Comorbidity Scores and Machine Learning Methods Can Improve Risk Assessment in Radical Cystectomy for Bladder Cancer
Abstract
BACKGROUND:
Pre-operative risk assessment in radical cystectomy (RC) is an ongoing challenge especially in elderly patients.
OBJECTIVE:
To evaluate the ability of comorbidity indices and their combination with clinical parameters in machine learning models to predict mortality and morbidity after RC.
METHODS:
In 392 patients who underwent open RC, complication and mortality rates were reported. The predictive values of the age-adjusted Charlson Comorbidity index (aCCI), the Elixhauser Index (EI), the Physical Status Classification System (ASA) and Gagne’s combined comorbidity Index (GCI) were evaluated using regression analyses. Various machine learning models (Gaussian naïve bayes, logistic regression, neural net, decision tree, random forest) were additionally investigated.
RESULTS:
The aCCI, ASA and GCI showed significant results for the prediction of complications (χ2 = 8.8, p < 0.01, χ2 = 15.7, p < 0.01 and χ2 = 4.6, p = 0.03) and mortality (χ2 = 21.1, p < 0.01, χ2 = 25.8, p < 0.01 and χ2 = 2.4, p = 0.04) after RC while the EI showed no significant prediction. However, areas under receiver characteristic curves (AUROCs) revealed good performance only for the prediction of mortality by the aCCI and ASA (0.81 and 0.78, CGI 0.63) while the prediction of complications was poor (aCCI 0.6, ASA 0.63, CGI 0.58). The combination of ASA, age, body mass index and sex in machine learning models showed a better prediction. Gaussian naïve bayes (0.79) and logistic regression (0.76) showed the best performance using a hold-out test set.
CONCLUSIONS:
The ASA and aCCI show good prediction of mortality after RC but fail predicting complications accurately. Here, the combination of comorbidity indices and clinical parameters in machine learning models seems promising.
INTRODUCTION
In 2020, more than 500,000 patients were diagnosed with bladder cancer and > 200,000 bladder cancer related deaths worldwide were reported [1]. For muscle-invasive bladder cancer (MIBC), (neo-)adjuvant chemotherapy and radical cystectomy (RC) is recommended as the curative therapy of choice in non-metastatic patients [2]. The operative technique of RC has significantly improved over the last decades so that today a 30-day mortality rate < 3.5–5% can be achieved [3]. However, the 90-day complication rate still is reported to be higher than 50%, even in high-volume hospitals [4]. Due to these relevant risks despite surgical improvement, it is necessary to conduct a thorough preoperative risk evaluation. Factors adversely influencing the early postoperative morbidity and mortality seem to be comorbidity, age and lower hospital volume among others [3, 5].
Comorbidity indices such as the Charlson Comorbidity Index (CCI) or its age-adjusted version (aCCI) [6, 7], Elixhauser Index (EI) [8] and the American Society of Anesthesiology classification system (ASA) [9] have been successfully investigated for their prediction of mortality after RC. These scores proved to be significant predictors of mortality after radical cystectomy [10, 11]. Recently, Gagne et al developed the combined comorbidity index (GCI), a combination of the aCCI and van Walraven’s modification of the EI [12]. The GCI performed better than the individual indices in the prediction of short- and long-term mortality in general emergency patients > 65 years [13]. However, its use has not been validated in the prediction of postoperative mortality after RC.
For the preoperative risk evaluation of postoperative morbidity, validated instruments are lacking. The use of comorbidity indices to predict complications showed only limited predictive value [14]. Further, risk calculators from general surgery, such as the National Surgical Quality Improvement Program risk calculator, have not shown a sufficient performance in RC patients [15]. Thus, a thorough preoperative risk assessment remains a challenging task in clinical practice. This leads to an underutilization of RC with MIBC, especially in comorbid or elderly patients [16, 17].
Hence, we evaluated the ability of the GCI in comparison to the aCCI, EI and ASA in the prediction of postoperative mortality after RC at a German high-volume tertiary referral university hospital. Further, we evaluated the performance of the comorbidity indices and state-of-the-art machine learning (ML) models in predicting complications after RC.
MATERIAL AND METHODS
Study design
This study made use of a prospective RC database at a German high-volume tertiary referral university hospital. Patients were included in the analysis if they had histologically confirmed bladder cancer treated with open RC between January 2016 and August 2020. Patients undergoing RC due to non-oncological reasons or for palliative symptom control were excluded. This analysis was approved by the local ethics committee (2018-585N-MA), patients gave informed consent.
Comorbidity indices
We included the following comorbidity indices and classifications:
The scores of the aCCI, EI and CGI were calculated according to the information provided by the respective validation studies using the documented comorbidities in the patient files. In addition, the ASA was collected directly from the patient files as each patient gets preoperatively classified routinely by the anesthesiologist performing the anesthesia during RC.
Surgical technique
RC at our hospital involves the surgical removal of the urinary bladder and the prostate in men or the adnexa in women and a pelvic lymphadenectomy. Pelvic lymphadenectomy includes the resection of the lymph nodes of the obturator fossa, along the artery and vein iliaca externa and communis up to the aortic bifurcation while the lateral border of the lymph nodes to be resected is formed by the genitofemoral nerve.
The perioperative management of our patients is standardized using a multidisciplinary ERAS protocol, including the use of systemic opioid-sparing epidural analgesia, measures to prevent postoperative ileus, early postoperative feeding, early mobilization and other measures. Postoperatively, every patient is monitored on the intermediate care unit for at least one night. Transfusions are administered strictly in accordance with the Cross-Sectional Guidelines for Therapy with Blood Components and Plasma Derivatives, as transfusion has been shown to have a potential impact on oncological results [18]. The commonly applied urinary diversions are the ileum conduit, ileum neobladder, catheterizable pouch (usually ileum or ileocecal) and ureterocutaneostomy. Intraoperatively inserted ureteral stents are usually removed on the 10th postoperative day. The catheters of the neobladder and the pouch are usually removed after a cystogram ruled out leakage on the 14th and 21st postoperative day respectively.
Morbidity and mortality
Patients in the database were usually followed up annually. Additionally, regular vital status queries for all patients were conducted. To assess the postoperative morbidity, postoperative 30-day complications were extracted from the database and classified according to the Clavien-Dindo-classification (CDC) [19]. Of note, only the highest CDC was reported. In-hospital mortality, 30-day and 90-day mortality were reported. All deaths, including non-cancer or surgery-related deaths, were included.
Statistical analysis
Mean and standard deviation or median and interquartile range (IQR) were calculated for descriptive statistics. Univariable logistic regression analysis was performed for the respective indices to evaluate the performance in morbidity and mortality prediction. Wald’s Chi-Square (χ2) and p-value were reported for the goodness-of-fit and the area under the receivers operating characteristic curve (AUROC) to evaluate the predictive performance. Statistical significance was set at p < 0.05. These calculations were performed using JMP version 15.2.1 (SAS Institute Inc. 2019, NC, USA).
Machine Learning models to prognosticate the occurrence of severe complications
Gaussian Naïve Bayes (GNB), multivariable logistic regression (MLR), decision tree (DT), random forest (RF) and a neural net (NN) were evaluated in their ability to predict severe complications, defined as CDC IIIb-V. The best performing comorbidity index in univariable analysis, body mass index (BMI), age and sex were used as variables to predict severe complications since high BMI, older age and male sex have been shown to be possible risk factors for complications after RC and can be easily obtained preoperatively [20, 21]. The models were calculated in Python 3.7.7 (Python Software Foundation, Beaverton, OR, USA) extended with the libraries scikit-learn [22], fast.ai [23] and optuna [24]. The cohort was split into a training and test set in a 3:1 ratio. The complication group in the training set was upsampled using the resample function from scikit-learn so that an even distribution was achieved. The best parameters for GNB, MLR, DT and RF were calculated using GridSearchCV by scikit-learn in a 10-fold cross-validation. For the NN, fast.ai was used for the implementation and the optuna framework to find the best net architecture using a learning rate of 0.001 and training for 6 epochs. The models performing best on the training set were then evaluated on the hold-out test set.
RESULTS
Patient characteristics
N = 424 patients were screened for inclusion of which n = 392 adult patients were included in the final analysis. N = 32 patients were excluded because the RC was performed for non-oncological reasons (n = 29), for palliative symptom control (n = 2) or because a partial cystectomy was performed (n = 1).
Detailed patient characteristics can be found in Table 1. The median aCCI was 3 (IQR 2 –4), median GCI was 0 (–1 –1) and median EI was 0 (0 –3) while n = 155 patients (40%) had an ASA of 3 or 4.
Table 1
Patient cohort
Variable | n |
Male, n (%) | 311 (79%) |
Age, median [years] (IQR) | 68.5 (61–76) |
BMI, median [kg/m2] (IQR) | 26.8 (23.88–29.85) |
ASA Classification≥3, n (%) | 155 (40%) |
Age-adjusted Charlson Comorbidity Index, median [score] (IQR) | 3 (2–4) |
Elixhauser Index (van Walraven), median [score] (IQR) | 0 (0–3) |
Combined comorbidity index, median [score] (IQR) | 0 (–1–1) |
Muscle invasive bladder cancer in TURB, n (%) | 264 (67%) |
Operating time, median [minutes] (IQR) | 222 (181.25–275.75) |
Intraoperative estimated blood loss, median [ml] (IQR) | 600 ml (450–1000) |
pT3 / pT4 tumor in RC, n (%) | 158 (40%) |
Resected lymph nodes, median [number] (IQR)* | 17 (12–23) |
Lymph node metastasis (N+), n (%) | 96 (24%) |
Non-urothelial cancer, n (%) | 8 (2%) |
squamous cell carcinoma | 3 (0.8%) |
neuroendocrine small cell carcinoma | 2 (0.5%) |
Adenocarcinoma | 1 (0.2%) |
sarcomatoid carcinoma | 1 (0.2%) |
bladder-infiltrating prostate | 1 (0.2%) |
carcinoma | |
Continent urinary diversion, n (%) | 180 (46%) |
*n = 15 patients did not receive bilateral lymphadenectomy because this had already been performed in a previous oncological operation.
Morbidity and mortality
17% (n = 67) of the patients experienced at least one severe complication, as illustrated in Table 2. 2.6% (n = 10) of the patients died during the postoperative hospital stay. Overall, the 30-day mortality was 1.8% (n = 7) and the 90-day mortality 4.8% (n = 19) respectively. The 90-day mortality included two patients who died from postoperatively diagnosed cerebral or liver metastasis.
Table 2
Severe 30-day complications and respective management
Variable | n (%) | |
30-day complications CDC IIIb-V and management | 67 (17%) | |
CDC IIIb | management | 37 (9.4%) |
Fascial dehiscence | Fascia suture | 22 |
Small bowel obstruction | Open laparotomy with adhesiolysis | 3 |
Wound dehiscence | VAC therapy | 2 |
Insufficient intestinal anastomosis | Open laparotomy with re-anastomosis | 2 |
Insufficient ureter-intestinal anastomosis | Open laparotomy with re-anastomosis | 2 |
Gastrointestinal perforation | Open laparotomy with colostomy | 3 |
Postoperative bleeding | Open laparotomy with hemostasis | 1 |
Herniated intervertebral disc | Discectomy | 1 |
Epididymitis with abscess | Epididymectomy | 1 |
CDC IVa | management | 15 (3.8%) |
NSTEMI | Coronary angiography, Intensive Care Unit (ICU) | 4 |
Severe pneumonia / exacerbated COPD | (Non-)invasive ventilation, ICU | 5 |
Acute kidney failure | Hemodialysis | 3 |
Ischemic stroke | Surveillance on ICU | 1 |
Insufficient ureter-intestinal anastomosis | Open laparotomy, ICU | 1 |
Bradyarrhythmia absoluta | Cardioversion, ICU | 1 |
CDC IVb | management | 5 (1.3%) |
Insufficient intestinal anastomosis and tachyarrhythmia absoluta | Open laparotomy and cardioversion, ICU | 1 |
Tachycardia bradycardia syndrome | Reanimation, ICU | 1 |
Bradyarrhythmia absoluta | Reanimation, ICU | 1 |
Postoperative cardiac arrest | Reanimation after open laparotomy, ICU | 1 |
Lung artery embolism with acute kidney failure | Anticoagulation, hemodialysis, ICU | 1 |
CDC V | 10 (2.6%) | |
Peritonitis with septic shock and multi organ failure | 3 | |
Colon ischemia with sepsis and multi organ failure | 1 | |
Intraabdominal abscess with septic shock | 1 | |
Pneumonia and acute sepsis (no invasive ventilation on patient’s request) | 1 | |
STEMI with cardiogenic shock | 1 | |
Aspiration pneumonia with septic shock and multiorgan failure | 1 | |
Urinoma with septic shock and diffuse intraabdominal bleeding | 1 | |
Cardiac arrest (unknown cause) | 1 |
Comorbidity indices to predict mortality and severe complications
The univariable logistic regression analysis showed significant results for the prediction of 90-day mortality for the ASA (χ2 = 25.8, p < 0.001), the aCCI (χ2 = 21.1, p < 0.001) and the GCI (χ2 = 2.4, p = 0.04). AUROCs demonstrated good performance for the ASA (0.77) and for the aCCI (0.81) while the CGI’s AUROC (0.63) revealed only moderate performance, as demonstrated in Fig. 1A. EI did not show a significant prediction (χ2 = 1.87, p = 0.17, AUROC = 0.58).
Fig. 1
ROC curves for the index prediction of 90-day mortality and severe 30-day complications. The figure shows the individual ROC curves and corresponding AUROCs of the four comorbidity indices for the respective predictions. A) Prediction of 90-day mortality by the four comorbidity indices in univariable logistic regression analysis. B) Prediction of severe 30-day complications (CDC IIIb-V) by the four comorbidity indices in univariable logistic regression analysis.
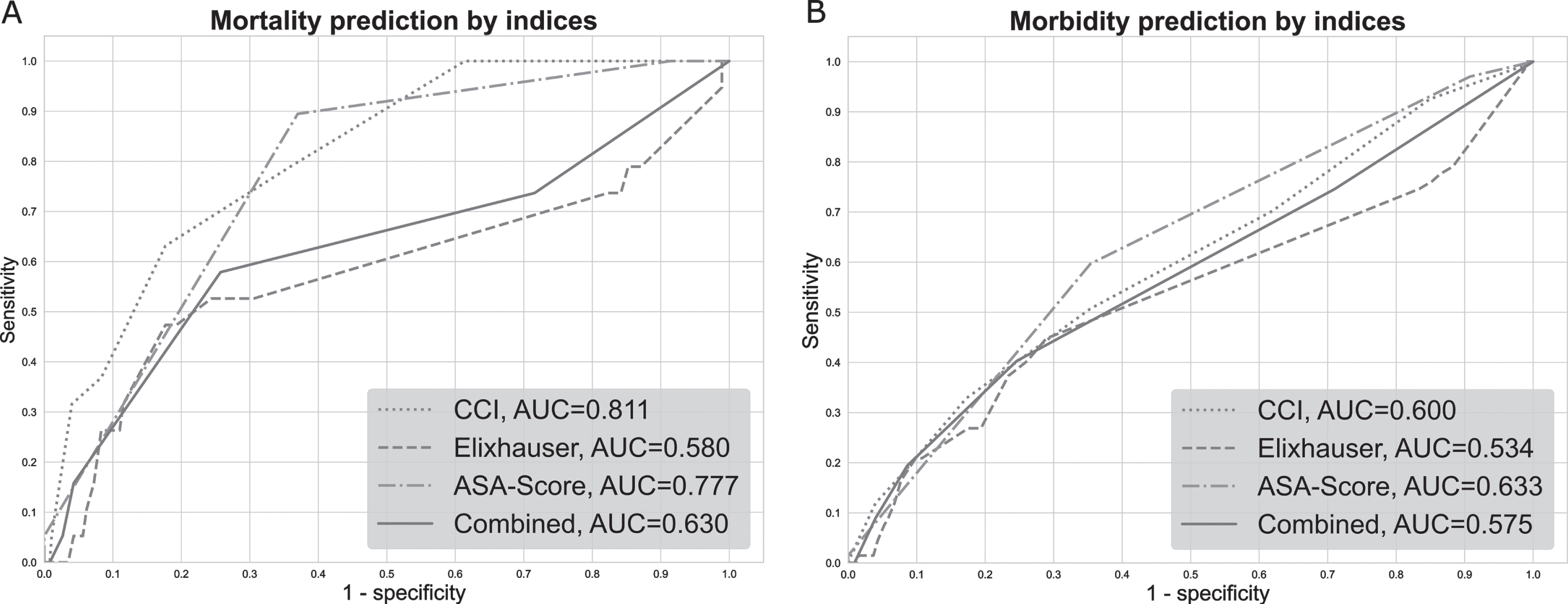
The prediction of severe 30-day complications showed significant results for the ASA (χ2 = 15.7, p < 0.001), the aCCI (χ2 = 8.8, p < 0.01) and the GCI (χ2 = 4.6, p = 0.03). However, AUROCs showed only moderate to poor performance, 0.63, 0.60 and 0.57, respectively, as illustrated in Fig. 1B. Again, EI was no significant predictor of severe 30-day complications (χ2 = 0.93, p = 0.3; AUROC = 0.53).
Machine Learning models to predict morbidity
Different ML models were developed to predict severe complications after RC. Due to the low number of deaths, the development of ML models to predict mortality was not considered expedient. The ASA as the best performing comorbidity index, body mass index, age and sex were used as variables. In 10-fold cross validation, all models showed an AUROC > 0.7 (Table S.1 in the supplementary). Interestingly, the performance of the NN, RF and DT dropped significantly when evaluated on the hold out test set (n = 98) while GNB and MLR showed slightly improved results. On the test set, the GNB showed the highest AUROC (0.79, χ2 = 7.3, p < 0.01) followed by the MLR (AUROC 0.76, χ2 = 9.0, p < 0.01), RF (AUROC 0.75, χ2 = 7.43, p < 0.01) and NN (AUROC 0.74, χ2 = 4.76, p = 0.03) as depicted in Fig. 2. The worst performance was seen for the DT (AUROC 0.58, χ2 = 0.89, p = 0.57). The hyperparameters chosen for the different models and the results on the training set are stated in Table S.1 in the supplementary.
Fig. 2
ROC curves for the prediction of 30-day severe complications by the machine learning models. The figure shows the individual ROC curves and corresponding AUROCs of the five ML models for the prediction of severe 30-day complications (CDC IIIb-V) on the hold-out test set.
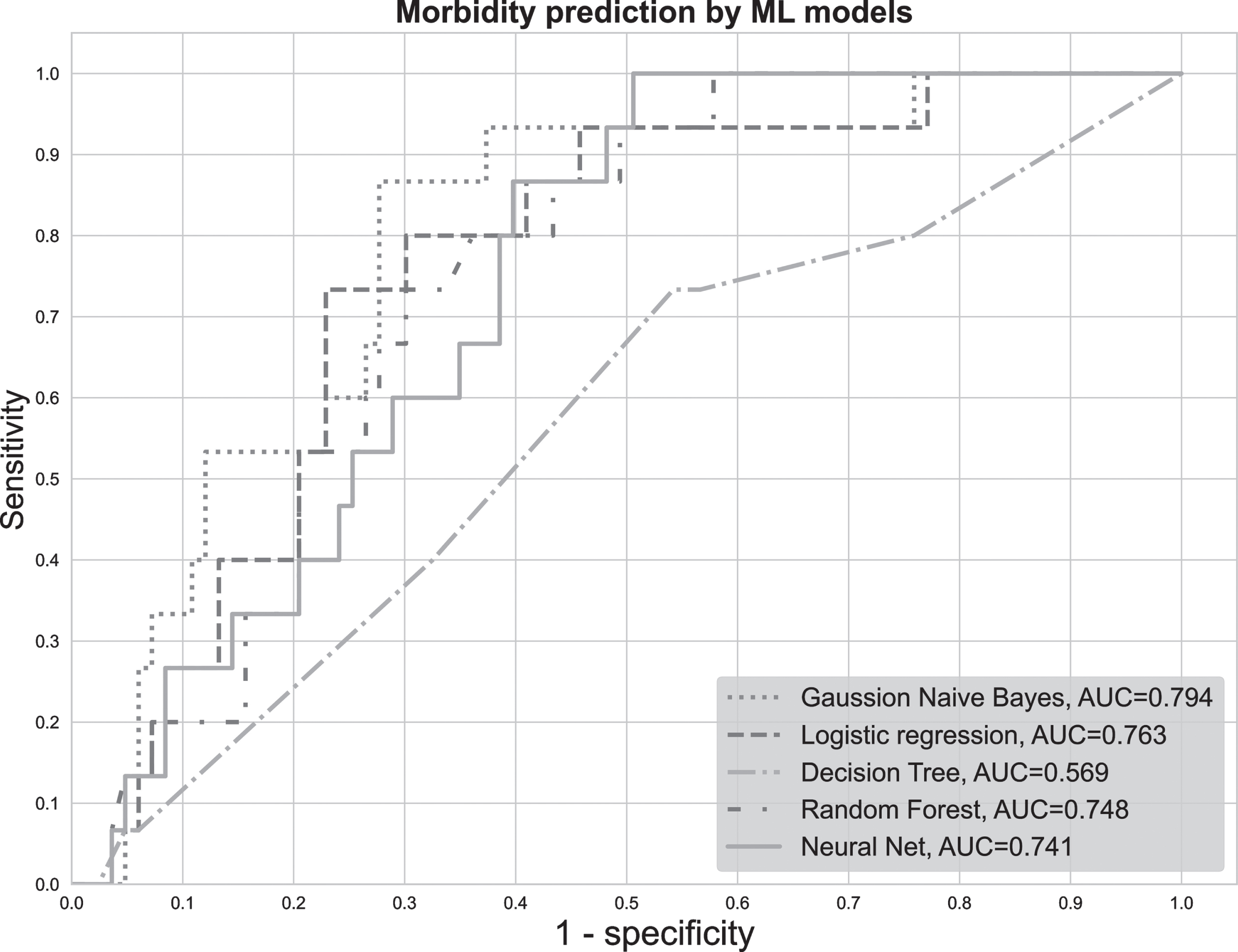
Interestingly, when replacing the comorbidity index with individual comorbidities as variables for the ML models, no outperformance was achieved.
DISCUSSION
The present study investigated the value of four comorbidity indices in their prediction of postoperative morbidity and mortality after RC. A good performance was shown for the prediction of mortality. However, prediction of complications was worse but can be improved using easily obtainable preoperative variables in state-of-the-art ML models.
The predictions of four different indices were evaluated in this study. The aCCI, EI and CGI are established indices that objectively indicate the severity of comorbidity by means of a sum score but are usually not collected in routine clinical practice. The ASA, on the other hand, is a simple and quick semi-subjective categorization score that is used almost ubiquitously. The indices showed their strength in predicting mortality. The in-hospital mortality in our cohort was 2.6% while the 30-day and 90-day mortality were 1.8% and 4.8% respectively. These results are in line with the mortality rate reported by other high-volume centers [3]. The best prediction of mortality was provided by the aCCI and the ASA followed by the GCI while the EI showed no significant prediction. A good performance of the CCI and aCCI in mortality prediction is well documented in the current literature. Froehner et al. reported an AUROC of 0.74 using the CCI to predict 90-day mortality in a larger cohort [25]. Prayer Galetti et al. showed that the aCCI as a significant predictor of mortality in elderly patients undergoing RC [11]. The AUROC of 0.81 in our analysis again underlines the predictive capability of the aCCI in RC candidates. Comparable results are also shown for the ASA. Our study revealed an AUROC of 0.77 which is concordant to recent studies investigating the predictive ability of the ASA [14]. Boorjian et al. found the ASA to be a strong risk factor of 90-day mortality after RC [10]. They additionally found the EI to be a risk factor. However, this could not be confirmed in our analysis. Regarding the CGI, a significant result was also shown, but the AUROC was worse than that of the ASA and aCCI. In our cohort, there was no advantage to be gained from using this index.
The index-based prediction of severe complications was also evaluated. Severe complications were defined as CDC≥IIIb. Although CDC IIIa complications are often included in the definition of severe complications, they were not included in our analysis. These complications, such as nephrostomy insertion for hydronephrosis, usually have a fundamentally different etiology and significantly less influence on the postoperative outcome than complications CDC≥IIIb. The preoperative prediction of CDC IIIb-V complication was therefore considered more clinically relevant. In the univariable analyses, the aCCI, ASA and GCI proved to be significant risk factors of severe complications which occurred in 17%. This again is in line with recent works. Roghman et al. showed a comparable complication rate [26]. Furthermore, they also identified the aCCI and ASA as risk factors for severe complications. However, the use of the indices as stand-alone models for the prognostication of complications seems to be not sufficient since AUROCs were < 0.70 for all indices in our study. A significant improvement could be achieved by introducing the ASA, sex, BMI and age as variables in different ML models. Specifically, the GNB, the MLR, the RF and the NN showed an AUROC above 0.70 on the hold-out test set. A similar approach was used by Taylor et al. [27]. They used ML models to predict adverse events after RC including multiple variables. They reported an AUROC of 0.67 for the prediction of serious complications. However, a direct comparison to our results seems difficult since a non-standardized definition of serious complications was used.
While the prognostication of complications after RC remains challenging, our results suggests that the combination of easily obtainable risk factors in combination with the ASA in ML models can provide a reasonable estimation of the risk of severe complications. Due to the monocenter design of our study, it can be assumed that an application of the presented models in other institutions would be associated with a drop in performance. Thus, for the development of a model that aims to be implemented in clinical practice, a multicenter cohort should be used. The most promising models were the GNB, the MLR, the RF and NN. All models showed stable results when evaluated on the hold-out test set while the DT model showed a drop in performance. Especially MLR has the advantage of being widely accepted, easy to interpret and implement in manually applicable risk models [28]. Therefore, it might be the most promising model for clinical implementation in this setting with a limited number of variables included. When a larger number of variables is to be analyzed, the more complex models such as GNB, NN and RF might show an advantage. The calculation of the risk score of these models is computer-based, so that an online application offers a good solution for easy access.
In recent years, frailty has emerged as another predictor of morbidity and mortality. In contrast to comorbidity indices, frailty focuses more on the actual physical performance and less on the pre-existing conditions [29]. Recent studies showed promising results for this concept in RC patients, especially since it can add additional value to the use of comorbidity scores [30, 31]. It will be exciting to see to what extent frailty establishes itself as a preoperative screening tool before RC or as a part of a multivariable model. As these scores usually need to be collected prospectively, an evaluation in this regard was not possible in our study but represents a highly interesting aspect for the further development and improvement of the models.
Of note, only patients undergoing open RC were included in our study. Overall, current studies suggest comparable mortality and severe complication rates for robotic-assisted RC [32]. However, blood loss, transfusion rate and length of stay have been reported to be lower when robotic-assisted RC is used, while open RC has significant shorter operating times [33]. The extent to which this reduction may improve postoperative morbidity and mortality in specific patient groups, such as cardiac high-risk patients, remains to be explored.
LIMITATIONS
First, although the data was mainly prospectively collected, naturally the power of the retrospective analysis of such database is limited. Second, due to the low absolute number of deaths, the mortality analyses must be confirmed in larger studies. Third, the ML models were not validated on an external dataset. To reduce the risk of overfitting, the cohort was split into a training and hold-out test set and results showed robustness of the model in our cohort. Still, only external validation can assess the generalizability of the models.
CONCLUSION
The ASA and the aCCI can help to assess the risk of mortality after RC with good accuracy. However, their prediction of postoperative severe complications is limited. State-of-the-art ML models show an improved prediction and thus might help to provide a clinically useful prognostication of severe complications.
ACKNOWLEDGMENTS
The authors have no acknowledgments.
FUNDING
The authors MC Kriegmair and MS Michel are financially supported by the German Cancer Aid (Deutsche Krebshilfe, project ID 73000189). The sponsor had no role in the design and conduct of the study; collection, management, analysis and interpretation of the data, preparation, review or approval of the manuscript, and decision to submit the manuscript for publication.
AUTHOR CONTRIBUTIONS
Frederik Wessels: Conceptualization, Methodology, Formal analysis, Investigation, Data Curation, Writing –Original Draft, Writing –Review & Editing, Visualization
Isabelle Bußhoff: Data Curation, Writing –Review & Editing
Sophia Adam: Data Curation, Writing –Review & Editing
Karl-Friedrich Kowalewski: Methodology, Writing –Review & Editing
Manuel Neuberger: Methodology, Writing –Review & Editing
Philipp Nuhn: Writing –Review & Editing, Resources
Maurice Stephan Michel: Resources, Writing –Review & Editing, Supervision
Maximilian C. Kriegmair: Conceptualization, Resources, Methodology, Writing –Original Draft, Writing –Review & Editing, Supervision, Funding acquisition
ETHICAL CONSIDERATIONS
This collection of patient data and this analysis was approved by the local ethical committee (2018-585N-MA) and was performed in accordance with the European General Data Protection Regulation. Patients gave informed consent to the collection and analysis of their patient data.
CONFLICTS OF INTEREST
Frderik Wessels has no conflict of interest to report.
Isabelle Bußhoff has no conflict of interest to report.
Sophia Adam has no conflict of interest to report.
Karl-Friedrich Kowalewski has no conflict of interest to report.
Manuel Neuberger has no conflict of interest to report.
Philipp Nuhn has no conflict of interest to report.
Maurice Stephan Michel is financially supported by the German Cancer Aid (Deutsche Krebshilfe, project ID 73000189). The sponsor had no role in the design and conduct of the study; collection, management, analysis and interpretation of the data, preparation, review or approval of the manuscript, and decision to submit the manuscript for publication.
Maximilian C. Kriegmair is financially supported by the German Cancer Aid (Deutsche Krebshilfe, project ID 73000189). The sponsor had no role in the design and conduct of the study; collection, management, analysis and interpretation of the data, preparation, review or approval of the manuscript, and decision to submit the manuscript for publication.
Availability of data and material
Data is available only internally due to its sensitive nature and its protection under the European General Data Protection Regulation. Sharing of data with external researchers might only be possible upon reasonable request and after obtaining ethics approval.
SUPPLEMENTARY MATERIAL
[1] The supplementary material is available in the electronic version of this article: https://dx.doi.org/10.3233/BLC-211640.
REFERENCES
[1] | Sung H , Ferlay J , Siegel RL , Laversanne M , Soerjomataram I , Jemal A , et al. Global cancer statistics 2020: GLOBOCAN estimates of incidence and mortality worldwide for 36 cancers in 185 countries. CA Cancer J Clin. 2021. |
[2] | Witjes JA , (Chair) HMB , Cathomas R , Compérat E , Cowan NC , Gakis G , Hernández V , Lorch A , Ribal (Vice-chair) MJ , Thalmann GN, , van der Heijden AG , Veskimäe E , Gakis G , EAU guidelines. Muscle-invasive and Metastatic Bladder Cancer. Edn. presented at the EAU Annual Congress Amsterdam 2020.2020. |
[3] | Lavallee LT , Schramm D , Witiuk K , Mallick R , Fergusson D , Morash C , et al. Peri-operative morbidity associated with radical cystectomy in a multicenter database of community and academic hospitals. PLoS One. (2014) ;9: (10):e111281. |
[4] | Diamantopoulos LN , Sekar RR , Holt SK , Khaki AR , Miller NJ , Gadzinski A , et al. Patterns and timing of perioperative blood transfusion and association with outcomes after radical cystectomy. Urologic oncology. 2021. |
[5] | Kim S , Kokorovic A , Sharma V , Boorjian S , Rendon RA , Bell D , et al. Factors predicting early mortality after radical cystectomy for urothelial carcinoma in a contemporary cohort of patients. Canadian Urological Association journal=Journal de l’Association des urologues du Canada. (2020) ;14: (12):E636–E42. |
[6] | Charlson ME , Pompei P , Ales KL , MacKenzie CR . A new method of classifying prognostic comorbidity in longitudinal studies: development and validation. J Chronic Dis. (1987) ;40: (5):373–83. |
[7] | Charlson M , Szatrowski TP , Peterson J , Gold J . Validation of a combined comorbidity index. J Clin Epidemiol. (1994) ;47: (11):1245–51. |
[8] | Elixhauser A , Steiner C , Harris DR , Coffey RM . Comorbidity measures for use with administrative data. Med Care. (1998) ;36: (1):8–27. |
[9] | Doyle DJG E. H. . American Society of Anesthesiologists Classification (ASA Class) [Updated 2017 Oct 6] StatPearls [Internet]:Treasure Island (FL): StatPearls Publishing; (2017) [Available from: https://www.ncbi.nlm.nih.gov/books/NBK441940/. |
[10] | Boorjian SA , Kim SP , Tollefson MK , Carrasco A , Cheville JC , Thompson RH , et al. Comparative performance of comorbidity indices for estimating perioperative and 5-year all cause mortality following radical cystectomy for bladder cancer. The Journal of Urology. (2013) ;190: (1):55–60. |
[11] | Prayer Galetti T , Soligo M , Morlacco A , Lami V , Nguyen AAL , Iafrate M , et al. Morbidity, mortality, and quality assessment following open radical cystectomy in elderly patients with bladder cancer. Aging Clin Exp Res. 2020. |
[12] | van Walraven C , Austin PC , Jennings A , Quan H , Forster AJ . A modification of the Elixhauser comorbidity measures into a point system for hospital death using administrative data. Med Care. (2009) ;47: (6):626–33. |
[13] | Gagne JJ , Glynn RJ , Avorn J , Levin R , Schneeweiss S . A combined comorbidity score predicted mortality in elderly patients better than existing scores. J Clin Epidemiol. (2011) ;64: (7):749–59. |
[14] | Williams SB , Kamat AM , Chamie K , Froehner M , Wirth MP , Wiklund PN , et al. Systematic Review of Comorbidity and Competing-risks Assessments for Bladder Cancer Patients. Eur Urol Oncol. (2018) ;1: (2):91–100. |
[15] | Golan S , Adamsky MA , Johnson SC , Barashi NS , Smith ZL , Rodriguez MV , et al. National Surgical Quality Improvement Program surgical risk calculator poorly predicts complications in patients undergoing radical cystectomy with urinary diversion. Urologic Oncology. (2018) ;36: (2):77–e1- e7. |
[16] | Williams SB , Huo J , Chamie K , Hu JC , Giordano SH , Hoffman KE , et al. Underutilization of Radical Cystectomy Among Patients Diagnosed with Clinical Stage T2 Muscle-invasive Bladder Cancer. European Urology Focus. (2017) ;3: (2-3):258–64. |
[17] | Gore JL , Litwin MS , Lai J , Yano EM , Madison R , Setodji C , et al. Use of radical cystectomy for patients with invasive bladder cancer. J Natl Cancer Inst. (2010) ;102: (11):802–11. |
[18] | Uysal D , Egen L , Grilli M , Wessels F , Lenhart M , Michel MS , et al. Impact of perioperative blood transfusions on oncologic outcomes after radical cystectomy: A systematic review and meta-analysis of comparative studies. Surgical Oncology. (2021) ;38: :101592. |
[19] | Dindo D , Demartines N , Clavien PA . Classification of surgical complications: a new proposal with evaluation in a cohort of patients and results of a survey. Ann Surg. (2004) ;240: (2):205–13. |
[20] | Bostrom PJ , Kossi J , Laato M , Nurmi M . Risk factors for mortality and morbidity related to radical cystectomy. BJU International. (2009) ;103: (2):191–6. |
[21] | Wessels F , Kriegmair MC , Oehme A , Rassweiler-Seyfried MC , Erben P , Oberneder R , et al. Radical cystectomy under continuous antiplatelet therapy with acetylsalicylic acid. European Journal of Surgical Oncology: The Journal of the European Society of Surgical Oncology and the British Association of Surgical Oncology. (2019) ;45: (7):1260–5. |
[22] | Pedregosa F , Varoquaux G , Gramfort A , Michel V , Thirion B , Grisel O , et al. Scikit-learn: Machine learning in Python. The Journal of Machine Learning Research.. (2011) ;12: :2825–30. |
[23] | Howard J , Gugger S . Fastai: A layered API for deep learning. Information. (2020) ;11: (2):108. |
[24] | Akiba T , Sano S , Yanase T , Ohta T , Koyama M Optuna: A Next-generation Hyperparameter Optimization Framework. Proceedings of the 25th ACMSIGKDD International Conference on Knowledge Discovery & Data Mining; Anchorage, AK, USA: Association for Computing Machinery; 2019. pp. 2623-31. |
[25] | Froehner M , Koch R , Heberling U , Borkowetz A , Hubler M , Novotny V , et al. Validation of a Questionnaire-Suitable Comorbidity Index in Patients Undergoing Radical Cystectomy. Urol Int. (2020) ;104: (7-8):567–72. |
[26] | Roghmann F , Trinh QD , Braun K , von Bodman C , Brock M , Noldus J , et al. Standardized assessment of complications in a contemporary series of European patients undergoing radical cystectomy. Int J Urol. (2014) ;21: (2):143–9. |
[27] | Taylor J , Meng X , Renson A , Smith AB , Wysock JS , Taneja SS , et al. Different models for prediction of radical cystectomy postoperative complications and care pathways. Therapeutic Advances in Urology. (2019) ;11: :1756287219875587. |
[28] | Sullivan LM , Massaro JM , D’Agostino RB Sr , Presentation of multivariate data for clinical use: The Framingham Study risk score functions. Stat Med. (2004) ;23: (10):1631–60. |
[29] | Kojima G , Iliffe S , Walters K . Frailty index as a predictor of mortality: a systematic review and meta-analysis. Age Ageing. (2018) ;47: (2):193–200. |
[30] | van der Vlies E , Los M , Stijns PEF , van Hengel M , Blaauw NMS , Bos WJW , et al. Preoperative frailty and outcome in patients undergoing radical cystectomy. BJU International. (2020) ;126: (3):388–95. |
[31] | Selvi I , Arik AI , Baydilli N , Basay MS , Basar H . Evaluation of comorbidity indices in determining the most suitable candidates for uro-oncological surgeries in elderly men. Cent European J Urol. (2021) ;74: (1):24–38. |
[32] | Parekh DJ , Reis IM , Castle EP , Gonzalgo ML , Woods ME , Svatek RS , et al. Robot-assisted radical cystectomy versus open radical cystectomy in patients with bladder cancer (RAZOR): an open-label, randomised, phase 3, non-inferiority trial. Lancet. (2018) ;391: (10139):2525–36. |
[33] | Rai BP , Bondad J , Vasdev N , Adshead J , Lane T , Ahmed K , et al. Robotic versus open radical cystectomy for bladder cancer in adults. The Cochrane database of systematic reviews. (2019) ;4: :CD011903. |