Ethical challenges in argumentation and dialogue in a healthcare context
Abstract
As the average age of the population increases, so too do the number of people living with chronic illnesses. With limited resources available, the development of dialogue-based e-health systems that provide justified general health advice offers a cost-effective solution to the management of chronic conditions. It is however imperative that such systems are responsible in their approach. We present in this paper two main challenges for the deployment of e-health systems, that have a particular relevance to dialogue and argumentation: collecting and handling health data, and trust. For both challenges, we look at specific issues therein, outlining their importance in general, and describing their relevance to dialogue and argumentation. Finally, we go on to propose six recommendations for handling these issues, towards addressing the main challenges themselves, that act both as general advice for dialogue and argumentation research in the e-health domain, and as a foundation for future work on this topic.
1.Introduction
Chronic conditions such as diabetes and chronic pain are now highly prevalent and placing a significant strain on health care resources [7,8,28,65]. This is further exacerbated by the increasing average age of the population [57], meaning more people are living longer with chronic conditions. With limits to health care professionals available, the development of e-health systems that provide general health advice, such as recommendations related to physical activity or diet, offer a cost-effective method of helping people manage their condition. There are however significant issues in developing such systems, ranging from user experience and engagement [50], to highly sensitive legal [4] and ethical [15] considerations. In particular, it is important that such advice-giving systems do not exceed what is legally and ethically permitted before they might be considered a medical device and thus subject to regulatory approval [39].
Dialogue and argumentation can play an important role in addressing these issues [20,31,48], especially in e-health systems designed around health coaching [30,52]. Advice and support that is based on sound models of reasoning and delivered through dialogue-based interaction can simultaneously deliver a high level of user experience and engagement (through, for example, mixed-initiative dialogues [53] with multiple agent actors providing the advice), while also ensuring that legal and ethical standards are adhered to. There are nevertheless certain challenges that must be overcome for this to be realised in practice; in tackling the latter in particular, a responsible approach to innovation is required to ensure that the use of argumentation and dialogue does not overstep the bounds of what is legally and ethically allowed.
Responsible Research & Innovation (RRI) is a process by which research and innovation activities become aligned with societal values, needs and expectations [58]. The concept of RRI first appeared in the EU’s 7th Framework Programme for Research and Development (FP7) and has gained in importance during the implementation of Horizon 2020. With this concept, the European Commission has sought to frame the question of how best to respond to a number of issues that have to do with the relationship between research and innovation (R&I) activities funded by the Commission and society at large. The concept was taken up by the European Council (in this case the gathering of the science and innovation ministers of the EU Member States) in its 2014 Rome Declaration, which called for “all stakeholders to act together” to achieve a more societally responsible approach to innovation [9]. It has also been adopted by national actors, including research councils in the Netherlands,11 Norway,22 and the UK.33
We should however be clear about how far the consensus on RRI goes. Firstly, it is important to observe that the concept of RRI is not meant to describe a new phenomenon; something that is already there and just needed to be properly conceptualised. Rather, RRI is an inherently normative concept linked to high-level research and innovation (R&I) policy, which sets out a goal for how the relationship between science and society ought to be. Secondly, all actors agree to emphasize that RRI should be thought of as a process by which R&I activities become aligned with societal values, needs and expectations [58].
We present in this paper two main challenges that were identified using an RRI process, each with specific issues that have a particular relevance to the use of dialogue and argumentation in the development of e-health systems aimed at delivering health advice: collecting and handling health data, incorporating privacy and informed consent and handling conflicts; and appropriate trust, incorporating fairness and explanation. The relationship between the main challenges and issues is shown in Fig. 1. For each issue in each main challenge, we outline what it is and why it is relevant to argumentation and dialogue. We then go on to present a series of recommendations aimed at handling these issues, towards addressing the main challenges themselves.
Fig. 1.
Relationship between main challenges and issues.
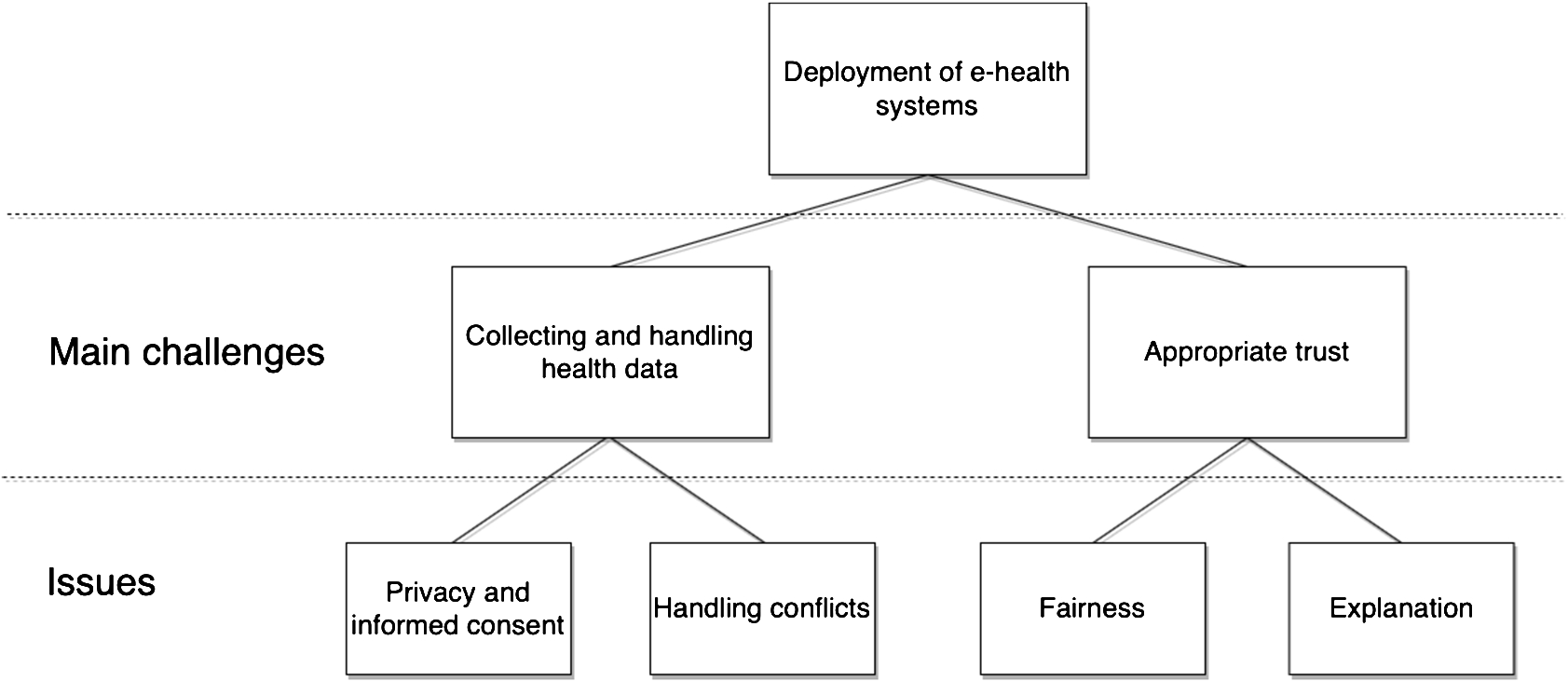
In Section 2 we place the work in context and describe the RRI process that was followed which led to the identification of the main challenges and issues; in Sections 3 and 4 we present the two main challenges of, respectively, collecting and handling health data, and trust; in Section 5 we provide our recommendations for handling these challenges; and in Section 6 we conclude the paper and provide a summary of our recommendations as directions for future work.
2.Methods
2.1.Context
Before describing the RRI process that identified the challenges and associated issues presented in this paper, we first briefly describe the context in which that process was carried out.
Council of Coaches44 (COUCH) is a project funded under the European Union’s Horizon-2020 programme,55 which aims to develop a virtual council that can assist users with life-changing diagnoses in achieving their health goals [41]. In doing so, it aims to answer the research question: How can we create a behaviour change support tool that takes into account the multi-dimensional issues of age-related impairments in a way that is fun and engaging in the long term? Our solution is to design and develop a virtual coaching concept based on multiple autonomous, embodied virtual coaches, each specialised in their own domain, that interact with each other and with the user to inform, motivate and discuss health and well-being related issues (such physical, cognitive, mental and social well-being). The individual coaches will listen to the user, ask questions, inform, discuss between themselves, jointly set personal goals and inspire the user to take control of his or her health. To be able to provide the user with a sufficient level of coaching, solutions should be able to cope with complex reasoning and argumentative structures, as the underlying theories and strategies applied in human-human coaching are often equally complex in nature.
2.2.RRI-process
RRI-issues is a concept first developed in the COUCH application process and also forms a conceptual contribution to practical RRI work in research projects at large. Together with the RRI-Vision, these issues serve as the core framework of the RRI approach described here. The main objective is to provide a practical approach, a terminology and a conceptual toolbox to flesh out and translate the abstract areas of importance defined by the European RRI-Framework into a practical methodology. By identifying areas of importance in a concrete project and making the consortium formally agree to keeping these issues under scrutiny throughout the project, the project working hypothesis dictates that this pact intentionally and unintentionally will help the consortium to keep its focus and make RRI an integrated and organic part of project results and everyday work ethics as such. The purpose of this process is to realise recommendations from the Responsible Industry project to accompany prototyping and innovation processes in ICT with dialogue and reflection on societal values as a means of operationalising responsible research and innovation in practice [56]. The agreed-upon issues and their rationales are then written into the founding RRI-Vision, which also doubles as an early project delivery. Methodologically, the workshopping process draws on Socio-Technical Integration Research (STIR) as developed by Fischer and others (see e.g. [17]).
In the case of Council of Coaches, the practical process leading to the RRI-Vision had the format of a structured workshopping process during which the Council of Coaches consortium members engaged in stakeholder dialogue and ethical reflection at the outset of formulating the RRI-issues. The workshopping process was designed and facilitated by the Danish Board of Technology66 (DBT); a non-technical research organisation specialised in participatory methods. In the consortium’s interactions with external stakeholders, the DBT played the role of an “honest broker” – a 3rd party go-between facilitating frank discussions between the technical members of the consortium and the viewpoints of external stakeholders.
The first step of the RRI process was to come to the agreement within the consortium about which societal responsibilities – that go beyond simple legal compliance – the consortium would want to make their concern during the project implementation. We defined our putative RRI-Vision as “an agreement between the project partners about what responsibilities arise from the ambitions of the project, who needs to bear those responsibilities, and how the project is going to ensure that they do” [40, p.9]. To prepare such an agreement, we conducted desktop research, an pilot external stakeholder dialogue workshop, and a consortium reflection workshop each of which contributed to the step-wise identification of the RRI issues pertinent to the project. Overall, we defined an RRI-issue as “an opportunity for adding societal value that also implies a risk of impacting society negatively” [ibid., p. 34]. What this definition implies is that a project that wishes to assume responsibility for the societal value of the research or innovation conducted in the project must become able to look sideways at the potential scientific advances and beneficial innovations at the core of the project’s ambition in order to reveal to see what harmful unintended consequences might follow from the project’s success. Precisely because researchers are no better or worse than anyone else at second-guessing their own motives and best laid plans, achieving such a sideways perspective on the project demands an interactive process involving stakeholders external to the project.
The steps taken in this first phase amount to a funnelling of the very broad challenge of “being responsible” down to a list of concrete challenges that the project can meaningfully attempt to solve during its implementation. A first step in this funnelling process was the production of a working document [Ibid.] introducing the policy background that has led the European Commission – the funder of the project – along with national funding agencies in several European countries to channel public demands for the sustainability and equity of public investments in research and innovation into a new discourse on responsibility. Our introduction sought to make clear that responsibility is not about each individual researcher bearing the weight of the world on their shoulders but rather about organising research and innovation in such a way that societal values are actively integrated in the steering of the project towards results and impacts. With this basic caveat in place, the working document went on to explore the potential meaningfulness of a range of tangentially related codes of ethics to the ambition of the project. At the particular juncture where this exercise was taking place, no particular ethical code or framework had been adopted by the industry to guide the development of virtual coaching apps. We therefore used as a reference point a patchwork of such codes, frameworks and research recommendations; e.g. for responsible ICT in general, for e-health in particular, for social robotics, and for the conduct of human coaches. Altogether, these provided us with a patchwork of attention points to help guide the consideration of RRI-issues in the Council of Coaches.
With this ethical patchwork in hand, we carefully selected 10 stakeholders for the first stakeholder workshop to brainstorm and discuss the project, its ambitions, and its societal implications. The invited stakeholder group was composed to include diverse interests and types of expertise, including representatives from a patient organisation, a consumer protection agency, and a regional health authority. From the consortium, besides facilitators from the DBT, representatives of the Coordinator team took part. After a thorough presentation of the project plan and long term ambitions, we asked the participants in pairs or groups of three to identify RRI-issues relevant to the project as seen from their particular professional or organisational vantage point. Each pair or group wrote down their reflections under the rubrics: “What is your issue?”, “Why is it important?” and “How might the project address it?”. At the end of the group session, which produced a total of seven suggested RRI issues, each group presented its issue(s), which were discussed in the plenary. The next day, we gathered the full consortium for a briefing on the inputs from the stakeholder group. Afterwards, two days were spent with the consortium during which the DBT organisation facilitated the “funnelling” process. Firstly, the patchwork of ethical standards were presented, and consortium members were divided into pairs or groups of three to brainstorm possible RRI issues on the basis of the patchwork. Secondly, the inputs from the stakeholders produced the day before were presented, followed by a plenary discussion. Thirdly, the consortium members (in pairs or threes) went through the same idea generation exercise as the stakeholders the day before, producing a bulk list of about 12–15 RRI-issues. This exercise was rounded off with a round of voting to prioritise the listed issues. The top four issues were selected as focus points for further reflection throughout the project: privacy and informed consent, handling conflict, keeping knowledge bases up to date, and appropriate trust. Furthermore, in a session of plenary discussion, responsibility for following up on each of these main RRI issues were assigned to specific work package leaders. A visual summary of the RRI process is provided in Fig. 2.
For the purposes of the present work, we categorise these issues into two main challenges: collecting and handling health data, and appropriate trust. The former consists of two of the issues (privacy and informed consent, and handling conflict), while the latter presents the fourth issue as a main challenge in its own right; this is because trust is a significant challenge in AI that belies several issues of its own, of which we focus on two: fairness and explanation.
Fig. 2.
Summary of the RRI process.
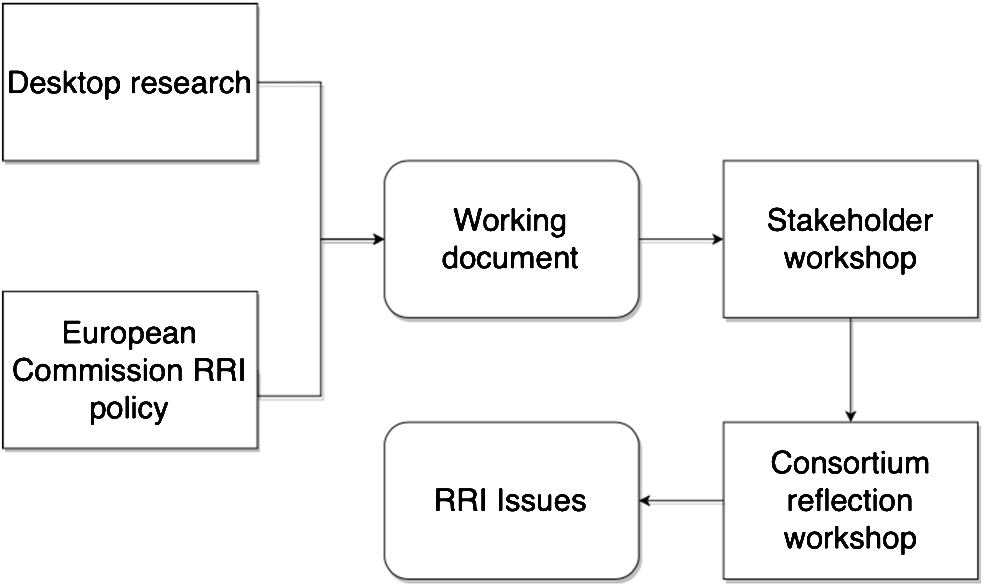
3.First main challenge: Collecting and handling health data
3.1.Background
For e-health solutions to function optimally, they must be able to collect and process multiple strands of personal and medical data. This presents several related issues, from user understanding to how their data is to be used, underpinned by legal and ethical expectations, to ensuring that new data is properly accommodated and conflicts are appropriately handled.
As part of the RRI process described in Section 2, these issues relevant to dialogue and argumentation were identified and described as:
(1) Privacy and informed consent: users of an e-health system must provide informed consent for their health data to be used, while also having certain expectations of privacy;
(2) Handling conflicts: in the face of new data that conflicts with existing data, a system must be capable of identifying how that conflict should be resolved to ensure no harm comes to the users;
In the remainder of this section, we expand on these issues and examine their relevance to dialogue and argumentation.
3.2.Privacy and informed consent
From a legal standpoint, effective handling of privacy and consent has become even more important in the European Union since the coming into force of the General Data Protection Regulations (GDPR) [10]. There are however moral obligations that both complement and, to an extent, conflict with the legal position. Collectors of personal and medical data are ethically bound to keep that data private; however, in the context of a semi-autonomous e-health system, we need to consider the extent to which data collected from the user can be shared with real medical professionals – for instance, if there are signs that the user potentially has a serious medical condition. It is therefore necessary to establish a careful balance between a user’s expectations of privacy, and ensuring that appropriate treatment is received. This is achieved through a careful consent-gathering process in which, amongst other aspects, the patient is fully informed as to what data will be shared and under what circumstances.
In a traditional medical context, where a physician requires a patient’s consent for some purpose, the gathering of consent is a process that has three distinct components [14]: disclosure, which is the provision of relevant information by the clinician and its comprehension by the patient; capacity, which is the patient’s ability to understand the information and appreciate the consequences of their decision; and voluntariness, which is the patient’s right to come to a decision freely without force, coercion or manipulation.
This traditional consent-gathering process is intrinsically dialogical: a clinician asks for consent for a specific purpose (disclosure), and the patient can then ask for further information, or accept. This has similarities to an information-seeking dialogue in the Walton & Krabbe typology [61], in terms of both the clinician gathering consent,77 and the patient asking for more details. It has been shown that information-seeking dialogues can be formalised for the purposes of agent-communication [45]; as such, it is possible in principle to develop a dialogue protocol or protocols to model consent-gathering in an e-health system.
In developing such a dialogue protocol, however, pitfalls involved in traditional consent-gathering should be avoided. Rubinelli & Schulz [46] observe that when doctors provide information in response to a patient query, they find themselves “arguing” in favour of a course of treatment. This breaks the principle of vountariness. Any dialogical process for consent-gathering in an e-health system must therefore be carefully designed such that it does not appear persuasive in response to a user’s query. Instead, it should provide reasons in the form of explanations, which we cover in more detail in Section 4.3.
3.3.Handling conflicts
Health care is a domain in which conflict will inevitable occur. Some conflicts are relatively trivial in nature and are easily resolved by the patient themselves, while others are much more significant and require deep medical knowledge to avoid causing harm. Consider for instance the difference between choosing what type of exercise to take, and choosing between two possible treatments for a life-threatening illness. The former is very much within the purview of e-health systems designed to offer general health advice, whereas the latter is not. Nevertheless, the possibility of a user providing information that creates a genuine medical-related conflict should not be discounted. Should such conflicts arise, the system must be capable of first identifying this more serious type of conflict, then handling it effectively and appropriately.
Conflict is of course widely-studied in argumentation theory and forms a cornerstone of models of dialogue. Our concern in the present work is not however with specific conflicts, but rather how we might categorise different types of conflict. Accurate identification of these types is essential for both realistic operation of the system, but more importantly patient safety. The three primary types of conflict we consider are system-resolved, user-resolved and professionally-resolved.
As the name suggests, system-resolved conflict will be resolved by the system itself, possibly without the user or anyone else being aware that a conflict even existed. Consider, for instance, a user sustaining a broken leg; this conflicts with being able to go for a run, but resolving that conflict is straightforward. In some cases, however, “playing-out” a conflict in front of the user can have advantages, such as where there are two equally beneficial but mutually-exclusive courses of action. For example, whether the user should spend some spare time by going for a swim, or preparing a healthy meal for dinner. This feeds into the process of user-resolved conflict.
User-resolved conflict, empowers the user to choose between conflicting advice or courses of action and is broadly similar to the concept of shared decision making (SDM) [12]. This allows them to take ownership of the decision, something which is an important part of coaching strategies such as goal-setting [35,52]. As with system-resolved conflict, all possible outcomes from the resolution process should not be harmful to the user in any way.
Finally, conflict should be professionally-resolved if a system should find itself holding data that creates a conflict between two or more pieces of medical advice (as opposed to general health advice). Neither the user nor the system should attempt to resolve such conflicts because there is no guarantee that the chosen outcome is correct, and as such could cause significant harm. The conflict should therefore be referred to at least one medical professional to make an effective decision on the matter.
4.Second main challenge: Appropriate trust
4.1.Background
Designing for appropriate trust is an increasingly important issue in AI and more widely in computing. Lee & See view trust as “the attitude that an agent will help achieve an individual’s goals in a situation characterized by uncertainty and vulnerability.” [34]. They argue that trust is a meaningful concept to describe human-machine interaction in both naturalistic and laboratory settings, and that humans respond socially to technology. Factors which influence human-human relationships, such as emotional and attitudinal factors, can also contribute to human-machine relationships. In some ways, our reactions to computers can be similar to our reactions to human collaborators. This work is consolidated in the Social Machines research programme within the Berners-Lee paradigm [24], in which combinations of people and computers are viewed as a single problem-solving entity. Of practical utility is Lee and See’s argument that we can measure trust consistently in human-machine relationships.
Designers of systems in the sharing economy have used findings in behavioural science to build and maintain trust between their users. For instance, the hospitality service Airbnb,88 which depends on building trust between people who had never met, uses techniques such as reputation (for both host and guest), introductions (with hints for what to say and appropriate length) and personal photos to form trust (eg, see [1,13,36]).
At the other end of the spectrum, early experiments with the 1966 computer “Rogerian therapist” Eliza [63] have shown that, for some people, too much trust in a system can be an issue. Eliza was built as a parody, intended to demonstrate the superficiality of communication between people and machines [38]; yet due to our tendency to anthropomorphise seemed to demonstrate quite the opposite, with users attributing far greater understanding, intelligence and empathy than was in the system, inducing “powerful delusional thinking in quite normal people” [63].
Too much trust can lead to over-reliance or complacency (greater than that warranted by the system’s functionality, reliability or robustness). We see this in over-trust of autopilot and automated navigation systems. This occurred in the Air Inter Flight 148 Airbus A320 crash, in which pilots, trusting the ability of the autopilot, failed to intervene and take manual control even as the autopilot crashed the plane, killing 87 of the 96 people on board [54]. Similarly, failure of a crew to intervene in a malfunctioning automated navigation system, in which the Royal Majesty cruise ship drifted off course for 24 hours and then ran aground [33] (both cases reported in lee:04).
Shneiderman points out that new social traditions are needed to enhance trust in people-machine settings, to complement conventional ways of establishing trust between people, such as eye contact and handshakes [49]. He makes the point that “strategic trust is difficult to generate, shaken easily, and once shaken extremely difficult to rebuild.” [Ibid.] and offers guidelines for gaining initial trust and enhancing and maintaining it. His guidelines for ensuring trust, which are pertinent to new users are as follows: disclosing patterns of past performance; providing references from past and current users; using third party certifications; and making it easy to locate, read, and enforce policies involving privacy and security. Guidelines for enhancing trust involve clarifying each participant’s responsibilities; providing clear guarantees with compensation; and supporting dispute resolution and mediation services.
“Trusted AI”. IBM have built a model of AI and trust [5,27], in which they deconstruct trust into four “fundamental pillars”:
(1) Robustness: AI systems should be secure and reliable. This can be achieved by exposing and fixing their vulnerabilities.
(2) Fairness: AI systems should not take on and amplify our biases. This can be achieved by new methodologies which detect and mitigate bias through the life cycle of AI applications
(3) Explainability: AI systems should be transparent about how they have arrived at an outcome. This can be achieved by researching interpretability of models and their output, training for interpretable models and visualization of information flow within models, and teaching explanations.
(4) Lineage: AI systems should ensure all their components and events are trackable. This can be achieved by including details of their development, deployment, and maintenance so they can be audited throughout their lifecycle.
Of these, Fairness and Explainability are particularly relevant to argumentation and healthcare: Fairness is related to all work in AI and explanations are very close to argunents, both syntactically and semantically. We expand on these in the context of argumentation and dialogue below in Sections 4.2 and 4.3.
4.2.Fairness
The fairness criterion concerns the danger of taking on and amplifying our biases, often unconsciously. In a review of literature and research practices in AI around issues such as gender, race and class, West et al. [64] conclude that there is a diversity crisis in AI. This is exemplified in systems which detect, classify, and predict: for instance, image recognition services which make offensive classifications of minorities; object-recognition systems which perform poorly on objects in countries with low income levels; or hiring tools for ranking job candidates which discriminate against females. Our increasing incorporation of AI systems into everyday life means that such discriminatory systems will reinforce and magnify inequalities, historical biases and power imbalances, possibly without precedent, potentially without awareness, certainly without consent. West et al. argue that there is a direct link between the lack of diversity in the AI workforce and the lack of diversity in AI systems, in what they call a “discrimination feedback loop” [Ibid., p. 15]. This affects how AI companies work, what products are built, who they are designed to serve, and who benefits from their development.
While AI is often seen as synomymous with machine learning in this context, the problems highlighted here are inherent in large sectors of academic and technological evolution. Argumentation takes place in a sociocultural context, and an arguer’s cultural background, cognitive and social abilities, age, gender, attitude to social conflict and social harmony, and so on, will all contribute to the forms which their argumentation takes. Muller Mirza et al. have studied argumentation from a psychosocial perspective [37], and argue that:
“Argumentative dynamics occur in specific sociocultural contexts, which orient, constrain and contribute to the form that they will take. In this light, argumentation is always “situated” [37, p.3]
They suggest that some commonly accepted models of argument, such as Toulmin’s layout, are culturally Euro-centred, and that there are key differences between this style of argumentation and, for instance, indirect styles of justification which are more common in Asian cultures. Other studies exploring crosscultural aspects of argumentative discourse further consolidate these findings: differences, for example, between North American and Japanese styles of argument [55], and the motivations and practices of argumentation in India and the United States [23]. Even within specific, highly structured domains such as legal settings, argument structures and patterns differ across different systems: for instance, the different types of argument used in Islamic Law and Common Law [22].
The context of an argument has cognitive as well as cultural implications. The series of experiments on Wason’s Selection Task [62] show that people are typically able to perform logical inferences on problems when framed in a familiar context, but unable to do so in contexts which are unfamiliar or abstract. These demonstrate the highly context-dependent nature of inference itself, regardless of how that is expressed in argument.
Further examples of bias in argumentation can be found in data sources. It is increasingly common to conduct studies in argumentation with real world data such as Wikipedia, social media, reddit, comments on news websites and so on. These are subject to biases of Internet culture, with contributors often being young, male, English-speaking, educated, technologically aware, and over a certain income threshold. Some of these sources have been shown to suffer from specific biases: for instance, there are strong gender, geographic and systemic biases in Wikipedia, resulting in unevenness of coverage and style [25]. These may affect argumentation styles: Jeong discusses gender interaction patterns and participation in [29].
Such considerations mean that researchers in argumentation must be aware of the cultural context within which they are working, and should seek to diversify where possible. This applies to argumentation in the context of health care in terms of topic, style, inferences, and so on. As Muller Mirza et al. argue:
“...it matters who you are arguing with, it matters what you are arguing about, it matters what context you are arguing in, and it matters why you are arguing.” [37, p.13]
A further consideration in a health care domain is the potential for asymmetrical relationships between different parties, in particular between doctors and patients [43]. Pilnick and Dingwall suggest that:
“...asymmetry lies at the heart of the medical enterprise: it is, in short, founded in what doctors are there for.” [Ibid.]
They do nevertheless argue from a position that this is based on a misunderstanding of the nature and role of medicine. Indeed, effective doctor/patient communication is an area of study in itself [51]. Moves have also been made towards a more patient-centred approach, with explanations, illustrations and negotiations being communication [16]. Care must be taken however to avoid patient-centred approaches becoming patient-led, where patient pressure or expectation can lead to doctors prescribing or carrying out unnecessary treatments [2].
Lakoff has shown how framing and metaphors affect argumentation [32]; and the role that metaphors play in healthcare communication is well documented (eg. [21]), along with possible benefits and drawbacks. For instance, the common framing of cancer as a battle metaphor, with its emphasis on winning or losing is described as “masculine, power-based, paternalistic and violent” in [6] (cited in [21]). The journey metaphor, with its emphasis on meaning and personal growth, provides a different way of understanding illness. Religious and ethical viewpoints of both patient and caregiver will similarly affect narrative, metaphor and topics selection. People’s emotional experience of illness will further affect the form and style of the argumentation and communication used.
4.3.Explanation
AI is becoming more sophisticated as it surpasses rather than supplements our capabilities. Recent advances in AI such as Google’s AlphaGo99 and self-driving cars have come largely at the price of transparency with systems increasingly becoming “black boxes”, particularly in the machine learning community [11,42]. The importance of explanations in AI and Computing in general is now a research movement gaining traction under the banner of Explainable Artificial Intelligence (XAI) (formerly known as Explanation-aware Computing), with XAI set to be key to new models of communication in AI [3]. As AI evolves it will become ethically, legally and socially imperative for it to explain and justify its processes and output [Ibid.]. This is especially true for a health care setting [26].
Ethically, we have a responsibility to ensure that in addition to increased intelligence, AI-powered e-health systems offer at least as much transparency as their human counterparts. Such issues became a legal concern when the European Union’s new General Data Protection Regulation (GDPR) took effect in 2018 [10], affecting all software used in the EU. This legislation introduced the right of citizens to receive human-intelligible explanations for algorithmic decisions. From a social standpoint some aspects of AI, such as persuasive technologies, are already seen in the context of “social actors” [18].
The necessity and value of explanations in healthcare is well documented. For instance, Fong Ha and Longnecker argue [19] that a lack of sufficient explanation can lead to poor patient understanding and feelings of disempowerment, which can result in therapeutic failure. Explanations which are balanced and understood are key to collaborative communication between patient and doctor [19]. Salmon et al further show that certain types of explanation can improve patient’s wellbeing and help to reduce demands on health services [47]. The value of taking into account patients’ own views and experience of health problems when explaining their conditions is considered in [44]; with tailored explanations increasing salience for particular groups of patients.
Explanations and arguments are closely connected, but as Walton observes [60] they serve a different dialogical purpose:
“The goal of an explanation is not to convince or persuade the party that a particular proposition is true but to express the queried proposition in some more familiar terms or relate it to another set of propositions that can be put together so that it is more familiar or comprehensible to him”
In an e-health system, the advice the system selects to provide to the user can be underpinned by models of argument; for instance, an argument for “do 150 minutes of moderate-intensity activity this week” could be constructed on the basis of two premises: that the World Health Organisation (WHO) recommending this for adults aged 18–64 [66], and the user falls into that age bracket. Those same premises could be used to offer an explanation to the user, should they question the advice, however what is key is the manner in which those premises are communicated; as with the issue of consent-gathering (Section 3.2), it should be purely factual and not in any way persuasive.
5.Recommendations
In this section, we provide a series of recommendations for the use of dialogue and argumentation in a health care context, that are relevant to the specific RRI challenges and issues discussed in this paper. These recommendations range from general advice, to directions for future research aimed at addressing the specific challenges. A summary of the recommendations is provided in Table 1, highlighting the challenge(s) they address.
Table 1
Summary of recommendations
Recommendation | Main challenge(s) addressed | Issue(s) addressed |
(1) Understand the dialogical nature of the consent-gathering process and aim to ensure it is accurately modelled in a virtual context | Collecting and handling health data | Privacy and informed consent |
(2) Understand the difference between persuasion and information-giving and ensure this is built in to the consent gathering process | Collecting and handling health data | Privacy and informed consent |
(3) Be alert to conflict if and when it arises, and be ready to react correctly to ensure it is handled in way that does not risk harming the user | Collecting and handling health data | Handling conflicts |
(4) Ensure that any advice given by an e-health system is justifiable, both internally (through explanation) and externally (through accurate and appropriate sourcing) | Appropriate trust | Fairness; Explanation |
(5) Be aware of the culture and context of deployment to avoid amplifying human biases | Appropriate trust | Fairness |
(6) Ensure that an e-health system is capable of explaining its advice without arguing for that advice | Appropriate trust | Explanation |
(1) Understand the dialogical nature of the consent-gathering process and aim to ensure it is accurately modelled in a virtual context: the process of consent-gathering in a traditional medical context is intrinsically dialogical; this allows a patient to question the need for consent, and ask for clarification should it be required. Thus any attempt to model this process computationally through models of dialogue should provide these opportunities for user response (Section 3.2).
(2) Understand the difference between persuasion and information-giving and ensure this is built in to the consent gathering process: as noted in recommendation (1), the consent-gathering process must allow a user to question the need for consent and ask for clarifications, and for the system to response appropriately. Care needs to be taken to ensure the answers and clarifications are purely factual, and not persuasive (Section 3.2).
(3) Be alert to conflict if and when it arises, and be ready to react correctly to ensure it is handled in way that does not risk harming the user: conflict is inevitable in a health care domain, with different types of conflict being more serious than others. While conflict and its resolution is a cornerstone of many models of argument and dialogue, there are limits to what an e-health system should attempt to achieve. Such systems should therefore be capable of identifying when neither it nor the user should attempt to resolve conflict, and instead refer it (or the user) to an appropriate medical practitioner (Section 3.3).
(4) Ensure that any advice given by an e-health system is justifiable, both internally (through explanation) and externally (through accurate and appropriate sourcing): an e-health system should be able to justify its advice, not just in terms of the process followed in arriving at that advice, but also the foundation upon which it was built, e.g. verifiable and trustworthy sources of health advice. (Sections 4.2 and 4.3).
(5) Be aware of the culture and context of deployment to avoid amplifying human biases: an e-health system should be sensitive to the culture and context in which it is deployed, and tailor its advice accordingly. If practical, diversify the domain, the data used, and the researchers conducting the work (Section 4.2)
(6) Ensure that an e-health system is capable of explaining its advice without arguing for that advice: a user of an e-health system should always be able to ask for an explanation of the advice given. In satisfying recommendation (5), an e-health system can use arguments to determine what advice to give; however, there is a subtle but important difference between argument and explanation. The latter should not in any way be persuasive, but simply set out the facts (Section 4.3).
6.Summary and conclusions
We have in this paper examined two main challenges for Responsible Research and Innovation (RRI) that have particular relevance to the use of dialogue and argumentation in the deployment of e-health systems for advice giving. These challenges, collecting and handling health data and appropriate trust are each further sub-divided into two issues: privacy and informed consent and handling conflict; and fairness and explanation respectively. In the case of the latter, these constitute two of the pillars of trust in AI [27].
Dialogue has an important role to play in e-health systems, and computational models of formalised dialogue games can underpin this. There are nevertheless several pitfalls that need to be avoided to ensure that those dialogue models are effective, ethical and legal. These issues arise throughout the use of an e-health system, from the consent gathering process, to conflict resolution and the provision of advice.
One reason for dialogue-based interactions is to allow the patient (or, in the case of an e-health system, user) to ask questions. This can be either to query the need for consent, or actual advice given. In real doctor/patient interactions, there is a risk that when a patient queries a suggested course of treatment, the doctor “argues” in favour it rather than providing a purely factual explanation [46]. This distinction between argument and explanation is subtle but important [60].
Trust is a significant challenge in artificial intelligence, and in particular in AI-based e-health systems. Users must be able to trust the advice such a systems give for them to be of practical use, but building that trust requires careful consideration and design. As well as ensuring that advice is appropriately-sourced, there are other, perhaps more subtle factors that should be taken into account. For instance, when designing algorithms to select advice it is important that researchers’ own biases are not amplified, even unknowingly, which is a problem for AI in general [64]. It is also important to take into account cultural considerations with different cultures taking different approaches to argument and argumentation [22,23,55]. Indeed, cultural differences also manifest themselves in doctor/patient interactions [16]; how this might translate to user interaction with an e-health system presents an interesting avenue for future research.
The recommendations we present in this paper are designed partly to show where the challenges lie in using argumentation and dialogue in an e-health context, but also to act as prompts for future discussion and research. Such future work might focus on a specific recommendation (for instance, the design, implementation and evaluation of a dialogue protocol for consent-gathering), or examine the recommendations more generally with a view to developing guidelines and frameworks that help developers of e-health systems navigate the challenges and issues. We also do not consider the list of recommendations to be exhaustive, but instead see them as a starting point for further recommendations that cover other challenges and issues.
Dialogue and argumentation have a key role in ensuring responsible innovation in an e-health context and the recommendations presented in this paper provide a solid foundation for that role to be realised.
Notes
7 While in general, consent-gathering could be seen as information-giving, “information-giving” and “information-seeking” can be considered the same [59, p.44].
Acknowledgements
This project has received funding from the European Union’s Horizon 2020 research and innovation programme under Grant Agreement #769553. This result reflects only the authors’ view and the EU is not responsible for any use that may be made of the information it contains. The authors are also grateful to colleagues in the Council of Coaches consortium and the anonymous reviewers for their input and feedback on earlier versions of this work.
References
[1] | B. Abrahaoa, P. Parigib, A. Guptac and K.S. Cooka, Reputation offsets trust judgments based on social biases among Airbnb users, in: Proceedings of the National Academy of Sciences of the United States of America, (2017) . |
[2] | Academy of Medical Royal Colleges, Forty treatments that bring little or no benefit to patients, 2016, https://www.aomrc.org.uk/wp-content/uploads/2016/10/Choosing_wisely_PR_211016-3.pdf, Accessed: 2020-05-27. |
[3] | A. Adadi and M. Berrada, Peeking inside the black-box: A survey on explainable artificial intelligence (XAI), IEEE Access 6: ((2018) ), 52138–52160. doi:10.1109/ACCESS.2018.2870052. |
[4] | J.G. Anderson, Social, ethical and legal barriers to e-health, International journal of medical informatics 76: (5–6) ((2007) ), 480–483. |
[5] | M. Arnold, R.K.E. Bellamy, M. Hind, S. Houde, S. Mehta, A. Mojsilovic, R. Nair, K. Natesan Ramamurthy, D. Reimer, A. Olteanu, D. Piorkowski, J. Tsay and K.R. Varshney, FactSheets: Increasing trust in AI services through supplier’s declarations of conformity, arXiv:1808.07261v2, 2019. |
[6] | A. Bleakley, R. Marshall and D. Levine, He drove forward with a yell: Anger in medicine and Homer, Medical Humanities 40: ((2014) ), 22–30. doi:10.1136/medhum-2013-010432. |
[7] | H. Breivik, B. Collett, V. Ventafridda, R. Cohen and D. Gallacher, Survey of chronic pain in Europe: Prevalence, impact on daily life, and treatment, European journal of pain 10: (4) ((2006) ), 287. doi:10.1016/j.ejpain.2005.06.009. |
[8] | H. Breivik, E. Eisenberg and T. O’Brien, The individual and societal burden of chronic pain in Europe: The case for strategic prioritisation and action to improve knowledge and availability of appropriate care, BMC public health 13: (1) ((2013) ), 1229. doi:10.1186/1471-2458-13-1229. |
[9] | Council of the European Union, Rome declaration on responsible research and innovation in Europe, 2014, https://ec.europa.eu/research/swafs/pdf/rome_declaration_RRI_final_21_November.pdf, Accessed: 2020-05-28. |
[10] | Council of the European Union, Council Regulation (EC) No. 2016/679 on the protection of natural persons with regard to the processing of personal data and on the free movement of such data, and repealing Directive 95/46/EC (General Data Protection Regulation), 2016, https://publications.europa.eu/en/publication-detail/-/publication/3e485e15-11bd-11e6-ba9a-01aa75ed71a1/language-en. |
[11] | K. de Fine Licht and J. de Fine Licht, Artificial intelligence, transparency, and public decision-making, AI & SOCIETY ((2020) ), 1–10. |
[12] | G. Elwyn, D. Frosch, R. Thomson, N. Joseph-Williams, A. Lloyd, P. Kinnersley, E. Cording, D. Tomson, C. Dodd, S. Rollnick et al., Shared decision making: A model for clinical practice, Journal of general internal medicine 27: (10) ((2012) ), 1361–1367. doi:10.1007/s11606-012-2077-6. |
[13] | E. Ert, A. Fleischer and N. Magen, Trust and reputation in the sharing economy: The role of personal photos in Airbnb, Advances in Consumer Research 43: ((2015) ). |
[14] | E. Etchells, G. Sharpe, P. Walsh, J.R. Williams and P.A. Singer, Bioethics for clinicians: 1. Consent, Canadian Medical Association Journal 155: (2) ((1996) ), 177–180. |
[15] | G. Eysenbach, Towards ethical guidelines for e-health: JMIR theme issue on eHealth ethics, J Med Internet Res 2: (1) ((2000) ), e7. |
[16] | W.J. Ferguson and L.M. Candib, Culture, language, and the doctor-patient relationship, FMCH Publications and Presentations (2002), 61. |
[17] | E. Fisher and D. Schuurbiers, Socio-technical integration research: Collaborative inquiry at the midstream of research and development, in: Opening up the Laboratory: Approaches for Early Engagement with New Technology, Vol. 16: , I. van de Poel, M.E. Gorman, N. Doorn and D. Schuurbiers, eds, (2013) , pp. 97–110. |
[18] | B.J. Fogg, Persuasive technology: Using computers to change what we think and do, Ubiquity 2002: (December) ((2002) ), 2. doi:10.1145/764008.763957. |
[19] | J. Fong Ha and N. Longnecker, Doctor-patient communication: A review, The Ochsner Journal 10: ((2010) ), 38–43. |
[20] | M.A. Grando, L. Moss, D. Sleeman and J. Kinsella, Argumentation-logic for creating and explaining medical hypotheses, Artificial intelligence in medicine 58: (1) ((2013) ), 1–13. doi:10.1016/j.artmed.2013.02.003. |
[21] | A.W. Gustafsson and C. Hommerberg, “It is completely ok to give up a little sometimes”: Metaphors and normality in Swedish cancer talk, Critical Approaches to Discourse Analysis across Disciplines 10: (1) ((2018) ), 1–16. |
[22] | W.B. Hallaq, The logic of legal reasoning in religious and non-religious cultures: The case of Islamic law and the common law, Clevland State Law Review 34: (79) ((1985) –(1986) ), 79–96. |
[23] | D. Hample and D. Anagondahalli, Understandings of arguing in India and the United States: Argument frames, personalization of conflict, argumentativeness, and verbal aggressiveness, Journal of Intercultural Communication Research 44: (1) ((2015) ), 1–26. doi:10.1080/17475759.2014.1000939. |
[24] | J. Hendler and T. Berners-Lee, From the semantic web to social machines: A research challenge for AI, Artificial Intelligence 174: ((2010) ), 156–161. doi:10.1016/j.artint.2009.11.010. |
[25] | B.M. Hill and A. Shaw, The Wikipedia gender gap revisited: Characterizing survey response bias with propensity score estimation, PLoS ONE 8: (6) ((2013) ). |
[26] | A. Holzinger, C. Biemann, C. Pattichis and D. Kell, What do we need to build explainable AI systems for the medical domain? 12 (2017). |
[27] | IBM Research, Trusting AI, https://www.research.ibm.com/artificial-intelligence/publications/2018/download/pdf/trustingAI.pdf, 2018. |
[28] | International Diabetes Federation (IDF), Diabetes Atlas seventh edition, 4.2 Europe, 2017, https://diabetesatlas.org/en/, Accessed: 2020-05-28. |
[29] | A. Jeong, Gender interaction patterns and gender participation in computer-supported collaborative argumentation, American Journal of Distance Education 20: (4) ((2006) ), 195–210. doi:10.1207/s15389286ajde2004_2. |
[30] | R.B. Kantharaju, A. Pease, D. Reidsma, C. Pelachaud, M. Snaith, M. Bruijnes, R. Klaassen, T. Beinema, G. Huizing, D. Simonetti et al., Integrating argumentation with social conversation between multiple virtual coaches, in: Proceedings of the 19th ACM International Conference on Intelligent Virtual Agents, (2019) , pp. 203–205. doi:10.1145/3308532.3329450. |
[31] | N. Kökciyan, M. Chapman, P. Balatsoukas, I. Sassoon, K. Essers, M. Ashworth, V. Curcin, S. Modgil, S. Parsons and E.I. Sklar, A collaborative decision support tool for managing chronic conditions, in: MedInfo, (2019) , pp. 644–648. |
[32] | G. Lakoff, Don’t Think of an Elephant: Know Your Values and Frame the Debate, Chelsea Green Publishing Co., (1990) . |
[33] | J.D. Lee and T.F. Sanquist, Augmenting the operator function model with cognitive operations: Assessing the cognitive demands of technological innovation in ship navigation, IEEE Transactions on Systems, Man, and Cybernetics – Part A: Systems and Humans 30: ((2000) ), 273–285. doi:10.1109/3468.844353. |
[34] | J.D. Lee and K.A. See, Trust in automation: Designing for appropriate reliance, Human Factors 46: (1) ((2004) ), 50–80. doi:10.1518/hfes.46.1.50.30392. |
[35] | E.A. Locke and G.P. Latham, Building a practically useful theory of goal setting and task motivation: A 35-year odyssey., American psychologist 57: (9) ((2002) ), 705. doi:10.1037/0003-066X.57.9.705. |
[36] | C. Mittendorf, What trust means in the sharing economy: A provider perspective on Airbnb.com, in: Twenty-Second Americas Conference on Information Systems, San Diego, (2016) . |
[37] | N. Muller Mirza, A.-N. Perret-Clermont, V. Tartas and A. Iannaccone, Psychosocial processes in argumentation, in: Argumentation and Education. Theoretical Foundations and Practices, N. Muller Mirza and A.-N. Perret-Clermont, eds, Springer, (2009) . doi:10.1007/978-0-387-98125-3. |
[38] | T. Nadelson, The inhuman computer / the too-human psychotherapist, American journal of psychotherapy 41: ((1987) ), 489–498. doi:10.1176/appi.psychotherapy.1987.41.4.489. |
[39] | National Health Service Health Research Authority, I’m developing medical software, 2019, https://www.hra.nhs.uk/planning-and-improving-research/research-planning/how-were-supporting-data-driven-technology/im-developing-medical-software/, Accessed: 2020-05-22. |
[40] | R.Ø. Nielsen and B. Bedsted, The COUCH RRI vision, 2018, https://council-of-coaches.eu/wp-content/uploads/2019/03/D2.1-The-COUCH-RRI-Vision-v1.0.1.pdf. |
[41] | H. op den Akker, R. op den Akker, T. Beinema, O. Banos, D. Heylen, B. Bedsted, A. Pease, C. Pelachaud, V.T. Salcedo, S. Kyriazakos and H. Hermen, Council of coaches: A novel holistic behavior change coaching approach, in: 4th International Conference on Information and Communication: Technologies for Aging Well and e-Health, (2018) . |
[42] | F. Pasquale, The Black Box Society, Harvard University Press, (2015) . doi:10.4159/harvard.9780674736061. |
[43] | A. Pilnick and R. Dingwall, On the remarkable persistence of asymmetry in doctor/patient interaction: A critical review, Social science & medicine 72: (8) ((2011) ), 1374–1382. doi:10.1016/j.socscimed.2011.02.033. |
[44] | C. Pope, P.v. Royen and R. Bake, Qualitative methods in research on healthcare quality, Qual Saf Health Care 11: ((2002) ), 148–152. doi:10.1136/qhc.11.2.148. |
[45] | C. Reed, Dialogue frames in agent communication, in: Proceedings of the Third International Conference on Multi-Agent Systems (ICMAS 1998), Y. Demazeau, ed., IEEE Press, Paris, France, (1998) , pp. 246–253. |
[46] | S. Rubinelli and P.J. Schulz, “Let me tell you why!”. When argumentation in doctor-patient interaction makes a difference, Argumentation 20: ((2006) ), 353–375. doi:10.1007/s10503-006-9014-y. |
[47] | P. Salmon, S. Peters and I. Stanley, Patients’ perceptions of medical explanations for somatisation disorder: Qualitative analysis, BMJ 318: (7180) ((1999) ), 372–376. doi:10.1136/bmj.318.7180.372. |
[48] | I. Sassoon, N. Kökciyan, E. Sklar and S. Parsons, Explainable argumentation for wellness consultation, in: International Workshop on Explainable, Transparent Autonomous Agents and Multi-Agent Systems, Springer, (2019) , pp. 186–202. doi:10.1007/978-3-030-30391-4_11. |
[49] | B. Shneiderman, Designing trust into online experiences, COMMUNICATIONS OF THE ACM 43: (12) ((2000) ), 57–59. doi:10.1145/355112.355124. |
[50] | C.E. Short, A. DeSmet, C. Woods, S.L. Williams, C. Maher, A. Middelweerd, A.M. Müller, P.A. Wark, C. Vandelanotte, L. Poppe, M.D. Hingle and R. Crutzen, Measuring engagement in eHealth and mHealth behavior change interventions: Viewpoint of methodologies, J Med Internet Res 20: (11) ((2018) ), e292. |
[51] | J. Silverman, S. Kurtz and J. Draper, Skills for Communicating with Patients, CRC Press, (2016) . doi:10.1201/9781910227268. |
[52] | M. Snaith, D. De Franco, T. Beinema, H. op den Akker and A. Pease, A dialogue game for multi-party goal-setting in health coaching, in: Proceedings of the 7th International Conference on Computational Models of Argument (COMMA 2018), IOS Press, Warsaw, Poland, (2019) , pp. 337–344. |
[53] | M. Snaith, J. Lawrence and C. Reed, Mixed initiative argument in public deliberation, Online Deliberation (2010), 2. |
[54] | P. Sparaco, Airbus seeks to keep pilot, new technology in harmony, Aviation Week and Space Technology ((1995) ), 62–63. |
[55] | H. Tamura, The Japanese/American interface: A crosscultural study on the approach to discourse, PhD thesis, Portland State University, 1983. |
[56] | The Responsible Industry Consortium, Guide for the implementation of Responsible Research and Innovation (RRI) in the industrial context, 2017, http://www.responsible-industry.eu. |
[57] | United Nations Department of Economic, World Population Prospects: The 2017 Revision, http://www.un.org/en/development/desa/population/events/other/21/index.shtml, 2017, Accessed: Apr 2018. |
[58] | R. von Schomberg, Towards responsible research and innovation in the information and communication technologies and security technologies fields, Available at SSRN 2436399 (2011). |
[59] | D. Walton, One-Sided Arguments: A Dialectical Analysis of Bias, SUNY Press, (1999) . |
[60] | D. Walton, Fundamentals of Critical Argumentation, Cambridge University Press, (2005) . doi:10.1017/CBO9780511807039. |
[61] | D.A. Walton and E.C.W. Krabbe, Commitment in Dialogue: Basic Concepts of Interpersonal Reasoning, SUNY Press, (1995) . |
[62] | P.C. Wason and D. Shapiro, Natural and contrived experience in a reasoning problem, Quarterly Journal of Experimental Psychology 23: ((1971) ), 63–71. doi:10.1080/00335557143000068. |
[63] | J. Weizenbaum, Computer Power and Human Reason, Freeman, San Francisco, (1976) . |
[64] | S.M. West, M. Whittaker and K. Crawford, Discriminating systems: Gender, race and power in AI, https://ainowinstitute.org/discriminatingsystems.html, 2019. |
[65] | World Health Organisation, Global strategy and action plan on ageing and health (2016–2020), 2016, https://www.who.int/ageing/GSAP-Summary-EN.pdf, Accessed: 2020-05-28. |
[66] | World Health Organisation, Physical activity, 2018, https://www.who.int/news-room/fact-sheets/detail/physical-activity, Accessed: 2020-05-28. |