Impact of the peripheral blood inflammatory indices and modified nomogram-revised risk index on survival of Extranodal Nasal-Type Natural Killer/T-Cell lymphoma
Abstract
BACKGROUND:
At present, peripheral blood markers are easily accessible information and clinically valuable prognostic indicators in extranodal nasal-type natural killer/T-cell lymphoma (ENKTCL). Nevertheless, the role of its comprehensive score in ENKTCL remains to be determined.
OBJECTIVE:
Therefore, this study aimed to investigate the prognostic effect of the peripheral inflammation score on ENKTCL.
METHODS:
The retrospective study included 183 patients with ENKTCL. Univariate Cox regression analyses and least absolute shrinkage and selection operator (LASSO) Cox regression were used to construct the inflammation-related prognostic index named Risk. Univariate and multivariate Cox regression analyses and regression adjustment with propensity score matching (PSM) were used to evaluate the prognostic ability of risk. The performance of the modified nomogram-revised risk index (NRI) by integrating risk was evaluated with the area under the time-dependent receiver operating characteristic (ROC) curve (AUC), decision curve analysis (DCA), and integrated Brier score (IBS).
RESULTS:
The risk cut-off value, constructed by the lymphocyte count, platelet count, albumin level, LMR, and PNI, was
CONCLUSIONS:
Risk is an easily accessible and inexpensive indicator that may be used as a prognostic marker and could improve NRI predictive power in patients with ENKTCL.
1.Introduction
Extranodal Nasal-Type Natural Killer/T-Cell Lymphoma (ENKTCL) is an aggressive extranodal lymphoma of natural killer (NK)-cell or T-cell lineage lymphoma characterized by vascular damage and destruction, prominent necrosis, a cytotoxic phenotype, and is associated with Epstein-Barr virus [1]. ENKTCL is more prevalent among Asian, Mexican, Central American, and South American indigenous populations. ENKTCL is more common in males than females, with a median age of 44–54 years at diagnosis [2].
The most common prognostic scores of ENKTCL include the Korean Prognostic Index (KPI), the International Prognostic Index (IPI), and the prognostic index of natural killer lymphoma (PINK) [3, 4, 5, 6]. However, these prognostic scores still have some limitations in clinical application. They cannot always accurately predict the prognosis, cannot help doctors customize the initial therapy, and cannot identify risk groups that are beneficial to prognosis in early patients [7]. Yang et al. [8] developed and validated an easily used nomogram-revised risk index (NRI) that stratified patients based on age, Ann Arbor stage, Eastern Cooperative Oncology Group (ECOG) scores, lactate dehydrogenase (LDH) levels, and primary tumour invasion (PTI). The NRI can evaluate the outcome more accurately than the KPI, IPI, and PINK. The risk-adaptive therapy proposed based on its conclusions can effectively identify patients who will benefit from radiotherapy followed by chemotherapy [7, 8, 9, 10].
However, the risk factors for NRI did not involve inflammatory biomarkers. Previous studies have shown that inflammation is closely related to the development and prognosis of malignant tumours and has been well validated in central nervous system lymphoma, small cell lung carcinoma, pancreatic cancer, and others [9, 10, 11]. Albumin, monocytes, lymphocytes, platelets, neutrophil-to-lymphocyte ratio (NLR), derived neutrophil-to-lymphocyte ratio (dNLR), platelet-to-lymphocyte ratio (PLR), lymphocyte-to-monocyte ratio (LMR), and prognostic nutritional index (PNI) have been reported to be associated with the prognosis of ENKTCL [12, 13, 14, 15]. Although these inflammatory biomarkers are essential, they provide limited predictive performance when evaluated separately.
This study aimed to evaluate the association between inflammatory biomarkers and ENKTCL patient prognosis and construct a peripheral blood inflammatory index named Risk. Furthermore, we assessed an additional improvement of combined Risk to NRI prediction performance.
2.Patients and methods
2.1Patients
A total of 183 patients with a pathological diagnosis of ENKTCL admitted to Shanxi Provincial Cancer Hospital from January 2002 to December 2018 were retrospectively reviewed. The inclusion criteria were the same as those described in our previous study [11]. This study was approved by the Ethics Committee of Shanxi Province Cancer Hospital, Shanxi Hospital Affiliated to Cancer Hospital, Chinese Academy of Medical Sciences, and Cancer Hospital Affiliated to Shanxi Medical University. The Review Committee exempted informed consent requirements.
2.2Laboratory measurement
The laboratory indicators and their derived indicators included albumin, monocyte, lymphocyte, platelet, NLR, dNLR, PLR, LMR, and PNI within one week before treatment. The compound prognostic scores were calculated by Eq. (2.2).
(1)
2.3Statistics
Overall survival (OS) was defined as the time from the date of diagnosis to the date of any cause of death or final follow-up. Progression-free survival (PFS) was measured from the date of diagnosis to the date of first recurrence, progression, death, or last follow-up. Maximally selected rank statistics determine the optimal cut-off value of continuous variables. Variables with a
3.Results
3.1Patient characteristics
The clinical characteristics of all patients are summarized in Table 1. The median age of the patients was 46 years (range 9–81 years). The number of patients receiving radiotherapy and L-asparaginase (L-ASP) was 61 and 83, respectively. The median OS was 137 months, and 86 patients (47.0%) died during the follow-up period. The 3-year and 5-year OS rates were 56.7% and 51.7%, respectively. The 3-year and 5-year PFS were 50.6% and 43.3%, respectively.
Table 1
Distribution of clinical characteristics of patients with ENKTCL
Characteristics | Number of patients (%) |
---|---|
Age | |
| 147 (80.33%) |
| 36 (19.67%) |
Gender | |
Male | 144 (78.69%) |
Female | 39 (21.31%) |
ECOG | |
0–1 | 147 (80.33%) |
| 36 (19.67%) |
Ann arbor stage | |
I–II | 141 (77.05%) |
III–IV | 42 (22.95%) |
B symptoms | |
Yes | 66 (36.07%) |
No | 117 (63.93%) |
No. of extranodal sites | |
| 158 (86.34%) |
| 25 (13.66%) |
LN | |
Yes | 66 (36.07%) |
No | 117 (63.93%) |
Primary site | |
UADT | 169 (92.35%) |
Non-UADT | 14 (7.65%) |
Treatment | |
RT alone | 14 (7.65%) |
CT alone | 61 (33.33%) |
CRT | 108 (59.02%) |
CT regimen | |
L-Asp-based | 83 (45.36%) |
Gemcitabine | 28 (15.30%) |
CHOP or CHOP-like | 39 (21.31%) |
Others | 19 (10.38%) |
UADT, upper aerodigestive tract NK/T-cell lymphoma; LN, Regional lymph node involvement; RT, radiotherapy; CT, chemotherapy; CRT, chemoradiotherapy; L-Asp, L-asparaginase.
3.2Risk construction and survival analysis
The cut-off values of inflammatory biomarkers are listed in Table S1. Higher PNI (
(2)
Before PSM, the 5-year PFS and OS rates in the high-risk group were 24.0% vs. 33.1%, respectively, whereas those in the low-risk group were 58.6% vs. 66.5%, respectively (Fig. 1). The baseline characteristics distribution between high risk and low risk has significant differences. To better control the bias caused by retrospective clinical data, the PSM method was applied to adjust the differences between variables so that all characteristics were well-balanced (Table 2). After PSM, the 5-year PFS and OS rates in the high-risk group were 29.2% vs. 40.8%, respectively, whereas those in the low-risk group were 62.1% vs. 72.4%, respectively (Fig. 1).
Figure 1.
The survival curves of OS and PFS for risk groups before (A, C) and after PSM (B, D).
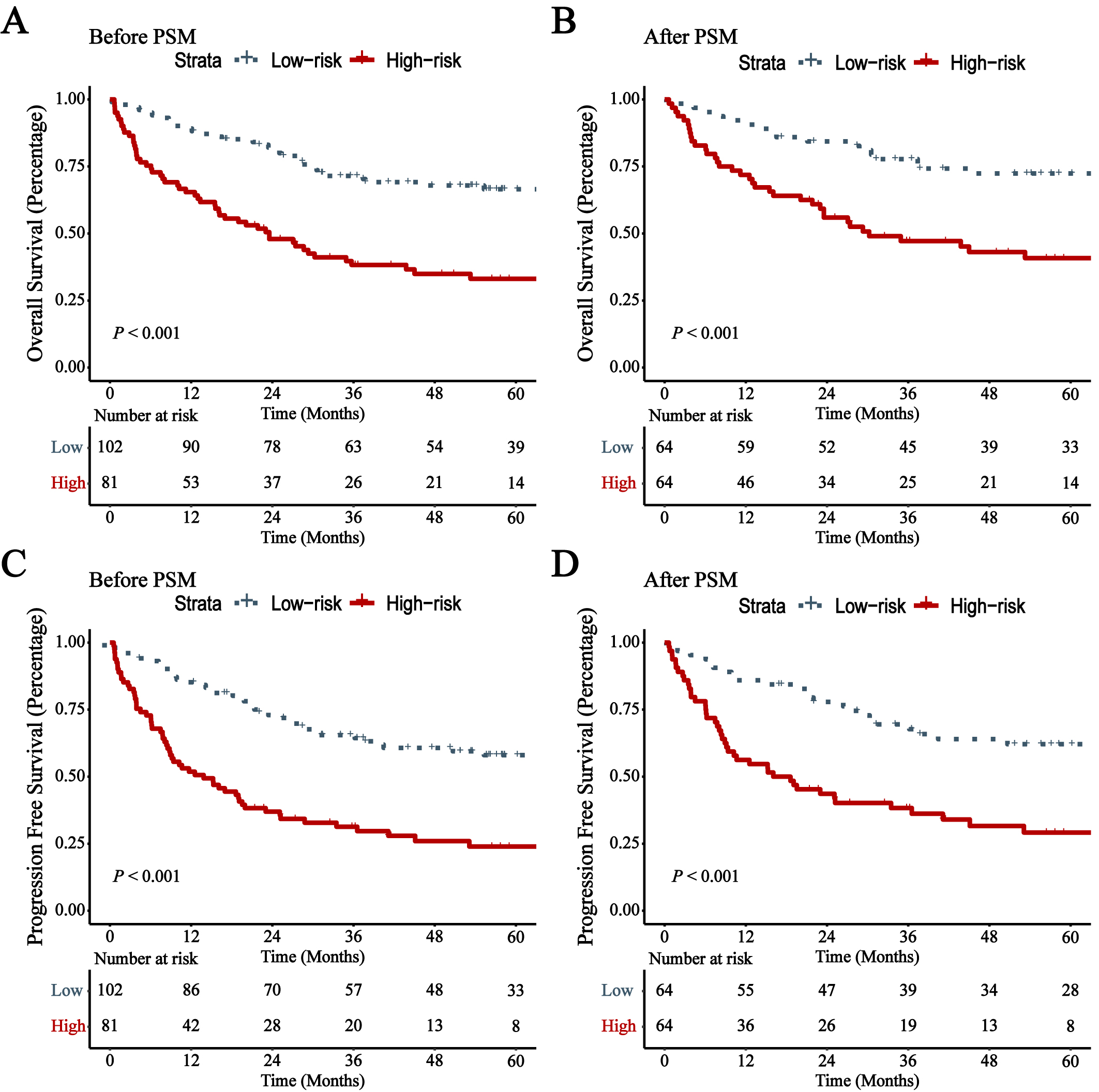
Table 2
Distribution of clinical characteristics of risk before and after PSM
Characteristics | Before PSM | After PSM | ||||
---|---|---|---|---|---|---|
Low risk | High risk | Low risk | High risk | |||
Age | 0.439 | 0.214 | ||||
| 84 (82.35%) | 63 (77.78%) | 57 (89.06%) | 52 (81.25%) | ||
| 18 (17.65%) | 18 (22.22%) | 7 (10.94%) | 12 (18.75%) | ||
Gender | 0.037 | 0.051 | ||||
Male | 86 (84.31%) | 58 (71.6%) | 55 (85.94%) | 46 (71.88%) | ||
Female | 16 (15.69%) | 23 (28.4%) | 9 (14.06%) | 18 (28.12%) | ||
ECOG | 0.626 | |||||
0–1 | 93 (91.18%) | 54 (66.67%) | 55 (85.94%) | 53 (82.81%) | ||
| 9 (8.82%) | 27 (33.33%) | 9 (14.06%) | 11 (17.19%) | ||
Ann arbor stage | 0.023 | 0.536 | ||||
I–II | 85 (83.33%) | 56 (69.14%) | 50 (78.12%) | 47 (73.44%) | ||
III–IV | 17 (16.67%) | 25 (30.86%) | 14 (21.88%) | 17 (26.56%) | ||
B symptoms | 0.016 | 0.719 | ||||
Yes | 29 (28.43%) | 37 (45.68%) | 25 (39.06%) | 27 (42.19%) | ||
No | 73 (71.57%) | 44 (54.32%) | 39 (60.94%) | 37 (57.81%) | ||
No. of extranodal sites | 0.010 | 0.435 | ||||
| 94 (92.16%) | 64 (79.01%) | 57 (89.06%) | 54 (84.38%) | ||
| 8 (7.84%) | 17 (20.99%) | 7 (10.94%) | 10 (15.62%) | ||
LN | 0.388 | 0.102 | ||||
Yes | 34 (33.33%) | 32 (39.51%) | 12 (18.75%) | 20 (31.25%) | ||
No | 68 (66.67%) | 49 (60.49%) | 52 (81.25%) | 44 (68.75%) | ||
Primary site | 0.653 | 1 | ||||
UADT | 95 (93.14%) | 74 (91.36%) | 59 (92.19%) | 59 (92.19%) | ||
Non-UADT | 7 (6.86%) | 7 (8.64%) | 5 (7.81%) | 5 (7.81%) | ||
RT | 0.002 | 1 | ||||
Yes | 78 (76.47%) | 44 (54.32%) | 41 (64.06%) | 41 (64.06%) | ||
No | 24 (23.53%) | 37 (45.68%) | 23 (35.94%) | 23 (35.94%) | ||
L-Asp-based CT | 0.706 | 0.215 | ||||
No | 57 (55.88%) | 43 (53.09%) | 26 (40.62%) | 33 (51.56%) | ||
Yes | 45 (44.12%) | 38 (46.91%) | 38 (59.38%) | 31 (48.44%) |
UADT, upper aerodigestive tract NK/T-cell lymphoma; LN, Regional lymph node involvement; RT, radiotherapy; L-Asp, L-asparaginase; CT, chemotherapy.
Then, we used univariate and multivariate analyses to evaluate the prognostic value of the included factors. The results are shown in Table 2. Before PSM, multivariate analysis showed that Risk was significantly associated with OS (HR
Table 3
Univariate and multivariate Cox analyses for OS before and after PSM
Characteristics | Overall survival | |||||||
---|---|---|---|---|---|---|---|---|
Before PSM | After PSM | |||||||
Univariate analyses | Multivariate analyses | Univariate analyses | Multivariate analyses | |||||
HR (95% CI) | HR (95% CI) | HR (95% CI) | HR (95% CI) | |||||
Age | 1.669 (1.027–2.712) | 0.039 | 1.662 (1.019–2.711) | 0.042 | 1.932 (1.017–3.672) | 0.044 | 1.888 (0.985–3.618) | 0.056 |
Gender | 1.282 (0.784–2.098) | 0.322 | 1.394 (0.760–2.559) | 0.283 | ||||
ECOG | 3.109 (1.971–4.905) | 1.887 (1.129–3.154) | 0.015 | 2.104 (1.125–3.936) | 0.020 | 2.124 (1.127–4.004) | 0.020 | |
Ann arbor stage | 1.505 (0.931–2.432) | 0.095 | 1.417 (0.779–2.578) | 0.254 | ||||
B symptoms | 0.935 (0.600–1.458) | 0.768 | 0.762 (0.440–1.320) | 0.332 | ||||
No. of extranodal sites | 1.644 (0.924–2.924) | 0.091 | 1.182 (0.533–2.622) | 0.680 | ||||
LN | 0.628 (0.408–0.966) | 0.034 | 0.565 (0.317–1.007) | 0.053 | ||||
Primary site | 1.027 (0.474–2.228) | 0.945 | 1.254 (0.499–3.151) | 0.630 | ||||
RT | 0.483 (0.315–0.740) | 0.001 | 0.578 (0.365–0.916) | 0.020 | 0.553 (0.325–0.942) | 0.029 | 0.528 (0.309–0.902) | 0.019 |
L-Asp-based CT | 0.557 (0.357–0.869) | 0.010 | 0.501 (0.316–0.792) | 0.003 | 0.635 (0.366–1.100) | 0.105 | ||
Risk | 2.921 (1.886–4.524) | 2.577 (1.614–4.114) | 2.849 (1.616–5.022) | 2.829 (1.601–5.001) | ||||
Progression free survival | ||||||||
Age | 1.295 (0.814–2.061) | 0.275 | 1.364 (0.731–2.545) | 0.330 | ||||
Gender | 1.336 (0.850–2.099) | 0.209 | 1.388 (0.802–2.405) | 0.242 | ||||
ECOG | 2.662 (1.721–4.116) | 1.532 (0.936–2.508) | 0.090 | 1.824 (1.010–3.296) | 0.046 | 1.605 (0.885–2.911) | 0.120 | |
Ann arbor stage | 1.151 (0.723–1.834) | 0.553 | 0.992 (0.558–1.761) | 0.977 | ||||
B symptoms | 0.953 (0.635–1.430) | 0.817 | 0.767 (0.469–1.254) | 0.291 | ||||
No. of extranodal sites | 1.293 (0.734–2.277) | 0.374 | 0.873 (0.399–1.911) | 0.734 | ||||
LN | 0.702 (0.471–1.047) | 0.083 | 0.713 (0.415–1.224) | 0.219 | ||||
Primary site | 1.100 (0.555–2.180) | 0.785 | 1.144 (0.494–2.647) | 0.753 | ||||
RT | 0.476 (0.322–0.704) | 0.591 (0.386–0.903) | 0.015 | 0.520 (0.323–0.836) | 0.007 | 0.506 (0.313–0.816) | 0.005 | |
L-Asp-based CT | 0.645 (0.433–0.960) | 0.031 | 0.567 (0.377–0.853) | 0.007 | 0.713 (0.439–1.157) | 0.170 | ||
Risk | 2.911 (1.952–4.341) | 2.679 (1.744–4.114) | 2.837 (1.715–4.695) | 2.877 (1.735–4.770) |
UADT, upper aerodigestive tract NK/T-cell lymphoma; LN, Regional lymph node involvement; RT, radiotherapy; L-Asp, L-asparaginase; CT, chemotherapy.
Figure 2.
The survival curves of OS and PFS for high- and low-risk patients who received L-asp before (A, B) and after PSM (E, F); The survival curves of OS and PFS for high- and low-risk patients who received RT before (C, D) and after PSM (G, H).
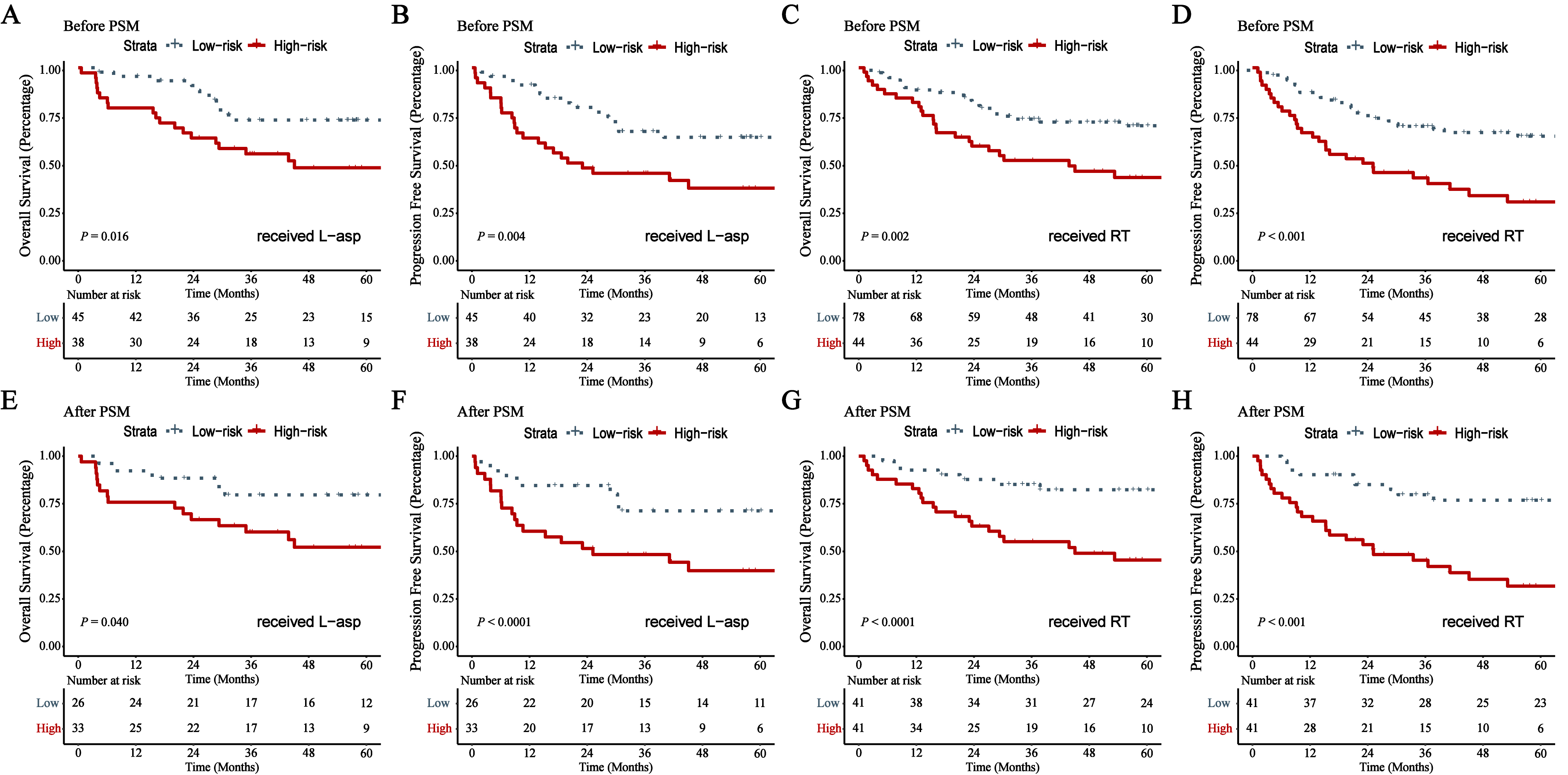
Figure 3.
Time-dependent ROC curves of the NRI and RISK-NRI.
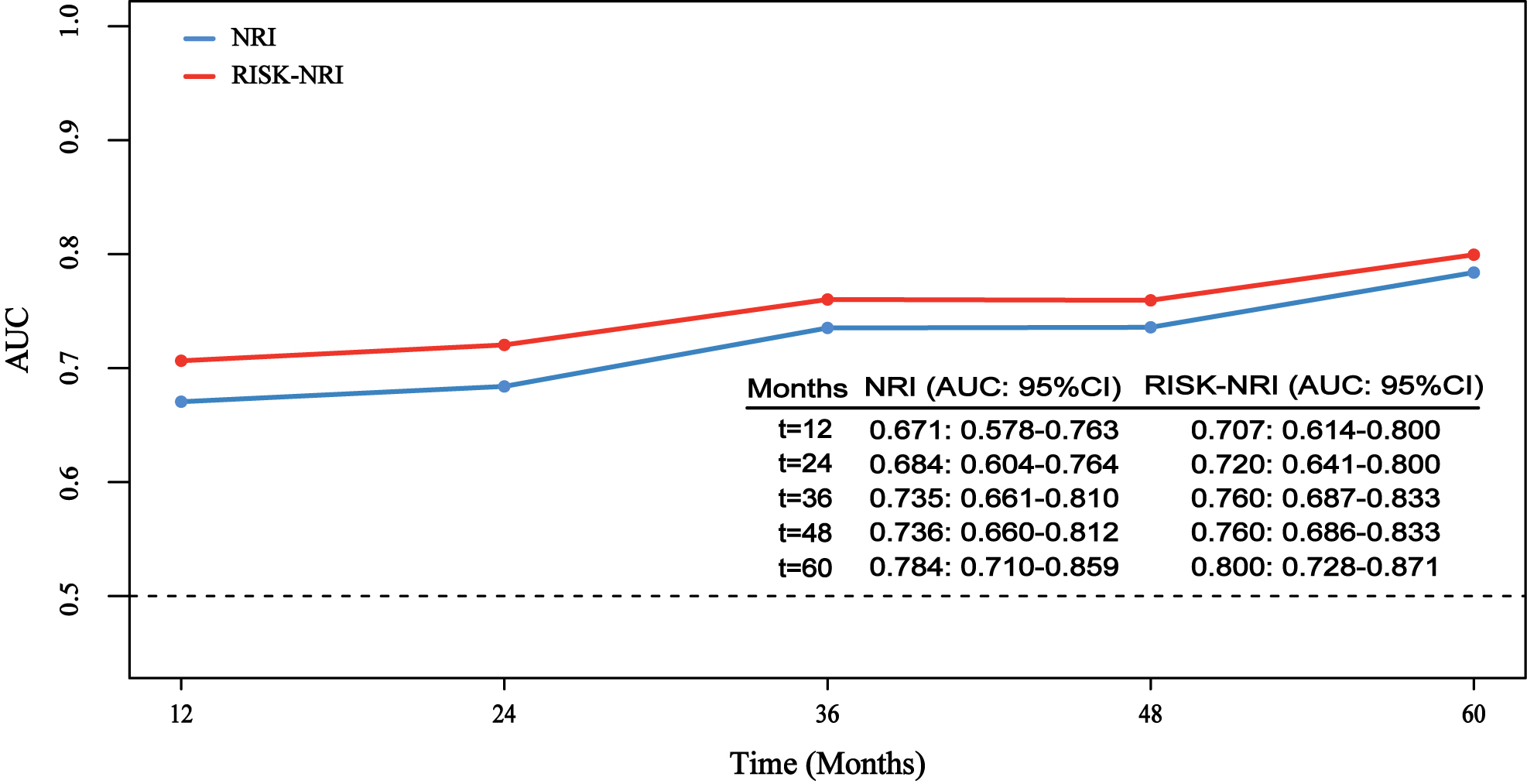
Figure 4.
Decision curve analysis of the NRI and Risk-NRI.
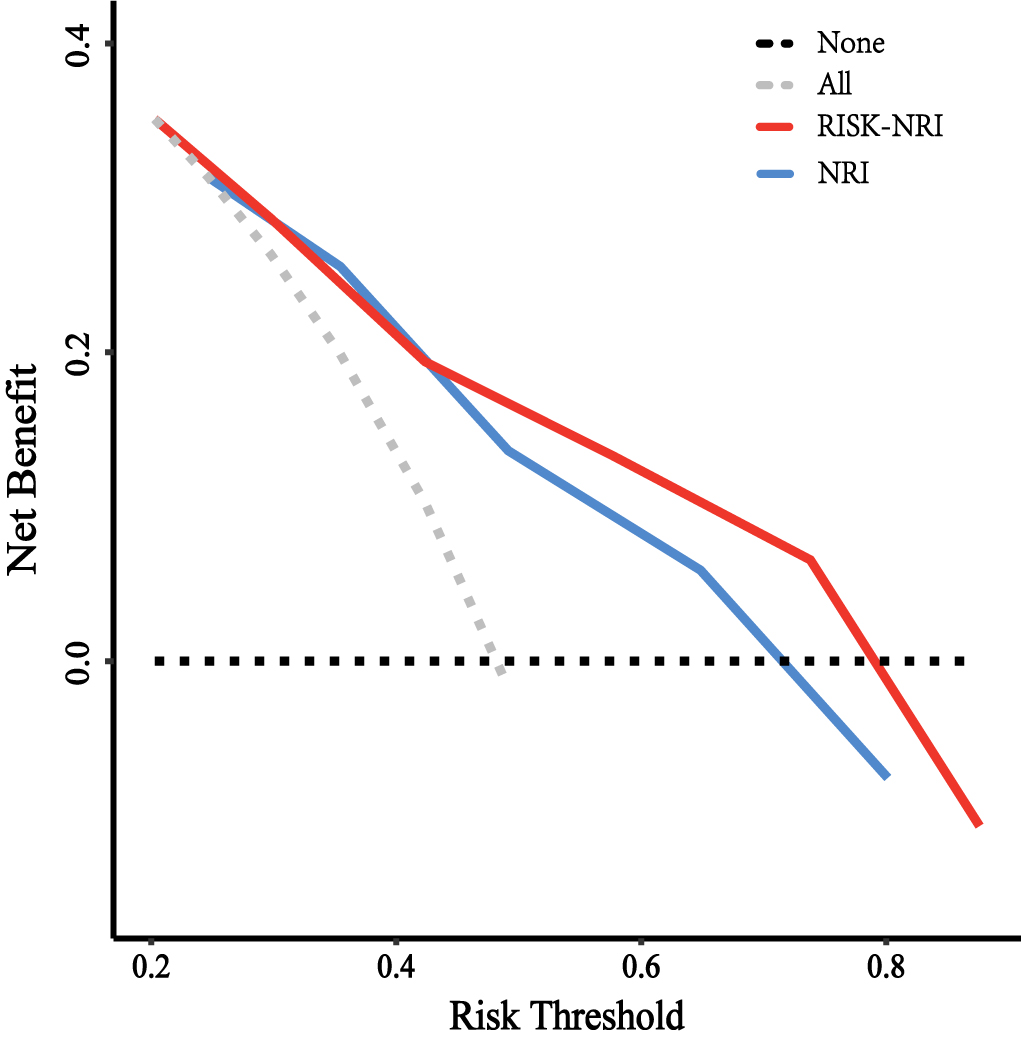
3.3Subgroup analysis
Before PSM, among 122 patients who received radiotherapy, the 5-year OS rates of the high- and low-risk groups were 42.4% and 69.6%, respectively (
3.4Modified NRI by integrating risk
At 12, 24, 36, 48, and 60 months, the revised NRI predicted AUCs for OS of 0.707, 0.720, 0.760, 0.760, and 0.800, respectively, while the NRI predicted AUCs for OS of 0.671, 0.684, 0.735, 0.736, and 0.784, respectively (Fig. 3). The 5-fold cross-validation also showed that the AUC of the modified NRI in predicting the prognosis of ENKTCL at 0–60 months was higher than that of the NRI (Fig. S2). The decision curve showed that when predicting 5-year OS, the NRI had more clinical net benefit than “none” or “all” in the threshold probability from 0.246 to 0.801. In contrast, the modified NRI had a higher clinical net benefit in the threshold probability from 0.203 to 0.876 (Fig. 4). The IBSs in the NRI were 0.100, 0.139, 0.162, 0.173, and 0.179, respectively, and 0.096, 0.133, 0.154, 0.164, and 0.169 in the modified NRI, respectively (Fig. S3). The integrated discrimination improvement (IDI), continuous-net reclassification improvement (NRI), and median improvement in risk score were used to assess additional prognostic discrimination of the modified NRI (Table S2), and significant predictive performance improvements were observed compared with the NRI within 12, 24, 36, 48 and 60 months.
4.Discussion
ENKTCL is usually characterized by necrosis and inflammation. However, little is known about the effects of systemic inflammatory markers in ENKTCL. We used univariate Cox regression and LASSO Cox to integrate independent prognostic inflammatory markers proven by previous studies and constructed a comprehensive inflammatory index named Risk. We found that Risk was a prognostic factor for PFS and OS in ENKTCL patients. Moreover, the combination of Risk and NRI improves the performance of the NRI.
Systemic inflammation and malnutrition are important components of cancer [17, 18]. Malnutrition is responsible for nearly one-fifth of patients dying [19]. In addition to liver function, albumin is also one of the indicators of nutritional status and has been proven to be associated with the prognosis of various malignant tumours. Li et al. [20] found that albumin was independent of PFS (RR
In our study, the Risk based on six inflammatory biomarkers effectively stratified OS and PFS in ENKTCL patients. High-risk patients benefit less from L-Asp-based chemotherapy and radiotherapy. Moreover, even if age, sex, extranodal site of involvement, ECOG score, Ann Arbor Staging System, B symptoms, regional lymph node involvement, primary site, and radiotherapy were adjusted by PSM analysis and multivariate analysis, the OS and PFS of patients with high risk were worse than those of patients with low risk. Furthermore, after combining Risk into the NRI, the modified NRI performance was improved, which can be demonstrated by AUC, IBS, and clinical net benefit. In the modified NRI performance at 12, 24, 36, 48, and 60 months, the AUC increased by 0.036, 0.036, 0.025, 0.024, and 0.016, respectively. IBS decreased by 0.004, 0.004, 0.008, 0.009, and 0.01, respectively. DCA shows that the modified NRI has favourable clinical application across a wider range of threshold probabilities. Adding Risk to the NRI also improved IDI, continuous NRI, and median improvement in risk score.
Although this study showed that integrating Risk could significantly improve the predictive performance of the NRI, there are still some limitations. First, this was a single-centre retrospective study with a small sample size and a lack of external validation. Second, because C-reactive protein was not counted, Glasgow’s prognostic score was not included in the analysis. Third, the optimal cut-off value of the inflammatory index was determined according to the maximally selected rank statistics method, which may be inconsistent between different centres. In conclusion, further multicentre large-scale studies are needed to confirm the conclusions of this study.
5.Conclusions
Risk is routinely available and inexpensive and can predict the prognosis of ENKTCL. Combining Risk and NRI can effectively improve the prediction performance.
Author contributions
Conception: Cao J.
Interpretation or analysis of data: Hou Q, Li H, Liang Y, Yao N, Cao X, Sun B, Feng P, Zhang W, Liu J.
Preparation of the manuscript: Hou Q, Li H, Yao N, Cao X, Liu J, Sun B, Feng P, Zhang W.
Revision for important intellectual content: Liu J, Cao J.
Supervision: Cao J.
Funding
This research was funded by the Applied Basic Research Projects of Shanxi Province [No. 20210302124598], Wu Jieping Medical Foundation No. 320.6750.2022-1-53, Lianyungang Yixing Medical Health Foundation, the Research Project Supported by Shanxi Scholarship Council of China No. [2022]210, and the Four “Batches” Innovation Project of Invigorating Medical through Science and Technology of Shanxi Province No. 2022XM32.
Institutional review board statement
This study was approved by the Ethics Committee of Shanxi Province Cancer Hospital, Shanxi Hospital Affiliated to Cancer Hospital, Chinese Academy of Medical Sciences, Cancer Hospital Affiliated to Shanxi Medical University, and the review committee exempted informed consent requirements on 3 September 2019. (Approval code 2019091).
Informed consent statement
Written informed consent was waived due to the retrospective study design.
Data availability statement
The datasets generated and analysed during this study are not publicly available due to ethical conditions but are available from the corresponding author on reasonable request.
Supplementary data
The supplementary files are available to download from http://dx.doi.org/10.3233/CBM-230067.
Conflict of interest
The authors declare no conflict of interest.
References
[1] | H. Wang, B.B. Fu, R.P. Gale and Y. Liang, Nk-/t-cell lymphomas, Leukemia 35: (9) ((2021) ), 2460–2468. |
[2] | S.H. Swerdlow, E. Campo, N.L. Harris, E.S. Jaffe, S.A. Pileri, H. Stein, J. Thiele, D.A. Arber, R.P. Hasserjian, M.M. Le Beau and R.S. Attilio Orazi, Who classification of tumours of haematopoietic and lymphoid tissues, 4th ed., IARC, 2017. |
[3] | J. Lee, C. Suh, Y.H. Park, Y.H. Ko, S.M. Bang, J.H. Lee, D.H. Lee, J. Huh, S.Y. Oh, H.C. Kwon, H.J. Kim, S.I. Lee, J.H. Kim, J. Park, S.J. Oh, K. Kim, C. Jung, K. Park and W.S. Kim, Extranodal natural killer t-cell lymphoma, nasal-type: A prognostic model from a retrospective multicenter study, J. Clin. Oncol. 24: (4) ((2006) ), 612–618. |
[4] | International Non-Hodgkin’s Lymphoma Prognostic Factors Project, A predictive model for aggressive non-hodgkin’s lymphoma, N. Engl. J. Med. 329: (14) ((1993) ), 987–994. |
[5] | A. Gallamini, C. Stelitano, R. Calvi, M. Bellei, D. Mattei, U. Vitolo, F. Morabito, M. Martelli, E. Brusamolino, E. Iannitto, F. Zaja, S. Cortelazzo, L. Rigacci, L. Devizzi, G. Todeschini, G. Santini, M. Brugiatelli and M. Federico, Peripheral t-cell lymphoma unspecified (ptcl-u): A new prognostic model from a retrospective multicentric clinical study, Blood 103: (7) ((2004) ), 2474–2479. |
[6] | S.J. Kim, D.H. Yoon, A. Jaccard, W.J. Chng, S.T. Lim, H. Hong, Y. Park, K.M. Chang, Y. Maeda, F. Ishida, D.Y. Shin, J.S. Kim, S.H. Jeong, D.H. Yang, J.C. Jo, G.W. Lee, C.W. Choi, W.S. Lee, T.Y. Chen, K. Kim, S.H. Jung, T. Murayama, Y. Oki, R. Advani, F. D’Amore, N. Schmitz, C. Suh, R. Suzuki, Y.L. Kwong, T.Y. Lin and W.S. Kim, A prognostic index for natural killer cell lymphoma after non-anthracycline-based treatment: A multicentre, retrospective analysis, Lancet Oncol. 17: (3) ((2016) ), 389–400. |
[7] | S.Y. Chen, Y. Yang, S.N. Qi, Y. Wang, C. Hu, X. He, L.L. Zhang, G. Wu, B.L. Qu, L.T. Qian, X.R. Hou, F.Q. Zhang, X.Y. Qiao, H. Wang, G.F. Li, Y.J. Zhang, Y. Zhu, J.Z. Cao, S.M. Lan, J.X. Wu, T. Wu, S.Y. Zhu, M. Shi, L.M. Xu, Z.Y. Yuan, J. Yahalom, R. Tsang, Y.Q. Song, J. Zhu, H. Su and Y.X. Li, Validation of nomogram-revised risk index and comparison with other models for extranodal nasal-type nk/t-cell lymphoma in the modern chemotherapy era: Indication for prognostication and clinical decision-making, Leukemia 35: (1) ((2021) ), 130–142. |
[8] | Y. Yang, Y. Zhang, Y. Zhu, J. Cao, Z. Yuan, L. Xu, J. Wu, W. Wang, T. Wu, B. Lu, S. Zhu, L. Qian, F. Zhang, X. Hou, Q. Liu and Y. Li, Prognostic nomogram for overall survival in previously untreated patients with extranodal nk/t-cell lymphoma, nasal-type: A multicenter study, Leukemia 29: (7) ((2015) ), 1571–1577. |
[9] | Y. Yang, Y. Zhu, J.Z. Cao, Y.J. Zhang, L.M. Xu, Z.Y. Yuan, J.X. Wu, W. Wang, T. Wu, B. Lu, S.Y. Zhu, L.T. Qian, F.Q. Zhang, X.R. Hou and Y.X. Li, Risk-adapted therapy for early-stage extranodal nasal-type nk/t-cell lymphoma: Analysis from a multicenter study, Blood 126: (12) ((2015) ), 1424–1432, 1517. |
[10] | J.A. van Doesum, A. Niezink, G.A. Huls, M. Beijert, A. Diepstra and T. van Meerten, Extranodal natural killer/t-cell lymphoma, nasal type: Diagnosis and treatment, Hemasphere 5: (2) ((2021) ), e523. |
[11] | A. Mantovani, P. Allavena, A. Sica and F. Balkwill, Cancer-related inflammation, Nature 454: (7203) ((2008) ), 436–444. |
[12] | K.M. Tan, B. Chia, J.Q. Lim, L.P. Khoo, C.L. Cheng, L. Tan, E. Poon, N. Somasundaram, M. Farid, T. Tang, M. Tao, D. Cheah, Y. Laurensia, J. Pang, T. Song, J. Tan, D. Huang, S.J. Kim, W.S. Kim, C.K. Ong, S.T. Lim and J.Y. Chan, A clinicohaematological prognostic model for nasal-type natural killer/t-cell lymphoma: A multicenter study, Sci. Rep. 9: (1) ((2019) ), 14961. |
[13] | N. Yao, Q. Hou, S. Zhang, H. Xiao, Y. Liang, X. Xu, R. Guo, H. Li, S. Lan, H. Si and J. Cao, Prognostic nutritional index, another prognostic factor for extranodal natural killer/t cell lymphoma, nasal type, Front. Oncol. 10: ((2020) ), 877. |
[14] | S. Zhang, M. Li, F. Yuan, L. Chen, R. Mi, X. Wei, Y. Song and Q. Yin, The predictive value of dynamic monitoring of peripheral blood lymphocyte to monocyte ratio in patients with extranodal nk/t cell lymphoma, Cancer Cell Int. 19: ((2019) ), 272. |
[15] | X. Zhou, X. Sun, W. Zhao, X. Fang and X. Wang, Prognostic significance of peripheral blood absolute lymphocyte count and derived neutrophil to lymphocyte ratio in patients with newly diagnosed extranodal natural killer/t-cell lymphoma, Cancer Manag. Res. 11: ((2019) ), 4243–4254. |
[16] | V. Jurinovic, R. Kridel, A.M. Staiger, M. Szczepanowski, H. Horn, M.H. Dreyling, A. Rosenwald, G. Ott, W. Klapper, A.D. Zelenetz, P.M. Barr, J.W. Friedberg, S. Ansell, L.H. Sehn, J.M. Connors, R.D. Gascoyne, W. Hiddemann, M. Unterhalt, D.M. Weinstock and O. Weigert, Clinicogenetic risk models predict early progression of follicular lymphoma after first-line immunochemotherapy, Blood 128: (8) ((2016) ), 1112–1120. |
[17] | S.I. Grivennikov, F.R. Greten and M. Karin, Immunity, inflammation, and cancer, Cell 140: (6) ((2010) ), 883–899. |
[18] | P. Anandavadivelan and P. Lagergren, Cachexia in patients with oesophageal cancer, Nat. Rev. Clin. Oncol. 13: (3) ((2016) ), 185–198. |
[19] | J. Richards, M.B. Arensberg, S. Thomas, K.W. Kerr, R. Hegazi and M. Bastasch, Impact of early incorporation of nutrition interventions as a component of cancer therapy in adults: A review, Nutrients 12: (11) ((2020) ). |
[20] | Y.J. Li, P.Y. Yi, J.W. Li, X.L. Liu, X.Y. Liu, F. Zhou, Z. Ouyang, Z.Y. Sun, L.J. Huang, J.Q. He, Y. Yao, Z. Fan, T. Tang and W.Q. Jiang, Increased body mass index is associated with improved overall survival in extranodal natural killer/t-cell lymphoma, nasal type, Oncotarget 8: (3) ((2017) ), 4245–4256. |
[21] | G.P. Dunn, L.J. Old and R.D. Schreiber, The immunobiology of cancer immunosurveillance and immunoediting, Immunity 21: (2) ((2004) ), 137–148. |
[22] | J.M. Price, H.B. Mistry, G. Betts, E.J. Cheadle, L. Dixon, K. Garcez, T. Illidge, Z. Iyizoba-Ebozue, L.W. Lee, A. Mcpartlin, R. Prestwich, S. Papageorgiou, D.J. Pritchard, A. Sykes, C.M. West and D.J. Thomson, Pretreatment lymphocyte count predicts benefit from concurrent chemotherapy with radiotherapy in oropharyngeal cancer, J. Clin. Oncol. 40: (20) ((2022) ), 2203–2212. |
[23] | B. Weide, A. Martens, J.C. Hassel, C. Berking, M.A. Postow, K. Bisschop, E. Simeone, J. Mangana, B. Schilling, A.M. Di Giacomo, N. Brenner, K. Kähler, L. Heinzerling, R. Gutzmer, A. Bender, C. Gebhardt, E. Romano, F. Meier, P. Martus, M. Maio, C. Blank, D. Schadendorf, R. Dummer, P.A. Ascierto, G. Hospers, C. Garbe and J.D. Wolchok, Baseline biomarkers for outcome of melanoma patients treated with pembrolizumab, Clinical Cancer Research: An Official Journal of the American Association for Cancer Research 22: (22) ((2016) ), 5487–5496. |
[24] | M.E. Johnson, F. Zhu, T. Li, H. Wu, T.J. Galloway, J.M. Farma, C.S. Perlis and A. Turaka, Absolute lymphocyte count: A potential prognostic factor for merkel cell carcinoma, J. Am. Acad. Dermatol. 70: (6) ((2014) ), 1028–1035. |
[25] | X. Zhu, S.Q. Wu, R. Xu, Y.H. Wang, Z.H. Zhong, L. Zhang and X.K. Zhao, The evaluation of monocyte lymphocyte ratio as a preoperative predictor in urothelial malignancies: A pooled analysis based on comparative studies, Sci. Rep. 9: (1) ((2019) ), 6280. |
[26] | T. Ding, J. Xu, F. Wang, M. Shi, Y. Zhang, S. Li and L. Zheng, High tumor-infiltrating macrophage density predicts poor prognosis in patients with primary hepatocellular carcinoma after resection, Hum. Pathol. 40: (3) ((2009) ), 381–389. |
[27] | S. Yin, J. Huang, Z. Li, J. Zhang, J. Luo, C. Lu, H. Xu and H. Xu, The prognostic and clinicopathological significance of tumor-associated macrophages in patients with gastric cancer: a meta-analysis, Plos One 12: (1) ((2017) ), e170042. |
[28] | S. Asgharzadeh, J.A. Salo, L. Ji, A. Oberthuer, M. Fischer, F. Berthold, M. Hadjidaniel, C.W. Liu, L.S. Metelitsa, R. Pique-Regi, P. Wakamatsu, J.G. Villablanca, S.G. Kreissman, K.K. Matthay, H. Shimada, W.B. London, R. Sposto and R.C. Seeger, Clinical significance of tumor-associated inflammatory cells in metastatic neuroblastoma, J. Clin. Oncol. 30: (28) ((2012) ), 3525–3532. |
[29] | J.J. Huang, Y.J. Li, Y. Xia, Y. Wang, W.X. Wei, Y.J. Zhu, T.Y. Lin, H.Q. Huang, W.Q. Jiang and Z.M. Li, Prognostic significance of peripheral monocyte count in patients with extranodal natural killer/t-cell lymphoma, Bmc Cancer 13: ((2013) ), 222. |
[30] | J.H. Liang, L. Lu, H.Y. Zhu, W. Li, L. Fan, J.Y. Li and W. Xu, The prognostic role of circulating epstein-barr virus dna copy number in angioimmunoblastic t-cell lymphoma treated with dose-adjusted epoch, Cancer Res. Treat. 51: (1) ((2019) ), 150–157. |
[31] | H. Chen, Y. Qin, J. Yang, P. Liu, X. He, S. Zhou, C. Zhang, L. Gui, S. Yang and Y. Shi, The pretreatment platelet count predicts survival outcomes of diffuse large b-cell lymphoma: An analysis of 1007 patients in the rituximab era, Leuk. Res. 110: ((2021) ), 106715. |
[32] | M. Choi, J.O. Lee, J. Jung, J.Y. Lee, E. Lee, H. Lee, S.M. Bang, H.S. Eom and J.S. Lee, Prognostic value of platelet count in patients with peripheral t cell lymphoma, Acta Haematol. 141: (3) ((2019) ), 176–186. |
[33] | P.P. Xu, Y. Wang, Y. Shen, L. Wang, Z.X. Shen and W.L. Zhao, Prognostic factors of chinese patients with t/nk-cell lymphoma: A single institution study of 170 patients, Med. Oncol. 29: (3) ((2012) ), 2176–2182. |
[34] | M. Li, H. Xia, H. Zheng, Y. Li, J. Liu, L. Hu, J. Li, Y. Ding, L. Pu, Q. Gui, Y. Zheng, Z. Zhai and S. Xiong, Red blood cell distribution width and platelet counts are independent prognostic factors and improve the predictive ability of ipi score in diffuse large b-cell lymphoma patients, Bmc Cancer 19: (1) ((2019) ), 1084. |