Capillary microsampling-based single-cell metabolomics by mass spectrometry and its applications in medicine and drug discovery
Abstract
Characterization of cellular metabolic states is a technical challenge in biomedicine. Cellular heterogeneity caused by inherent diversity in expression of metabolic enzymes or due to sensitivity of metabolic reactions to perturbations, necessitates single cell analysis of metabolism. Heterogeneity is typically seen in cancer and thus, single-cell metabolomics is expectedly useful in studying cancer progression, metastasis, and variations in cancer drug response. However, low sample volumes and analyte concentrations limit detection of critically important metabolites. Capillary microsampling-based mass spectrometry approaches are emerging as a promising solution for achieving single-cell omics. Herein, we focus on the recent advances in capillary microsampling-based mass spectrometry techniques for single-cell metabolomics. We discuss recent technical developments and applications to cancer medicine and drug discovery.
1.Introduction
Cellular heterogeneity is now an established principle in the field of biology, where variations in genetic, proteomic, metabolic, and structural levels contribute to the individuality of single-cells [1]. Traditionally, population averaging methods have been used to gain insights into cellular biology and tissue functions. While convenient and undoubtedly powerful, they disregard any potential heterogeneity between cells. Since the measurements are performed with averaged populations, potential sub-populations such as resistant tumor sub-populations are often obscured. Therefore, single-cell measurements in biological studies are indispensable to account for the inherent heterogeneity of cells. In contrast to the significant advancements in single-cell genomics and transcriptomics, single cell analyses of proteomics and metabolomics have somewhat lagged behind. This is due to the fact that unlike genetic materials, proteins and metabolites signals cannot be amplified, thus, unprecedented detection sensitivities are required (Table 1).
Table 1
Comparison between the advantages and limitations of current single-cell omics approaches
Single cell omics | Genomics and transcriptomics | Proteomics | Metabolomics |
---|---|---|---|
Advantages |
|
|
|
Limitations |
|
|
|
Metabolomics is concerned with the analysis of small molecular weight compounds, typically known as metabolites, where metabolic reactions describe the biochemical activities governing the cell behavior in real-time. This in turn helps in deciphering the functional phenotype of a given cell. Nonetheless, the lack of adequate metabolic data has created a phenotype-genotype gap in our understanding of the individual variations in cellular behavior. Overall, metabolomics offers a comprehensive image of the cellular reaction network and unraveling it in single-cells can help us address a myriad of scientific and medical questions, including cancer and antimicrobial drug resistance, immune cell plasticity, and cellular growth variability.
Analyzing the metabolome of single-cells is not an easy task. The high degree of dynamic change in metabolic reactions (minutes to seconds), coupled with the pico-scale volumes of single-cells [2] require complex sample preparation steps as well as ultra-sensitive analytical methods. Assuming that the metabolic reactions in a given cell have been quenched successfully, the success of any given method in analyzing single-cells depends on two key parameters: First, its ability to detect relatively low concentrations of a given metabolite (sensitivity) and second, its ability to distinguish between metabolites appearing in the same range (resolution). Thus, the sensitivity power of a method is crucial for determining its ability to perform single-cell measurements. Furthermore, since the volumes involved are in the pico-liter scale, most traditional separation techniques are often not suitable due to the possible significant sample dilution and loss. Instead, the resolution of a given method is critical in distinguishing between closely related molecules. With the aforementioned considerations in mind, mass spectrometry (MS) stands out among the multitude of approaches developed to successfully measure the metabolism in single-cells. MS measures mass to charge ratios of molecules and their fragments, from which one can deduce the chemical formula and the structure of a given compound. In addition to qualitative information, one can also produce quantitative data about the abundance of the compound. MS is especially suited for metabolic studies, since most metabolites are polar, which makes them easily ionized and measured by MS. Overall, MS-based approaches are uniquely suited for single-cell metabolic studies using capillary microsampling-based techniques.
In this review, some of the MS-based approaches that were used in single-cell metabolic studies are highlighted, mainly, capillary microsampling-based approaches. Moreover, the biological, pharmaceutical, and medical applications of single-cell metabolomics are discussed.
2.Methodologies
2.1Sample considerations
Sample preparation is one of the most essential aspects of single-cell MS analysis. There are multiple approaches to extract and enrich single-cell contents, which are excellently summarized in previous publications [3, 4, 5, 6, 7]. Regardless of the method used, two important caveats should be kept in mind: The first is ensuring that there are minimal perturbations to the cellular microenvironment, and secondly, metabolic reactions must be halted in a way that is efficient, fast, and with minimal metabolite leakage outside the cell. This is critical to ensure that the measured metabolites and metabolic reactions are as accurate as possible in representing the cellular state [8]. In mammalian cells, quenching of the metabolic processes can be achieved by snap freezing the cell after sampling, chemical fixation or by adding cold mixture (
Table 2
Comparison of different specifications of available mass spectrometry analyzers
Mass analyzer | Resolution | Mass accuracy | Sensitivity | Scan speed | Footprint | Cost |
---|---|---|---|---|---|---|
Time of flight (TOF) | Moderate | Moderate | High | Moderate | Moderate to low | Moderate to low |
Orbitrap | High | High | Moderate | Low | Moderate | High |
Triple quadrupole | Low | Low | High | High | Very low | Low |
Ion trap | Low | Low | Moderate | High | Low | Low |
Fourier Transform (FTICR) | Very high | Very high | Moderate | Very low | High | High |
Table 3
Comparison between the advantages and limitations of online and offline microsampling techniques
Microsampling techniques | Offline | Online |
---|---|---|
Advantages |
|
|
Limitations |
|
|
The recent advances in MS analyzers performance (Table 2), i.e., high selectivity and sensitivity, multiplexing capability, and the ability to perform unlabeled analysis, made MS the method of choice for single-cell metabolomics studies. Single-cell MS analysis is generally performed by microsampling the cell in question and subsequent MS analysis. It generally entails using a pulled glass capillary to sample the whole cell, or part of it, followed by introducing the sampled cellular contents into the MS instrument for the analysis, typically, using nano electrospray ionization (nano-ESI).
Minimizing dilution is essential in microsampling single-cells, mainly due to the low sample volumes which is in the picolitres scale for a typical mammalian cell [10]. Therefore, most microsampling techniques forgo extensive sample manipulation and treatment and favor more simple and integrated approaches that can transfer and extract the sampled cells with minimal dilution prior to MS analysis. The other concern, shared among all microsampling methods, is minimizing perturbation to the cellular microenvironment during sampling. To address this, most techniques use some forms of pulled glass capillary as a pipette to aspirate the target cell, or part of it, with visual feedback given by some forms of microscopy. The glass capillary is usually controlled by a micromanipulator capable of moving in three dimensions with resolution approaching 1
2.2Offline microsampling techniques
In offline microsampling methods, parts of, or all three steps (sampling, treatment, and analysis) are separated into discrete, and disconnected modules. Masujima group is among the first to attempt this with their live single cell mass spectrometry approach (LSC-MS) [11]. LSC-MS attempts to reduce dilution by utilizing the same pulled glass capillary in all stages of the experiment (sampling, treatment, and measurement), which results in sensitive analysis due to minimal sample manipulation and dilution (Fig. 1a).
Figure 1.
Working principle of Live-Single-Cell Mass Spectrometry platform (LSC-MS). Single cells or their contents are first sampled using pulled glass capillaries under microscopic observation and are then directly nanosprayed for subsequent MS measurements (a). Incorporation of ion mobility setup can improve the analysis resolution (b).
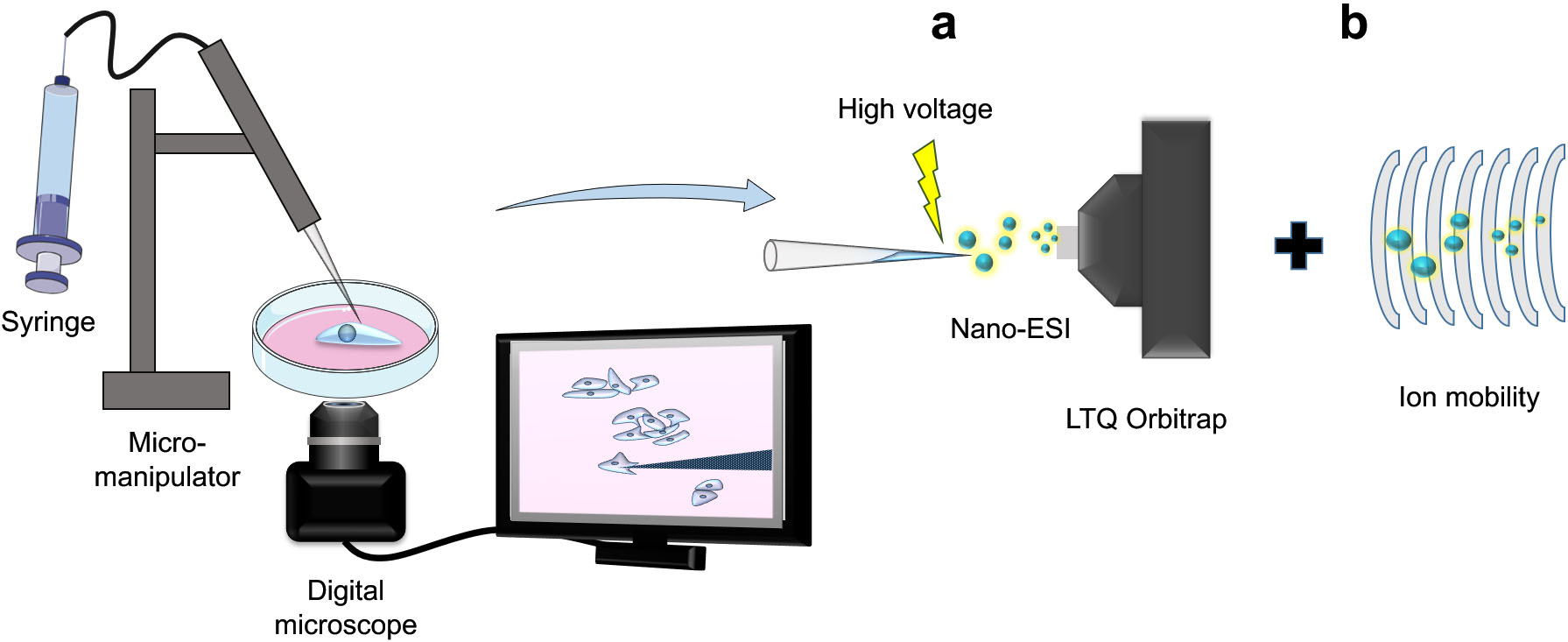
Figure 2.
Workflow of the nanomanipulation-coupled mass spectrometry technique. This technique utilizes two nanomanipulators, one for injecting the extraction solvent into a target cell, while the other for breaching the cell membrane before the extraction process to prevent clogging. Afterwards, the extracted cellular contents will be aspirated using the first nanomanipulator for direct MS analysis.
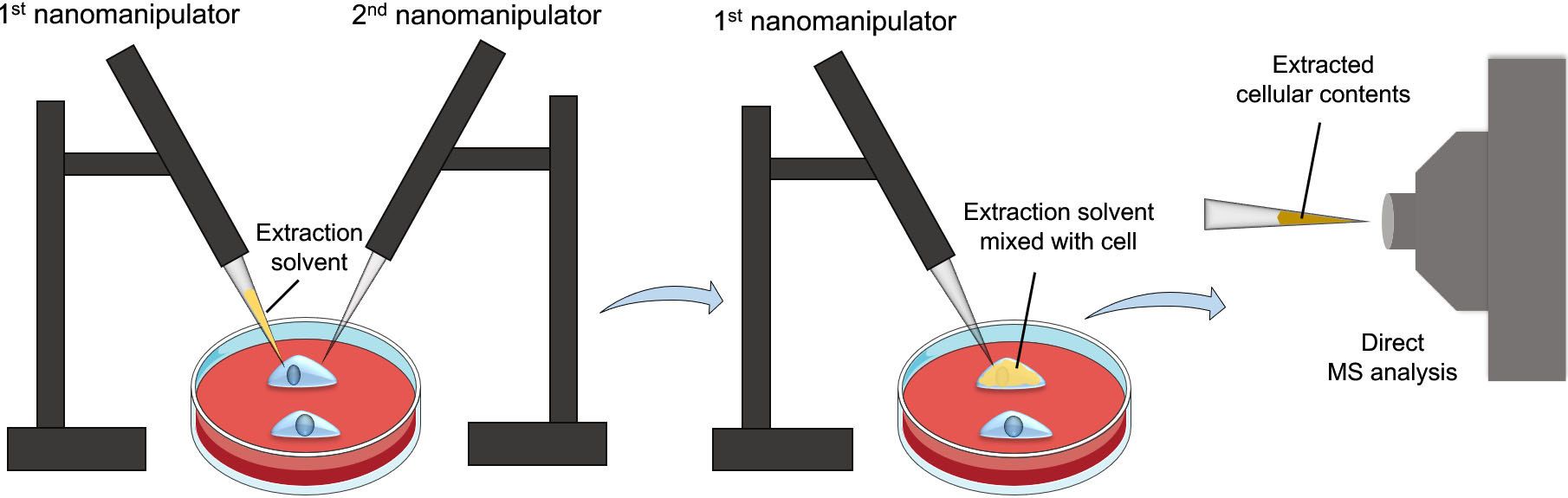
LSC-MS employs a pulled glass capillary, with tip diameters ranging from 1–10
One limitation of LSC-MS and similar approaches is that the extracted cellular contents trapped in the capillary are directly introduced into the MS instrument without chromatographic separation, i.e., while improving sensitivity, it negatively impacts selectivity. For example, discerning between isometric compounds is not possible using LSC-MS. Several groups attempted to rectify this by incorporating ion mobility into the protocol (Fig. 1b) [11, 19]. By incorporating a separation step after introducing the sample into the MS instrument, overall specificity of the method can be increased, without compromising sensitivity. However, it is worth noting that this complicates the data processing and analysis, especially in untargeted metabolomics experiments.
To enhance specificity and selectivity of the detected metabolites even further, an interesting approach has been developed by Pheleps et al., termed nanomanipulation-coupled nanospray mass spectrometry [20]. The principle of this approach is to insert the tip of a coated capillary, prefilled with an extraction solvent, to a target cell in a tissue section or live cells and then inject the extraction solvent into the cell. The cellular contents are then immediately aspirated back into the tip for direct MS analysis (Fig. 2). To prevent capillary clogging and the analysis of unwanted cell membranes’ analytes, the authors incorporated another nanospray capillary to breach the cell’s membrane prior to extraction. Using this system, distinct difference and heterogeneity in triacylglycerols (TAGs) profiles of healthy and tumorous adipocytes were detected. Besides the advantages of combining nanomanipulation with nano-ESI, the precision of the nanoextraction process provides high analyte selectivity and resolution which eliminates the need for separation and pretreatment steps. In addition, rapid and accurate analysis of the analytes can be achieved without damaging the remains of the tissue section or cell culture [21].
The combination of the two inherent properties in single cell mass spectrometry (small sample size, and destructive measurement approach) means that there is often little time to perform comprehensive measurements of target cells. One consequence of this, is the difficulty of analyzing the structure of metabolites by fragmenting them and studying the fragmentation patterns (MS/MS analysis). Pico-ESI-MS is a technique that attempts to introduce a degree of miniaturized sample treatment to enable MS/MS profiling of more than 300 phospholipids [22]. In this technique, the sample capillary is used to introduce few nanoliters of a suitable extraction solvent to the target cell. Afterwards, the solvent, and the extract are sampled using the same capillary. Evaporation and reconstitution with 2 nanoliters of an ionization solvent is then performed and the capillary contents are introduced to the MS instrument for MS/MS analysis.
Figure 3.
Schematic of the improved T-probe platform. It consists of three components, a sampling probe for sampling a single cell, a lysing solvent-providing capillary and a nano-ESI emitter, all connected with a T-junction.
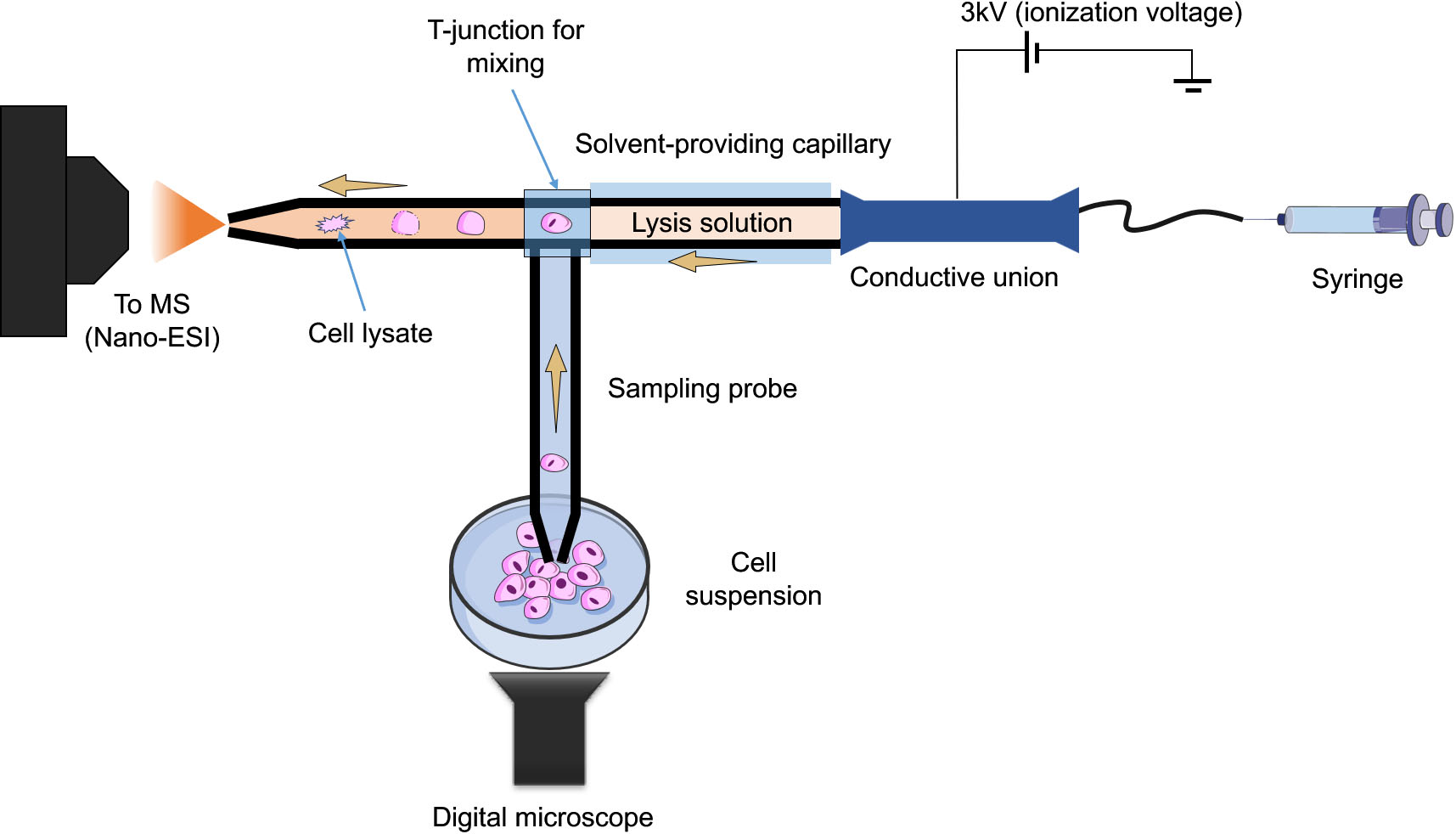
Figure 4.
Illustrative diagram showing the working principle of Internal Electrode Capillary Pressure Probe Electrospray Ionization Mass Spectrometry (IEC-PPESI-MS). The tips of the capillaries are directly inserted into the single cells (a) and cell components are electrosprayed by applying a high voltage to the internal electrode (b).
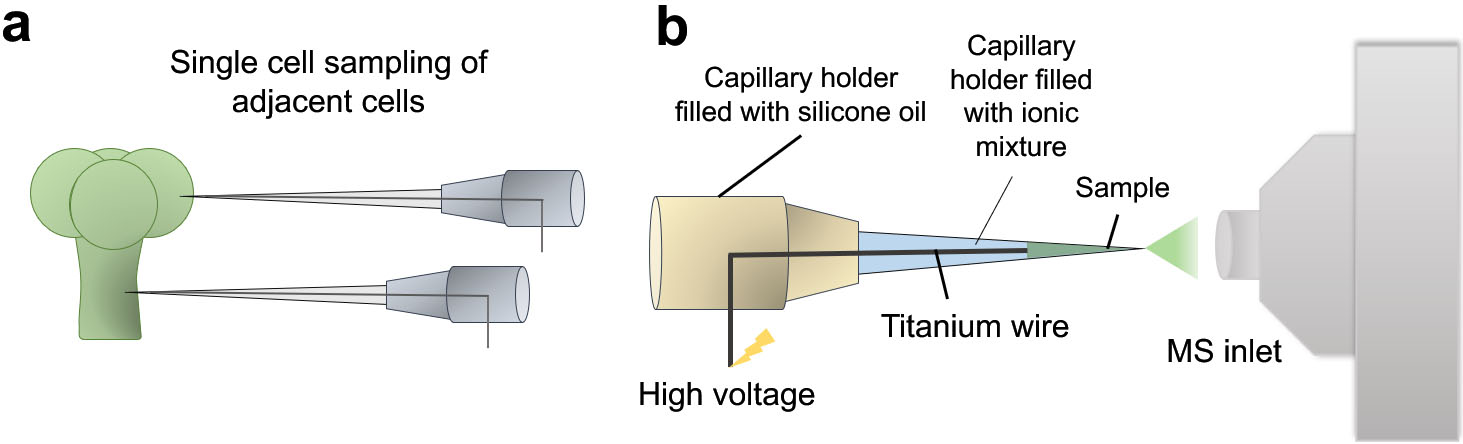
In offline microsampling techniques, sampling and analysis are usually separate. This often results in less complicated setups that are generally more robust. It also introduces the possibility of performing the sampling and the measurements in two different physical locations, since the sampled cells can be easily frozen, and transferred to the analytical laboratory, which can be of great value in multidisciplinary collaborations and experimental setups spanning different locations. Despite their utility and flexibility, offline microsampling methods share some limitations. Owing to their inherent properties, they cannot be used in real-time measurements on subcellular, or single cell level. Furthermore, they often suffer from low throughput, and need highly skilled operators for single cell manipulation and sampling. To address these challenges, several online microsampling techniques were developed, which mitigate some of the limitations of the offline techniques. In the next section, some of these techniques will be highlighted, as well as their advantages, and potential limitations.
2.3Online microsampling techniques
Online microsampling techniques integrate single or subcellular sampling, treatment, and MS analysis in one platform. This is especially useful in experimental setups where live, real-time, measurements are required. The single-probe [23] and its successor, the T-probe [24], both pioneered by Yang’s group are two notable examples of such approach. In the single-probe method, the sampling capillary has dual channels, in which the extraction solvent flows from one, to the other by means of a liquid junction at the very tip of the capillary. When the tip of the capillary touches the cell of interest, the extraction process is initiated. The analytes are transported in one of the channels to be introduced to the MS instrument via nano-ESI. The design was simplified further and the dual channel sampling capillary was replaced by a single channel, where the sample is aspirated through, and mixed with the extraction solvent in a T junction, prior to nano-ESI-MS analysis. The T-probe was successfully used to profile the metabolic differences between drug treated and non-treated individual HeLa cells. They further improved upon the T-probe design in order to perform in situ, real time lysis of floating single living cells [25]. The innovative aspects of this improved design are that it can be applied to non-adherent cells, unlike the previous design, as well as it provides in-capillary lysis for enhanced metabolites extraction (Fig. 3). The redesigned device consists of three parts, a sampling probe, a capillary containing lysis solution (e.g., acetonitrile), and a nano-ESI emitter, where subsequent ionization and MS measurements take place following cell lysis. This improvement allowed for the rapid and online lysis of floating cells without sample loss and then, immediately analyzed via MS. Differences in the metabolic profile between treated (using anticancer drug, irinotecan) and non-treated colon cancer cells (HCT-116) was observed. In particular, significant changes in lipid metabolism and composition were linked to the drug treatment.
One common limitation associated with most of the current microsampling-based single-cell metabolomics approaches is that the metabolites cannot be fully identified nor quantified. Undeniably, there are several hurdles in quantifying intracellular metabolites in single cells. To address this issue, internal electrode capillary pressure probe electrospray ionization MS (IEC-PPESI-MS) [26] has been developed by Nakashima et al. In addition to the use of pressure probe, the incorporation of IEC and ionic liquid mixture in the capillary notably improved the sensitivity and spatial resolution in sampling. The developed tip is directly inserted into the single cells and then, the capillary is depressurized for sampling (Fig. 4a). The sampled cell ingredients are directly electrosprayed for MS analysis (Fig. 4b). The quantitation of an analyte of interest takes place based on the microscopic images of the tip for volume calculation. The authors used the developed system to study the metabolic profile of single stalk and glandular cells of intact trichomes. The study revealed distinct differences in the metabolite composition between the two adjacent cell types as well as differences across trichome types, in particular, in flavonoids and acyl sugars compositions.
As previously mentioned, in online microsampling techniques, all the steps of the analysis are usually integrated into one system. This integration allows for in-situ real-time sampling and analysis of cells, with immediate feedback from the analytical instrument of choice, which is difficult in offline setups. They are also typically more suited for integration with other separation techniques such as capillary electrophoresis. Moreover, the probes used in online setups are usually more resilient, which makes them more suitable for sampling of higher density matrices, such as cells embedded in tissues [27]. Finally, they are arguably easier to automate due to their integrated nature. However, online techniques share some of the limitations of their counterparts, mainly, the high skill requirements to operate and sample cells via 3D micromanipulation. They also typically involve more complicated setups, which make them more susceptible to capillary plugging issues, which is not as common in offline techniques, where the sampling capillaries are only used once per cell.
3.Applications
3.1Biological
Traditional bulk measurements are certainly useful. However, they are ill-suited for cases where single-cell or subcellular information is needed. In bulk measurements, all cell-specific data is lost during sample preparation, whereas single-cell metabolomics is uniquely suited for this task. One example is a medical plant, Catharanthus roseus (L.) G. Don, which produces vinblastine and vincristine, two terpenoid indole alkaloids (TIAs) that show anti-cancer activities. TIA’s metabolic pathway has already been revealed using traditional bulk measurements, however, intracellular distribution inside the cells could not be assayed by traditional measurements. Yamamoto et al., combined two MS techniques including imaging MS and LSC-MS to tackle this issue. First, imaging MS was applied to image longitudinal tissue sections of C. roseus, which revealed that TIAs are synthesized in idioblast and laticifer cells, in addition to epidermal cells, contrary to previous studies. LSC-MS was then used to confirm this data, together with a quantitative assay of the levels of several TIAs in different cell types [13].
Another study demonstrated the utility of integrating ion mobility MS with capillary microsampling for subcellular level measurements, performed by Zhang et al. Whereby, they successfully sequenced peptides from single identified Type 1 and Type 2 F group (Fgp) neurons from the Lymnaea stagnalis central nervous system as well as measuring the subcellular distribution of peptides between cytoplasm and the nucleus of the neurons [28]. Furthermore, they succeeded in identifying and sequencing 28-residue novel neuropeptide by tandem MS in a single Fgp neuron. This study highlights the potential role of microsampling based single-cell metabolomics techniques in detecting changes in the subcellular level that can help identify and localize novel peptides in rare cells that in turn can help answering fundamental questions in neuroscience. Another interesting platform that integrated patch-clamp with modified nano-ESI MS, termed induced nano-ESI (InESI) technique, was applied to study the metabolic profile of the intracellular components of a single neuron from mouse brain slices as well as monitoring its physiological changes and activities [29]. The study allowed for the rapid and sensitive identification of more than 50 cytoplasmic metabolites in a single living neuron. Furthermore, the study showcased differences in the intracellular levels of glutamine, glutamate, and gamma-aminobutyric acid, in different brain areas and neuron subtypes, thus, further highlighting the potential use of single cell metabolomics in the field of neuroscience.
3.2Pharmaceutical and drug discovery
Single-cell data can help shed light into the role played by cellular heterogeneity in cancer resistance to chemotherapy [30, 31], as well as reduce failure rates in phase II clinical trials of new promising drugs [32]. Fukano et al. showcased the cell-to-cell heterogeneity of drug metabolism in a stable cell-line population [18]. Ali et al. built up on this work by integrating LSC-MS with Raman spectroscopy where non-invasive measurements of single HepG2 cells were performed by Raman to attempt to detect the metabolic fingerprint of the cellular response to the anti-cancer drug, tamoxifen, and then LSC-MS was used on the same cells to obtain semi-quantitative data about tamoxifen and its metabolite (4-hydroxy tamoxifen) [17]. Raman spectroscopy succeeded in identifying and predicting tamoxifen induced hepatotoxicity. Its spectral fingerprint could identify the cellular heterogeneity in response to drug treatment from single-cell data. MS analysis of the same cells was additionally performed and showed significant heterogeneity in the drug abundance (151%RSD), which was even more severe in the case of its metabolite (238%RSD), suggesting diversity in cellular drug uptake, as well as its metabolism. These two analyses were shown to correlate with each other, indicating potential correlations between Raman spectral intensities with cellular capacity to metabolize the drug.
Figure 5.
Lipid class distribution in CTCs. Bar plot of the unique distribution of lipid classes across CTCs from gastric and colorectal cancer patients is shown. Reprinted from [15] under creative commons license.
![Lipid class distribution in CTCs. Bar plot of the unique distribution of lipid classes across CTCs from gastric and colorectal cancer patients is shown. Reprinted from [15] under creative commons license.](https://content.iospress.com:443/media/cbm/2022/33-4/cbm-33-4-cbm210184/cbm-33-cbm210184-g005.jpg)
Currently, drug response to a certain chemotherapy treatment is often determined after a few rounds of chemotherapy by monitoring the tumor mass using (molecular) imaging techniques [33]. However, recent evidence has shown that even two tumors originating from the same organ show different responses to drug treatment [34]. This means that occasionally, precious time is lost, where patients are subjected to ineffective, and relatively toxic treatments, thus, worsening their prognosis. This is complicated further by the fact that even within the tumor, cells exhibit different behavior in their response to chemotherapeutic agents due to intratumor heterogeneity [35]. Yang’s group attempted to circumvent this by combining single-cell analysis and machine learning to predict cancer resistance based on the metabolic phenotypes of drug resistant and drug responsive cells [36]. The same method was then used to predict chemotherapy-induced drug resistance based on the metabolic profiles of resistant, somewhat resistant, and responsive colon cancer HCT-116 cancer cells [37]. Single-cells exposed to 1
3.3Medical
The current gold standard in cancer diagnosis involves direct biopsy of the tumor mass, i.e., acquiring a physical sample of the tumor using a long needle. This process is often invasive, and not suitable for all cancer types, such as brain tumors and leukemia. Another technique, termed liquid biopsy, eschews traditional tissue biopsy and uses peripheral blood samples instead. It presents a non-invasive option to diagnose certain types of cancer, monitor cancer progression, and evaluate drug efficacy. One of the targets for liquid biopsy is circulating tumor cells (CTCs), i.e., cells that shed from the primary tumor and circulate through bloodstream. While their exact role in cancer pathology is not yet well known, they are thought to contribute to tumor metastasis and their presence usually correlates to poor prognosis. Recent studies depicted that CTCs represent the spatial and temporal heterogeneity of the primary tumor [39, 40]. Therefore, single cell analysis of CTCs is crucial to characterize tumor heterogeneity that will, in turn, help gain insights on the mechanisms and biology behind tumor metastasis and resistance. One limiting factor in CTC analysis is their natural scarcity (1–10 cells in 7.5 mLs of peripheral blood). Masujima group attempted to circumvent this by integrating LSC-MS to flow cytometry [16], where the metabolic profile of a single CTC obtained from a neuroblastoma patient has been depicted. Another approach used by Masujima’s group included a microfluidics-based technique to isolate and enrich single CTCs [15]. In their most recent work, single CTCs were isolated and enriched from gastric and colorectal cancer patients and subsequently analyzed by LSC-MS. The metabolomic differences between CTCs and lymphocytes (control cells) as well as those between CTCs obtained from different cancer types have been shown for the first time on the single cell level. Among the results presented in this study are new potential biomarkers, particularly, the specific distribution of lipid classes in each cancer types (Fig. 5), highlighting the emerging role of lipids in tumorigenesis and progression as well as its potential role in cancer treatment.
Lipids have been recently proposed to play significant roles in tumors, e.g., altered lipid metabolism such as enhanced synthesis or uptake, altered energy metabolism by behaving as a source of energy, compositional changes in biological membranes, which as a result contribute to tumor development [41]. Recently, it has been depicted that tumor tissues have characteristic lipid compositions that are distinct from healthy tissue samples. Studies have also demonstrated that these lipid compositions differ depending on the stage of the tumor and its malignancy characteristics. In particular, cancer associated adipocytes (CAAs) were reported to play a role in tumor progression and development [42, 43, 44], whereas the mechanisms of how CAAs aid in tumorigenesis remain largely unknown. To help in the efforts in understanding these underlying molecular mechanisms, Phelps et al. investigated the TAGs profiles in CAAs and healthy adipocytes in breast tissues. The study showcased distinct differences in TAGs profiles between tumorous and healthy adipocytes, as well as significant heterogeneity in TAGs profiles between small and large lipid droplets in healthy adipocytes [45]. These results demonstrate that single cell analysis is prerequisite to account for cellular heterogeneity and highlight the potential of single-cell metabolomics in monitoring disease progression and biomarker discovery for early diagnosis. In another study, Zhang et al. demonstrated the important role of lipids in cancer development and progression. They characterized the metabolic profile differences between normal human astrocyte cells and glioblastoma cancer cells and found over 300 phospholipids unique to glioblastoma cancer cells [22]. Furthermore, the utilization of tandem mass spectrometry (MS/MS spectra) allowed for distinguishing between PC/PE isomers for the first time on the single cell level, in which the coexistence of PC (17:1) and PE (20:1) has been reported as a unique feature to cancer glioblastoma cells. This method is not limited to studying differentiating glioblastoma cells but can also be applied to other cancer cells, potentially aiding in the efforts for novel biomarker discovery and early disease detection.
In addition to CTCs and CAAs and their potential role in tumor development and progression, cancer stem cells (CSCs) were recently reported to largely contribute to therapy resistance leading to tumor relapse and metastasis [46]. CSCs are scarce subpopulation of cancer cells that are known for their ability to self-renew, differentiate and initiate tumors [47]. These heterogeneous cells are also known for their unique and hyperactive metabolism and their notable ability to reprogram host metabolism to survive harsh environments [48]. Thus, understanding metabolic processes unique to CSCs, will provide promising therapeutic targets and can potentially aid in preventing tumor relapse. However, one major limitation associated with CSCs studies, is that they typically represent a very small percentage of the tumor mass, making it difficult to obtain enough cells for subsequent analysis especially using traditional bulk approaches [49]. One study surmounted this by utilizing single-probe based mass spectrometry approach for the analysis of single CSCs obtained from colorectal cell line HCT-116 [50]. The study succeeded in detecting metabolic profile difference between CSCs and non-stem cancer cells (NSCCs), in particular, significant abundance in the tricarboxylic acid (TCA) cycle metabolites were reported in CSCs in comparison to NSCCs, which indicates significant difference in the energy production pathways between the two cell types. Additionally, CSCs were reported to have a higher abundance of unsaturated lipids and fatty acids than NSCCs, which are considered as key metabolic features of CSCs and corroborates with recent evidence that upregulated de novo fatty acid synthesis pathway and increased accumulation of lipid droplets in CSCs are essential for tumor development and differentiation [51].
4.Future perspectives
Despite recent efforts and developments in MS-based single-cell metabolomics, the field is still at its infancy. Current single-cell metabolomics techniques are facing several challenges, including low-throughput, inability to distinguish between technical and biological variabilities. Furthermore, low method sensitivity limits the detection and quantitation of low abundant metabolites or those exhibiting low ionization efficiency. Another limitation is the difficulty of including a biological/clinical relevance to the results which requires improvements in the software and databases used for metabolite identification. Since most MS techniques destroy the sample during measurements, real-time measurements are limited. An interesting approach to address this problem involves coupling single-cell MS techniques with other non-destructive analytical tools, such as optical spectroscopy-based techniques (e.g., Raman spectroscopy) [17, 52], microscopy-based techniques such as fluorescence microscopy [53] and patch-clamp techniques [29]. Finally, it is worth noting that there is no perfect analytical method, this is doubly true in the case of single-cell metabolomics. Instead, the experimental need of sensitivity, throughput, metabolic coverage, and accessibility usually dictates the most suitable single-cell analysis technique.
Acknowledgments
YA and AM acknowledge the support by the Leiden University Fund (W19340-5-EML) and Netherlands Organization for Scientific Research (NWA.1228.191. 329). This work was supported by an intramural Grant-in-Aid from the RIKEN Center for Biosystems Dynamics Research (to YS).
Author contributions
Conception: YA, AA, KM, AM, YS
Preparation of the manuscript: YA, AA
Revision for important intellectual content: YA, AM, YS
Supervision: AM, YS
References
[1] | T.M.J. Evers et al., Deciphering metabolic heterogeneity by single-cell analysis, Analytical Chemistry 91: (21) ((2019) ), 13314–23. |
[2] | L. Zhang and A. Vertes, Single-cell mass spectrometry approaches to explore cellular heterogeneity, Angewandte Chemie International Edition 57: (17) ((2018) ), 4466–77. |
[3] | A. Ali et al., Single-cell metabolomics by mass spectrometry: Advances, challenges, and future applications, Trends in Analytical Chemistry 120: ((2019) ), 115436. |
[4] | S. Emara, S. Amer, A. Ali, Y. Abouleila, A. Oga and T. Masujima, Single-cell metabolomics, in: Metabolomics: From Fundamentals to Clinical Applications, A. Sussulini, ed., Cham: Springer, (2017) , pp. 323–43. |
[5] | S.J. Altschuler and L.F. Wu, Cellular heterogeneity: do differences make a difference? Cell 141: (4) ((2010) ), 559–63. |
[6] | P. Hu, W. Zhang, H. Xin and G. Deng, Single Cell Isolation and Analysis, Frontiers in Cell and Developmental Biology 4: ((2016) ), 116. |
[7] | A. Gross, J. Schoendube, S. Zimmermann, M. Steeb, R. Zengerle and P. Koltay, Technologies for single-cell isolation, International Journal of Molecular Sciences 16: (8) ((2015) ), 16897–919. |
[8] | M. Shamir, Y. Bar-On, R. Phillips and R. Milo, SnapShot: Timescales in cell biology, Cell 164: (6) ((2016) ), 1302–1302. |
[9] | R. Vijay Kapoore, R. Coyle, C.A. Staton, N.J. Brown and S. Vaidyanathan, Influence of washing and quenching in profiling the metabolome of adherent mammalian cells: a case study with the metastatic breast cancer cell line MDA-MB-231, Analyst 142: (11) ((2017) ), 2038–49. |
[10] | F. Vella, Molecular biology of the cell (third edition): By B. Alberts, D. Bray, J. Lewis, M. Raff, K. Roberts and J.D. Watson, Garland Publishing, New York and London. (1994) , p. 1361. |
[11] | T. Fujii et al., Direct metabolomics for plant cells by live single-cell mass spectrometry, Nature Protocols 10: (9) ((2015) ), 1445–56. |
[12] | A. Ali et al., Quantitative live single-cell mass spectrometry with spatial evaluation by three-dimensional holographic and tomographic laser microscopy, Analytical Sciences 32: (2) ((2016) ), 125–7. |
[13] | K. Yamamoto et al., Cell-specific localization of alkaloids in Catharanthus roseus stem tissue measured with Imaging MS and Single-cell MS, Proceedings of the National Academy of Sciences of the United States of America 113: (14) ((2016) ), 3891–6. |
[14] | S. Enya et al., Dual roles of glutathione in ecdysone biosynthesis and antioxidant function during larval development in Drosophila, Genetics 207: (4) ((2017) ), 1519–32. |
[15] | Y. Abouleila et al., Live single cell mass spectrometry reveals cancer-specific metabolic profiles of circulating tumor cells, Cancer Science 110: (2) ((2019) ), 697–706. |
[16] | E. Hiyama et al., Direct lipido-metabolomics of single floating cells for analysis of circulating tumor cells by live single-cell mass spectrometry, Analytical Sciences 31: (12) ((2015) ), 1215–7. |
[17] | A. Ali et al., Single-cell screening of tamoxifen abundance and effect using mass spectrometry and raman-spectroscopy, Analytical Chemistry 91: (4) ((2019) ), 2710–8. |
[18] | Y. Fukano, N. Tsuyama, H. Mizuno, S. Date, M. Takano and T. Masujima, Drug metabolite heterogeneity in cultured single cells profiled by pico-trapping direct mass spectrometry, Nanomedicine 7: (9) ((2012) ), 1365–74. |
[19] | L. Zhang, L.L. Allworth and A. Vertes, Identification of Metabolites in Single Cells by Ion Mobility Separation and Mass Spectrometry, In: Single Cell Metabolism: Methods and Protocols, B. Shrestha, ed., Springer, New York, (2020) , pp. 9–18. |
[20] | M. Phelps, J. Hamilton and G.F. Verbeck, Nanomanipulation-coupled nanospray mass spectrometry as an approach for single cell analysis, Review of Scientific Instruments 85: (12) ((2014) ), 124101. |
[21] | M.S. Phelps and G.F. Verbeck, Analysis of Lipids in Single Cells and Organelles Using Nanomanipulation-Coupled Mass Spectrometry, In: Single Cell Metabolism: Methods and Protocols, B. Shrestha, ed., Springer, New York, (2020) , pp. 19–30. |
[22] | X.C. Zhang et al., Combination of droplet extraction and Pico-ESI-MS allows the identification of metabolites from single cancer cells, Analytical Chemistry 90: (16) ((2018) ), 9897–903. |
[23] | N. Pan, W. Rao, N.R. Kothapalli, R. Liu, A.W.G. Burgett and Z. Yang, The single-probe: A miniaturized multifunctional device for single cell mass spectrometry analysis, Analytical Chemistry 86: (19) ((2014) ), 9376–80. |
[24] | R. Liu, N. Pan, Y. Zhu and Z. Yang, T-probe: An integrated microscale device for online in situ single cell analysis and metabolic profiling using massspectrometry, Analytical Chemistry 90: (18) ((2018) ), 11078–85. |
[25] | Y. Zhu, R. Liu, and Z. Yang, Redesigning the T-probe for mass spectrometry analysis of online lysis of non-adherent single cells, Analytica Chimica Acta 1084: ((2019) ), 53–9. |
[26] | T. Nakashima, H. Wada, S. Morita, R. Erra-Balsells, K. Hiraoka and H. Nonami, Single-cell metabolite profiling of stalk and glandular cells of intact trichomes with internal electrode capillary pressure probe electrospray ionization mass spectrometry, Analytical Chemistry 88: (6) ((2016) ), 3049–57. |
[27] | W. Rao, N. Pan and Z. Yang, High resolution tissue imaging using the single-probe mass spectrometry under ambient conditions, Journal of the American Society for Mass Spectrometry 26: (6) ((2015) ), 986–93. |
[28] | L. Zhang et al., Subcellular peptide localization in single identified neurons by capillary microsampling mass spectrometry, Scientific Reports 8: (1) ((2018) ), 12227. |
[29] | H. Zhu, G. Zou, N. Wang, M. Zhuang, W. Xion and G. Huang, Single-neuron identification of chemical constituents, physiological changes, and metabolism using mass spectrometry, Proceedings of the National Academy of Sciences of the United States of America 114: (10) ((2017) ), 2586–91. |
[30] | D.A. Kessler, R.H Austin and H. Levine, Resistance to chemotherapy: Patient variability and cellular heterogeneit, Cancer Research 74: (17) ((2014) ), 4663–70. |
[31] | M.D. Brooks, M.L. Burness and M.S. Wicha, Therapeutic implications of cellular heterogeneity and plasticity in breast cancer, Cell Stem Cell 17: (3) ((2015) ), 260–71. |
[32] | M.E. Bunnage, E.L.P. Chekler and L.H. Jones, Target validation using chemical probes, Nature Chemical Biology 9: (4) ((2013) ), 195–9. |
[33] | T.H. Lippert, H-J. Ruoff and M. Volm, Current status of methods to assess cancer drug resistance, International Journal of Medical Sciences 8: (3) ((2011) ), 245–53. |
[34] | M. Cusnir and L. Cavalcante, Inter-tumor heterogeneity, Human Vaccines & Immunotherapeutics 8: (8) ((2012) ), 1143–5. |
[35] | B. Zhao, J.R. Pritchard, D.A. Lauffenburger and M.T. Hemann, Addressing genetic tumor heterogeneity through computationally predictive combination therapy, Cancer Discovery 4: (2) ((2014) ), 166–74. |
[36] | R. Liu, G. Zhang and Z. Yang, Towards rapid prediction of drug-resistant cancer cell phenotypes: single cell mass spectrometry combined with machine learning, Chemical Communications 55: (5) ((2019) ), 616–9. |
[37] | R. Liu, M. Sun, G. Zhang, Y. Lan and Z. Yang, Towards early monitoring of chemotherapy-induced drug resistance based on single cell metabolomics: Combining single-probe mass spectrometry with machine learning, Analytica Chimica Acta 1092: ((2019) ), 42–8. |
[38] | N. Pan et al., Quantification of drug molecules in live single cells using the single-probe mass spectrometry technique, Analytical Chemistry 91: (14) ((2019) ), 9018–24. |
[39] | M. Tellez-Gabriel, M.F. Heymann and D. Heymann, Circulating tumor cells as a tool for assessing tumor heterogeneity, Theranostics 9: (16) ((2019) ), 4580–94. |
[40] | M. Tellez-Gabriel, D. Cochonneau, M. Cadé, C. Jubelin, M.F. Heymann and D. Heymann, Circulating tumor cell-derived pre-clinical models for personalized medicine, Cancers 11: (1) ((2019) ), 19. |
[41] | L.M. Butler et al., Lipids and cancer: Emerging roles in pathogenesis, diagnosis and therapeutic intervention, Advanced Drug Delivery Reviews 159: ((2020) ), 245–93. |
[42] | Q. Wu, B. Li, Z. Li, J. Li, S. Sun and S. Sun, Cancer-associated adipocytes: key players in breast cancer progression, Journal of Hematology & Oncology 12: (1) ((2019) ), 95. |
[43] | C. Zhao et al., Cancer-associated adipocytes: emerging supporters in breast cancer, Journal of Experimental & Clinical Cancer Research 39: (1) ((2020) ), 156. |
[44] | G. Cantini, A. Di Franco, M. Mannelli, A. Scime, M. Maggi and M. Luconi, The role of metabolic changes in shaping the fate of cancer-associated adipose stem cells, Frontiers in Cell and Developmental Biology 8: ((2020) ), 332. |
[45] | M.S. Phelps and G.F. Verbeck, A lipidomics demonstration of the importance of single cell analysis, Analytical Methods 7: ((2015) ), 3668–70. |
[46] | D.A. Lawson et al., Single-cell analysis reveals a stem-cell program in human metastatic breast cancer cells, Nature 526: (7571) ((2015) ), 131–5. |
[47] | Z. Yu, T.G. Pestell, M.P. Lisanti and R.G. Pestell, Cancer Stem Cells, The International Journal of Biochemistry & Cell Biology 44: (12) ((2012) ), 2144–51. |
[48] | U.P Yadav et al., Metabolic adaptations in cancer stem cells, Frontiers in Oncology 10: ((2020) ), 1010. |
[49] | B. Bao, A. Ahmad, A.S. Azmi, S. Ali and F.H. Sarkar, Overview of Cancer Stem Cells (CSCs) and mechanisms of their regulation: implications for cancer therapy, Current Protocols in Pharmacology 61: (1) ((2013) ), 1–14. |
[50] | M. Sun and Z. Yang, Metabolomic studies of live single cancer stem cells using mass spectrometry, Analytical Chemistry 91: (3) ((2019) ), 2384–91. |
[51] | L. Tirinato et al., An overview of lipid droplets in cancer and cancer stem cells, Stem Cells International 2017: ((2017) ), 1656053. |
[52] | E.J. Lanni et al., Correlated Imaging with C 60 -SIMS and Confocal Raman Microscopy: Visualization of cell-scale molecular distributions in bacterial biofilms, Analytical Chemistry 86: (21) ((2014) ), 10885–91. |
[53] | Q. Li et al., Multicolor fluorescence detection-based microfluidic device for single-cell metabolomics: Simultaneous quantitation of multiple small molecules in primary liver cells, Analytical Chemistry 86: (21) ((2014) ), 10885–91. |