Left Frontal Hub Connectivity during Memory Performance Supports Reserve in Aging and Mild Cognitive Impairment
Abstract
Reserve in aging and Alzheimer’s disease (AD) is defined as maintaining cognition at a relatively high level in the presence of neurodegeneration, an ability often associated with higher education among other life factors. Recent evidence suggests that higher resting-state functional connectivity within the frontoparietal control network, specifically the left frontal cortex (LFC) hub, contributes to higher reserve. Following up these previous resting-state fMRI findings, we probed memory-task related functional connectivity of the LFC hub as a neural substrate of reserve. In elderly controls (CN, n = 37) and patients with mild cognitive impairment (MCI, n = 17), we assessed global connectivity of the LFC hub during successful face-name association learning, using generalized psychophysiological interaction analyses. Reserve was quantified as residualized memory performance, accounted for gender and proxies of neurodegeneration (age, hippocampus atrophy, and APOE genotype). We found that greater education was associated with higher LFC-connectivity in both CN and MCI during successful memory. Furthermore, higher LFC-connectivity predicted higher residualized memory (i.e., reserve). These results suggest that higher LFC-connectivity contributes to reserve in both healthy and pathological aging.
INTRODUCTION
Reserve designates the ability to maintain cognitive performance relatively well in the face of developing neurodegeneration. The idea of reserve emerged from the observation that a subset of subjects with neuropathological postmortem diagnosis of Alzheimer’s disease (AD) were free of dementia during life [1]. These findings suggested that the absence of dementia cannot be attributed to lower levels of neuropathology but are likely due to protective brain features [1]. To date, an open question remains about what constitutes such protective brain features. Higher pre-morbid whole brain volume and regional grey matter volume has been associated with higher reserve in aging and AD [2–5]. Apart from such volumetric differences, functional brain features may contribute to reserve. Higher FDG-PET metabolism or hippocampus activation has been observed in patients with mild cognitive impairment or AD dementia, suggesting compensatory activation [6–9]. However, the cognitive benefit of such hyperactivity is questionable, as higher hippocampus activity and functional connectivity have been shown to be a sign of transient diffuse hyperactivation on a trajectory of functional and cognitive decline [10–13]. Another common approach to probe for functional brain changes underlying reserve is to employ protective factors such IQ, education or occupation as predictors of altered brain activity that may underlie reserve. The idea is that early life experiences, e.g., education, may shape brain function in a long-lasting way, and thus confer protection against the impact of neurodegeneration in late life, an effect also called cognitive reserve [14]. Functional MRI (fMRI) studies reported such life-experience factors to be associated with altered activation during tasks such as language comprehension, visual encoding, and working memory in aging and mild cognitive impairment (MCI) [15–17]. Although the findings showed variability in terms of the brain regions involved, there is now accumulating evidence that protective factors are associated particularly with frontal lobe function across different tasks and during resting state [18–21].
A core candidate brain region underlying reserve is a hub region in the left frontal cortex (LFC, covering BA 6/44) of the frontoparietal control network. In young to middle-age subjects, resting-state connectivity of that LFC hub region was associated with protective factors such as IQ and higher cognitive performance [22, 23]. We recently showed, based on resting-state fMRI, that global connectivity of the same LFC hub close to the Broca area is associated with both higher education and ameliorated impact of FDG-PET hypometabolism on memory in amyloid-positive MCI [24]. These results suggest that higher LFC-connectivity supports the ability to maintain better memory performance in the face of developing neurodegeneration in AD. Based on these previous results from resting-state fMRI, we asked here in the current task-related fMRI study the question whether LFC hub connectivity probed during an episodic memory task is associated with reserve in aging and MCI. Assessing the associationbetween task-related LFC-connectivity and reserve is a critical test for linking LFC-connectivity to cognitive processes of reserve. Following our previously introduced research criteria to test neural mechanisms of reserve [24], we hypothesized that 1) greater education is associated with higher task-related LFC-connectivity during successful memory performance (criterion of face validity), and that 2) higher task-related LFC-connectivity contributes to higher reserve, i.e., higher memory-task performance despite neurodegeneration as assessed by hippocampus atrophy (criterion of cognitive benefit). Specifically, we assessed reserve by adopting a previously established quantitative approach of reserve [25–27], including the residual of memory performance after accounting for age, APOE genotype and hippocampal volume as a scalar index of reserve. The residualized memory performance reflecting the extent to which memory is sustained despite old age, genetic risk of AD (APOE) and hippocampus-atrophy. Our hypotheses were tested independently in cognitively normal elderly (CN) and MCI to assess whether global LFC-connectivity consistentlysupports reserve in normal and pathological aging.
METHODS
Participants
We included 37 CN elderly and 17 MCI patients who were recruited at the outpatient clinic of the Institute for Stroke and Dementia Research, Munich. All participants underwent cognitive testing using the CERAD-NP-Plus battery, memory task-fMRI, and venipuncture for APOE genotyping. The CERAD-NP-Plus battery is a widely used cognitive test battery for cognitive evaluation of elderly subjects and patients with cognitive impairment or dementia. It encompasses a wide spectrum of tests assessing performance in naming, verbal fluency, verbal and visual memory as well as constructional praxis and cognitive speed [28].
Inclusion criteria for the current study were age >60 y, while exclusion criteria were presence of depressive symptoms, any history of substance abuse, evidence of past neurological or psychiatric disorders, diabetes mellitus, or MRI contraindications. Participants were defined as CN when scoring not lower than 1.5 standard deviations (SD) below the age, gender and education adjusted norms in all subtests of the CERAD-NP-Plus battery [29]. In contrast, amnestic MCI was diagnosed according to the Petersen criteria [30] when scoring 1.5 SD below these norms in at least one of the learning or recall subtests of the CERAD-NP-Plus battery. Test standardized group-average test scores of the MCI subjects can be found in Supplementary Table 1. Years of education were assessed in a standardized manner as the sum of years spent in school, professional training or university, following therecommendations of the CERAD-NP-Plus protocol.
Standard protocol approvals, registrations, and patient consents
The study was approved by the ethics department of the Ludwig-Maximilians-University. All participants were informed about study procedures and provided written informed consent.
fMRI associative memory paradigm
We employed a mixed block and event-related face-name learning task design, adapted from previous fMRI studies that assessed memory-related brain activation in CN and MCI samples [31, 32]. The task encompassed 14 blocks of face-name encoding, during each of which participants had to learn 8 novel face-name pairs that were subsequently shown for 5 s each. Each encoding block was followed by a block of recognition, during which face-name pairs were presented again together with a distractor name, where subjects were asked to select the correct name via button press. Here, correct responses were classified as successful recognition, incorrect responses as incorrect recognition. Based on responses during the recognition block, the corresponding encoding trials were classified as successful or incorrect encoding, respectively. The overall fMRI accuracy was calculated as the percentage of face-name pairs that were correctly recognized. For a more detailed description of the fMRI-task and the technical equipment used, please see Supplementary Methods 1.
MRI data acquisition
MRI data was acquired on a Siemens Verio 3T MRI scanner (Siemens AG, Erlangen, Germany), and a 12-channel head coil. Initially, a high-resolution T1-MPRAGE sequence (TR/TE = 1750/2.52 ms, Flip angle = 9°) with 1×1×1 mm voxel resolution was obtained. Task-fMRI was recorded using a T2*-weighted echo-planar imaging (EPI) pulse sequence (TR/TE = 2000/30 ms, Flip angle = 90°) with an in-plane resolution of 3.4 mm, a slice thickness of 3 mm and an interslice gap of 1 mm. Overall, 900 EPI volumes were recorded. Prior to the fMRI task, gradient-echo fieldmaps were recorded to assess magnetic field inhomogeneities (TR/TE1/TE2 = 488/4.92/7.38 ms).
Preprocessing of MRI data and hippocampus volume assessment
Preprocessing was performed using Statistical Parametric Mapping (SPM) 12 (Wellcome Trust Centre for Neuroimaging, UCL, London, UK). Spatial normalization of structural and functional images to MNI space was conducted using a high-dimensional non-linear algorithm implemented in SPM [33]. Functional images were motion-, slice-time- and field-map corrected and subsequently smoothed using an 8 mm FWHM kernel. As a surrogate for neuronal loss in aged and MCI cohorts that is highly related to memory performance [34], we extracted hippocampal volume via a pre-established protocol [35] using bilateral hippocampal masks of the AAL atlas [36]. Detailed procedures on MRI preprocessing and hippocampus volumetric assessment can be found in Supplementary Methods 2.
Task-fMRI analysis
Subject level task-fMRI data, was analyzed using a fixed-effects general linear model (GLM) as implemented in SPM. The design matrix was modeled entering condition regressors (successful encoding, incorrect encoding, successful recognition, incorrect recognition) and instruction regressors each convolved with a canonical hemodynamic response function (HRF) plus a multivariate Taylor expansion (i.e., temporal and dispersion derivatives). The six motion parameters that were estimated during preprocessing were entered as nuisance regressors. To assess brain activation that best reflected brain activity specific for successful memory performance, we modeled the contrasts successful encoding > incorrect encoding and successful recall > incorrect recall for each participant, similar to a previous study [37].
Definition of LFC and control regions of interest (ROIs)
To define ROIs for task-related functional connectivity analyses, we followed the protocol of our previous resting-state fMRI study [24] using three 8 mm spherical ROIs, i.e., the LFC and two control ROIs to later assess whether our hypotheses were specific for the LFC. In brief, the LFC ROI (i.e., transition zone between Brodman areas 6 and 44; MNI: x = –42, y = 6, z = 28) was previously defined as meta-analytically derived peak activation associated with cognitive control (see [24] for detailed methods). The control ROIs were centered around the occipital pole (MNI: x = –19, y = –102, z = –3), and the precuneus (MNI: x = 7, y = –60, z = 21). We used the occipital pole since it harbors a unimodal sensory region, which is in contrast to the LFC not involved in higher cognition. In contrast, the precuneus was selected because it is a functional hub region, which is like the LFC globally connected to the rest of the brain [38, 39] and involved in higher cognition, including memory [32, 37].
Generalized psychophysiological interaction (gPPI) analysis
We applied gPPI analyses to assess condition-specific LFC-connectivity during memory processing (i.e., successful encoding and successful recognition) for each subject. We used gPPIs since they are more sensitive than standard PPIs, as they allow independent modeling of different conditions [40]. Following a recommended protocol [41], we extracted the LFC time course that was deconvolved and multiplied with the task regressor (successful encoding or successful recognition) and subsequently reconvolved with a canonical HRF yielding the PPI interaction terms. For subject-level gPPI analyses, we created a design matrix including PPI terms for successful encoding and successful recognition, condition regressors (successful encoding, incorrect encoding, successful recognition, incorrect recognition), and instruction regressors each convolved with a canonical HRF plus a multivariate Taylor expansion. The six motion parameters and the overall LFC time course were entered as nuisance regressors, following a pre-established protocol [42]. To assess LFC-connectivity during successful encoding and successful recognition, we modeled the PPI contrasts successful encoding > 0 and successful recognition > 0 for each subject. Equivalent analyses were conducted for both control ROIs (i.e., precuneus and occipital pole).
Statistical analysis
Baseline demographics were compared between CN and MCI groups using 2-sample t-tests for continuous measures and Chi-square tests forcategorical measures.
Significant group-level brain activation related to successful memory was assessed based on voxel-wise t-tests >0 on the GLM contrasts of successful encoding > incorrect encoding and successful recognition > incorrect recognition, controlling for age and gender. Group differences between CN and MCI in these contrasts were assessed using a voxel-wise ANCOVA controlling for age, gender and voxel-wise grey matter volume by entering the subjects’ modulated, smoothed, and normalized GM maps as covariate images as implemented in the biological parametric mapping toolbox [43]. For all voxel-wise analyses, activation differences were considered significant when surpassing a voxel threshold of α= 0.001 and a FWE corrected cluster threshold at α= 0.05. Stratified by diagnostic group, we subsequently tested whether greater education was associated with greater task activation in the above listed contrasts, using robust multiple regression to reduce sensitivity against outliers controlling for age, gender, and voxel-wise grey matter volume. To test whether greater LFC activation (rather than functional connectivity) was related to higher reserve in the memory domain, we extracted the mean activation of the LFC-ROI from the GLM maps (i.e., successful encoding > incorrect encoding and successful recognition > incorrect recognition). We quantified memory reserve adopting a modified approach of computing residualized memory performance by regressing out demographics, a measure of brain atrophy and genetic risk for AD [25]. The principle of this reserve measure is illustrated in Fig. 1. In brief, we determined to what extent an individual’s observed memory performance was better or worse than the performance levels as expected based on age, hippocampus atrophy, and APOE genotype. To this end, linear regression with mean fMRI-task accuracy as the dependent variable, and age, gender, hippocampal volume, APOE genotype, and mean fMRI-task reaction time was computed. The residuals were saved for each subject and used as a measure of memory reserve in subsequent analyses. Residuals showed no significant deviation from a Gaussian normal distribution as assessed via a Shapiro-Wilk test and were standardized to z-scores for further analyses. In an exploratory approach, we created a second residual score, this time also regressing out the MMSE score, to more specifically assess residual memory performance that is independent of global cognition, following a previous approach [44]. Using linear regression stratified by diagnostic group, we then tested whether greater LFC-activation predicted higher residualizedmemory accounted for MMSE.
Fig.1
Illustration of the principle underlying the reserve measure used for the current study. The actual level of memory performance is plotted against the memory performance as predicted by age, hippocampal volume, APOE carrier-status, and gender. Individuals whose actual memory performance level is higher than predicted (green circles) have high reserve, whereas individuals whose actual memory performance is lower than predicted (blue circles) have low reserve in the memory domain.
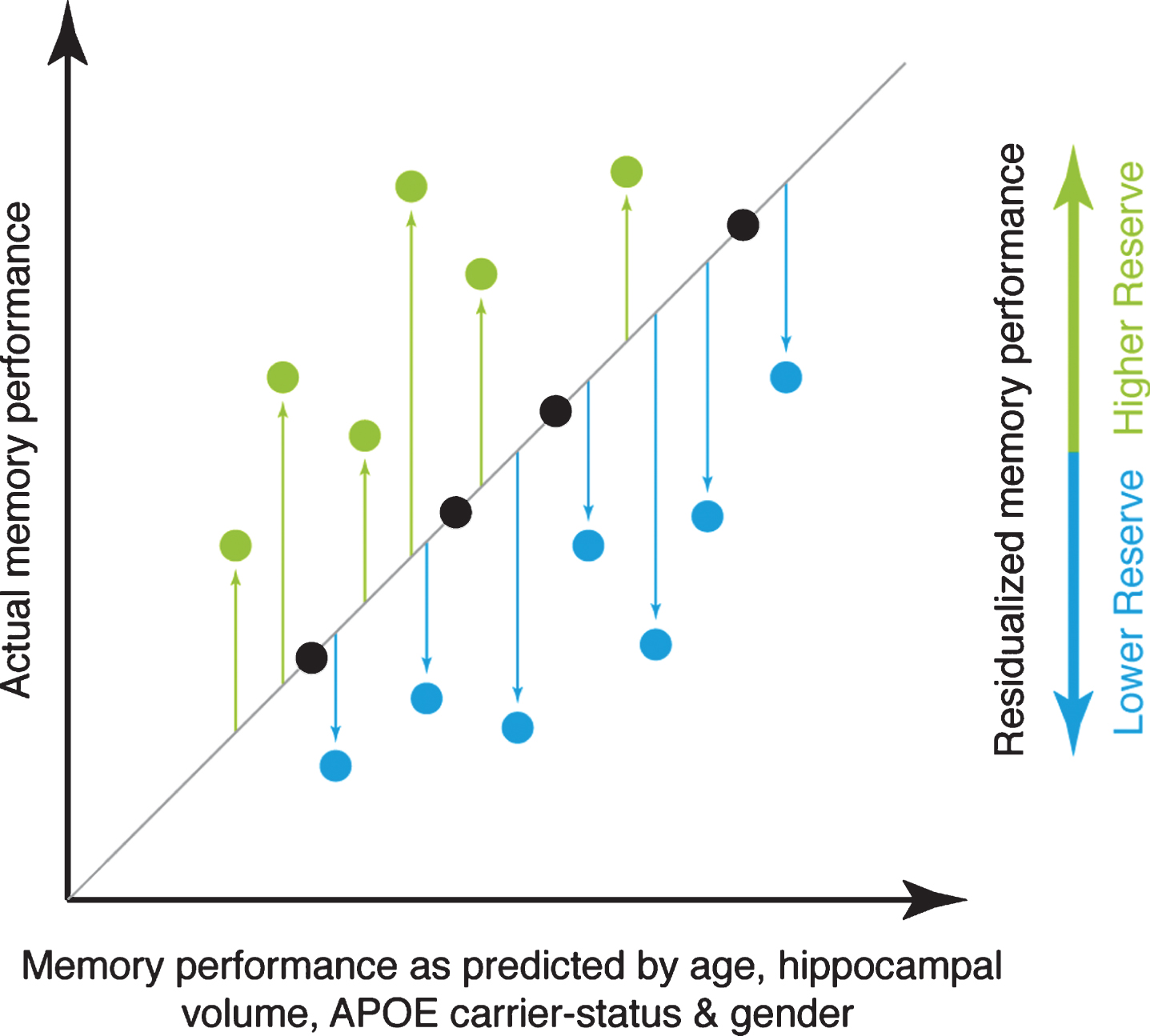
Next, we assessed the spatial pattern of condition-specific LFC-connectivity during successful encoding and successful recognition. To this end, we applied voxel-wise one-sample t-tests >0 to the PPI derived contrasts of successful encoding > 0 and successful recognition > 0, again applying a voxel threshold of α= 0.001, FWE cluster corrected at α= 0.05, controlling for age, gender, fMRI task accuracy and voxel-wise grey matter. Subsequently, for each subject the PPI-derived LFC-connectivity values were averaged across voxels that fell within clusters of significant effects and saved as a measure of task-related LFC-connectivity that was specific for successful encoding or successful recognition. This LFC-connectivity measure is related to the resting-state fMRI derived global connectivity score, which we have previously shown to support reserve in MCI [24]. To assess whether LFC-connectivity was decreased in the MCI group, we compared the levels of task-related LFC-connectivity between CN and MCI using an ANCOVA controlling for age, gender, and APOE genotype.
We next tested our first hypothesis (criterion of face validity) that greater education is associated with higher LFC-connectivity during memory processing. To this end, we used multiple regression with LFC-connectivity (successful encoding or successful recognition) as a dependent variable, and education as an independent variable, controlling for age, gender, and the APOE genotype. This analysis was conducted stratified by diagnostic group, in order to assess whether associations between education and LFC-connectivity can be consistently detected across normal (i.e., CN) and pathological (i.e., MCI) aging.
Second, we hypothesized that higher task-related LFC-connectivity is associated with greater cognitive reserve in the memory domain (criterion of cognitive benefit). Again, this analysis was stratified by diagnostic group. Specifically, we tested our hypothesis that higher LFC-connectivity predicted higher memory reserve, by applying linear regression using the residualized memory performance measure as a dependent variable and LFC-connectivity as anindependent variable.
All voxel-wise analyses reported in this manuscript were restricted to a group specific GM mask. Multiple linear regression models were computed using R statistical software and regression weights are reported as standardized beta values [45]. Linear model assumptions (skewness, kurtosis, heteroscedasticity, multicolinearity) were tested using the gvlma function in R. For all models reported, no significant violations of linear model assumptions were found.
RESULTS
Baseline demographics of the current sample are presented in Table 1. fMRI task accuracy was correlated with word list learning scores from the CERAD-NP-Plus battery in CN (r = 0.37, p = 0.044) but not in MCI (r = 0.07, p = 0.787).
Table 1
Sample demographics and neuropsychological characteristics
CN | MCI | p-value | |
(n = 37) | (n = 17) | ||
Age | 72.33±5.77 | 73.4±6.66 | 0.505 |
Gender (male/female) | 12/25 | 6/11 | 0.836 |
Education, y | 13.51±3.02 | 13.82±4.1 | 0.850 |
APOE genotype (ɛ4/other) | 12/25 | 9/8 | 0.151 |
fMRI task: Reaction Time [ms] | 2,254.39±316.09 | 2,507.98±500.10 | <0.001 |
fMRI task: Accuracy [% ] | 0.79±0.06 | 0.63±0.11 | <0.001 |
MMSE score | 29.32±0.91 | 28.35±2.4 | 0.120 |
Memory-related brain activation
In a first step, we assessed brain activation that was specific for successful memory performance. For the GLM contrast successful encoding > incorrect encoding, brain activation was found predominantly in left frontotemporal and parietal brain areas (see Fig. 2A; for detailed cluster statistics, see Supplementary Table 2). For the contrast, successful recognition > incorrect recognition, significant brain activation was found bilaterally in the mediofrontal, posterior-cingulate, occipital and inferior temporal cortex, and hippocampus (see Fig. 2B, for detailed cluster statistics Supplementary Table 3). When assessing voxel-wise group differences using ANCOVAS, we did not detect brain activation differences between MCI and CN.
Fig.2
Brain areas that showed significant activation in the GLM analysis of the fMRI memory task. Depicted are clusters where brain activation was significantly greater during successful than incorrect encoding (A) or recognition (B) at a voxel threshold of α= 0.001 and a FWE corrected cluster threshold at α= 0.05.
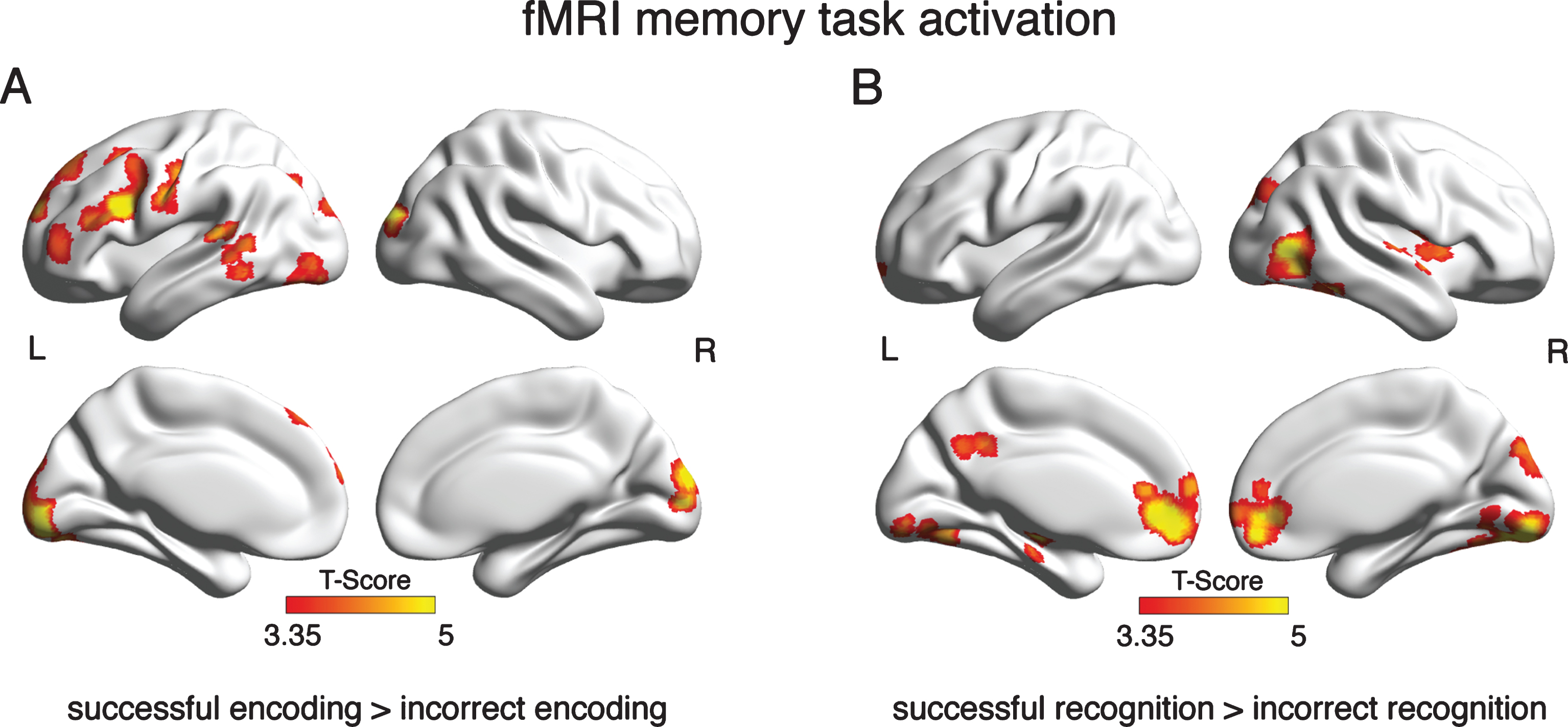
Brain activation during episodic memory is not related to education or residualized memory
We subsequently assessed whether more years of education were related to greater brain activation; however, we found no significant clusters in both CN and MCI. This suggests that greater education is not related to higher memory-related brain activation. We further tested whether the mean activation of the LFC-ROI during memory task fMRI (i.e., successful recognition > incorrect recognition and successful encoding > incorrect encoding) was related to higher residualized memory; however, no association was detected for CN and MCI.
Greater education is associated with increased task-related LFC-connectivity
We found the LFC to exhibit widespread connectivity during both successful encoding (Fig. 3A) and successful recognition (Fig. 3B), which is in agreement with the notion that the LFC is a globally connected functional hub [23, 24]. When comparing between groups the level of task-related LFC-connectivity, i.e., connectivity averaged across voxels showing significant LFC-connectivity (Fig. 3A,B), we did not detect significant group differences, neither for LFC-connectivity during successful encoding (Fig. 4A) nor during successful recognition (Fig. 4B). These findings suggest that task-related LFC-connectivity is not pathologically altered in the course of MCI.
Fig.3
Results of the gPPI analysis and associations between LFC-connectivity and education. Shown in the upper panel are clusters of significant connectivity of the LFC (blue ROI) during successful encoding (A) and successful recognition (B) as assessed by voxel-wise t-tests against zero, applying a voxel threshold of α= 0.001 and a FWE-corrected cluster threshold of α= 0.05. Depicted in the lower panels is the association between years of education and LFC-connectivity (i.e., connectivity averaged across significant voxels shown in the upper panel) for CN (C, D) and MCI (E, F).
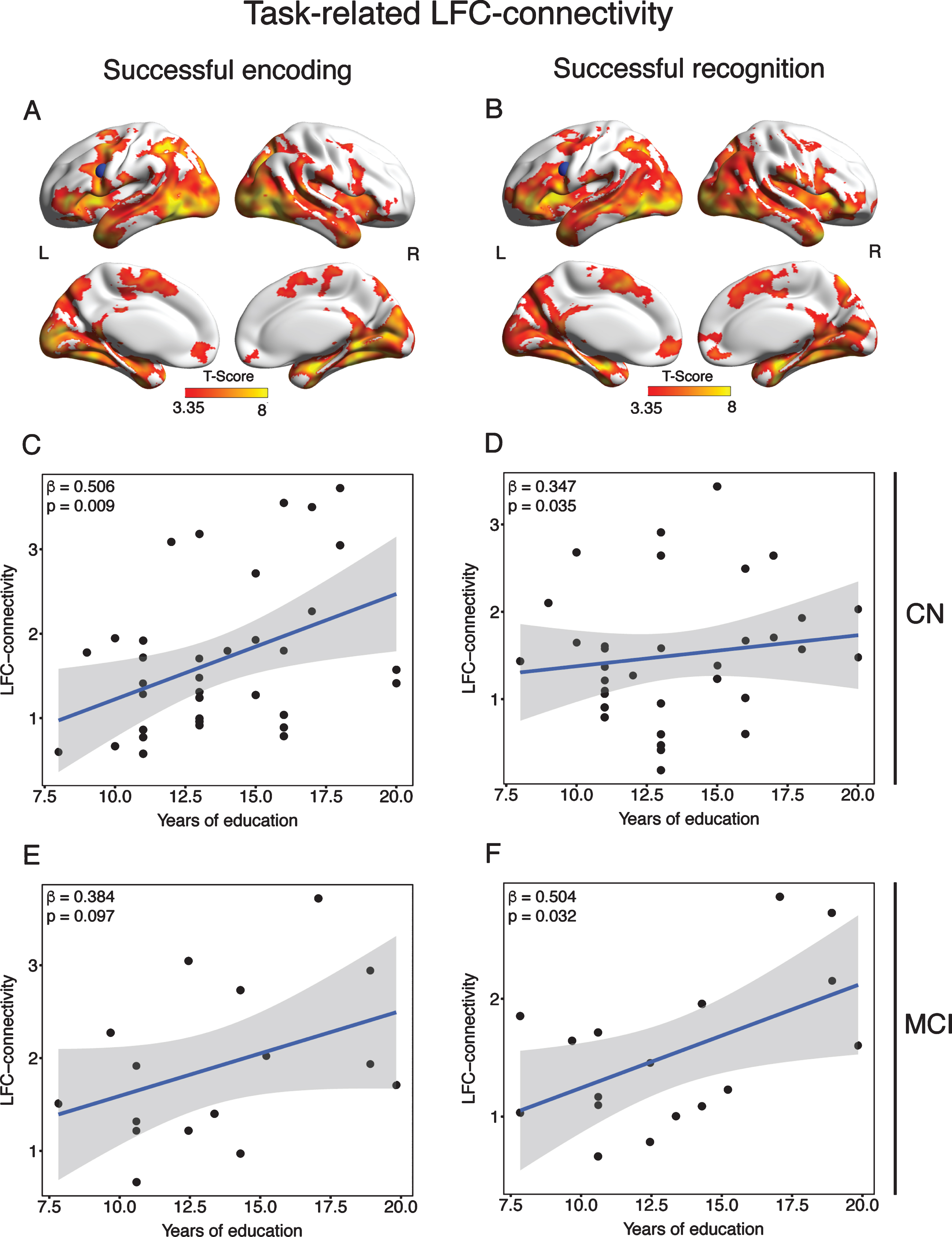
Fig.4
Boxplots illustrating mean task-related LFC-connectivity across diagnostic groups for successful encoding (A) and successful recognition (B).
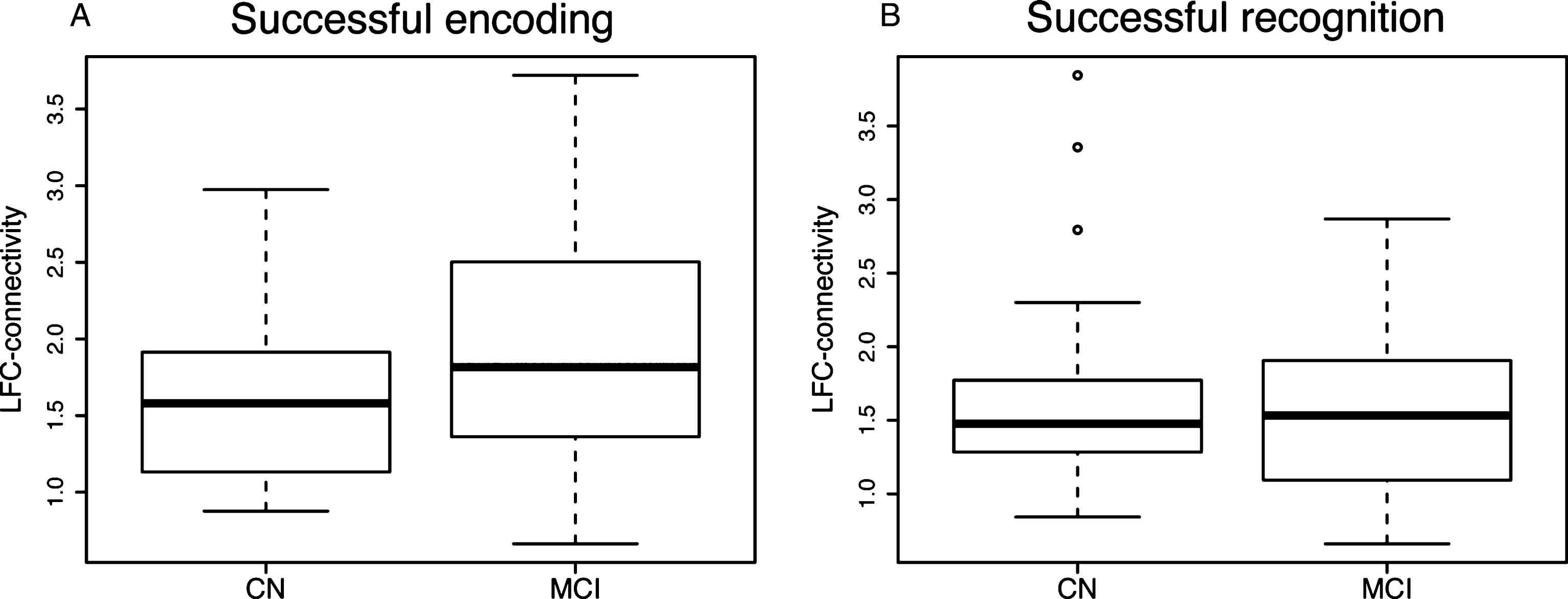
We next tested our first hypothesis (i.e., criterion of face validity) that greater education is associated with greater task-related LFC-connectivity, stratified by diagnostic group. Supporting our hypothesis, we found greater education to predict higher LFC-connectivity during successful encoding (β= 0.506, p = 0.009; Fig. 3C) and successful recognition (β= 0.347, p = 0.035; Fig. 3D) in the CN group. When tested in the MCI group, we found a trend-level association between greater education and higher LFC-connectivity during successful encoding (β= 0.384, p = 0.097; Fig. 3E) and a significant association for education and successful recognition (β= 0.504, p = 0.032; Fig. 3F).
In contrast, greater education was not significantly associated with higher task-related connectivity of two control ROIs (occipital pole and precuneus), neither in CN nor in MCI, suggesting specificity of the current findings for the LFC.
Greater task-related LFC-connectivity is associated with higher residualized memory
Next, we tested our second hypothesis (i.e., criterion of cognitive benefit), that greater task-related LFC-connectivity was associated with higher reserve in the memory domain, i.e., residualized memory task performance after accounting for effects of age, hippocampal atrophy, APOE genotype, gender, and mean fMRI-task reaction time (illustrated in Fig. 1). Supporting our hypothesis, higher residualized fMRI-task performance was associated with higher LFC-connectivity during both successful encoding (β= 0.294, p = 0.041; Fig. 5A) and successful recognition (β= 0.402, p = 0.008; Fig. 5B) in the CN group. Similarly, in the MCI group, higher residualized fMRI-task performance was associated with higher LFC-connectivity during successful encoding (β= 0.526, p = 0.037; Fig. 5C) but not during successful recognition (β= 0.246, p = 0.198; Fig. 5D). Together, these findings support the hypothesis that higher LFC-connectivity is associated with higher reserve in the memory domain across normal and pathological aging.
Fig.5
Scatterplots illustrating the association between LFC-connectivity during successful encoding (left panels) or recognition (right panels) on the x-axis and residualized memory performance on the y-axis for CN (upper panels) and MCI (lower panels).
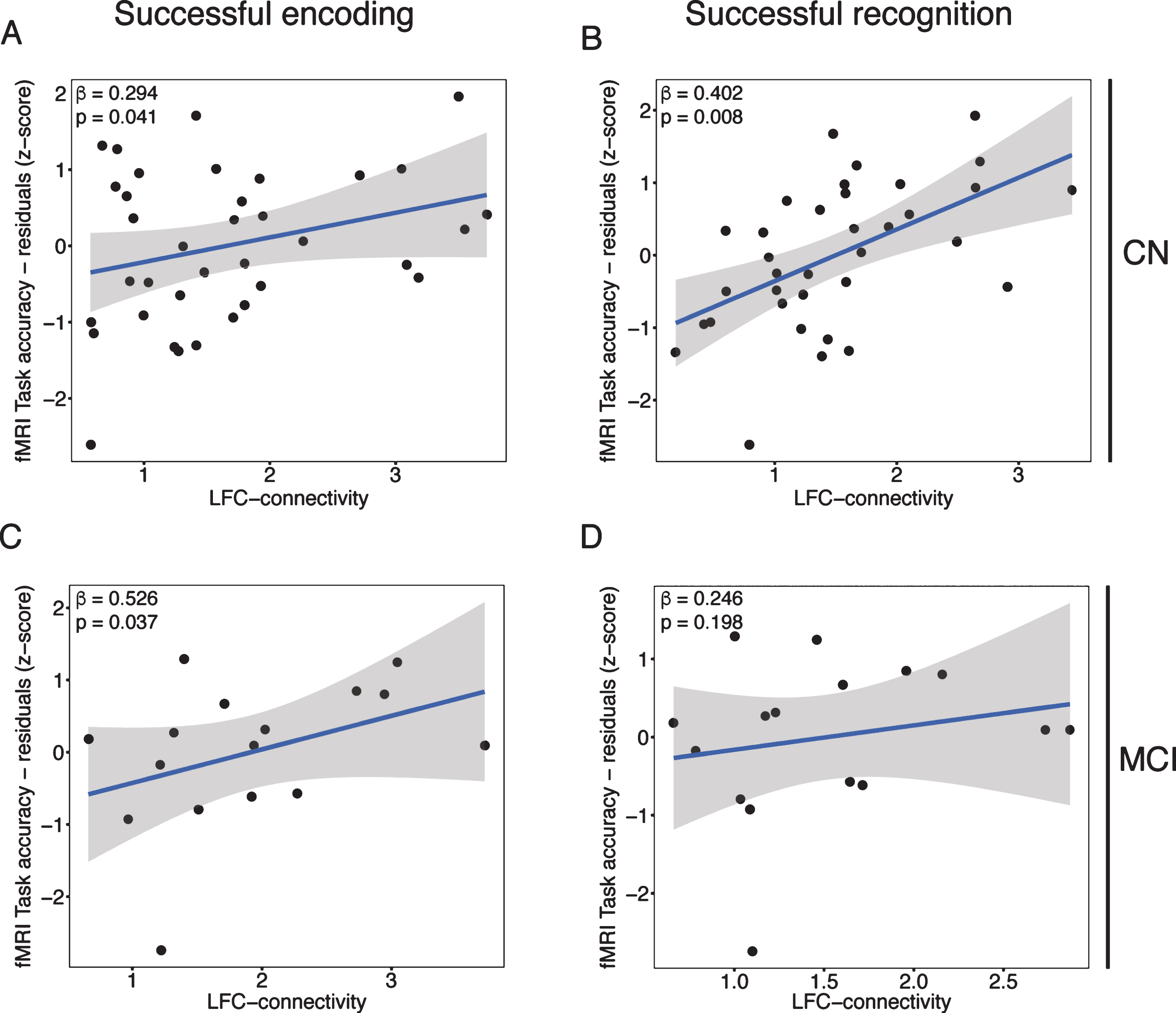
When restricting this analysis to LFC-connectivity to those brain regions that were specifically activated during successful encoding and successful recognition (as shown in Fig. 2), we obtained a similar result pattern but less strong effects (successful encoding: CN, β= 0.232, p = 0.087; MCI, β= 0.484, p = 0.054; successful recognition: CN, β= 0.381, p = 0.011; MCI, β= 0.193, p = 0.257).
Again, no significant associations were found between residualized memory performance measures and connectivity of the control ROIs (occipital pole and precuneus) suggesting specificity of our findings for the LFC. All results described in this paragraph remained virtually unchanged when repeating the analyses using the residualized memory score from which we additionally regressed out the MMSE score as a measure of global cognition.
DISCUSSION
The major results of the current study showed that 1) more years of education were associated with increased LFC-connectivity during memory processing, and 2) increased LFC-connectivity was associated with higher reserve in the memory domain. This result pattern was found in both CN and MCI, suggesting that connectivity of a key hub of thefrontoparietal control network contributes to reserve in both normal and pathological aging.
The current findings confirm and extend our previous finding on resting-state fMRI assessed LFC-connectivity and reserve in MCI [24]. The current results from memory task fMRI suggest that the active engagement of the LFC hub of the frontoparietal control network during the task facilitates reserve. Reserve was quantified as the residualized memory performance which expresses the difference between the actual observed memory task performance and the performance level predicted by age, brain atrophy, and individual AD risk [25, 46]. Although such a residualized measure may also reflect in part a random prediction error of memory, previous studies have shown higher residualized memory scores to be predictive of slower longitudinal cognitive decline, lower incident dementia and attenuated impact of neurodegeneration on cognition [25–27], suggesting the residualized memory a meaningful measure of reserve. Higher LFC-connectivity both during rest [24] and memory task was associated with higher education, a protective factor associated with lower risk of dementia [47]. Interestingly, higher resting-state LFC-connectivity was previously found to be associated with higher IQ, i.e., another protective factor in aging, and cognitive performance in younger adults [23]. Together these results suggest that a higher LFC-connectivity may be a long-lasting trait susceptible to environmental influences such as education, consistent with the idea of cognitive reserve as a capacity that is modifiable during life [14]. Note that in the current study the levels of task-related LFC-connectivity were similar when compared between MCI and CN, again suggesting that higher LFC-connectivity was not a compensatory change triggered by pathological neurodegeneration but rather reflects a stable brain feature that confers higher resilience in normal and pathologicalaging.
The LFC is a brain region particularly well-suited to subserve reserve. First, the LFC (BA 6/44) ranks among the top 5% of brain regions in terms of number of connections in the brain [38, 39] and is a key connector hub between different functional networks [22]. As a hub of the frontoparietal control network, the LFC exhibits widespread multi-task connectivity across different cognitive domains, and may thus be a key region to sustain cognition [48]. The frontoparietal control network has been proposed to play a vital role in mental health [49] and has recently been proposed to be associated with higher reserve capacity [19]. The current results are in line with these notions.
Note that education was associated with higher LFC-connectivity but not with the strength of brain activation, neither in the LFC nor in any other region. This is partially in conflict with studies reporting an association between education or lifestyle factors and brain activation during task [50, 51]. However, so far no consistent association between education or other protective factors and a specific regional pattern of task-related activation could be established [52]. A possible explanation is that the amplitude of activation is highly variable and may be majorly driven by task demands and transient states of learning, whereas the intrinsic brain architecture as revealed by functional connectivity is more closely correlated with stable traits [53, 54] and general cognitive capacity [23]. Functional connectivity of the frontoparietal control network was found to be highly stable across time [55], where global connectivity within that network may serve as a robust marker of higher reserve in MCI [39].
For the interpretation of the current findings some caveats should be considered. Firstly, we employed a hypothesis-driven approach focusing on the LFC, which has the advantage to limit the risk of random false positive findings. This comes at the cost of potentially missing relevant connectivity changes in brain areas other than the LFC. However, we analyzed control regions including the occipital pole (harboring primary sensory areas) and the precuneus (as a region of multimodal associations and higher cognitive abilities including memory), where no association with education or residualized memory performance was observed. This supports the specificity of our findings for the LFC; however, a role of other brain areas in reserve cannot be excluded and may be systematically assessed by future studies. It should also be considered that our sample of CN subjects included carriers of the ApoE ɛ4 allele, which increases the likelihood of incipient AD pathology. To account for this, we have included the APOE genotype in the computation of the residualized memory score, so that genetic AD risk is considered when estimating individual reserve levels.
In conclusion, education, i.e., a protective factor that is associated with a lower dementia risk, may be specifically associated with LFC-connectivity which in turn may underlie increased reserve in late-life that helps to maintain memory performance relatively well in normal and pathological aging. The current results encourage testing the modifiability of LFC-connectivity as a potential therapeutic intervention. Transcranial direct-current stimulation (tDCS) [56, 57], or cognitive training [58, 59] may increase cognitive performance and frontal lobe function in normal aging and MCI. Connectivity of the LFC may thus provide an attractive therapeutic target as well as a read out in drug or cognitive intervention trials.
ACKNOWLEDGMENTS
The study was funded by an ERC career integration grant (PCIG12-GA-2012-334259 to ME), LMUexcellent (to ME), and Alzheimer Forschung Initiative (to MD and ME).
Authors’ disclosures available online (http://j-alz.com/manuscript-disclosures/17-0360r1).
SUPPLEMENTARY MATERIAL
[1] The supplementary material is available in the electronic version of this article: http://dx.doi.org/10.3233/JAD-170360.
REFERENCES
[1] | Katzman R , Terry R , DeTeresa R , Brown T , Davies P , Fuld P , Renbing X , Peck A ((1988) ) Clinical, pathological, and neurochemical changes in dementia: A subgroup with preserved mental status and numerous neocortical plaques. Ann Neurol 23: , 138–144. |
[2] | Perneczky R , Wagenpfeil S , Lunetta KL , Cupples LA , Green RC , Decarli C , Farrer LA , Kurz A ((2010) ) Head circumference, atrophy, and cognition: Implications for brain reserve in Alzheimer disease. Neurology 75: , 137–142. |
[3] | Guo LH , Alexopoulos P , Wagenpfeil S , Kurz A , Perneczky R , Alzheimer’s Disease Neuroimaging Initiative ((2013) ) Brain size and the compensation of Alzheimer’s disease symptoms: A longitudinal cohort study. Alzheimers Dement 9: , 580–586. |
[4] | Negash S , Xie S , Davatzikos C , Clark CM , Trojanowski JQ , Shaw LM , Wolk DA , Arnold SE ((2013) ) Cognitive and functional resilience despite molecular evidence of Alzheimer’s disease pathology. Alzheimers Dement 9: , e89–e95. |
[5] | Gaser C , Franke K , Kloppel S , Koutsouleris N , Sauer H , Alzheimer’s Disease Neuroimaging Initiative ((2013) ) BrainAGE in mild cognitive impaired patients: Predicting the conversion to Alzheimer’s disease. PLoS One 8: , e67346. |
[6] | Dickerson BC , Salat DH , Greve DN , Chua EF , Rand-Giovannetti E , Rentz DM , Bertram L , Mullin K , Tanzi RE , Blacker D , Albert MS , Sperling RA ((2005) ) Increased hippocampal activation in mild cognitive impairment compared to normal aging and AD. Neurology 65: , 404–411. |
[7] | Huijbers W , Mormino EC , Schultz AP , Wigman S , Ward AM , Larvie M , Amariglio RE , Marshall GA , Rentz DM , Johnson KA , Sperling RA ((2015) ) Amyloid-beta deposition in mild cognitive impairment is associated with increased hippocampal activity, atrophy and clinical progression. Brain 138: , 1023–1035. |
[8] | Huijbers W , Mormino EC , Wigman SE , Ward AM , Vannini P , McLaren DG , Becker JA , Schultz AP , Hedden T , Johnson KA , Sperling RA ((2014) ) Amyloid deposition is linked to aberrant entorhinal activity among cognitively normal older adults. J Neurosci 34: , 5200–5210. |
[9] | Morbelli S , Perneczky R , Drzezga A , Frisoni GB , Caroli A , van Berckel BN , Ossenkoppele R , Guedj E , Didic M , Brugnolo A , Naseri M , Sambuceti G , Pagani M , Nobili F ((2013) ) Metabolic networks underlying cognitive reserve in prodromal Alzheimer disease: A European Alzheimer disease consortium project. J Nucl Med 54: , 894–902. |
[10] | Zhang Y , Simon-Vermot L , Araque Caballero MA , Gesierich B , Taylor AN , Duering M , Dichgans M , Ewers M , Alzheimer’s Disease Neuroimaging Initiative ((2016) ) Enhanced resting-state functional connectivity between core memory-task activation peaks is associated with memory impairment in MCI. Neurobiol Aging 45: , 43–49. |
[11] | Sperling RA , Dickerson BC , Pihlajamaki M , Vannini P , LaViolette PS , Vitolo OV , Hedden T , Becker JA , Rentz DM , Selkoe DJ , Johnson KA ((2010) ) Functional alterations in memory networks in early Alzheimer’s disease. Neuromolecular Med 12: , 27–43. |
[12] | Hillary FG , Grafman JH ((2017) ) Injured brains and adaptive networks: The benefits and costs of hyperconnectivity. Trends Cogn Sci 21: , 385–401. |
[13] | Bakker A , Krauss GL , Albert MS , Speck CL , Jones LR , Stark CE , Yassa MA , Bassett SS , Shelton AL , Gallagher M ((2012) ) Reduction of hippocampal hyperactivity improves cognition in amnestic mild cognitive impairment. Neuron 74: , 467–474. |
[14] | Stern Y ((2012) ) Cognitive reserve in ageing and Alzheimer’s disease. Lancet Neurol 11: , 1006–1012. |
[15] | Bosch B , Bartrés-Faz D , Rami L , Arenaza-Urquijo EM , Fernández-Espejo D , Junqué C , Solé-Padullés C , Sánchez-Valle R , Bargalló N , Falcón C , Molinuevo JL ((2009) ) Cognitive reserve modulates task-induced activations and deactivations in healthy elders, amnestic mild cognitive impairment and mild Alzheimer’s disease. Cortex 46: , 451–461. |
[16] | Solé-Padullés C , Bartrés-Faz D , Junqué C , Vendrell P , Rami L , Clemente IC , Bosch B , Villar A , Bargalló N , Jurado MA , Barrios M , Molinuevo JL ((2009) ) Brain structure and function related to cognitive reserve variables in normal aging, mild cognitive impairment and Alzheimer’s disease. Neurobiol Aging 30: , 1114–1124. |
[17] | Stern Y , Zarahn E , Habeck C , Holtzer R , Rakitin BC , Kumar A , Flynn J , Steffener J , Brown T ((2008) ) A common neural network for cognitive reserve in verbal and object working memory in young but not old. Cerebral Cortex 18: , 959–967. |
[18] | Bartres-Faz D , Sole-Padulles C , Junque C , Rami L , Bosch B , Bargallo N , Falcon C , Sanchez-Valle R , Molinuevo JL ((2009) ) Interactions of cognitive reserve with regional brain anatomy and brain function during a working memory task in healthy elders. Biol Psychol 80: , 256–259. |
[19] | Medaglia JD , Pasqualetti F , Hamilton RH , Thompson-Schill SL , Bassett DS ((2017) ) Brain and cognitive reserve: Translation via network control theory. Neurosci Biobehav Rev 75: , 53–64. |
[20] | Arenaza-Urquijo EM , Landeau B , La Joie R , Mevel K , Mezenge F , Perrotin A , Desgranges B , Bartres-Faz D , Eustache F , Chetelat G ((2013) ) Relationships between years of education and gray matter volume, metabolism and functional connectivity in healthy elders. Neuroimage 83: , 450–457. |
[21] | Santarnecchi E , Rossi S , Rossi A ((2015) ) The smarter, the stronger: Intelligence level correlates with brain resilience to systematic insults. Cortex 64: , 293–309. |
[22] | Cole MW , Ito T , Braver TS ((2015) ) Lateral prefrontal cortex contributes to fluid intelligence through multinetwork connectivity. Brain Connect 5: , 497–504. |
[23] | Cole MW , Yarkoni T , Repovs G , Anticevic A , Braver TS ((2012) ) Global connectivity of prefrontal cortex predicts cognitive control and intelligence. J Neurosci 32: , 8988–8999. |
[24] | Franzmeier N , Duering M , Weiner M , Dichgans M , Ewers M , Alzheimer’s Disease Neuroimaging Initiative ((2017) ) Left frontal cortex connectivity underlies cognitive reserve in prodromal Alzheimer disease. Neurology 88: , 1054–1061. |
[25] | Reed BR , Mungas D , Farias ST , Harvey D , Beckett L , Widaman K , Hinton L , DeCarli C ((2010) ) Measuring cognitive reserve based on the decomposition of episodic memory variance. Brain 133: , 2196–2209. |
[26] | Zahodne LB , Manly JJ , Brickman AM , Narkhede A , Griffith EY , Guzman VA , Schupf N , Stern Y ((2015) ) Is residual memory variance a valid method for quantifying cognitive reserve? A longitudinal application. Neuropsychologia 77: , 260–266. |
[27] | Zahodne LB , Manly JJ , Brickman AM , Siedlecki KL , Decarli C , Stern Y ((2013) ) Quantifying cognitive reserve in older adults by decomposing episodic memory variance: Replication and extension. J Int Neuropsychol Soc 19: , 854–862. |
[28] | Schmid NS , Ehrensperger MM , Berres M , Beck IR , Monsch AU ((2014) ) The extension of the German CERAD neuropsychological assessment battery with tests assessing subcortical, executive and frontal functions improves accuracy in dementia diagnosis. Dement Geriatr Cogn Dis Extra 4: , 322–334. |
[29] | Luck T , Riedel-Heller S , Wiese B ((2009) ) CERAD-NP-Testbatterie: Alters-, geschlechts- und bildungsspezifischen Normen ausgewählter Subtests. Z Gerontol Geriatr 42: , 372–384. |
[30] | Petersen RC , Caracciolo B , Brayne C , Gauthier S , Jelic V , Fratiglioni L ((2014) ) Mild cognitive impairment: A concept in evolution. J Intern Med 275: , 214–228. |
[31] | Celone KA , Calhoun VD , Dickerson BC , Atri A , Chua EF , Miller SL , DePeau K , Rentz DM , Selkoe DJ , Blacker D , Albert MS , Sperling RA ((2006) ) Alterations in memory networks in mild cognitive impairment and Alzheimer’s disease: An independent component analysis. J Neurosci 26: , 10222–10231. |
[32] | Pihlajamaki M , Sperling RA ((2009) ) Functional MRI assessment of task-induced deactivation of the default mode network in Alzheimer’s disease and at-risk older individuals. Behav Neurol 21: , 77–91. |
[33] | Ashburner J ((2007) ) A fast diffeomorphic image registration algorithm. Neuroimage 38: , 95–113. |
[34] | Petersen RC , Jack CR Jr , Xu YC , Waring SC , O’Brien PC , Smith GE , Ivnik RJ , Tangalos EG , Boeve BF , Kokmen E ((2000) ) Memory and MRI-based hippocampal volumes in aging and AD. Neurology 54: , 581–587. |
[35] | Mak HK , Zhang Z , Yau KK , Zhang L , Chan Q , Chu LW ((2011) ) Efficacy of voxel-based morphometry with DARTEL and standard registration as imaging biomarkers in Alzheimer’s disease patients and cognitively normal older adults at 3.0 Tesla MR imaging. J Alzheimers Dis 23: , 655–664. |
[36] | Tzourio-Mazoyer N , Landeau B , Papathanassiou D , Crivello F , Etard O , Delcroix N , Mazoyer B , Joliot M ((2002) ) Automated anatomical labeling of activations in SPM using a macroscopic anatomical parcellation of the MNI MRI single-subject brain. Neuroimage 15: , 273–289. |
[37] | Huijbers W , Vannini P , Sperling RA , Pennartz CM , Cabeza R , Daselaar SM ((2012) ) Explaining the encoding/retrieval flip: Memory-related deactivations and activations in the posteromedial cortex. Neuropsychologia 50: , 3764–3774. |
[38] | Cole MW , Pathak S , Schneider W ((2010) ) Identifying the brain’s most globally connected regions. Neuroimage 49: , 3132–3148. |
[39] | Franzmeier N , Caballero MÁA , Taylor ANW , Simon-Vermot L , Buerger K , Ertl-Wagner B , Mueller C , Catak C , Janowitz D , Baykara E , Gesierich B , Duering M , Ewers M , Alzheimer’s Disease Neuroimaging Initiative ((2016) ) Resting-state global functional connectivity as a biomarker of cognitive reserve in mild cognitive impairment. Brain Imaging Behav 1–15. |
[40] | McLaren DG , Ries ML , Xu G , Johnson SC ((2012) ) A generalized form of context-dependent psychophysiological interactions (gPPI): A comparison to standard approaches. Neuroimage 61: , 1277–1286. |
[41] | Gitelman DR , Penny WD , Ashburner J , Friston KJ ((2003) ) Modeling regional and psychophysiologic interactions in fMRI: The importance of hemodynamic deconvolution. Neuroimage 19: , 200–207. |
[42] | O’Reilly JX , Woolrich MW , Behrens TE , Smith SM , Johansen-Berg H ((2012) ) Tools of the trade: Psychophysiological interactions and functional connectivity. Soc Cogn Affect Neurosci 7: , 604–609. |
[43] | Yang X , Beason-Held L , Resnick SM , Landman BA ((2011) ) Robust biological parametric mapping: An improved technique for multimodal brain image analysis. Proc SPIE Int Soc Opt Eng 7962: , 79623X. |
[44] | Serra L , Bruschini M , Di Domenico C , Gabrielli GB , Marra C , Caltagirone C , Cercignani M , Bozzali M ((2017) ) Memory is not enough: The neurobiological substrates of dynamic cognitive reserve. J Alzheimers Dis 58: , 171–184. |
[45] | R Development Core Team ((2013) ) R Foundation for Statistical Computing, Vienna, Austria. |
[46] | Jones RN , Manly J , Glymour MM , Rentz DM , Jefferson AL , Stern Y ((2011) ) Conceptual and measurement challenges in research on cognitive reserve. J Int Neuropsychol Soc 17: , 593–601. |
[47] | Valenzuela MJ , Sachdev P ((2006) ) Brain reserve and dementia: A systematic review. Psychol Med 36: , 441–454. |
[48] | Cole MW , Reynolds JR , Power JD , Repovs G , Anticevic A , Braver TS ((2013) ) Multi-task connectivity reveals flexible hubs for adaptive task control. Nat Neurosci 16: , 1348–1355. |
[49] | Cole MW , Repovs G , Anticevic A ((2014) ) The frontoparietal control system: A central role in mental health. Neuroscientist 20: , 652–664. |
[50] | Fernandez-Cabello S , Valls-Pedret C , Schurz M , Vidal-Pineiro D , Sala-Llonch R , Bargallo N , Ros E , Bartres-Faz D ((2016) ) White matter hyperintensities and cognitive reserve during a working memory task: A functional magnetic resonance imaging study in cognitively normal older adults. Neurobiol Aging 48: , 23–33. |
[51] | Bosch B , Bartres-Faz D , Rami L , Arenaza-Urquijo EM , Fernandez-Espejo D , Junque C , Sole-Padulles C , Sanchez-Valle R , Bargallo N , Falcon C , Molinuevo JL ((2010) ) Cognitive reserve modulates task-induced activations and deactivations in healthy elders, amnestic mild cognitive impairment and mild Alzheimer’s disease. Cortex 46: , 451–461. |
[52] | Barulli D , Stern Y ((2013) ) Efficiency, capacity, compensation, maintenance, plasticity: Emerging concepts in cognitive reserve. Trends Cogn Sci 17: , 502–509. |
[53] | Mueller S , Wang D , Fox MD , Yeo BT , Sepulcre J , Sabuncu MR , Shafee R , Lu J , Liu H ((2013) ) Individual variability in functional connectivity architecture of the human brain. Neuron 77: , 586–595. |
[54] | Finn ES , Shen X , Scheinost D , Rosenberg MD , Huang J , Chun MM , Papademetris X , Constable RT ((2015) ) Functional connectome fingerprinting: Identifying individuals using patterns of brain connectivity. Nat Neurosci 18: , 1664–1671. |
[55] | Choe AS , Jones CK , Joel SE , Muschelli J , Belegu V , Caffo BS , Lindquist MA , van Zijl PC , Pekar JJ ((2015) ) Reproducibility and temporal structure in weekly resting-state fMRI over a period of 3.5 years. PLoS One 10: , e0140134. |
[56] | Hsu WY , Ku Y , Zanto TP , Gazzaley A ((2015) ) Effects of noninvasive brain stimulation on cognitive function in healthy aging and Alzheimer’s disease: A systematic review and meta-analysis. Neurobiol Aging 36: , 2348–2359. |
[57] | Sole-Padulles C , Bartres-Faz D , Junque C , Clemente IC , Molinuevo JL , Bargallo N , Sanchez-Aldeguer J , Bosch B , Falcon C , Valls-Sole J ((2006) ) Repetitive transcranial magnetic stimulation effects on brain function and cognition among elders with memory dysfunction. A randomized sham-controlled study. Cereb Cortex 16: , 1487–1493. |
[58] | Belleville S , Clement F , Mellah S , Gilbert B , Fontaine F , Gauthier S ((2011) ) Training-related brain plasticity in subjects at risk of developing Alzheimer’s disease. Brain 134: , 1623–1634. |
[59] | Franzmeier N , Unterauer E , Ewers M , Düring M , Müller C , Ruiescu D , Ertl-Wagner B , Teipel S , Fuchs C , Coloma Andrews L , Dichgans M , Bürger K ((2016) ) Effects of age, APOE ɛ4, cognitive reserve and hippocampal volume on cognitive intervention outcome in amnestic mild cognitive impairment. J Alzheimers Dis Parkinsonism 6: . doi: 10.4172/2161-0460.1000246 |