An Academic Publishers’ GO FAIR Implementation Network (APIN)
Abstract
Presented here is a proposal for the academic publishing industry to get actively involved in the formulation of protocols and standards that make published scientific research material machine-readable in order to facilitate data to be findable, accessible, interoperable, and re-usable (FAIR). Given the importance of traditional journal publications in scholarly communication worldwide, active involvement of academic publishers in advancing the more routine creation and reuse of FAIR data is highly desired.
1.Academic publishing since the emergence of the Web
The proposals in this article must be seen in the context of developments in academic publishing and further trends we can foresee. The scholarly publishing environment has changed dramatically in the last 25 years. The change was precipitated by the—quite sudden—emergence of the World Wide Web (commonly just called ‘the Web’), the common system for navigating the Internet. The idea that the Web would make the postal service irrelevant for sharing scientific information was suddenly not whimsical anymore, even though in the early days, it seemed a bit of an exaggeration. All information, and therefore also scholarly, scientific information, could be distributed and shared electronically. Its reach was henceforth only limited to access to the Internet itself. And it was not just the potential of worldwide reach that was made possible, it was also the high speed—well-nigh instantaneous—with which information could be delivered. The end of the role of print was in sight. ‘Paper’ took on a different, virtual, meaning, albeit that ‘papers’ were, and are, often enough still printed, though increasingly by the recipient of the electronic version and not in a central printer facility and bound in issues. ‘Print’ itself survived as a concept, now virtual, especially in words like ‘preprint’. With printed journal issues disappearing, postal charges, not an insignificant part of subscription costs, and a significant source of profit for at least some publishers, who used to charge more than the actual postage cost, vanished as well. The Internet, and the Web technology riding on it, also made it possible to distribute scholarly material to a vastly larger audience than had been the case with printed issues, as the marginal cost per copy became an irrelevance and disappeared, to all intents and purposes.
The Web made it possible, at least in principle, to distribute the results of scientific research more widely, more equitably. The one impediment that remained – for Academia – was the cost of access. The technology for distribution changed very rapidly, from print on paper to electronic, but the business model of publishers defraying their costs and making money via subscription charges, proved very resilient. The need to cover costs is inevitable (though efficiency gains could possibly reduce those costs), but it was particularly the desire – especially by the larger commercial publishers – to preserve profit margins that made new business models unpopular. A desire among researchers for open access, whereby the reader would not be confronted with paywalls and costs, grew. For a long time, many publishers resisted. A business model that would deliver open access, would also imply that income could not be realised by charging the reader. Consequently, the author should carry all the cost, or some other way should be found to secure income at the input side of the publishing process. Publishing would have to become a business providing services to scientists in their role as authors rather than in their role as readers. Charges would have to be levied for actual services on an article-by-article basis, and not for the availability “just-in-case-it-is-needed” to bundles of articles in journals. In such models, a ‘journal’ would become a ‘label’ attached to an article, and not the bundle of articles bound into issues known from traditional publishing. The convenient opaqueness of the subscription model, in which costs could be allocated, made pricing a fine art (a gamble at the beginning of a new journal, and an estimate to ensure gross revenue preservation once a journal became established – juggling factors such as exchange rate fluctuations, growth- or attrition rates of the number of subscribers, increase or decrease in submissions and acceptance rates, et cetera). Instead, costs and profit of an open access publishing environment would have to be covered by what became known as ‘Article Processing Charges’ (APCs), which are inherently less suitable to being kept opaque, so the pressure on being transparent was – and still is – increasing. Consequently, the potential for downward pressure on the ‘profit’ part of the equation could well become high.
2.Changing role of publishers
There is more: for decades now, authors’ manuscripts have almost universally been prepared electronically, and the publishers’ role was more or less reduced to arranging peer-review for, and some formatting of, the ‘version of record’ of articles. Of course, sometimes there was some copy-editing, too, but the stories of copy-editing being inadequate or even introducing errors that were not in the manuscript that authors submitted, are not exceptional. The example of the German sz-ligature, ß, being used by authors for the Greek beta, 𝛽, or this inappropriate substitution not being spotted and corrected by copy-editors, is illustrative. In print, the ß/𝛽 issue may not matter much, as the human eye reads what it expects: ß-carotene looks pretty similar to 𝛽-carotene on paper. Electronic versions change that, of course. If read by a machine, the Unicode for ß (U+00DF) is quite different to the one for 𝛽 (U+03B2). It matters, if articles have to be processed and analysed with the assistance of machines, which is increasingly necessary as a result of the overwhelming numbers of articles that are being published (for example the flood of Covid-19 articles which were being published at the time this article was being written [1]). It also means that copy-editing, if done well, remains useful, certainly as long as authors aren’t always as diligent as they should be when composing the text of their articles. If it weren’t for these roles, of arranging peer-review and copy-editing, scholarly communication could have easily escaped the scholarly publishing ‘ecosystem’ (or ‘ego-system’, as it is sometimes mockingly called, due to the strong association with researchers’ career and reputation enhancement consequences and effects).
3.Open access
The large-scale transition to electronic publishing made open access, as defined in the Budapest Open Access Initiative of 2002 [2] feasible, from a technical point of view. But it required new business models, to defray costs and sustain profits (for commercial publishers) and surpluses or financial buffers (for not-for profit outfits). The various options that have been explored include subsidies (mainly for not-for-profits) and input-side (author-side) payment: the APCs mentioned above. The latter means, in practice, payment by the organisations that fund the authors’ research. An initiative that coordinates funders’ policies in that regard has been launched and is known as ‘Plan S’. The model of author-side payment does, of course, present problems for authors who are not, or minimally, funded. The growth of less formal, ‘provisional’ publication, usually called ‘preprint’ posting, offers relief, as does deposition in an openly accessible repository of the final author manuscript, the version accepted by a journal, which is known as ‘green’ open access, and is also recognised by Plan S [3] as a legitimate form of providing the required open access to research the participating funding bodies have funded.
As the term ‘ego-system’ mentioned above implies, formal journal publications remain important for many – possibly most – researchers’ career prospects and reputations. Articles are ‘advertisements’, drawing attention to researchers’ scientific prowess. Calling them ‘advertisements’ was, of course, anathema, not only because of the inevitable protests from researchers, but, albeit perhaps secondarily, because of the consequences for postage rates for printed issues [4].
This remains so, at least for now.
Ironically, as data publication (as opposed to the narrative) becomes more important, the possibility of data publications delivering to the researchers the same sort of credit as articles is not (yet) there, so the article remains more important for career- and reputation enhancement. According to Tatiana Khayrullina ([email protected] – lead analyst at Outsell Inc. [5]) in a recent report (only accessible, by the way, to Outsell customers), “Data brings transparency and reproducibility to scholarly communications when it is analyzed and presented in an accessible and interactive way. Publishers that focus on making data a bona fide research artifact create a compelling reason for authors to choose their journals.”
The notion of creating – by providing services in support of FAIR data publication – a compelling reason for authors to choose a particular journal to submit their manuscripts to, should be attractive to publishers. But it should also be attractive to preprint platforms and other open repositories hosting authors’ final manuscripts, given the status given to those types of publications, not least by initiatives such as Plan S, and to provide a route to full participation in FAIR data communication to researchers with no or little funding to cover the cost of publication.
4.Motivation: Data driven
Communication of scientific research results is one of the pillars on which scientific progress stands. Traditionally, the published (narrative) literature was the main material from which to construct this pillar. It used to be so that data were supplementary to published articles, but in the last decades this relation has undergone an inversion, where these articles have increasingly become supplementary to the core research results, the data, as they describe the latter’s interpretation, provenance, and significance. Under such an inversion, we might start to refer to ‘data publication’, with ‘supplementary articles’. An article should be seen to belong to the set of ‘rich provenance metadata’ (although itself not machine readable, but enabling humans to determine actual ‘reusability’ and ‘fitness for purpose’.
Furthermore, data repositories are playing an increasingly important role in the scientific communication and discovery process, as data availability and reusability becomes a crucial element in science’s efficiency and efficacy. The old method, which relied on “data are available upon request” (or even on “all data are available from the corresponding author upon reasonable request.” [italics added for emphasis]) is not fit for purpose any longer, even when, or rather, if, data were easily available and usable that way. More and more science is also reliant on data sets that are either too large and complex to simply being made available by request, reasonable or not, from the authors following idiosyncratic methods of exchange.
In many disciplines, such as medical and psychological sciences, data are often too privacy-sensitive to be‘shared’in the classical sense. They need to be anonymised, and even then, levels of trust necessary to guarantee privacy cannot always be achieved. One solution is that data are not shared by means of making them available in the traditional way, but instead, by means of making it possible to analyse data by ‘visiting’ them in their privacy-secure silos. A good example is the ‘Personal Health Train’ [6]: The key concept here is to bring algorithms to the data where they happen to be, rather than bringing all data to a central place. The algorithms just ‘visit’ the data, as it were, which means that no data need to be duplicated or downloaded to another location. The Personal Health Train is designed to give controlled access to heterogeneous data sources, while ensuring privacy protection and maximum engagement of individual patients, citizens, and other entities with a strong interest in the protection of data privacy. The Data Train concept is a generic one and is clear that it can also be applied in areas other than health sciences. There is, for instance, also a Farm Data Train [7]. For the Data Train concept to work, data need to be FAIR. FAIR has a distinctive and precise meaning in this context. It stands for data and services that are Findable, Accessible, Interoperable and Reusable by machines [8,9].
The FAIR guiding principles for data (several academic publishers have been involved in formulating and co-authoring them) encompass, in detail:
Findability – for data to be findable requires:
F-1. assigning (meta)data a globally unique and persistent identifier;
F-2. describing data with rich metadata (defined by R1 below);
F-3. clearly and explicitly including the identifier of the data it describes in the metadata;
F-4. registering or indexing (meta)data in a searchable resource.
Accessibility – for data to be accessible requires:
A-1. (meta)data to be retrievable by their identifier using a standardized communications protocol;
A-1.1. the protocol being open, free, and universally implementable;
A-1.2. the protocol allowing for an authentication and authorization procedure, where necessary;
A-2. metadata to be accessible, even when the data are no longer available.
Interoperability – for data to be interoperable it is required that:
I-1. (meta)data use a formal, accessible, shared, and broadly applicable language for knowledge representation;
I-2. (meta)data use vocabularies that follow FAIR principles;
I-3. (meta)data includes qualified references to other (meta)data.
For data to be Reusable they are required:
R-1. meta(data) are richly described with a plurality of accurate and relevant attributes
R-1.1. (meta)data are released with a clear and accessible data usage license
R-1.2. (meta)data are associated with detailed provenance
R-1.3. (meta)data meet domain-relevant community standards.
It is – relatively – easy to see why these principles should apply to datasets, in order for them to be optimally usable for analysis and scientific knowledge discovery. But the text of published articles is also full of claims and assertions, which are ‘data’ in their own right. They have to be recognised and treated as such, and FAIR principles must be applied to these ‘assertional data’ in order for them to be usable in the same way by automated systems as the data in what are more commonly regarded ‘datasets’. It is obvious that narrative text will not easily be ‘directly’ machine readable and actionable. Narrative text is meant, after all, for communication between people. That should remain the case and we should not fall into the trap of trying and creating something like ‘machine readable text’ that is as difficult for humans to read as narrative text is for machines. So, if we regard an article as supplementary to the data contained or referenced in it, and as a piece of ‘metadata’ to those data, it remains a ‘research object’ in its own right and its own metadata can describe its level of FAIRness and define it as ‘narrative’ so machines recognise that text-mining, Natural Language Processing (NLP) – and sometimes even Optical Character Recognition (OCR) before that – will be needed to make it at least partially processable by machines for scientific analysis. FAIR metadata are needed for an article just as for any other research object.
5.Metadata
In the new models supporting FAIR data publications, metadata is key. The most important role of publishers and preprint platforms is to ensure that detailed, domain-specific, and machine-actionable metadata are provided with all publications, including text, datasets, tabular material, and images. In other words, with all ‘research objects’ that they publish. These metadata need to comply with standards that are relevant for the domains and disciplines involved (Principle R-1.3 above). And they need to be properly applied drawing on the controlled vocabularies created by or in close consultation with the practicing domain experts. Some of the metadata components required are already (almost) universally used, such as Digital Object Identifiers (DOIs), although not in all cases in a way that makes them useful for machine-reading. Often enough, a DOI links to a landing page or an abstract, designed to be seen by humans, where the human eye and finger is relied upon to perform the final link through to the object itself, e.g. a PDF. Clearly, that approach is not satisfactory for machine analysis of larger amounts of data and information.
Given the information and data-density of the text in articles, it makes sense to convert assertions into semantically-rich, machine-actionable objects as well, according to the Resource Description Framework (RDF), a family of the World Wide Web Consortium (W3C) specifications [10]. Where possible, assertions that leverage domain-specific vocabularies and RDF markup could be presented as semantic triples along with their appropriate metadata (‘nanopublications’ [11,12]). If all is well, the assertions and claims mentioned in an article’s abstract should capture the gist of the article (at least to the author’s satisfaction), but also tabular data could be treated in this way. The result of the above should be a FAIR Digital Object (FDO), a digital ‘twin’ of the originally submitted research object. It is not suggested that the onus of doing all this should be exclusively on the shoulders of publishers, but instead, should be a collaboration of publishers and (prospective) authors and increasingly, their data stewards. Publishers would provide some of the appropriate tools, and where necessary train (or constrain), authors to indicate what the significant assertions and claims in their articles are. Examples of useful RDF metadata tools that make FAIR publication easy (or at least easier) are available from the Center for Expanded Data Annotation and Retrieval (CEDAR) and the BioPortal repository with more than 800 controlled vocabularies, many from special domains [13,14]. Increasingly CEDAR has been deployed as the primary tooling for the so-called Metadata for Machines workshops, where FAIR metadata experts are teamed up with domain-experts to work together to create appropriate FAIR metadata artefacts [15].
6.Internet of FAIR Data & Services (IFDS)
Given the important role of the material that is provided by scientists via their publications, it is rather obvious that the ability to find, access, interoperate, and thus effectively reuse and combine that material is equally important to the efficiency of scientific progress. It is also obvious that a set of standards and protocols are needed to achieve this, the Internet of FAIR Data & Services [16]. It follows that it would be desirable if academic publishers implemented the protocols and standards to facilitate embedding the material they publish in this IFDS. This would be realised if academic publishers were to form a consortium committed to defining and creating specific materials and tools as generic elements of the IFDS – a FAIR Implementation Network (IN). In various areas of academic research and industrial R&D such an Implementation Network (INs) has already started or is underway to be formed [17]. Discussions, in various stages of progress, are being held about the establishment of further INs (e.g. with representatives of the pharmaceutical and allied industries that are members of the Pistoia Alliance [18]). An Academic Publishing Implementation Network (APIN) with wide participation from publishers would materially strengthen the IFDS, and should be initiated as well. Discussions with GO-FAIR should be undertaken sooner rather than later.
7.What does all this mean for academic publishers?
Much of what is proposed should be possible to incorporate into current processes and workflows. Publishers already add metadata to whatever they publish. Extending this metadata, and increasing its granularity is unlikely to be a fundamental change for most publishers. It speaks for itself that authors will need to be involved in determining what are the ‘significant assertions’ in the text of their articles, and what is the meaning of any terms used. If an author uses the term NLP, for instance, it should not be for the publisher to decide if it means ‘Neuro-Linguistic Programming’ or ‘Natural Language Processing’. There are various technologies available that would enable the realisation of simple and efficient tools for authors to add semantically unequivocal assertions to their manuscripts, in the way that they currently add keywords (although the meaning of those is often enough quite ambiguous, which must be avoided for key assertions).
The publishing process should not differ much from the one currently used, as Figure 1 shows. The main thing is to create (arrange for the creation of) a FAIR ‘twin’ of the original research object as submitted.
Fig. 1.
The flow of research objects through the publishing process.
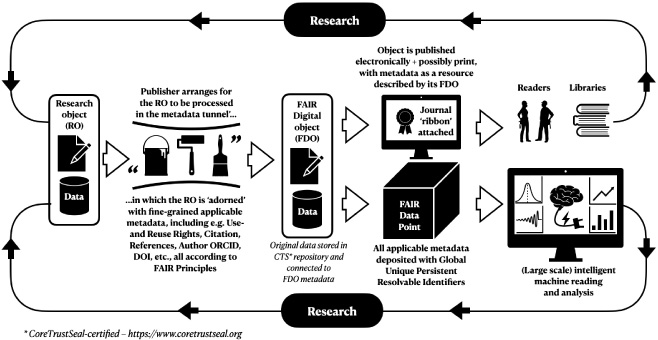
8.Proposed manifesto
For an Academic Publishers’Implementation Network (APIN) to be recognised as a GO-FAIR Implementation Network, a ‘manifesto’ is required. Such a manifesto is meant to articulate critical issues that are of generic importance to the objectives of FAIR, and on which the APIN partners have reached consensus.
The proposed manifesto is as follows, and at this stage is subject to agreement, modification, and acceptance by the initial APIN partners.
The APIN is envisaged to consists of academic publishing outfits of any stripe, be they commercial ones, society publishers, or preprint platforms. Its main purpose is to develop and promote best practices to ‘publish for machines’in alignment with the FAIR principles [19,20]. These best practices would apply to traditional scholarly communication, but with proper addition of machine-readable renditions and components.
All APIN participants commit to comply with the Rules of Engagement of GO FAIR Implementation Networks “Rules of Engagement”, essentially committing to the FAIR Principles and embracing zero tolerance for vendor lock-in formally or otherwise [21].
9.Each APIN partner will
Comply with the FAIR Data Principles: This means that data resources, services, and training materials will be developed according to these principles and will be adorned with rich, machine-readable metadata, and that they will thus be Findable, Accessible, Interoperable, and Reusable under well-defined conditions, by machines and humans.
Abide by the Governance Principles: APIN partners will formally acknowledge and endorse the general governance principles of the GO FAIR initiative [22].
Accept to be stakeholder-governed: The GO FAIR implementation approach for the IFDS is stakeholder-governed. A self-coordinating, board-governed organisation drawn from the stakeholder Implementation Network community creates trust that the organisation will take decisions driven by community consensus, considering different interests.
Accept non-discriminatory membership: When willing to sign the Rules of Engagement, any stakeholder may express an interest in and should be welcome to join GO FAIR.
Conduct transparent operations: Achieving trust in the selection of representatives in governance groups will be best achieved through transparent processes and operations in general (within the constraints of privacy laws).
Targeted Objectives of APIN:
Provide for each narrative article (or element thereof) a machine-resolvable globally unique, persistent and resolvable identifier for semantic artefacts [23].
Provide the same for each major concept in the article (as an extension of the classical ‘keywords’).
Request from, and assist, authors of articles to indicate, and where possible format as machine-readable semantic triples, the main assertions and claims in their original contributions.
Determine, with the guidance of GO-FAIR, which are appropriate tools for publishers to offer to authors to enable them to do what is requested of them in point 3 above.
Request from authors to deposit supplementary material, including large datasets, where possible in machine-readable formats, assist authors who have difficulties doing that themselves, and, where providing the data in the dataset in machine-readable format proves not (yet) possible, provide at least the dataset as such with machine readable metadata.
10.Initial Primary Tasks
Coordinate with the partners in the APIN a detailed plan of execution and a roadmap for further development as soon as the process of becoming a GO FAIR Implementation Network has started.
Develop rules for authors to stimulate proper FAIR data publishing in trusted repositories with a FAIR data point to support maximum machine Findability, Accessibility and Interoperability for Reuse.
References
[1] | LitCovid, a curated literature hub for tracking up-to-date scientific information about the 2019 novel Coronavirus. https://www.ncbi.nlm.nih.gov/research/coronavirus/. |
[2] | The Budapest Open Access Initiative, February 14, 2002, https://www.budapestopenaccessinitiative.org/read. |
[3] | Why Plan S – Open Access is Foundational to the Scientific Enterprise, September 4, 2018, https://www.coalition-s.org/why-plan-s/. |
[4] | Postage Rates for Periodicals: A Narrative History. https://about.usps.com/who-we-are/postal-history/periodicals-postage-history.htm. |
[5] | Outsell Inc. Corporate web site. https://www.outsellinc.com. |
[6] | The Personal Health Train Network. https://pht.health-ri.nl/. |
[7] | The Farm Data Train. https://vimeo.com/215975839. |
[8] | M. Wilkinson, M. Dumontier, I. Aalbersberg , The FAIR Guiding Principles for scientific data management and stewardship, Sci Data 3: ((2016) ), 160018. doi:10.1038/sdata.2016.18. |
[9] | E. Schultes, A role for medical writersin overcoming commonly held misconceptions around FAIR data, Medical Writing 29: (2) ((2020) ), https://journal.emwa.org/the-data-economy/a-role-for-medical-writers-in-overcoming-commonly-held-misconceptions-around-fair-data/. |
[10] | https://en.wikipedia.org/wiki/Resource_Description_Framework. |
[11] | B. Mons and J. Velterop, Nano-Publication in the e-science era – WC3 (https://www.w3.org/) workshop paper, 2009, https://www.w3.org/wiki/images/4/4a/HCLS$$ISWC2009$$Workshop$Mons.pdf. |
[12] | P. Groth, A. Gibson and J. Velterop, The anatomy of a nanopublication, Information Services & Use 30: (1-2) ((2010) ), 51–56, https://content.iospress.com/articles/information-services-and-use/isu613. |
[13] | Center for Expanded Data Annotation and Retrieval (CEDAR), Metadata Center. https://metadatacenter.org/. |
[14] | BioPortal, repository of biomedical ontologies. http://bioportal.bioontology.org. |
[15] | Making FAIR metadata. https://www.go-fair.org/today/making-fair-metadata/. |
[16] | GO-FAIR, The Internet of FAIR Data & Services. https://www.go-fair.org/resources/internet-fair-data-services/. |
[17] | GO-FAIR, Current Implementation Networks. https://www.go-fair.org/implementation-networks/overview/. |
[18] | The Pistoia Alliance, membership list. https://www.pistoiaalliance.org/membership/. |
[19] | Go-FAIR, FAIR Principles. https://www.go-fair.org/fair-principles/. |
[20] | See 5. |
[21] | Go-FAIR, Rules of Engagement. https://www.go-fair.org/resources/rules-of-engagement/. |
[22] | GO-FAIR, Governance. https://www.go-fair.org/go-fair-initiative/governance/. |
[23] | N. Juty, S. M. Wimalaratne, S. Soiland-Reyes, J. Kunze, C. A. Goble and T. Clark, Unique, persistent, resolvable: Identifiers as the foundation of FAIR, Data Intelligence 2: : ((2020) ), 30–39. doi:10.1162/dint_a_00025, http://www.data-intelligence-journal.org/p/31/. |