FAIR enabling tools.
FAIR-OLR defines principles and specifications on how to handle the behaviour that arises as a result of the interaction of FAIR tools with other FAIR tools and with FAIR enabling tools across different locales and jurisdictions. Ways of handling involve issues such as performance, trust, security, logging of transaction, mediation and orchestration of interoperability.
4.An African health data space
FAIR-OLR is an iteration of the WHO proposition of SMART [8] health data. Building on the idea that the interoperability of digital health data might be a key aspect of the future of health innovation, it is critical to reflect on the nature of a Health Data Space in which this interoperability is shaped, as well as the qualities of the data that will populate it. The advantage of an African Health Data Space is maximised if based on an inclusive, ethical FAIR data pipeline, and that respects the ownership of health data by the data subject; the localisation of data to where such data is produced; and compliance with the regulatory frameworks in place.
5.Conclusions
Data-visiting is the process in which data is stored in a distributed or federated way and can be visited in residence by visiting algorithms, in federated data architectures, based on federated tooling adapted to the conditions in a specific location. Data-visiting architectures help enhance research of the possibilities of promoting the ownership of data (by the data subject/through the data producer with consent), the localisation of data production, and the regulatory compliance of data handling within the regulatory frameworks relevant in each jurisdiction. This is referred to as FAIR-OLR and helps build the trust of a federated, ethical data pipeline that produces quality data for machine-learning and AI purposes, for horizontal (within community) and vertical (between community) use. The FAIR-OLR is a WHO Smart iteration that will help set the guidelines for a Health Data Space and, importantly, an African Health Data Space. This notion recognises that the value of health data from Africa belongs in Africa and must be used for the enhancement of quality health within the African continent.
Funding
The authors received funding from Invest International for this research.
Conflict of interest
The authors have no conflict of interest to report.
References
[1] | https://allegrograph.com/ (accessed: 08.11.2022). |
[2] | S.Y. Amare, G.T. Taye, T.G. Gebreselassie and M. Van Reisen, Realising health data-interoperability in low connectivity settings: The case of VODAN-Africa, FAIR Connect. doi: 10.3233/FC-221510. |
[3] | M. Basajja, M. Van Reisen and F. Oladipo, FAIR Equivalency with regulatory framework for digital health in Uganda, Data Intelligence 4(4) (2022). doi: 10.1162/dint_a_00170. |
[4] | Based on onteporta a vodana portal was established, which produces owl semantic data. |
[5] | K. Chindoza, Regulatory framework for eHealth data policies in Zimbabwe: Measuring FAIR Equivalency, Data Intelligence 4(4) (2022). doi: 10.1162/dint_a_00173. |
[6] | P.H. Jati, M. van Reisen, E. Flikkenschild, F. Oladipo, B. Meerman, R. Plug and N.S. Data, access, control, and privacy protection in the VODAN-Africa architecture, Data Intelligence (2021), 1–29. |
[7] | A.A. Kawu, E. Joseph, I. Abdullahi, J.Y. Maipanuku, S. Folorunso, M. Basajja, F. Oladipo and H.L. Ibrahim, FAIR Guidelines and data regulatory framework for digital health in Nigeria, Data Intelligence 4(4) (2022). doi: 10.1162/dint_a_00174. |
[8] | G. Mehl, Ö. Tunçalp, N. Ratanaprayul, T. Tamrat, M. Barreix, D. Lowrance, K. Bartolomeos, L. Say, N. Kostanjsek, R. Jakob and J. Grove, WHO SMART guidelines: Optimising country-level use of guideline recommendations in the digital age, The Lancet Digital Health 3: (4) ((2021) ), e213–e216. |
[9] | P.H. Purnama Jati, FAIR Equivalency in Indonesia’s digital health framework, Data Intelligence 4(4) (2022). doi: 10.1162/dint_a_00171. |
[10] | EU. 2016. Regulation (EU) 2016/679 of the European Parliament and of the Council of 27 April 2016 on the protection of natural persons with regard to the processing of personal data and on the free movement of such data, and repealing Directive 95/46/EC (General Data Protection Regulation) (Text with EEA relevance) OJ L 119, 4.5.2016, p. 1–88. |
[11] | EU. 2016. Regulation (EU) 2016/679 of the European Parliament and of the Council of 27 April 2016 on the protection of natural persons with regard to the processing of personal data and on the free movement of such data, and repealing Directive 95/46/EC (General Data Protection Regulation) (Text with EEA relevance) OJ L 119, 4.5.2016, p. 1–88. |
[12] | G.T. Taye, Y.S. Amare, G.T. Gebremeskel, A.A. Medhanyie, W. Ayele, T. Habtamu and M. Van Reisen, FAIR Equivalency with regulatory framework for digital health in Ethiopia, Data Intelligence 4(4) (2022). doi: 10.1162/dint_a_00172. |
[13] | E.I. Thea, R. Nalugala, W. Nandwa, F. Obwanda, A. Wachira and A.M. Cartaxo, FAIR Equivalency, regulatory framework and adoption potential of FAIR Guidelines in health in Kenya, Data Intelligence 4(4) (2022). doi: 10.1162/dint_a_00175. |
[14] | M. Van Reisen, F. Oladipo, M. Mpezamihigo, R. Plug, M. Basajja, A. Aktau, P.H. Purnama Jati, R. Nalugala, S. Folorunso, Y.S. Amare, I. Abdulahi, O.O. Afolabi, E. Mwesigwa, G.T. Taye, A. Kawu, M. Ghardallou, Y. Liang, O. Osigwe, A.A. Medhanyie and M. Mawere, Incomplete COVID-19 data: The curation of medical health data by the Virus Outbreak Data Network-Africa, Data Intelligence 4(4) (2022). doi: 10.1162/dint_e_00166. |
[15] | M. Van Reisen, F. Oladipo, M. Stokmans, M. Mpezamihigo, S. Folorunso, E. Schultes et al., Design of a FAIR digital data health infrastructure in Africa for COVID-19 reporting and research, Advanced Genetics 2(2) (2021). doi: 10.1002/ggn2.10050. |
[16] | M. Van Reisen, M. Stokmans, M. Basajja, A.O. Ong’ayo, C. Kirkpatrick and B. Mons, Towards the tipping point of FAIR implementation, Data Intelligence 2: ((2020) ), 264–275. doi: 10.1162/dint_a_00049. |
[17] | VODAN-Africa. Available from: https://vodan-totafrica.info/vodan-news-page.php?i=48&a=vodan-africa-to-deploy-digital-health-systems-across-africa (accessed: 10.10.2022). |
[18] | M. Wilkinson, M. Dumontier, I.J. Aalbersberg, G. Appleton, M. Axton, A. Baak et al., The FAIR guiding principles for scientific data management and stewardship, Scientific Data 3: (1) ((2016) ), 1–9. doi: 10.1038/sdata.2016.1. |