Involvement of FAM170B-AS1, hsa-miR-1202, and hsa-miR-146a-5p in breast cancer
Abstract
BACKGROUND:
FAM170B-AS1 is usually expressed low in all organs except for testicular tissues. No study was performed to explore its role in breast cancer (BC). Contradictory results were reported about hsa-miR-1202 and hsa-miR-146a-5p in BC.
OBJECTIVE:
The present study aimed to explore the involvement of FAM170B-AS1 in BC using bioinformatics predictive tools, followed by a practical validation besides exploring the impact of hsa-miR-1202 and hsa-miR-146a-5p in BC.
METHODS:
This study enrolled 96 female patients with BC, 30 patients with benign breast diseases (BBD), and 25 control subjects. The expressions of circulating FAM170B-AS1, hsa-miR-1202, and hsa-miR-146a-5p were quantified using qRT-PCR. These ncRNAs’ associations, predictive, and diagnostic roles in BC were statistically tested. The underlying miRNA/mRNA targets of FAM170B-AS1 in BC were bioinformatically predicted followed by confirmation based on the GEPIA and TCGA databases.
RESULTS:
The expression of FAM170B-AS1 was upregulated in sera of BC patients and hsa-miR-1202 was upregulated in sera of BBD and BC patients while that of hsa-miR-146a-5p was downregulated in BC. These FAM170B-AS1 was significantly associated with BC when compared to BBD. FAM170B-AS1 and hsa-miR-1202 were statistically associated with the BC’s stage, grade, and LN metastasis. FAM170B-AS1 and hsa-miR-146a-5p gave the highest specificity and sensitivity for BC. KRAS and EGFR were predicted to be targeted by FAM170B-AS1 through interaction with hsa-miR-143-3p and hsa-miR-7-5p, respectively. Based on the TCGA database, cancer patients having mutations in FAM170B show good overall survival.
CONCLUSIONS:
The present study reported that for the first time, FAM170B-AS1 may be a potential risk factor, predictive, and diagnostic marker for BC. In addition, FAM170B-AS1 might be involved in BC by interacting with hsa-miR-143-3p/KRAS and hsa-miR-7-5p/EGFR through enhancement or repression that may present a new therapeutic option for BC.
Abbreviations
AUC | Area under the curve |
BBD | Benign breast diseases |
BC | Breast cancer |
BP | Biological process |
CA15.3 | Carbohydrate antigen 15.3 |
CC | Cellular component |
CEA | Carcinoembryonic antigen |
CDC14A | Cell division cycle 14 homolog |
CI | A Confidence interval |
DCI | Ductal carcinoma in situ |
EMT | Epithelial to mesenchymal |
transition | |
ER | Estrogen receptor |
FAM170B-AS1 | Family with sequence similarity |
170 member B-antisense RNA1 | |
GEPIA | Gene expression profiling |
integrative analysis | |
GO | Gene Ontology |
GTEx | Genotype-Tissue Expression |
HCC | Hepatocellular carcinoma |
HER-2 | Human epidermal growth factor |
receptor-2 | |
HPA | Human protein atlas |
IDC | Invasive ductal carcinoma |
KEGG | Kyoto Encyclopedia of Genes and |
Genomes | |
LDAP | LncRNA-disease association |
prediction | |
LN | Lymph node |
LncRNA | Long non-coding RNA |
MF | molecular function |
miRNA | Micro RNA |
mRNA | Messenger RNA |
ncRNAs | Non-coding RNAs |
NSCLC | Non-small cell lung cancer |
OR | Odds ratio |
PPI | Protein-protein interaction |
PR | Progesterone receptor |
ROC | Receiver operating characteristic |
curve | |
TCGA | The cancer genome atlas |
TNBC | Triple negative breast cancer |
TNM | Tumor-node-metastasis |
VIF | Variance inflation factor |
| Standardized coefficient |
1.Introduction
Being the most common cancer globally among women makes breast cancer (BC) the first cause of cancer-related deaths [1]. About 2.2 million new cases are diagnosed with BC. Therefore, BC accounts for 11.7% of all cancer incidence [2]. In 2019, about 59% of women diagnosed with BC were
The presence of a variety of genetic aberrations against BC makes it a very heterogeneous disease [7]. Hence, BC has five main molecular subtypes identified depending on the level of expression of receptors for estrogen (ER), progesterone (PR), and human epidermal growth factor receptor-2 (HER-2) on the surface of BC cells [7, 8]. Some of these subtypes are marked by significantly aggressive clinical course, proliferative activity and growth rate, poor prognosis, early metastasis, different targeted therapies, and risk of disease recurrence [9, 10, 11, 12, 13]. Hence, early screening and diagnosing the more aggressive subtypes of BC and identifying suspicious breast tumors are vital to reduce morbidity and mortality [14, 15].
Long noncoding RNAs (lncRNAs) and microRNAs (miRNAs) are reported to be associated with different types of cancer [16, 17]. LncRNAs can suppress mRNA expression by recruiting histone modification complexes, controlling the alternative splicing, and nuclear import [18]. LncRNAs can interact with miRNAs [19, 20, 21] to de-repress gene expression by competing with miRNAs for interaction with shared target mRNAs. miRNA binds to its complementary sequences in the 3’-UTR of their target mRNAs and post-transcriptionally represses their translation into protein [22]. Therefore, predicting and validating the lncRNA/miRNA crosstalk can modulate gene expression patterns that drive physiological and pathological processes [23, 24, 25].
Little is known about the Family with sequence similarity 170 member B-antisense RNA1 (FAM170B-AS1; ENSG00000234736). It is located on chromosome 10: 49,121,839-49,151,547 forward strand with four transcripts (https://www.ensembl.org/index.html). Genotype-Tissue Expression portal (https://gtexportal.org/home/) shows the low expression of FAM170B-AS1 in all body tissues except for the testis. It also reveals that FAM170B-AS1 is expressed in the breast epithelial (luminal) cells. In 2018, 54 single nucleotide polymorphisms (SNPs) in 21 genes were recognized as independent and significant determinants of coronary artery disease. FAM170B-AS1, one of these 21 genes, was identified for the first time as a possible genetic risk factor for coronary artery disease in Japanese patients [26]. Ke et al. [27] and Zhou et al. [28] reported that FAM170B-AS1 is one of the differentially expressed genes in the skeletal muscle of diabetic patients and the monocyte-derived dendritic cells of patients with allergic rhinitis, respectively. Recently, bioinformatics analysis was performed by Hu et al. [29] to explore the hub mechanisms of ischemic stroke and illustrated the involvement of FAM170B-AS1 as one of 406 genes in ischemic stroke. Falcon et al. [30] and Zhang et al. [31] pointed out the participation of FAM170B-AS1 in hepatocellular carcinoma (HCC) and thyroid carcinoma, respectively, based on the data extracted from the cancer genome atlas (TCGA) database. It was reported that FAM170B-AS1 may cause homeostasis imbalance in HCC by allowing cancer cells to be affected by oxidative stress or even dysregulating cancer cell division rate [30, 32]. Recently, a significant alteration in the frequency of FAM170B-AS1 was reported and found to be correlated with allograft inflammatory factor 1 in pan-cancer based on bioinformatics analysis [33]. These bioinformatics studies opened a new window to clinical study of the possible roles of FAM170B-AS1 in several types of cancer.
hsa-miRNA-1202 and hsa-miRNA-146a were reported to be associated with cancer progression, positively or negatively. hsa-miRNA-1202 is transcriped by MIR1202 gene on chromosome 6: 155,946,797-155,946,879 forward strand. It is reported to be upregulated in breast cancer [34] endometrial cancer [35], and lung cancer [36] while it is revealed to be downregulated in glioma cells [37] and HCC [38]. hsa-miR-1202 was reported to inhibit the proliferation of glioma cells and induce endoplasmic reticulum stress and apoptosis via regulating Rab1A in glioma [37]. Another study reported the inhibitory role of hsa-miR-1202 on HCC cell migration and invasion by targeting cyclin-dependent kinase 14 [38].
hsa-miR-146a is transcribed by the MIR146A gene on chromosome 5: 160, 485, 352-160, 485, 450 forward strand. Wu et al. [39] indicated that hsa-miR-146a-5p is downregulated in non-small cell lung cancer (NSCLC) tissues, whereas Wang et al. [40] reported an up-regulation of hsa-miR-146a-5p in NSCLC patients. Liu et al. [41] revealed the oncogenic mechanism of hsa-miR-146a-5p in NSCLC cell survival and migration by suppressing its target gene, tumor necrosis factor receptor-associated factor 6. In BC, several studies indicated that hsa-miR-146a can function as oncomiR [42, 43, 44, 45]. Other studies have demonstrated hsa-miR-146a as a tumor suppressor miRNA [46, 47]. The reports of Park et al. [48] and Kumaraswamy et al. [49] illustrated the tumor-suppressive effect of hsa-miR-146a via inhibiting epithelial-to-mesenchymal transition (EMT) and the metastatic potential in many cancer types, including BC. It has been proposed that hsa-miR-146a can sustain an epithelial phenotype in triple negative breast cancer (TNBC) via direct targeting of the TGF
To the best of our knowledge, the current study aimed to predict and validate the role of FAM170B-AS1 for the first time in breast cancer using the bioinformatics tool and QPCR, respectively, to construct FAM170B-AS1/miRNA/mRNA network. Another goal of the present study is to explore the roles of both hsa-miR-1202 and hsa-miR-146a-5p in BC.
2.Subjects and methods
2.1Subjects
A total of 151 subjects were enrolled in the current study and divided into three groups: a control group (
Patients were chosen from those admitted to the National Cancer Institute, Cairo University, and Clinical Oncology Department, Faculty of Medicine, Ain Shams University, Cairo, Egypt. Full history and the pathological examinations of the breast mass from patients either with BBD or BC was obtained from their clinical sheets. The stages of BC, performed according to the tumor-node-metastasis classification system (TNM), were obtained from the clinical sheets. Furthermore, the expression of hormonal receptors (PR, ER, and HER-2) on the breast cancer tissue was examined using immunohistochemistry to identify the molecular subtype of BC.
The study was approved by the Scientific Research Ethics Committee, National Research Center, Dokki, Giza, Egypt (ID: 15209). Informed consent for using the samples for research purposes was obtained from all participants before beginning the study. The research was carried out under the Declaration of Helsinki, printed in the British Medical Journal (18 July 1964).
2.2Blood sample collection
Three milliliters blood samples were withdrawn from individuals in tubes with polymer gel and clot activator (Greiner bio-one, GmbH, Australia). The blood was left to clot at 37∘C for 30 minutes, and all samples were centrifuged at 10,000
2.3Tumor markers assay
The serum venous levels of carcinoembryonic antigen (CEA) and carbohydrate antigen 15.3 (CA 15.3) were estimated using commercial enzyme-linked immunosorbent assay kits provided by Immunospeccorporation, Netherlands.
2.4Prediction of FAM170B-AS1 association with breast cancer
Predicting the association of FAM170B-AS1 in different diseases was performed using miRWalk v.2 (http:// mirwalk.umm.uni-heidelberg.de/), released in 2019.
2.5Quantitative RT-PCR for hsa-miR-146a-5p and hsa-miR-1202 assays and FAM170B-AS1 validation
MiRNA was extracted from the sera of all the participants using an RNeasy mini kit (Qiagen, USA). The purity of the extracted RNA was detected at 260/280 nm using a nano-drop spectrophotometer (Quawell, Q-500, Scribner, USA). The extracted miRNA was reverse transcribed into cDNA using a MiScript II reverse transcription kit provided by Qiagen, USA. cDNA synthesis was performed using a thermal cycler (SureCycler 8800, Agilent, USA).
Real-time quantitative PCR was performed to analyse the expression of hsa-miR-1202 (HS_hsa-miR-1202 miScript Primer Assay, Cat no.MP00000259), hsa-miR-146-5p (HS_hsa-miR-146-5p miScript Primer Assay, Cat no.MS00003535), and FAM170B-AS1 (RT2 lncRNA qPCR Assay for Human, cat no. LPH11337A) using SYBR Green/ROX Master Mix (Qiagen, USA). Ready-made primers for the studied ncRNAs as well as their housekeeping genes [RNU6 for miRNA (HS_RNU6-2-11_ miScript Primer Assay, Cat no. MS00033740) and GAPDH for lncRNA (Hs_GPDH_1_SG QuantiTect Primer Assay, Cat no. QT00079247)] were provided from Qiagen, USA. The thermal reaction conditions were 95∘C for 15 min followed by 40 cycles at 94∘C for 15 s, 55∘C for 30 s, and 70∘C for 34 s using Max3005P QPCR system, Stratagene, Agilent biotechnology, USA. Expression for investigated miRNAs and lncRNA was assessed using the
2.6Targeted prediction and functional analysis
LncBase predicted v2 database (https://dianalab.e-ce.uth.gr/html/diana/web/index.php?r=lncbasev2%2Findex-predicted) was used to identify the target miRNAs of FAM170B-AS1 and their binding sites where the threshold was adjusted at 0.5. In addition, four target prediction algorithms were used to predict the interactions between miRNAs and genes associated with breast cancer. The four corresponding prediction algorisms were miRWalk v.2 (http://mirwalk.umm.uni-heidelberg.de/) database released in 2022, miRDB (http://www.mirdb.org/), TargetScan 7.2 (https://www.targetscan.org/vert_72/), and miRTarBase (https://mirtarbase.cuhk.edu.cn/∼miRTarBase/miRTarBase_2022/php/index.php). The overlapped miRNAs were identified via Venn diagrams (https://bioinformatics.psb.ugent.be/webtools/Venn/). The target genes of the overlapped miRNAs from LncBase Predicted v.2, miRWalk v.2, miRDB, TargetScan 7.2, and miRTarBase were identified by Excel function to construct FAM170B-AS1/miRNA/mRNA network in breast cancer.
To further consider the potential functions, pathways, and networks of these target genes, the DAVID database (https://david.ncifcrf.gov/tools.jsp) was used for Gene Ontology (GO) annotation and Kyoto Encyclopedia of Genes and Genomes (KEGG) pathway analysis. GO was divided into three functional groups: biological process (BP), cellular component (CC), and molecular function (MF). Cytoscape (version 3.9.0, https://cytoscape.org/) was applied to construct the functional FMA170B-AS1/miRNA/mRNA network.
In addition, the human protein atlas (HPA) (https://www.proteinatlas.org/) was used to explore the protein expression of target genes in breast cancer and normal breast tissues. The online database STRING v.11.5 (https://string-db.org/) was used to build protein-protein interaction (PPI) networks using the overlapped target genes of proteins expressed in breast tissue. The minimum required interaction pairs were adjusted at
2.7Correlation to GEPIA and TCGA data
Gene expression profiling integrative analysis (GEPIA) was used to investigate the expression level of the selected target genes in normal breast tissue and BRCA tumors. Furthermore, GEPIA was utilized to analyse the correlation between the FAM170B-AS1 gene and the target genes in the BRCA tumor. In addition, the genetic alteration of FAM170B in primary cancers was investigated based on TCGA with their overall survival analysis.
2.8Statistical analysis
The power of the current study was calculated utilizing G∗Power 3.1.9.7 software (Franz Faul, Universität Düsseldorf, Germany) (https://www.psychologie.hhu.de/arbeitsgruppen/allgemeine-psychologie-und-arbeitspsychologie/gpower). The test family was adjusted at
The Statistical Package for Social Science version 26 for Windows (SPSS software package, Chicago, USA) was used to perform all statistical analyses. The data distribution was statistically determined using the Kolmogorov-Smirnov test with Lilliefors significance correction. The categorical variables were expressed as frequencies (percentages), and non-parametric data were expressed as median (interquartile range). The non-parametric variables were statistically analysed using the Kruskal-Wallis one-way ANOVA (all pairwise) test for multiple comparisons.
Binary logistic regression analyses were used to investigate the strength of the association of the expression of FAM170B-AS1, hsa-miR-1202, and hsa-miR-146a-5p with the susceptibility to breast tumors between either benign breast lesion versus control group, malignant tumor versus control group, and malignant tumor versus benign breast lesion. The strength of associations was measured by the odds ratio (OR) with a 95% confidence interval (CI) adjusted for age and menopause. Multiple linear regression analysis was used to assess the association of the independent variables relative to the dependent variables after transforming non-parametric variables into the logistic scale. The regression models were constructed using the “enter” analysis.
The receiver operating characteristic curve (ROC) was performed using SPSS vs. 26 to detect the area under the curve (AUC), cutoff points, sensitivity, and specificity for the tested miRNA-1202, miRNA-146a-5P, and FAM10B-AS1 between BC, BBD, and control groups, compared to the traditional tumor markers. All
3.Results
The clinical characteristics of all individuals in the studied groups are listed in Table 1. Concerning patients with BBD, 36.7% were identified to have follicular hyperplasia, 26.7% had fibrocytic changes, and 36.7% had intraductal papillomatosis. Of women with BC, 38.5, 34.4, 35.4, and 47.9% were diagnosed to be at the early stage with low-grade, ductal carcinoma in situ (DCI) and no lymph node (LN) metastasis, respectively,
Table 1
Demographic characteristics of subjects in the different studied groups
Control ( | BBD ( | BC ( | |||
---|---|---|---|---|---|
Age (years) | 53 (13) | 50 (19) | 50 (18) | 0.915 | |
Menopause | |||||
Yes | 6 (24) | 18 (60) | 34 (35.40) | 1.56 | 0.436 |
No | 19 (76) | 12 (40) | 62 (64.60) | ||
Stage | |||||
Early | 37 (38.5) | 151 | |||
Late | 59 (61.5) | ||||
Grade | |||||
Low grade | 33 (34.4) | 151 | |||
High grade | 63 (65.6) | ||||
Pathological types | |||||
Follicular hyperplasia | 11 (36.7) | 302 | |||
Fibrocytic changes | 8 (26.7) | ||||
Intraductal papillomatosis | 11 (36.7) | ||||
DCI | 34 (35.4) | ||||
IDC | 62 (64.6) | ||||
Molecular subtypes | |||||
Luminal A (ER) | 6 (6.3) | 151 | |||
Luminal A (PR) | 2 (2.1) | ||||
Luminal B (ER, HER-2) | 2 (2.1) | ||||
Luminal B (PR, HER-2) | 19 (19.8) | ||||
Luminal B-like (ER, PR, HER-2) | 45 (46.9) | ||||
Basal-like (triple negative) | 22 (22.9) | ||||
LN metastasis | |||||
No | 46 (47.9) | 151 | |||
Yes | 50 (52.1) |
Data are expressed as Median (INTER QUARTILE RANGE) for non-parametric variables and frequencies (percentages) for categorical variables. BBD: benign breast diseases, BC: breast cancer, DCI: ductal carcinoma in situ, IDC: invasive ductal carcinoma, ER: estrogen receptor, PR: progesterone receptor, HER-2: human epidermal growth factor receptor-2, LN: lymph node. The mean difference is significant at
Table 2
Comparison of the levels of tumor markers as well as FAM170B-AS1, hsa-miR-1202, and hsa-miR-146a-5p expression in the different studied groups
Control ( | BBD ( | BC ( | ||
---|---|---|---|---|
CEA (pg/ml) | 8 | (5) | 14.2 (7.3) | 12 (8.7) |
0.024a | 0.014a, 1.000b | |||
Fold change | 1.78∗ | 1.5∗/0.85# | ||
CA15.3 (pg/ml) | 12.00 | (3.00) | 13.00 (9.00) | 22.00 (s10.00) |
0.379a | ||||
Fold change | 1.08∗ | 1.83∗/1.69# | ||
FAM170B-AS1 expression | 0.53 | (0.22) | 0.88 (0.27) | 12.85 (7.25) |
0.208a | ||||
Fold change | 1.66∗ | 24.25∗/14.60# | ||
hsa-miR-1202 expression | 5.72 | (1.44) | 19.50 (28.83) | 56.51 (82.29) |
0.005a | ||||
Fold change | 3.41∗ | 9.88∗/2.90# | ||
miR-146a-5p expression | 360 | (184.50) | 220 (180.00) | 31 (41.00) |
0.403a | ||||
Fold change | 0.61∗ | 0.09∗/0.14# |
Data are expressed as median (interquartile range). BBD: benign breast diseases, BC: breast cancer, CEA: carcinoembryonic antigen, CA 15.3: carbohydrate antigen 15.3. a: significance versus control group, b: significance versus benign breast diseases group. ∗: fold change versus control group, # : fold change versus benign breast diseases group. Significance had been adjusted by Bonferroni correction for multiple tests. The mean difference is significant at
Table 3
Relationship between CEA and CA15.3 levels as well as ncRNAs expression in serum with clinicopathological parameters of patients with breast benign diseases
Clinical pathological criteria | CEA level (pg/ml) | CA15.3 level (pg/ml) | FAM170B-AS1 expression | hsa-miR-1202 expression | hsa-miR-146a-5p expression | ||||||
Age | 15 | 13 | 0 | .88 | 10 | .10 | 220 | ||||
13 | 12 | 0 | .88 | 21 | .60 | 150 | .00 | ||||
0 | .214 | 0 | .121 | 0 | .583 | 0 | .916 | 0 | .255 | ||
Menopause status | Yes | 13 | .6 | 12 | 0 | .93 | 21 | .60 | 190 | .00 | |
No | 15 | 13 | 0 | .88 | 14 | .8 | 220 | .00 | |||
0 | .701 | 0 | .237 | 0 | .347 | 0 | .608 | 0 | .495 | ||
Pathological types | Follicular hyperplasia | 12 | 12 | 0 | .88 | 35 | .51 | 220 | .00 | ||
Fibrocytic changes | 15 | .55 | 17 | 0 | .88 | 28 | .55 | 130 | .50 | ||
Intraductal papillomatosis | 13 | 13 | 0 | .88 | 10 | .10 | 287 | .00 | |||
0 | .110 | 0 | .667 | 0 | .823 | 0 | .278 | 0 | .222 |
Data are expressed as a median. CEA: carcinoembryonic antigen, CA15.3: carbohydrate antigen 15.3. Significance had been adjusted by Bonferroni correction for multiple tests.
Table 4
Relationship between CEA and CA15.3 levels in serum with clinicopathological parameters of patients with breast cancer
Clinical pathological criteria | CEA level (pg/ml) | CA15.3 level (pg/ml) | |||
---|---|---|---|---|---|
Age | 14 | 0.036 | 22 | 0.562 | |
10.00 | 22 | ||||
Menopause status | Yes | 10.50 | 0.063 | 22.00 | 0.706 |
No | 12 | 22 | |||
Stage | Early | 12.00 | 0.561 | 23.00 | 0.553 |
Late | 11.00 | 22.00 | |||
Grade | Low | 10.00 | 0.069 | 22.00 | 0.559 |
High | 12.00 | 22.00 | |||
Pathological types | DCI | 11.00 | 0.200 | 22.00 | 0.994 |
IDC | 12.00 | 22.00 | |||
Molecular subtypes | ER | 7.00 | 0.248 | 22.00 | 0.098 |
PR | 2.30 | 14.00 | |||
ER, HER-2 | 9.00 | 35.00 | |||
PR, HER-2 | 15.30 | 21.00 | |||
ER, PR, HER-2 | 11.00 | 22.00 | |||
Basal-like | 12.00 | 23.00 | |||
LN metastasis | No | 11.00 | 0.597 | 18.00 | 0.133 |
Yes | 12.00 | 22.00 |
Data are expressed as a median. DCI: ductal carcinoma in situ, IDC: invasive ductal carcinoma, ER: estrogen receptor-positive, PR: progesterone receptor positive, HER-2: human epidermal growth factor receptor-2 positive, LN: lymph node. Significance had been adjusted by Bonferroni correction for multiple tests.
Table 5
Relationship between FAM170B-AS1, hsa-miR-1202, and hsa-miR-146a-5p expression with clinicopathological parameters of patients with breast cancer
Clinical pathological criteria | FAM170B-AS1 expression | hsa-miR-1202 expression | hsa-miR-146a-5p expression | ||||
---|---|---|---|---|---|---|---|
Age | 12.85 | 0.046 | 52.4 | 0.991 | 49 | ||
12.85 | 81.5 | 20 | |||||
Menopause status | Yes | 12.85 | 0.452 | 59.71 | 0.713 | 20 | 0.001 |
No | 12.85 | 53.3 | 42.00 | ||||
Stage | Early | 12.85 | 0.501 | 36.80 | 20.00 | 0.01 | |
Late | 12.85 | 91.30 | 44.00 | ||||
Grade | Low | 12.85 | 0.666 | 33.13 | 19.00 | ||
High | 12.85 | 96.34 | 44.00 | ||||
Pathological types | DCI | 12.85 | 0.143 | 33.13 | 19.50 | 0.001 | |
IDC | 12.85 | 93.82 | 44.50 | ||||
Molecular subtypes | ER | 12.85 | 0.084 | 24.10 | 19.50 | 0.038 | |
PR | 9.27 | 15.30 | 32.00 | ||||
ER, HER-2 | 4.70 | 52.40 | 40.00 | ||||
PR, HER-2 | 12.85 | 38.05 | 32.00 | ||||
ER, PR, HER-2 | 12.85 | 104.10 | 45.00 | ||||
Basal-Like | 12.85 | 41.05 | 19.50 | ||||
LN metastasis | No | 12.85 | 0.378 | 33.80 | 44.50 | 0.005 | |
Yes | 12.85 | 91.30 | 25.00 |
Data are expressed as a median. DCI: ductal carcinoma in situ, IDC: invasive ductal carcinoma, ER: estrogen receptor-positive, PR: progesterone receptor positive, HER-2: human epidermal growth factor receptor-2 positive, LN: lymph node. Significance had been adjusted by Bonferroni correction for multiple tests.
Table 6
Association of FAM170B-AS1, hsa-miR-1202, and hsa-miR-146a-5p expression with breast cancer risk, compared with the traditional tumor markers
BBD vs. control | BC vs. control | BC vs. BBD | ||||
---|---|---|---|---|---|---|
# Adjusted OR (95% CI) | # Adjusted OR (95% CI) | # Adjusted OR | ||||
(95% CI) | ||||||
CEA | 0.80 (0.69–0.93) | 0.004 | 0.90 (0.85–0.98) | 0.011 | 1.01 (0.941–1.08) | 0.848 |
CA15.3 | 0.89 (0.79–1.00) | 0.054 | 0.87 (0.81–0.94) | 0.93 (0.88–0.98) | 0.005 | |
FAM170B-AS1 | 0.00 (0.00–0.03) | 0.00 (0.00–0.00) | 0.989 | 0.33 (0.19–0.58) | ||
hsa-miR-1202 | 0.52 (0.33–0.80) | 0.003 | 0.56 (0.35–0.88) | 0.013 | 0.96 (0.95–0.98) | |
hsa-miR-146a-5p | 1.01 (1.00–1.01) | 0.008 | 1.08 (1.02–1.14) | 0.008 | 1.05 (1.03–1.07) |
BC: breast cancer, BBD: benign breast diseases, OR: odds ratio, 95% CI: 95% confidence interval. # : Adjusted for age and menopause.
Table 7
Multiple linear regression analysis for the association between FAM170B-AS1 and the studied miRNAs and the association of the tumor markers as well as the studied genes with the stage, grade, and LN metastasis
Dependent variable | Predictors |
|
|
|
|
| VIF | ||
---|---|---|---|---|---|---|---|---|---|
FAM170B-AS1 expression | hsa-miR-1202 expression | 0.67 | 0.44 | 118.00 | 0.67 | 10.86 | 1.00 | ||
hsa-miR-146a-5p expression | 0.80 | 0.64 | 288.66 | 1.00 | |||||
Stage | CEA | 0.87 | 0.76 | 89.52 | 0.44 | 1.09 | |||
CA 15.3 | 0.957 | 1.16 | |||||||
FAM170B-AS1 expression | 0.66 | 7.86 | 4.14 | ||||||
hsa-miR-1202 expression | 0.27 | 4.79 | 1.92 | ||||||
hsa-miR-146a-5p expression | 0.851 | 3.15 | |||||||
Grade | CEA | 0.88 | 0.78 | 103.40 | 0.700 | 1.09 | |||
CA 15.3 | 0.01 | 0.20 | 0.839 | 1.16 | |||||
FAM170B-AS1 expression | 0.67 | 8.40 | 4.14 | ||||||
hsa-miR-1202 expression | 0.33 | 6.20 | 1.92 | ||||||
hsa-miR-146a-5p expression | 0.05 | 0.75 | 0.457 | 3.15 | |||||
LN metastasis | CEA | 0.86 | 0.73 | 79.54 | 0.242 | 1.09 | |||
CA 15.3 | 0.06 | 1.35 | 0.179 | 1.16 | |||||
FAM170B-AS1 expression | 0.66 | 7.59 | 4.14 | ||||||
hsa-miR-1202 expression | 0.28 | 4.70 | 1.92 | ||||||
hsa-miR-146a-5p expression | 0.04 | 0.55 | 0.580 | 3.15 |
Data represented in Supplementary file Table S1 predicts the association of FAM170B-AS1 with different types of cancer in Homo Sapiens species. Using the lncRNA-disease association prediction (LDAP) method, FAM170B-AS1 was anticipated to be associated with breast cancer with a 0.1828 score.
Table 2 shows the expression levels of FAM170B-AS1, hsa-miR-1202, and hsa-miR-146a-5p in the sera of all the studied groups, compared to the serum levels of CEA and CA15.3 (traditional tumor markers). There were significant elevations in the serum level of CEA in both BBD patients (1.78-fold change,
Table 3 shows no significant difference (
Both the serum levels of CEA and CA15.3 were found to be similar (
Data in Table 5 illustrate the difference between the expression of the studied ncRNAs among BC patients with their age, menopause status, stage, grade, molecular subtypes, and LN metastasis. No significant difference was recorded in the expression of hsa-miR-1202 in BC patients with
The associations of the tumor markers and the studied ncRNAs with BBD and BC are illustrated in Table 6 using binary logistic regression analysis. The serum level of CA15.3 and the expression level of hsa-miR-146a-5p were not significantly associated with BBD (
Multiple linear regression analysis (Table 7) reported a significantly positive correlation between FAM170B-AS1 expression and the expression of hsa-miR-1202
Table 8 and Fig. 1 demonstrate the results of the ROC curve of the traditional tumor markers and the studied ncRNAs when comparing BC patients with non-BC patients (Control group
Table 8
The ROC curve plot of CEA, CA15.3, FAM170B-AS1, hsa-miR-1202, and hsa-miR-146a-5P in BC group, compared to non-BC individuals (control group
C | AUC | Specificity (%) | Sensitivity (%) | |
---|---|---|---|---|
CEA | 0.571 | 0.147 | 65.6 | 63.6 |
CA15.3 | 0.765 | 100 | 65.3 | |
FAM170B-AS1 | 0.992 | 100 | 97 | |
hsa-miR-1202 | 0.893 | 100 | 64.9 | |
hsa-miR-146a-5p | 0.967 | 100 | 88.9 |
AUC: area under the curve, CEA: carcinoembryonic antigen, CA 15.3: carbohydrate antigen 15.3.
Figure 1.
The receiver operating characteristic curve of all tested markers in BC group compared to the groups of control
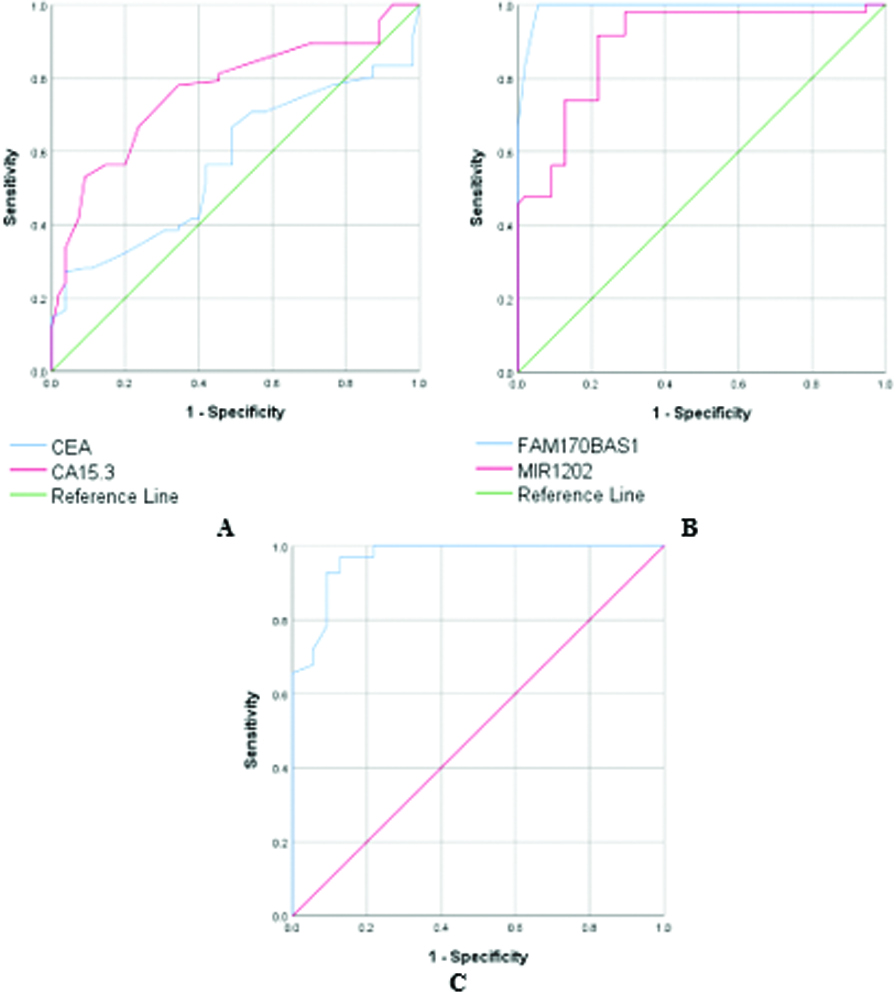
Figure 2.
A: Venn diagram identifies the overlapped miRNAs targeted by FAM170B-AS1 and associated with breast cancer identified. B: FAM170B-AS1-overlapped miRNA-mRNA network in breast cancer and constructed by Cytoscape.
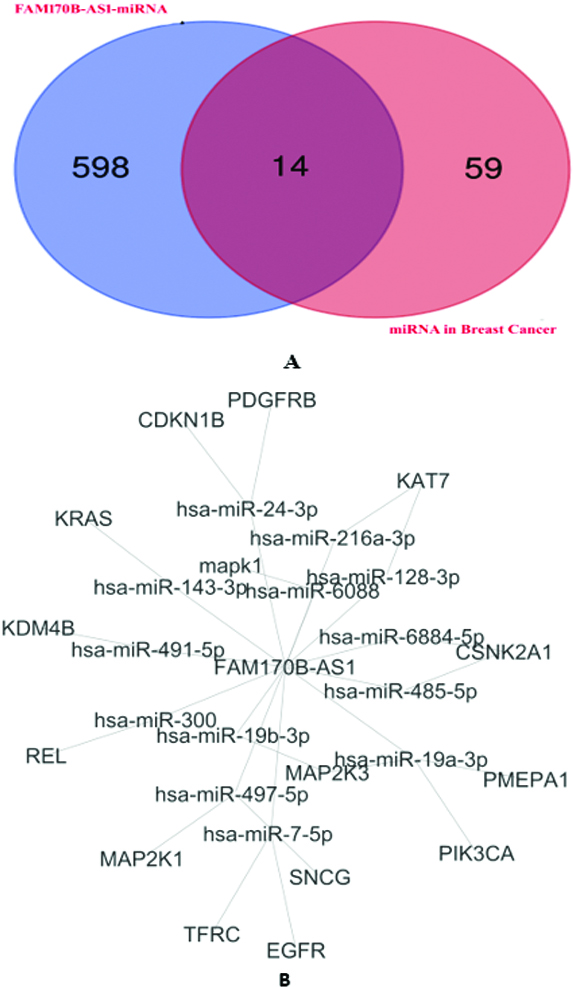
Figure 3.
Correlation between the gene expression of FAM170B-AS1 with MAPK1 (A), EGFR (B), MAP2K1 (C), and KRAS (D) in BRCA tumor based on the GEPIA database.
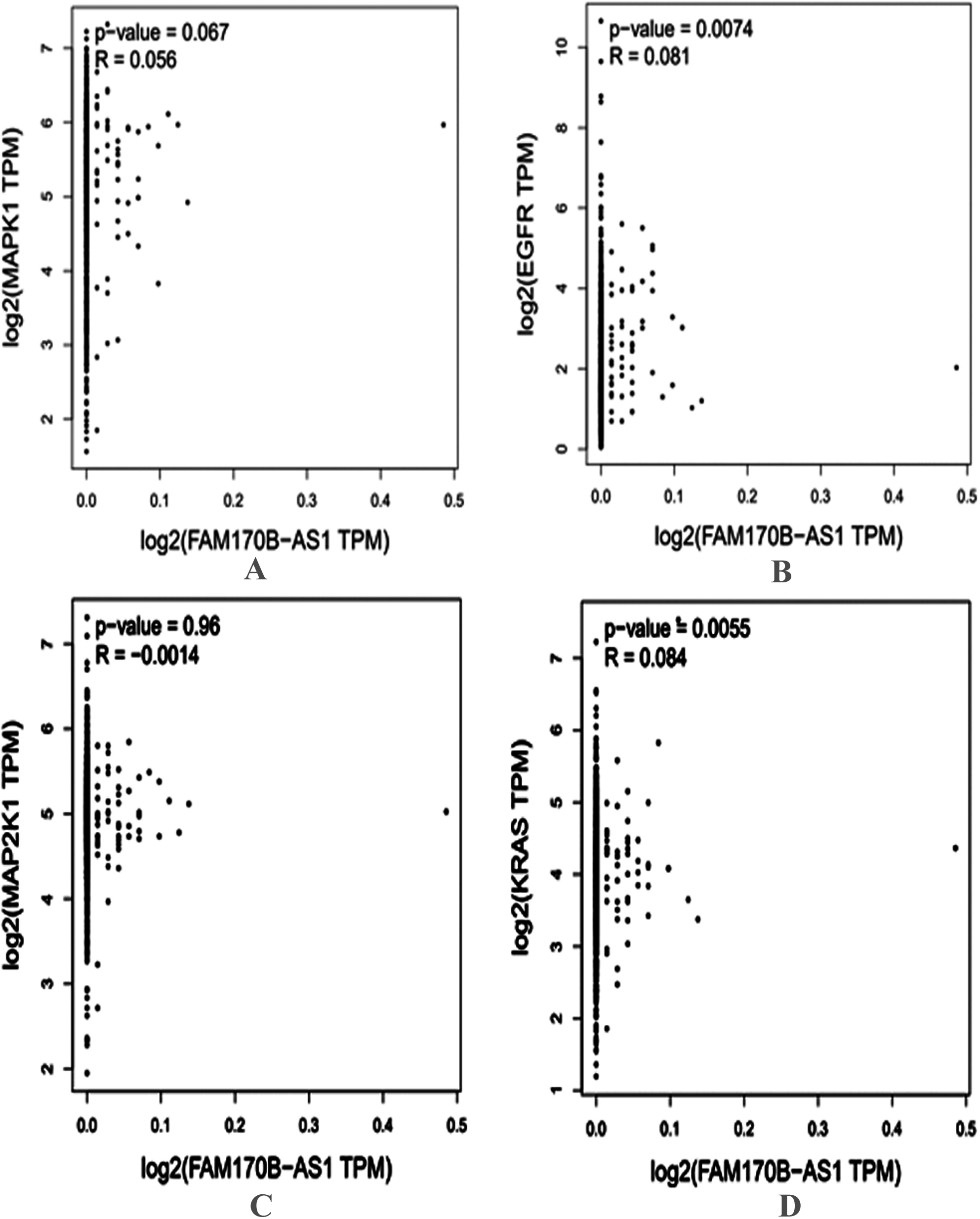
Figure 4.
The PPI network of the target genes.
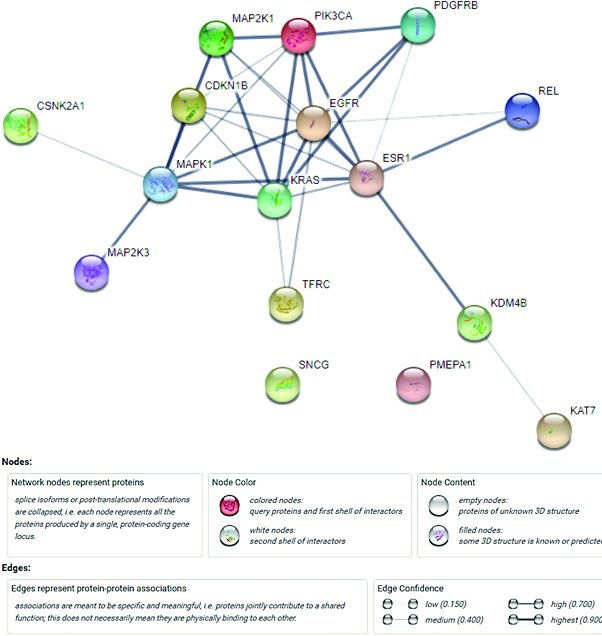
Figure 5.
Protein expression of the targeted genes in both normal breast and breast cancer tissues based on the HPA database. MAPK1 was moderately stained in glandular and myoepithelial cells of the normal breast (A-1) and was strongly stained in breast duct carcinoma (A-2). EGFR was moderately stained in glandular and myoepithelial cells of the normal breast (B-1) and was negatively stained in breast duct carcinoma (B-2). MAP2K1 was weakly stained in glandular cells of the normal breast (C-1) and was moderately stained in breast duct carcinoma (C-2). KRAS was negatively stained in glandular and myoepithelial cells of the normal breast (D-1) and was moderately stained in breast duct carcinoma (D-2).
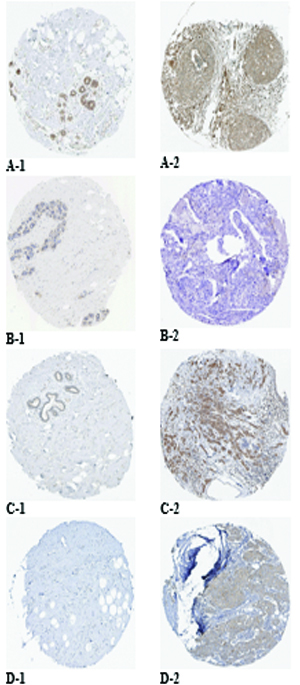
Figure 6.
Overall survival of FAM170B genetic alterations in different types of primary cancer extracted from TCGA.
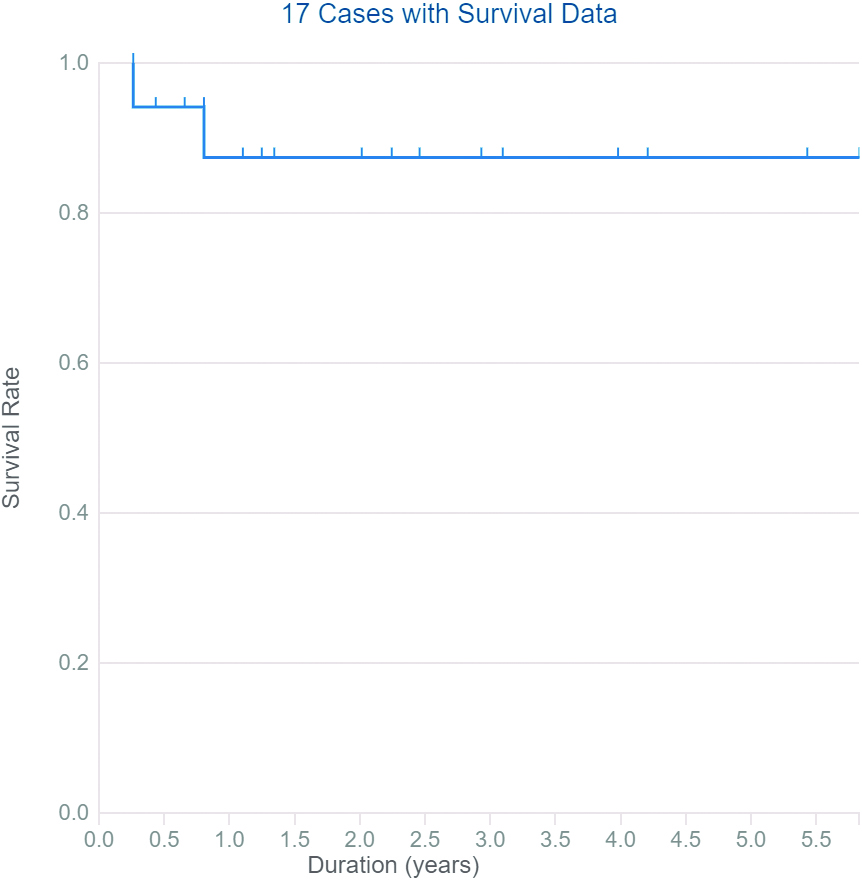
Supplementary file Table S2 illustrates the prediction of the interaction between FAM170B-AS1 and 710 miRNAs with the scores of predictions and binding besides the binding sites of miRNAs on FAM170B-AS1 lncRNA. Among these miRNAs, hsa-miR-1202 and hsa-miR-146a-5p were predicted to interact with FAM170B-AS1 with prediction scores of 0.611 and 0.539, respectively. The binding scores of hsa-miR-1202 and hsa-miR-146a-5p with FAM170B-AS1 were 0.018 and 0.007, respectively. One of the miRNAs predicted to interact with FAM170B-AS1 is hsa-miR-203a-3p with a prediction score of 0.555 and a binding score of 0.007. This miRNA is predicted by TargetScan 7.2 to interact with FAM170B gene (ENST00000311787.5) with 8mer which needs further practical validation. FAM170B is located on chromosome 10: 49, 131, 154-49, 134, 021 reverse strand. This gene codes for a protein with 283 amino acids.
In the current study, four miRNA target prediction algorithms were used to predict the potential target genes of miRNAs in breast cancer (Supplementary file Table S3). Several miRNA interactions with genes (259 interactions) were anticipated. The binding scores of miRNAs with genes were
The 16 genes were underwent for GO and pathways analyses. The GO analysis (Supplementary file Table S4) indicated that the target genes were involved in complex cellular pathways (BP), such as signal transduction, positive regulation of transcription, and cell proliferation. In addition, the target genes were located (CC) in the cytosol, nucleus, and plasma membrane. Furthermore, The GO molecular function of the target genes showed their involvement in numerous MFs, such as protein binding, ATP binding, protein kinase binding, and protein tyrosine kinase activity. The KEGG pathway analysis (Supplementary file Table S5) revealed that PD-L1 expression and PD-1 checkpoint pathway in cancer, PI3K-Akt signaling pathway, Ras signaling pathway, Rap1 signaling pathway, EGFR tyrosine kinase inhibitor resistance, ErbB signaling pathway, Estrogen signaling pathway, and MAPK signaling pathway are associated with the regulation of the 14 overlapped miRNAs.
In addition, the genes associated with PD-L1 expression and PD-1 checkpoint pathway in cancer, PI3K-Akt signaling pathway, Ras signaling pathway, Rap1 signaling pathway, EGFR tyrosine kinase inhibitor resistance, ErbB signaling pathway, Estrogen signaling pathway, and MAPK signaling pathway were selected based on the KEGG pathway analysis. Four genes (MAPK1, EGFR, KRAS, and MAP2K1) overlapped based on the Venn diagrams. Next, the current study investigated the preliminary expression level of the four genes based on GEPIA. Thus, MAPK1 and KRAS were upregulated, whereas MAP2K1 and EGFR were downregulated in BRCA tumors compared to normal BRCA tissues. Furthermore, positive correlations were found between the expression of FAM170B-AS1 and the genes expression of MAPK1 (
The String database was applied to construct the PPI network, and 66 PPI pairs with a combined score of
Moreover, based on the HPA database, strong staining in breast duct carcinoma was observed for MAPK1 (Fig. 5A-2), whereas moderate staining was observed for MAP2K1 (Fig. 5C-2) and KRAS (Fig. 5D-2) and negatively staining was observed for EGFR (Fig. 5B-2). Negative staining in normal breast tissues was observed for KRAS (Fig. 5D-1), while moderate staining was observed for MAPK1 (Fig. 5A-1) and EGFR (Fig. 5B-1), and weak staining was observed for MAP2K1 (Fig. 5C-1). Based on these results, EGFR and KRAS were selected. Using bioinformatics tool, it has been hypothesized that FAM170B-AS1 may dysregulate the expression of KRAS and EGFR genes in the PD-L1 expression and PD-1 checkpoint pathway in cancer, PI3K-Akt signaling pathway, Ras signaling pathway, Rap1 signaling pathway, EGFR tyrosine kinase inhibitor resistance, ErbB signaling pathway, Estrogen signaling pathway, and MAPK signaling pathway to participate in the different biological processes of BC.
Data collected from TCGA report 28 cases with ten types of primary cancers with somatic mutations in the FAM170B gene with different severity (six patients with the hematopoietic and reticuloendothelial system, five individuals with colon, four cases with bronchus and lung, three subjects with skin, two patients with breast, two individuals with other and ill-defined sites, two samples with the pancreas, and one sample for each of kidney, rectum, small intestine, and uterus). The types of mutations are listed in the Supplementary file Table S6. The high severity of mutations in FAM170B was observed in ductal & lobular neoplasm of breast cancer via deletion causing frameshift mutation and in ductal & lobular neoplasm of pancreatic cancer via substitution causing stop gained mutation. TCGA demonstrated that cancer patients with mutations in FAM170B show improved overall survival (Fig. 6).
4.Discussion
Since the most diagnosed cancer in women is BC, more efficient diagnostic and therapeutic methods for BC are required. In the last decades, discovering specific and sensitive molecular biomarkers with statistically significant association with BC has attracted important attention for prediction, prognosis, and diagnosis [53, 54]. Among molecular biomarkers, the lncRNAs and miRNAs circulating in the blood are of great importance because of their stability [47, 48]. lncRNAs are proven to be associated with numerous types of cancer via many pathways, including cell proliferation, invasion, and metastasis [57, 58, 59]. lncRNAs can control these pathways through lncRNAs/miRNAs/mRNA interaction networks either by acting as oncogenic or tumor suppressor lncRNAs directly or indirectly [60, 61, 62]. Therefore, bioinformatic prediction of lncRNAs followed by experimental validation becomes one of the most important research fields in cancer [63].
Discovering novel biomarkers that are more specific and sensitive to BC diagnosis is still mandatory because of the limited sensitivity and specificity of CEA and CA15.3 for BC, especially at the early stage [64, 65]. The recent study by Jintao et al. [66] reported 85.71% sensitivity, 63.49% specificity of CEA, 64.29% sensitivity, and 80.95% specificity of CA15.3 for BC. In addition, measuring the levels of CEA and CA15.3 in sera of BC patients could not significantly predict the stage, grade, and LN metastasis of BC [67]. As far as we know, the current study bioinformatically anticipated and practically validated the involvement of FAM170B-AS1 in breast tumors for the first time. The upregulation of FAM170B-AS1 gene expression in the serum of BC patients and its significant associations with BC could make FAM170B-AS1 a potential predictive marker for BC among BBD patients. In addition, the present study revealed the probable diagnostic role of FAM170B-AS1 in BC by giving higher specificity (100%) and sensitivity (97%) than that of CEA and CA15.3. Multiple linear regression analysis performed in the present study reports that serum FAM170B-AS1 expression was incorporated as an independent predictor of stage, grade, and LN metastasis of BC, while CEA and CA15.3 did not. Previous studies demonstrated that increased CEA and CA15.3 levels in the serum of BC patients at diagnosis are associated only with higher stage, tumor size, and positive axillary lymph nodes [68, 69, 70, 71, 72, 73]. All of these make serum FAM170B-AS1 a promising biomarker for BC.
MiRNAs function as regulators for multiple genes by binding to their 3’-UTRs and, therefore, up or down-regulate their expression [74]. Concerning hsa-miR-1202, various reports suggest its valuable role in predicting the prognosis of cancers [35, 75]. Chen et al. found that silenced hsa-miR-1202 in endometrial cancer could enhance the apoptotic level of tumor cells, arrest tumor cells in the G1 phase, and decline the migratory and invasive abilities of the tumor cells [76]. Wang et al. illustrated the association of the upregulated hsa-miR-1202 with lymph nodes in papillary thyroid carcinoma [75]. Quan et al. demonstrated that hsa-miR-1202 inhibits tumor cell proliferation by targeting Rab1A in glioma cells [37]. Du et al. indicated that hsa-miR-1202 is down-regulated in HCC, which was associated with metastasis, clinicopathologic features, and worse prognosis of HCC via direct binding to the CDK14 gene to exert its anti-tumor effects [38]. These reports suggested that hsa-miR-1202 may play an oncogene or tumor suppressor role in cancers by controlling cell proliferation, apoptosis, migration, and invasion.
The current study documented an upregulation in hsa-miR-1202 gene in both BBD and BC patients. This upregulation varied among BC patients with stage, grade, pathological types, molecular subtypes, and LN metastasis of BC. Also, hsa-miR-1202 showed significant associations with both BBD and BC, compared to the control group, making hsa-miR-1202 a probable risk factor for both BBD and BC. The multiple linear regression analysis performed in the present study reveals that the upregulation of hsa-miR-1202 in sera is incorporated as an independent predictor of stage, grade, and LN metastasis of BC, compared to the traditional tumor markers (CEA and CA15.3). Measuring the expression level of hsa-miR-1202 in sera gave high specificity but low sensitivity towards BC. It has been previously shown that hsa-miR-1202 is an oncomiR in BC [34]. The study of Hamam et al. [34] showed an upregulation of hsa-miR-1202 in BC patients with stages I, II, and III, compared to stage IV, with potential utilization as a tumor biomarker for early detection. Furthermore, its expression was slightly higher in the HER2 and TN compared to patients with luminal subtypes.
Regarding hsa-miR-146a role in BC, a controversial role has been illustrated. Particularly, hsa-miR-146a has been revealed to downregulate BRCA1 directly [77], where another study showed a binding between BRCA1 and the promoter of MIR146A, which activates the transcription of MIR146A leading to attenuation of EGFR expression in a hsa-miR-146a-dependent manner [49]. Interestingly, a negative association between hsa-miR-146a and EGFR, NOTCH2, and SOX2 genes was practically reported and data extracted from TCGA dataset confirmed the reported negative association of hsa-miR-146a with EGFR gene which is upregulated in TNBC and its protein product enhances tumorigenesis and immune escape via glycolysis stimulation [78] and controls TNBC aggressiveness and stemness through the management of E2/E
In the present study, hsa-miR-146a-5p was downregulated in BC patients only. Their downregulation varied among BC patients with menopause status, stage, grade, pathological types, molecular subtypes, and LN metastasis of BC. Also, hsa-miR-146a-5p showed significant association with BC, compared to the control and BBD groups, making hsa-miR-146a-5p a probable risk factor for BC. The multiple linear regression analysis performed in the present study reveals that the down regulation of hsa-miR-146a-5p in sera is not incorporated as an independent predictor of stage, grade, and LN metastasis of BC. Measuring the expression level of hsa-miR-146a-5p in sera gave high specificity and sensitivity towards BC. Iacona and Lutz explained the repression in hsa-miR-146a is mainly due to hypermethylation of promoter, methylation, and deacetylation of the histone [80]. Recently, repression of hsa-miR-146a in TNBC cells was reported, which could drive EGFR abundance and signaling, leading to tumor progression and poor disease outcome [81].
One of the functions of lncRNAs is their counteract with miRNAs, making miRNA sponges [82, 83]. Multiple linear regression analyses carried out in the present research display significant correlations of the expressions of FAM170B-AS1 with both hsa-miR-1202 and hsa-miR-146a-5p. This statistical evidence confirmed the bioinformatic prediction of FAM170B-AS1 interaction with both miRNAs using LncBase Predicted v2 database. Also, the current study revealed that FAM170B-AS1 and hsa-miR-146a-5p, other than hsa-miR-1202, may be considered excellent diagnostic markers because of their higher specificity, sensitivity, and AUC.
Cross-talks between LncRNAs/miRNAs form complex regulatory networks of post-transcriptional gene regulation. Relying on the specific lncRNA/miRNA interaction, the lncRNA/miRNA axis can have tumor suppressor or oncogenic effects [84]. The present study used the bioinformatic analysis tool to construct FAM170B-AS1/miRNA interaction network in BC for the first time, followed by identifying their target mRNAs. According to GO analysis, the target genes are involved in complex cellular pathways, such as signal transduction, transcription, and cell proliferation. The KEGG pathway analysis revealed that the PD-L1 expression and PD-1 checkpoint pathway in cancer, PI3K-Akt signaling pathway, Ras signaling pathway, Rap1 signaling pathway, EGFR tyrosine kinase inhibitor resistance, ErbB signaling pathway, Estrogen signaling pathway, and MAPK signaling pathway are potentially correlated with the regulation of the 14 predicted miRNAs. As reported, these pathways are associated with the escape of tumor cells from immune surveillance [85], resistance to endocrine therapy [86], proliferation, apoptosis, differentiation, and survival [86, 87, 88, 89].
The detailed roles for FAM170B-AS1 in BC need more explanation. The current research work combined FAM170B-AS1 analysis with TCGA and GEPIA databases to explore the potential biological functions of FAM170B-AS1 in BC. Data collected from the TCGA database display that having mutations in FAM170B showed improved OS of patients with different types of cancer, including breast cancer. The present study also bioinformatically predicted the genes from the KEGG signaling pathways and the hub genes from PPI and hypothesized that FAM170B-AS1 may affect both KRAS expression through interaction with hsa-miR-143-3p and EGFR expression by interaction with hsa-miR-7-5p to contribute to different biological processes of BC.
KRAS is involved in the tumorigenesis and progression of breast cancers via activating AKT/MEK/ERK pathways signaling [90]. In addition, overactivation of the KRAS pathway occurs in TNBCs, leading to chemoresistance [91]. Studies targeting the KRAS gene instead of the KRAS protein have attracted increasing attention in recent decades. miRNAs targeting the UTR of KRAS were reported to reduce the level of KRAS and thus inhibit the progression of TNBCs [92]. It was reported that hsa-miR-143-3p is downregulated in TNBC patients, which is associated with EMT, prognosis, and resistance to cancer therapy [93, 94]. This therapeutic resistance could be due to cytokine-induced apoptosis inhibitor-1 protein activation, which may repress hsa-miR-143-3p expression [95]. Hence, FAM170B-AS1 may affect KRAS expression through hsa-miR-143-3p according to BC subtype, which needs further investigation.
The epidermal growth factor receptor (EGFR), a member of the erbB receptor family, controls proliferation, differentiation, and development in several human tissues, including the breast [96]. The upregulation of EGFR is observed in several solid tumors causing cancer progression, poor prognosis, and resistance to chemotherapy & radiation therapy [97]. The expression of EGFR mRNA varies among different BC subtypes, either by upregulation or downregulation. Consequently, understanding the way by which EGFR is dysregulated attracted great attention [98]. hsa-miR-7 has a tumor suppressor effect in breast cancers via suppressing proliferation and enhancing apoptosis [99, 100, 101] as well as inhibiting invasion & metastasis and mediating cytotoxic T-lymphocyte-mediated lysis of breast cancer cells [102, 103]. It was reported that hsa-miR-7 suppresses EGFR mRNA in lung, breast, and glioblastoma by binding to sites in its 3’-UTR [104]. Thus, FAM170B-AS1 may affect EGFR expression through hsa-miR-7-5p according to BC subtype, which needs further investigation.
5.Conclusions and future perspectives
The current study made a spot for the first time on the involvement of FAM170B-AS1 in BC. The study experimentally validated the bioinformatic prediction of FAM170B-AS1 association with BC. The associations of FAM170B-AS1, hsa-miR-1202, and hsa-miR-146a-5p had been reported in BC, making them probable risk factors for BC. The statistically significant correlations of FAM170B-AS1 with hsa-miR-1202 and hsa-miR-146a-5p verified the bioinformatic prediction of their interactions. FAM170B-AS1 and hsa-miR-1202 might be considered predictors of BC stage, grade, and LN metastasis. In addition, FAM170B-AS1 and hsa-miR-146a-5p might be appraised as excellent diagnostic markers for BC. The current study could bioinformatically predict FAM170B-AS1/hsa-miR-143-3p/KRAS and FAM170B-AS1/hsa-miR-7-5p/EGFR network in BC. This network needs further practical study in breast cancer cells to be confirmed. The mechanism by which FAM170B-AS1 is upregulated in breast tumors needs to be elucidated by studying the FAM170B-AS1 promoter region and histone. The present work opened a new era to design a therapeutic approach against BC by targeting FAM170B-AS1 that might lead to novel therapeutic strategies. In addition, The link between FAM170B-AS1 and FAM170B in BC needs to be practically explored.
Declaration
The authors declare that there is no conflict of interest.
Funding
Not applicable.
Availability of data and materials
The datasets used and/or analysed during the current study are available from the corresponding author upon reasonable request.
Authors contribution
Conception: Hala Mostafa Ghanem, Menha Swellam, and AlShaimaa Mohamed Taha.
Interpretation or analysis of data: AlShaimaa Mohamed Taha and Ahmed Saeed Abd ELhafeez.
Preparation of the manuscript: AlShaimaa Mohamed Taha and Ahmed Saeed Abd ELhafeez.
Revision for important intellectual content: Hala Mostafa Ghanem, Menha Swellam, and AlShaimaa Mohamed Taha.
Supervision: Hala Mostafa Ghanem, Menha Swellam, and AlShaimaa Mohamed Taha.
Supplementary data
The supplementary files are available to download from http://dx.doi.org/10.3233/CBM-230396.
Acknowledgments
The instruments listed in the current study were purchased through a grant from Science The Science, Technology & Innovation Funding Authority (STDF) through Capacity Building Grant Fund (CBG) [No. 4940]. This work was partially supported by a grant from STDF through Basic and Applied Research Support Grant Project (BARG) [No.15089], Egypt.
References
[1] | F. Bray, J. Ferlay, I. Soerjomataram, R.L. Siegel, L.A. Torre and A. Jemal, Global cancer statistics 2018: GLOBOCAN estimates of incidence and mortality worldwide for 36 cancers in 185 countries, CA Cancer J Clin 68: ((2018) ), 394–424. |
[2] | H. Sung, J. Ferlay, R.L. Siegel, M. Laversanne, I. Soerjomataram, A. Jemal and F. Bray, Global cancer statistics 2020: GLOBOCAN estimates of incidence and mortality worldwide for 36 cancers in 185 countries, CA Cancer J Clin 71: ((2021) ), 209–249. |
[3] | C.E. DeSantis, J. Ma, M.M. Gaudet, L.A. Newman, K.D. Miller, A. Goding Sauer, A. Jemal and R.L. Siegel, Breast cancer statistics, 2019, CA Cancer J Clin 69: ((2019) ), 438–451. |
[4] | B. Orr and J.L. Kelley 3rd, Benign breast diseases: Evaluation and management, Clin Obstet Gynecol 59: ((2016) ), 710–726. |
[5] | F.C. Verdial, R. Etzioni, C. Duggan and B.O. Anderson, Demographic changes in breast cancer incidence, stage at diagnosis and age associated with population-based mammographic screening, J Surg Oncol 115: ((2017) ), 517–522. |
[6] | A. Stachs, J. Stubert, T. Reimer and S. Hartmann, Benign breast disease in women, Dtsch Arztebl Int 116: ((2019) ), 565–574. |
[7] | Q. Wang, M. Xu, Y. Sun, J. Chen, C. Chen, C. Qian, Y. Chen, L. Cao, Q. Xu, X. Du and W. Yang, Gene expression profiling for diagnosis of triple-negative breast cancer: A multicenter, retrospective cohort study, Front Oncol 9: ((2019) ), 354. |
[8] | P. Kumar and R. Aggarwal, An overview of triple-negative breast cancer, Arch Gynecol Obstet 293: ((2016) ), 247–269. |
[9] | N.D. Arvold, A.G. Taghian, A. Niemierko, R.F. Abi Raad, M. Sreedhara, P.L. Nguyen, J.R. Bellon, J.S. Wong, B.L. Smith and J.R. Harris, Age, breast cancer subtype approximation, and local recurrence after breast-conserving therapy, J Clin Oncol Off J Am Soc Clin Oncol 29: ((2011) ), 3885–3891. |
[10] | M.J. Engstrøm, S. Opdahl, A.I. Hagen, P.R. Romundstad, L.A. Akslen, O.A. Haugen, L.J. Vatten and A.M. Bofin, Molecular subtypes, histopathological grade and survival in a historic cohort of breast cancer patients, Breast Cancer Res Treat 140: ((2013) ), 463–473. |
[11] | S. Fayaz, G.A. Demian, M. El-Sherify, H. Eissa, M. Aziz and S. Abuzallouf, Triple negative breast cancer: 10-year survival update of the applied treatment strategy in kuwait, Gulf J Oncolog 1: ((2019) ), 53–59. |
[12] | C. Liedtke, A. Rody, O. Gluz, K. Baumann, D. Beyer, E.-B. Kohls, K. Lausen, L. Hanker, U. Holtrich, S. Becker and T. Karn, The prognostic impact of age in different molecular subtypes of breast cancer, Breast Cancer Res Treat 152: ((2015) ), 667–673. |
[13] | E. Senkus, S. Kyriakides, S. Ohno, F. Penault-Llorca, P. Poortmans, E. Rutgers, S. Zackrisson and F. Cardoso, Primary breast cancer: ESMO Clinical Practice Guidelines for diagnosis, treatment and follow-up, Ann Oncol Off J Eur Soc Med Oncol 26 Suppl 5: ((2015) ), v8-30. |
[14] | M. Hamann, S. Grill, J. Struck, A. Bergmann, O. Hartmann, M. Pölcher and M. Kiechle, Detection of early breast cancer beyond mammographic screening: A promising biomarker panel, Biomark Med 13: ((2019) ), 1107–1117. |
[15] | S. Mayor, Screening for early breast cancer reduces invasive cancer, study finds, BMJ 351: ((2015) ), h6576. |
[16] | K. Zhang, Z. Luo, Y. Zhang, L. Zhang, L. Wu, L. Liu, J. Yang, X. Song and J. Liu, Circulating lncRNA H19 in plasma as a novel biomarker for breast cancer, Cancer Biomark 17: ((2016) ), 187–194. |
[17] | M.J. Delás and G.J. Hannon, lncRNAs in development and disease: From functions to mechanisms, Open Biol 7: ((2017) ). |
[18] | A.M. Schmitt and H.Y. Chang, Long noncoding RNAs in cancer pathways, Cancer Cell 29: ((2016) ), 452–463. |
[19] | Y. Du, Y. Chen, T. Wu, X. Fan, W. Lin and Z. Jiang, miR-2682-3p antagonizes its host lncRNA-MIR137HG by interacting with the same target FUS to regulate the progression of gastric cancer, BMC Cancer 22: ((2022) ), 689. |
[20] | J. Matuszyk, MALAT1-miRNAs network regulate thymidylate synthase and affect 5FU-based chemotherapy, Mol Med 28: ((2022) ), 89. |
[21] | J. Ramírez-Moya, L. Wert-Lamas, A. Acuña-Ruíz, A. Fletcher, C. Wert-Carvajal, C.J. McCabe, P. Santisteban and G. Riesco-Eizaguirre, Identification of an interactome network between lncRNAs and miRNAs in thyroid cancer reveals SPTY2D1-AS1 as a new tumor suppressor, Sci Rep 12: ((2022) ), 7706. |
[22] | D.P. Bartel, MicroRNAs: Target recognition and regulatory functions, Cell 136: ((2009) ), 215–233. |
[23] | J.-H. Yoon, K. Abdelmohsen and M. Gorospe, Functional interactions among microRNAs and long noncoding RNAs, Semin Cell Dev Biol 34: ((2014) ), 9–14. |
[24] | M.-C.D. Wasson, J.M. Brown, J. Venkatesh, W. Fernando and P. Marcato, Datasets exploring putative lncRNA-miRNA-mRNA axes in breast cancer cell lines, Data Br 37: ((2021) ), 107241. |
[25] | Y. Shen, X. Peng and C. Shen, Identification and validation of immune-related lncRNA prognostic signature for breast cancer, Genomics 112: ((2020) ), 2640–2646. |
[26] | Y. Yamada, Y. Yasukochi, K. Kato, M. Oguri, H. Horibe, T. Fujimaki, I. Takeuchi and J. Sakuma, Identification of 26 novel loci that confer susceptibility to early-onset coronary artery disease in a Japanese population, Biomed Reports 9: ((2018) ), 383–404. |
[27] | J. Ke, X. Hu, C. Wang and Y. Zhang, Identification of the hub susceptibility genes and related common transcription factors in the skeletal muscle of type 2 diabetes mellitus, BMC Endocr Disord 22: ((2022) ), 276. |
[28] | Y. Zhou, X. Chen, Y. Zheng, R. Shen, S. Sun, F. Yang, J. Min, L. Bao, Y. Zhang, X. Zhao, J. Wang and Q. Wang, Long non-coding RNAs and mRNAs expression profiles of monocyte-derived dendritic cells from PBMCs in AR, Front Cell Dev Biol 9: ((2021) ), 636477. |
[29] | W. Hu, P. Li, N. Zeng and S. Tan, Exploring the hub mechanisms of ischemic stroke based on protein-protein interaction networks related to ischemic stroke and inflammatory bowel disease, Sci Rep 13: ((2023) ), 1741. |
[30] | T. Falcon, M. Freitas, A.C. Mello, L. Coutinho, M.R. Alvares-da-Silva and U. Matte, Analysis of the cancer genome atlas data reveals novel putative ncRNAs targets in hepatocellular carcinoma, Biomed Res Int 2018: ((2018) ), 1–9. |
[31] | Y. Zhang, T. Jin, H. Shen, J. Yan, M. Guan and X. Jin, Identification of Long Non-Coding RNA Expression Profiles and Co-Expression Genes in Thyroid Carcinoma Based on The Cancer Genome Atlas (TCGA) Database, Med Sci Monit 25: ((2019) ), 9752–9769. |
[32] | S. Ramalingam, Cancer Genes, Bentham Science Publishers, (2023) . |
[33] | X. Liu, D. Zhang, J. Hu, S. Xu, C. Xu and Y. Shen, Allograft inflammatory factor 1 is a potential diagnostic, immunological, and prognostic biomarker in pan-cancer, Aging (Albany NY) 15: ((2023) ), 2582–2609. |
[34] | R. Hamam, A.M. Ali, K.A. Alsaleh, M. Kassem, M. Alfayez, A. Aldahmash and N.M. Alajez, microRNA expression profiling on individual breast cancer patients identifies novel panel of circulating microRNA for early detection, Sci Rep 6: ((2016) ). |
[35] | H. Chen, Y. Fan, W. Xu, J. Chen, Y. Meng, D. Fang and J. Wang, Exploration of miR-1202 and miR-196a in human endometrial cancer based on high throughout gene screening analysis, Oncol Rep 37: ((2017) ), 3493–3501. |
[36] | A. Yan, X. Song, B. Liu and K. Zhu, IGF2BP3 Worsens Lung Cancer through Modifying Long Non-coding RNA CERS6-AS1/microRNA-1202 Axis, Curr Med Chem 30: ((2023) ), 878–891. |
[37] | Y. Quan, Q. Song, J. Wang, L. Zhao, J. Lv and S. Gong, MiR-1202 functions as a tumor suppressor in glioma cells by targeting Rab1A, Tumour Biol J Int Soc Oncodevelopmental Biol Med 39: ((2017) ), 1010428317697565. |
[38] | B. Du, P. Zhang, Z. Tan and J. Xu, MiR-1202 suppresses hepatocellular carcinoma cells migration and invasion by targeting cyclin dependent kinase 14, Biomed Pharmacother 96: ((2017) ), 1246–1252. |
[39] | C. Wu, Y. Cao, Z. He, J. He, C. Hu, H. Duan and J. Jiang, Serum levels of miR-19b and miR-146a as prognostic biomarkers for non-small cell lung cancer, Tohoku J Exp Med 232: ((2014) ), 85–95. |
[40] | R.-J. Wang, Y.-H. Zheng, P. Wang and J.-Z. Zhang, Serum miR-125a-5p, miR-145 and miR-146a as diagnostic biomarkers in non-small cell lung cancer, Int J Clin Exp Pathol 8: ((2015) ), 765–771. |
[41] | X. Liu, B. Liu, R. Li, F. Wang, N. Wang, M. Zhang, Y. Bai, J. Wu, L. Liu, D. Han, Z. Li, B. Feng, G. Zhou, S. Wang, L. Zeng, J. Miao, Y. Yao, B. Liang, L. Huang, Q. Wang and Y. Wu, miR-146a-5p Plays an Oncogenic Role in NSCLC via Suppression of TRAF6, Front Cell Dev Biol 8: ((2020) ), 847. |
[42] | H. Liang, W. Huang, Y. Wang, L. Ding and L. Zeng, Overexpression of MiR-146a-5p Upregulates lncRNA HOTAIR in Triple-Negative Breast Cancer Cells and Predicts Poor Prognosis, Technol Cancer Res Treat 18: ((2019) ), 1533033819882949. |
[43] | H. Tashkandi, N. Shah, Y. Patel and H. Chen, Identification of new miRNA biomarkers associated with HER2-positive breast cancers, Oncoscience 2: ((2015) ), 924–929. |
[44] | R. Elango, K.A. Alsaleh, R. Vishnubalaji, M. Manikandan, A.M. Ali, N. Abd El-Aziz, A. Altheyab, A. Al-Rikabi, M. Alfayez, A. Aldahmash and N.M. Alajez, MicroRNA Expression Profiling on Paired Primary and Lymph Node Metastatic Breast Cancer Revealed Distinct microRNA Profile Associated With LNM, Front Oncol 10: ((2020) ), 756. |
[45] | P. Cabello, S. Torres-Ruiz, A. Adam-Artigues, J. Forés-Martos, M.T. Martínez, C. Hernando, S. Zazo, J. Madoz-Gúrpide, A. Rovira, O. Burgués, F. Rojo, J. Albanell, A. Lluch, B. Bermejo, J.M. Cejalvo and P. Eroles, miR-146a-5p Promotes Angiogenesis and Confers Trastuzumab Resistance in HER2+ Breast Cancer, Cancers (Basel) 15: ((2023) ). |
[46] | D. Escuin, L. López-Vilaró, J. Mora, O. Bell, A. Moral, I. Pérez, C. Arqueros, B. García-Valdecasas, T. Ramón, Y. Cajal, E. Lerma and A. Barnadas, Circulating microRNAs in early breast cancer patients and its association with lymph node metastases, Front Oncol 11: ((2021) ), 627811. |
[47] | J.P. Long, L.F. Dong, F.F. Chen and Y.F. Fan, Mir-146a-5p targets interleukin-1 receptor-associated kinase 1 to inhibit the growth, migration, and invasion of breast cancer cells, Oncol Lett 17: ((2019) ), 1573–1580. |
[48] | D.H. Park, H.S. Jeon, S.Y. Lee, Y.Y. Choi, H.W. Lee, S. Yoon, J.C. Lee, Y.S. Yoon, D.S. Kim, M.J. Na, S.J. Kwon, D.S. Kim, J. Kang, J.Y. Park and J.W. Son, MicroRNA-146a inhibits epithelial mesenchymal transition in non-small cell lung cancer by targeting insulin receptor substrate 2, Int J Oncol 47: ((2015) ), 1545–1553. |
[49] | E. Kumaraswamy, K.L. Wendt, L.A. Augustine, S.R. Stecklein, E.C. Sibala, D. Li, S. Gunewardena and R.A. Jensen, BRCA1 regulation of epidermal growth factor receptor (EGFR) expression in human breast cancer cells involves microRNA-146a and is critical for its tumor suppressor function, Oncogene 34: ((2015) ), 4333–4346. |
[50] | S. Khawaled, G. Nigita, R. Distefano, S. Oster, S.-S. Suh, Y. Smith, A. Khalaileh, Y. Peng, C.M. Croce, T. Geiger, V.L. Seewaldt and R.I. Aqeilan, Pleiotropic tumor suppressor functions of WWOX antagonize metastasis, Signal Transduct Target Ther 5: ((2020) ), 43. |
[51] | P. Jiang, B. Liang, Z. Zhang, B. Fan, L. Zeng, Z. Zhou, Z. Mao, Q. Lin, W. Yao and Q. Shen, MicroRNA-146a-5p induces cell cycle arrest and enhances apoptosis in gastric cancer via targeting CDC14A, Front Cell Dev Biol 11: ((2023) ), 1181628. |
[52] | K.J. Livak and T.D. Schmittgen, Analysis of relative gene expression data using real-time quantitative PCR and the 2(-Delta Delta C(T)) Method, Methods 25: ((2001) ), 402–408. |
[53] | J.D. Cohen, L. Li, Y. Wang, C. Thoburn, B. Afsari, L. Danilova, C. Douville, A.A. Javed, F. Wong, A. Mattox, R.H. Hruban, C.L. Wolfgang, M.G. Goggins, M. Dal Molin, T.-L. Wang, R. Roden, A.P. Klein, J. Ptak, L. Dobbyn, J. Schaefer, N. Silliman, M. Popoli, J.T. Vogelstein, J.D. Browne, R.E. Schoen, R.E. Brand, J. Tie, P. Gibbs, H.-L. Wong, A.S. Mansfield, J. Jen, S.M. Hanash, M. Falconi, P.J. Allen, S. Zhou, C. Bettegowda, L.A.J. Diaz, C. Tomasetti, K.W. Kinzler, B. Vogelstein, A.M. Lennon and N. Papadopoulos, Detection and localization of surgically resectable cancers with a multi-analyte blood test, Science 359: ((2018) ), 926–930. |
[54] | J. Shen, N.W. Todd, H. Zhang, L. Yu, X. Lingxiao, Y. Mei, M. Guarnera, J. Liao, A. Chou, C.L. Lu, Z. Jiang, H. Fang, R.L. Katz and F. Jiang, Plasma microRNAs as potential biomarkers for non-small-cell lung cancer, Lab Invest 91: ((2011) ), 579–587. |
[55] | M.G. Schrauder, R. Strick, R. Schulz-Wendtland, P.L. Strissel, L. Kahmann, C.R. Loehberg, M.P. Lux, S.M. Jud, A. Hartmann, A. Hein, C.M. Bayer, M.R. Bani, S. Richter, B.R. Adamietz, E. Wenkel, C. Rauh, M.W. Beckmann and P.A. Fasching, Circulating micro-RNAs as potential blood-based markers for early stage breast cancer detection, PLoS One 7: ((2012) ), e29770. |
[56] | D.B. Martin, P.S. Nelson, B.S. Knudsen, R.K. Parkin, J. Noteboom, E.M. Kroh, K.C. O’Briant, C.W. Drescher, R.L. Vessella, R. Gentleman, B.R. Fritz, N. Urban, A. Peterson, M. Tewari, E.L. Pogosova-Agadjanyan, S.K. Wyman, A. Allen, D.L. Stirewalt, P.S. Mitchell and D.W. Lin, Circulating microRNAs as stable blood-based markers for cancer detection, Proc Natl Acad Sci 105: ((2008) ), 10513–10518. |
[57] | R. Gao, R. Zhang, C. Zhang, L. Zhao and Y. Zhang, Long noncoding RNA CCAT1 promotes cell proliferation and metastasis in human medulloblastoma via MAPK pathway, Tumori 104: ((2018) ), 43–50. |
[58] | L. Jin, H. Fu, J. Quan, X. Pan, T. He, J. Hu, Y. Li, H. Li, Y. Yang, J. Ye, F. Zhang, L. Ni, S. Yang and Y. Lai, Overexpression of long non-coding RNA differentiation antagonizing non-protein coding RNA inhibits the proliferation, migration and invasion and promotes apoptosis of renal cell carcinoma, Mol Med Rep 16: ((2017) ), 4463–4468. |
[59] | Z. Zhang, T. Liu, K. Wang, X. Qu, Z. Pang, S. Liu, Q. Liu and J. Du, Down-regulation of long non-coding RNA MEG3 indicates an unfavorable prognosis in non-small cell lung cancer: Evidence from the GEO database, Gene 630: ((2017) ), 49–58. |
[60] | G. Arun, S.D. Diermeier and D.L. Spector, Therapeutic Targeting of Long Non-Coding RNAs in Cancer, Trends Mol Med 24: ((2018) ), 257–277. |
[61] | D. Barsyte-Lovejoy, S.K. Lau, P.C. Boutros, F. Khosravi, I. Jurisica, I.L. Andrulis, M.S. Tsao and L.Z. Penn, The c-Myc oncogene directly induces the H19 noncoding RNA by allele-specific binding to potentiate tumorigenesis, Cancer Res 66: ((2006) ), 5330–5337. |
[62] | M. Huarte, M. Guttman, D. Feldser, M. Garber, M.J. Koziol, D. Kenzelmann-Broz, A.M. Khalil, O. Zuk, I. Amit, M. Rabani, L.D. Attardi, A. Regev, E.S. Lander, T. Jacks and J.L. Rinn, A large intergenic noncoding RNA induced by p53 mediates global gene repression in the p53 response, Cell 142: ((2010) ), 409–419. |
[63] | A. Rahimi, R. Sedighi, M. Emadi-Baygi, M.-A. Honardoost, S.-J. Mowla, H. Khanahmad and P. Nikpour, Bioinformatics prediction and experimental validation of a novel microRNA: hsa-miR-B43 within human CDH4 gene with a potential metastasis-related function in breast cancer, J Cell Biochem 121: ((2020) ), 1307–1316. |
[64] | M.J. Duffy, Serum tumor markers in breast cancer: Are they of clinical value, Clin Chem 52: ((2006) ), 345–351. |
[65] | A. Perrier, P.-Y. Boelle, Y. Chrétien, J. Gligorov, J.-P. Lotz, D. Brault, E. Comperat, G. Lefèvre and M. Boissan, An updated evaluation of serum sHER2, CA15.3, and CEA levels as biomarkers for the response of patients with metastatic breast cancer to trastuzumab-based therapies, PLoS One 15: ((2020) ), e0227356. |
[66] | J. Mi, H. Zhang, W. Cao and C. Yuan, FTO, PIK3CB serve as potential markers to complement CEA and CA15-3 for the diagnosis of breast cancer, Medicine (Baltimore) 102: ((2023) ), e35361. |
[67] | K.N. Seale and K.H.R. Tkaczuk, Circulating biomarkers in breast cancer, Clin Breast Cancer 22: ((2022) ), e319–e331. |
[68] | P. Gaglia, B. Caldarola, R. Bussone, F. Potente, D. Lauro, A. Jayme and L. Caldarola, Prognostic value of CEA and ferritin assay in breast cancer: A multivariate analysis, Eur J Cancer Clin Oncol 24: ((1988) ), 1151–1155. |
[69] | R. Molina, J. Jo, X. Filella, G. Zanon, J. Pahisa, M. Mu noz, B. Farrus, M.L. Latre, C. Escriche, J. Estape and A.M. Ballesta, c-erbB-2 oncoprotein, CEA, and CA 15.3 in patients with breast cancer: Prognostic value, Breast Cancer Res Treat 51: ((1998) ), 109–119. |
[70] | S. Wu, Z. He, J. Zhou, J. Sun, F. Li, Q. Lin, L. Guo and H. Lin, Serum levels of CEA and CA15-3 in different molecular subtypes and prognostic value in Chinese breast cancer, Breast 23: ((2014) ), 88–93. |
[71] | Y. Shao, X. Sun, Y. He, C. Liu and H. Liu, Elevated Levels of Serum Tumor Markers CEA and CA15-3 Are Prognostic Parameters for Different Molecular Subtypes of Breast Cancer, PLoS One 10: ((2015) ), e0133830. |
[72] | Y. Fu and H. Li, Assessing Clinical Significance of Serum CA15-3 and Carcinoembryonic Antigen (CEA) Levels in Breast Cancer Patients: A Meta-Analysis, Med Sci Monit Int Med J Exp Clin Res 22: ((2016) ), 3154–3162. |
[73] | D. Di Gioia, M. Dresse, D. Mayr, D. Nagel, V. Heinemann and P. Stieber, Serum HER2 in combination with CA 15-3 as a parameter for prognosis in patients with early breast cancer, Clin Chim Acta 440: ((2015) ), 16–22. |
[74] | M. Long, M. Zhan, S. Xu, R. Yang, W. Chen, S. Zhang, Y. Shi, Q. He, M. Mohan, Q. Liu and J. Wang, miR-92b-3p acts as a tumor suppressor by targeting Gabra3 in pancreatic cancer, Mol Cancer 16: ((2017) ), 167. |
[75] | Z. Wang, H. Zhang, P. Zhang, J. Li, Z. Shan and W. Teng, Upregulation of miR-2861 and miR-451 expression in papillary thyroid carcinoma with lymph node metastasis, Med Oncol 30: ((2013) ). |
[76] | M. Allaire and J.-C. Nault, Advances in management of hepatocellular carcinoma, Curr Opin Oncol 29: ((2017) ), 288–295. |
[77] | A.I. Garcia, M. Buisson, P. Bertrand, R. Rimokh, E. Rouleau, B.S. Lopez, R. Lidereau, I. Mikaélian and S. Mazoyer, Down-regulation of BRCA1 expression by miR-146a and miR-146b-5p in triple negative sporadic breast cancers, EMBO Mol Med 3: ((2011) ), 279–290. |
[78] | S.-O. Lim, C.-W. Li, W. Xia, H.-H. Lee, S.-S. Chang, J. Shen, J.L. Hsu, D. Raftery, D. Djukovic, H. Gu, W.-C. Chang, H.-L. Wang, M.-L. Chen, L. Huo, C.-H. Chen, Y. Wu, A. Sahin, S.M. Hanash, G.N. Hortobagyi and M.-C. Hung, EGFR signaling enhances aerobic glycolysis in triple-negative breast cancer cells to promote tumor growth and immune escape, Cancer Res 76: ((2016) ), 1284–1296. |
[79] | H. Zhang, Y. Zhang, W. Yan, W. Wang, X. Zhao, X. Ma, X. Gao and S. Zhang, Association between three functional microRNA polymorphisms (miR-499 rs3746444, miR-196a rs11614913 and miR-146a rs2910164) and breast cancer risk: a meta-analysis, Oncotarget 8: ((2017) ), 393–407. |
[80] | J.R. Iacona and C.S. Lutz, miR-146a-5p: Expression, regulation, and functions in cancer, Wiley Interdiscip Rev RNA 10: ((2019) ), e1533. |
[81] | K. Panoutsopoulou, Y. Liu, M. Avgeris, T. Dreyer, J. Dorn, V. Magdolen and A. Scorilas, Repression of miR-146a in predicting poor treatment outcome in triple-negative breast cancer, Clin Biochem 114: ((2023) ), 43–51. |
[82] | H. Ling, M. Fabbri and G.A. Calin, MicroRNAs and other non-coding RNAs as targets for anticancer drug development, Nat Rev Drug Discov 12: ((2013) ), 847–865. |
[83] | K. V Morris and J.S. Mattick, The rise of regulatory RNA, Nat Rev Genet 15: ((2014) ), 423–437. |
[84] | J. Venkatesh, M.-C.D. Wasson, J.M. Brown, W. Fernando and P. Marcato, LncRNA-miRNA axes in breast cancer: Novel points of interaction for strategic attack, Cancer Lett 509: ((2021) ), 81–88. |
[85] | R.K. Beckers, C.I. Selinger, R. Vilain, J. Madore, J.S. Wilmott, K. Harvey, A. Holliday, C.L. Cooper, E. Robbins, D. Gillett, C.W. Kennedy, L. Gluch, H. Carmalt, C. Mak, S. Warrier, H.E. Gee, C. Chan, A. McLean, E. Walker, C.M. McNeil, J.M. Beith, A. Swarbrick, R.A. Scolyer and S.A. O’Toole, Programmed death ligand 1 expression in triple-negative breast cancer is associated with tumour-infiltrating lymphocytes and improved outcome, Histopathology 69: ((2016) ), 25–34. |
[86] | E. Paplomata and R. O’Regan, New and emerging treatments for estrogen receptor-positive breast cancer: Focus on everolimus, Ther Clin Risk Manag 9: ((2013) ), 27–36. |
[87] | N. Normanno, C. Bianco, A. De Luca, M.R. Maiello and D.S. Salomon, Target-based agents against ErbB receptors and their ligands: A novel approach to cancer treatment, Endocr Relat Cancer 10: ((2003) ), 1–21. |
[88] | L. Lu, J. Wang, Y. Wu, P. Wan and G. Yang, Rap1A promotes ovarian cancer metastasis via activation of ERK/p38 and notch signaling, Cancer Med 5: ((2016) ), 3544–3554. |
[89] | A.S. Dhillon, S. Hagan, O. Rath and W. Kolch, MAP kinase signalling pathways in cancer, Oncogene 26: ((2007) ), 3279–3290. |
[90] | M.S. Kumar and C. Swanton, KRAS 3’-UTR variants and stratification of breast-cancer risk, Lancet Oncol 12: ((2011) ), 318–319. |
[91] | S. Loibl, D. Treue, J. Budczies, K. Weber, A. Stenzinger, W.D. Schmitt, W. Weichert, P. Jank, J. Furlanetto, F. Klauschen, T. Karn, N. Pfarr, G. von Minckwitz, M. Möbs, C. Jackisch, C. Sers, A. Schneeweiss, P.A. Fasching, C. Schem, M. Hummel, M. van Mackelenbergh, V. Nekljudova, M. Untch and C. Denkert, Mutational diversity and therapy response in breast cancer: A Sequencing Analysis in the Neoadjuvant GeparSepto Trial, Clin Cancer Res an Off J Am Assoc Cancer Res 25: ((2019) ), 3986–3995. |
[92] | H.A. Mokhlis, R. Bayraktar, N.N. Kabil, A. Caner, N. Kahraman, C. Rodriguez-Aguayo, E.P. Zambalde, J. Sheng, K. Karagoz, P. Kanlikilicer, A.A.H. Abdel Aziz, T.M. Abdelghany, A.A. Ashour, S. Wong, M.L. Gatza, G.A. Calin, G. Lopez-Berestein and B. Ozpolat, The Modulatory Role of MicroRNA-873 in the Progression of KRAS-Driven Cancers, Mol Ther Nucleic Acids 14: ((2019) ), 301–317. |
[93] | M. Koleckova, J. Ehrmann, J. Bouchal, M. Janikova, A. Brisudova, J. Srovnal, K. Staffova, M. Svoboda, O. Slaby, L. Radova, K. Vomackova, B. Melichar, L. Veverkova and Z. Kolar, Epithelial to mesenchymal transition and microRNA expression are associated with spindle and apocrine cell morphology in triple-negative breast cancer, Sci Rep 11: ((2021) ), 5145. |
[94] | J. Feng, L. Wang, K. Zhang, S. Ni, B. Li, J. Liu and D. Wang, Identification and panoramic analysis of drug response-related genes in triple negative breast cancer using as an example NVP-BEZ235, Sci Rep 13: ((2023) ), 5984. |
[95] | Y.W. Deng, W.J. Hao, Y.W. Li, Y.X. Li, B.C. Zhao and D. Lu, Hsa-miRNA-143-3p reverses multidrug resistance of triple-negative breast cancer by inhibiting the expression of its target protein cytokine-induced apoptosis inhibitor 1 in vivo, J Breast Cancer 21: ((2018) ), 251–258. |
[96] | S. Yano, K. Kondo, M. Yamaguchi, G. Richmond, M. Hutchison, A. Wakeling, S. Averbuch and P. Wadsworth, Distribution and function of EGFR in human tissue and the effect of EGFR tyrosine kinase inhibition, Anticancer Res 23: ((2003) ), 3639–3650. |
[97] | C.L. Arteaga, The epidermal growth factor receptor: From mutant oncogene in nonhuman cancers to therapeutic target in human neoplasia, J Clin Oncol Off J Am Soc Clin Oncol 19: ((2001) ), 32S–40S. |
[98] | R. Bianco, T. Troiani, G. Tortora and F. Ciardiello, Intrinsic and acquired resistance to EGFR inhibitors in human cancer therapy, Endocr Relat Cancer 12 Suppl 1: ((2005) ), S159-71. |
[99] | Y. Shi, X. Luo, P. Li, J. Tan, X. Wang, T. Xiang and G. Ren, miR-7-5p suppresses cell proliferation and induces apoptosis of breast cancer cells mainly by targeting REGγ, Cancer Lett 358: ((2015) ), 27–36. |
[100] | J.L. Horsham, F.C. Kalinowski, M.R. Epis, C. Ganda, R.A.M. Brown and P.J. Leedman, Clinical Potential of microRNA-7 in Cancer, J Clin Med 4: ((2015) ), 1668–1687. |
[101] | T.B. Hansen, J. Kjems and C.K. Damgaard, Circular RNA and miR-7 in cancer, Cancer Res 73: ((2013) ), 5609–5612. |
[102] | E. van Schooneveld, H. Wildiers, I. Vergote, P.B. Vermeulen, L.Y. Dirix and S.J. Van Laere, Dysregulation of microRNAs in breast cancer and their potential role as prognostic and predictive biomarkers in patient management, Breast Cancer Res 17: ((2015) ), 21. |
[103] | H. Zhang, K. Cai, J. Wang, X. Wang, K. Cheng, F. Shi, L. Jiang, Y. Zhang and J. Dou, MiR-7, inhibited indirectly by lincRNA HOTAIR, directly inhibits SETDB1 and reverses the EMT of breast cancer stem cells by downregulating the STAT3 pathway, Stem Cells 32: ((2014) ), 2858–2868. |
[104] | R.J. Webster, K.M. Giles, K.J. Price, P.M. Zhang, J.S. Mattick and P.J. Leedman, Regulation of epidermal growth factor receptor signaling in human cancer cells by microRNA-7, J Biol Chem 284: ((2009) ), 5731–5741. |