Special issue: CMNA coming of age: 18 years of the Computational Models of Natural Argument workshop
The workshop on Computational Models of Natural Argument (CMNA) is the longest standing event on Argument and Computation. Since its inception in San Francisco in 2001, numerous others have been established, dedicated to the various aspects of argumentation and computation, most notably the ArgMAS workshops, focusing on Multi-Agent Systems since 2004, the biennial COMMA conference series, focusing on computational models and tools since 2006, the TAFA workshop series, focusing on formal models since 2011, the Argument Mining series, since 2014, and the most recent SAFA series, focusing on systems and algorithms for formal argumentation, since 2016 (see Fig. 1).
Fig. 1.
Timeline of CMNA workshops and other major Argument and Computation events.
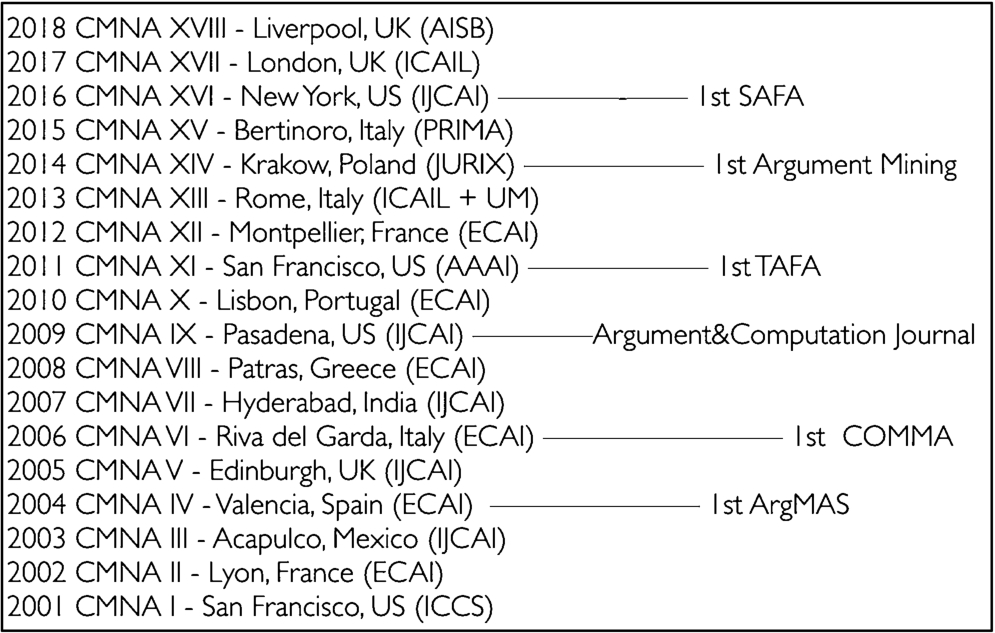
Despite the proliferations of venues, CMNA has managed throughout the years to maintain a unique informal and inclusive atmosphere, creating a true forum for discussion, a venue to foster exchange of ideas and encourage cooperation, rather than being a mere collection of presentations, and a space for exploring areas of enquiry outside mainstream argument and computation. As a further manifestation of this spirit, CMNA is characterized by a peripatetic nature, each year associating with different major events, in order to foster cross fertilisation of disciplines: CMNA has been held over the years in conjunction with the major European and the International Conferences on AI (ECAI, AAAI, IJCAI), with legal informatics venues (ICAIL and JURIX), with user modelling and simulation of behavior events (AISB and UMUAI), as well as Multi-Agent Systems conferences (PRIMA).
The present special issue is conceived as a celebration of the first 18 years of CMNA, and collects selected, extended papers from the 2016 and 2017 editions, all subjected to post-event resubmission and review procedure. The selected papers provide a testimony to the breadth of topics around how computational methods can be used to model “natural” argumentation, whether by mining authentic, human produced argumentative texts, or by concentrating on argumentation schemes used in context, or by focusing on the less explored rhetorical features of “real” arguments.
In this issue, Schneider and Jackson’s paper focuses on modeling how argument schemes are established, giving the Randomized Clinical Trial (RCT) as an example of a recently established inference rule in medical science. According to S&J, new inference rules are defended by argumentation, and are subject over time to challenges, refinement, and possibly abandonment in favor of competing rules. Thus, it is important to model the creation and evolution of argument schemes in computational models of natural argument. They illustrate how a Practical Reasoning scheme can be used to model justification for adoption of an RCT scheme. However, once a scheme such as RCT has been established, arguments making use of RCT typically do not challenge RCT itself, although that is possible. S&J also note that in medical science, there are preferences among different methods, with RCT as the currently most preferred method for drawing conclusions about effects of medical treatments. Thus, it is necessary also to model arguments about preferences among alternative inference schemes.
Gordon, Friedrich and Walton’s paper presents an approach for representing argumentation schemes as Constraint Handling Rules (CHR). The perspective on argumentation schemes in the paper is not so much the one of evaluating arguments, but rather the one of producing good argumentation. The paper provides de facto a high level programming language for representing and “executing” argumentation schemes. The approach is grounded on the authors long term “Carneades” project, but addresses important issues that can be generalized to other contexts. The CHR perspective in implementing argumentation schemes offers a number of conceptualization features, including the opportunity to distinguish between two categories of critical questions (by exception and by assumption), as well as practical ones, for example a more concise representation of schemes with a plurality of conclusions. The paper is enriched by a useful compendium of twenty argumentation schemes, with a full discussion on their implementation in the formalism.
Green’s paper proposes a semantics-informed approach to mining individual arguments in biomedical/biological research articles as an alternative to applying machine learning models derived from superficial features of a text. First, entities and relations would be extracted from a text using BioNLP tools. Then argument schemes implemented as logic programs in terms of semantic propositions could be used to identify an argument’s premises and conclusions, including implicit conclusions. The process is illustrated using argument schemes that were implemented based upon analysis of a biomedical research article. Next, Green examines how the arguments in that article are related to its discourse structure and to each other. Modeling the discourse structure in terms of current approaches to classifying text segments in scientific discourse, it is found that the text of arguments is not in one-to-one correspondence with discourse boundaries, although such an analysis may provide useful contextual information augmenting a semantic approach to argument mining. Finally, analyzing relationships between arguments in that article Green shows that a richer model of dialectical structure is needed to model the narrative of scientific discovery.
Saint-Dizier’s paper builds upon his earlier work using the Generative Lexicon (GL) for argument mining of controversial social issues, e.g. from newspaper articles. The GL is a repository of lexical and domain knowledge. Previously, he showed how it is necessary to use the GL to fully comprehend such arguments in order to mine them. The current paper addresses the problem of synthesis: combining and presenting the many extracted arguments on an issue in an intelligible manner. The GL is used to cluster the argument propositions that have been mined based upon their conceptual similarity. Then abstract linguistic patterns are used to realize the clusters in natural language. In addition to natural language realization, the presentation includes navigation facilities that allow users to see the original text from which the argument has been mined.
Finally, Harris et al.’s paper provides a welcome and much needed perspective on Computational Rhetoric. Advocating for a better account of Figural Logic for the tasks of argument mining, machine learning, or general argument understanding, the authors present an eXtensible Markup Language (XML) annotation scheme for Rhetorical Figures. Corroborated by numerous practical examples, the paper provides an important step towards a more sophisticated modelling of rhetorical figures, and also constitutes a useful introduction to the problem at hand, which is more fully explored in a dedicated special issue of this journal edited by the same authors (Argument & Computation – Volume 8, issue 3).
Confident that the issue will be an interesting read for a wider audience, and looking forward to many more years of CMNA, we wish to thank the CMNA programme committee, who has shown in the years a tremendous support, not only to the revision and selection of papers, but also to maintaining the “CMNA flavour” to the event, and to the colleagues who alternated in the steering committee since 2001, contributing to the success of the initiative: Chris Reed, Giuseppe Carenini, Rodger Kibble, Floris Bex, and Chrysanne de Marco.
Floriana Grasso
Nancy Green
(guest editors)